22.5 Advanced Experimental Designs
22.5.1 Semi-Random Experiments
In semi-random experiments, participants are not fully randomized into treatment and control groups. Instead, a structured randomization process ensures fairness while allowing some level of causal inference.
22.5.1.1 Example: Loan Assignment Fairness
A bank wants to evaluate a new loan approval policy while ensuring that the experiment does not unfairly exclude specific demographics.
To maintain fairness:
- Applicants are first stratified based on income and credit history.
- Within each stratum, a random subset is assigned to receive the new policy, while others continue under the old policy.
set.seed(123)
# Create stratified groups
data <- data.frame(
income_group = rep(c("Low", "Medium", "High"), each = 10),
# Stratified randomization
treatment = sample(rep(c("New Policy", "Old Policy"), each = 15))
)
# Display the stratification results
table(data$income_group, data$treatment)
#>
#> New Policy Old Policy
#> High 6 4
#> Low 6 4
#> Medium 3 7
This approach ensures that each income group is fairly represented in both treatment and control conditions.
22.5.1.2 Case Study: Chicago Open Enrollment Program
A well-known example of semi-random assignment is the Chicago Open Enrollment Program (Cullen, Jacob, and Levitt 2005), where students could apply to choice schools.
However, since many schools were oversubscribed (i.e., demand exceeded supply), they used a random lottery system to allocate spots.
Thus, while enrollment itself was not fully random, the lottery outcomes were random, allowing researchers to estimate the Intent-to-Treat effect while acknowledging that not all students who won the lottery actually enrolled.
This situation presents a classic case where:
- School choice is not random: Families self-select into applying to certain schools.
- Lottery outcomes are random: Among those who apply, winning or losing the lottery is as good as a random assignment.
Let:
- Enrollij=1 if student i enrolls in school j, and 0 otherwise.
- Winij=1 if student i wins the lottery, and 0 otherwise.
- Applyij=1 if student i applies to school j.
We define:
δj=E[Yi|Enrollij=1,Applyij=1]−E[Yi|Enrollij=0,Applyij=1]
θj=E[Yi|Winij=1,Applyij=1]−E[Yi|Winij=0,Applyij=1]
where:
- δj is the treatment effect (impact of actual school enrollment).
- θj is the intent-to-treat effect (impact of winning the lottery).
Since not all winners enroll, we know that:
δj≠θj
Thus, we can only estimate θj directly, and need an additional method to recover δj.
This distinction is crucial because simply comparing lottery winners and losers does not measure the true effect of enrollment—only the effect of being given the opportunity to enroll.
To estimate the treatment effect (δj), we use an Instrumental Variable approach:
δj=E[Yi|Wij=1,Aij=1]−E[Yi|Wij=0,Aij=1]P(Enrollij=1|Wij=1,Aij=1)−P(Enrollij=1|Wij=0,Aij=1)
where:
- P(Enrollij=1|Wij=1,Aij=1) = probability of enrolling if winning the lottery.
- P(Enrollij=1|Wij=0,Aij=1) = probability of enrolling if losing the lottery.
This adjustment accounts for the fact that some lottery winners do not enroll, and thus the observed effect (θj) underestimates the true treatment effect (δj).
This Instrumental Variable approach corrects for selection bias by leveraging randomized lottery assignment.
Numerical Example
Assume 10 students win the lottery and 10 students lose.
For Winners:
Type | Count | Selection Effect | Treatment Effect | Total Effect |
---|---|---|---|---|
Always Takers | 1 | +0.2 | +1 | +1.2 |
Compliers | 2 | 0 | +1 | +1 |
Never Takers | 7 | -0.1 | 0 | -0.1 |
For Losers:
Type | Count | Selection Effect | Treatment Effect | Total Effect |
---|---|---|---|---|
Always Takers | 1 | +0.2 | +1 | +1.2 |
Compliers | 2 | 0 | 0 | 0 |
Never Takers | 7 | -0.1 | 0 | -0.1 |
Computing Intent-to-Treat Effect
We compute the expected outcome for those who won and lost the lottery.
E[Yi|Wij=1,Aij=1]=1(1.2)+2(1)+7(−0.1)10=0.25E[Yi|Wij=0,Aij=1]=1(1.2)+2(0)+7(−0.1)10=0.05
Thus, the Intent-to-Treat Effect is:
Intent-to-Treat Effect=0.25−0.05=0.2
Now, we calculate the probability of enrollment for lottery winners and losers:
P(Enrollij=1|Wij=1,Aij=1)=1+210=0.3P(Enrollij=1|Wij=0,Aij=1)=110=0.1
Using the formula for treatment effect (δ):
Treatment Effect=0.20.3−0.1=1
This confirms that the true treatment effect is 1 unit.
To account for additional factors (Xi), we extend the model as follows:
Yia=δWia+λLia+Xiθ+uia
where:
- δ = Intent-to-Treat effect
- λ = True treatment effect
- W = Whether a student wins the lottery
- L = Whether a student enrolls in the school
- Xiθ = Control variables (i.e., reweighting of lottery), but would not affect treatment effect E(δ)
Since choosing to apply to a lottery is not random, we must consider the following:
E(λ)≠E(λ1)
This demonstrates why lottery-based assignment is a useful but imperfect tool for causal inference—winning the lottery is random, but who applies is not.
22.5.2 Re-Randomization
In standard randomization, baseline covariates are only balanced on average, meaning imbalance can still occur due to random chance. Re-randomization eliminates bad randomizations by checking balance before the experiment begins (Morgan and Rubin 2012).
Key Motivations for Re-Randomization
- Randomization does not guarantee balance:
- Example: For 10 covariates, the probability of at least one imbalance at α=0.05 is:
1−(1−0.05)10=0.40=40%
- This means a high chance of some imbalance across treatment groups.
- Example: For 10 covariates, the probability of at least one imbalance at α=0.05 is:
- Re-randomization increases precision: If covariates correlate with the outcome, improving covariate balance improves treatment effect estimation
- Accounting for re-randomization in inference: Since re-randomization filters out bad assignments, it is equivalent to increasing the sample size and must be considered when computing standard errors.
- Alternative balancing techniques:
- Stratified randomization (Johansson and Schultzberg 2022).
- Matched randomization (Greevy et al. 2004; Kapelner and Krieger 2014).
- Minimization (Pocock and Simon 1975).
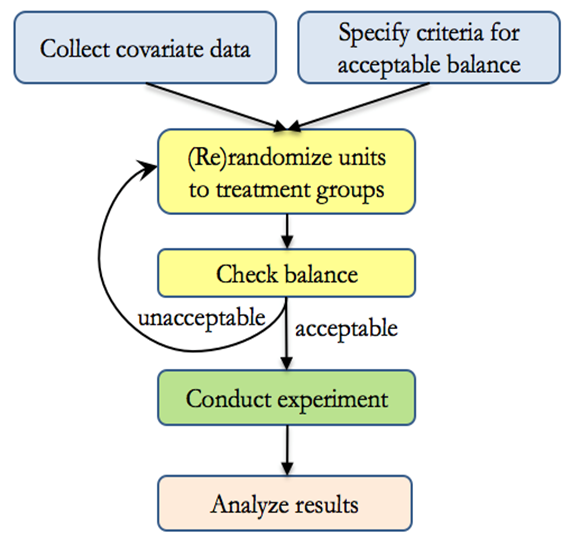
Example: Balancing Experimental Groups
An online retailer is testing two website designs (A and B) but wants to ensure that key customer demographics (e.g., age) are balanced across treatment groups.
We define a balance criterion to check if the mean age difference between groups is acceptable before proceeding.
set.seed(123)
# Define balance criterion: Ensure mean age difference < 1 year
balance_criterion <- function(data) {
abs(mean(data$age[data$group == "A"]) - mean(data$age[data$group == "B"])) < 1
}
# Generate randomized groups, repeat until balance criterion is met
repeat {
data <- data.frame(
age = rnorm(100, mean = 35, sd = 10),
group = sample(c("A", "B"), 100, replace = TRUE)
)
if (balance_criterion(data)) break
}
# Check final group means
tapply(data$age, data$group, mean)
#> A B
#> 35.91079 35.25483
22.5.2.1 Rerandomization Criterion
Re-randomization is based on a function of the covariate matrix (X) and treatment assignments (W).
Wi={1,if treated0,if control
A common approach is to use Mahalanobis Distance to measure covariate balance between treatment and control groups:
M=(ˉXT−ˉXC)′cov(ˉXT−ˉXC)−1(ˉXT−ˉXC)=(1nT+1nC)−1(ˉXT−ˉXC)′cov(X)−1(ˉXT−ˉXC)
where:
- ˉXT and ˉXC are the mean covariate values for treatment and control groups.
- cov(X) is the covariance matrix of the covariates.
- nT and nC are the sample sizes for treatment and control groups.
If the sample size is large and randomization is pure, then M follows a chi-squared distribution:
M∼χ2k
where k is the number of covariates to be balanced.
Choosing the Rerandomization Threshold (M>a)
Define pa as the probability of accepting a randomization:
- Smaller pa → Stronger balance, but longer computation time.
- Larger pa → Faster randomization, but weaker balance.
A rule of thumb is to re-randomize whenever:
M>a
where a is chosen based on acceptable balance thresholds.
We apply Mahalanobis Distance as a balance criterion to ensure that treatment and control groups are well-matched before proceeding with the experiment.
set.seed(123)
library(MASS)
# Generate a dataset with two covariates
n <- 100
X <- mvrnorm(n, mu = c(0, 0), Sigma = matrix(c(1, 0.5, 0.5, 1), 2, 2))
colnames(X) <- c("Covariate1", "Covariate2")
# Balance function using Mahalanobis Distance
balance_criterion <- function(X, group) {
X_treat <- X[group == 1, ]
X_control <- X[group == 0, ]
mean_diff <- colMeans(X_treat) - colMeans(X_control)
cov_inv <- solve(cov(X))
M <- t(mean_diff) %*% cov_inv %*% mean_diff
# Acceptable threshold
# (chi-squared critical value for k = 2, alpha = 0.05)
return(M < 3.84)
}
# Repeat randomization until balance is met
repeat {
group <- sample(c(0, 1), n, replace = TRUE)
if (balance_criterion(X, group)) break
}
# Display final balance check
table(group)
#> group
#> 0 1
#> 50 50
colMeans(X[group == 1, ]) # Treatment group means
#> Covariate1 Covariate2
#> 0.2469635 0.1918521
colMeans(X[group == 0, ]) # Control group means
#> Covariate1 Covariate2
#> 0.01717088 -0.14281124
22.5.3 Two-Stage Randomized Experiments
A two-stage randomized experiment involves sequential interventions, where treatment assignments depend on earlier responses. This design is widely used in:
- Adaptive Learning: Adjusting educational content based on student progress.
- Personalized Advertising: Targeting follow-up ads based on engagement.
- Medical Trials: Adapting treatments based on patient response.
By introducing a second randomization stage, researchers can evaluate:
- The effect of initial treatments.
- The effect of follow-up treatments.
- Potential interactions between the two stages.
22.5.3.1 Example: Personalized Advertising Experiment
A company tests two initial ad campaigns (Ad A vs. Ad B). After observing customer engagement, they apply a second-stage intervention (e.g., Discount vs. No Discount).
The two-stage experiment can be modeled as:
Yijk=μ+αi+βj(i)+ϵijk
where:
- μ = Overall mean outcome (e.g., conversion rate).
- αi = Effect of first-stage intervention (i = Ad A or Ad B).
- βj(i) = Effect of second-stage intervention, nested within first-stage groups.
- ϵijk = Random error term.
The nested structure ensures that the second-stage treatment (βj(i)) is assigned within each first-stage treatment group.
set.seed(123)
# Generate first-stage randomization (Initial Ad)
data <- data.frame(
stage1 = sample(c("Ad A", "Ad B"), 100, replace = TRUE),
stage2 = rep(NA, 100) # Placeholder for second-stage randomization
)
# Second-stage assignment based on first-stage response
data$stage2[data$stage1 == "Ad A"] <-
sample(c("Discount", "No Discount"),
sum(data$stage1 == "Ad A"),
replace = TRUE)
data$stage2[data$stage1 == "Ad B"] <-
sample(c("Discount", "No Discount"),
sum(data$stage1 == "Ad B"),
replace = TRUE)
# Display final randomization
table(data$stage1, data$stage2)
#>
#> Discount No Discount
#> Ad A 24 33
#> Ad B 22 21
This structure ensures:
Fair assignment of initial ads.
Adaptive targeting in the second stage based on user engagement.
22.5.4 Two-Stage Randomized Experiments with Interference and Noncompliance
In real-world experiments, interference and noncompliance complicate analysis (Imai, Jiang, and Malani 2021):
Interference: When treatment effects “spill over” from one group to another (e.g., social influence in marketing).
Noncompliance: When participants do not adhere to their assigned treatment (e.g., a customer ignoring an ad).
To handle noncompliance, we define:
Zik = Assigned treatment (e.g., Ad A or Ad B).
Dik = Actual treatment received (e.g., whether the user actually saw the ad).
Yik = Outcome (e.g., purchase).
A two-stage Instrumental Variable model adjusts for noncompliance:
Dik=γ0+γ1Zik+vikYik=β0+β1Dik+ϵik where:
γ1 measures the effect of assignment on actual treatment received.
β1 estimates the treatment effect, adjusting for noncompliance.
If individuals influence each other’s outcomes, traditional randomization is biased. Solutions include:
Cluster Randomization: Assigning treatments at the group level (e.g., entire social circles receive the same ad).
Partial Interference Models: Assume interference only occurs within predefined groups.
set.seed(123)
library(ivreg) # Load Instrumental Variable Regression Package
# Generate data for first-stage treatment assignment
n <- 500
data <- data.frame(
Z = sample(c(0, 1), n, replace = TRUE), # Initial assignment (randomized)
D = NA, # Actual treatment received (affected by compliance)
Y = NA # Outcome variable (e.g., purchase)
)
# Introduce noncompliance: 80% compliance rate
data$D <- ifelse(runif(n) < 0.8, data$Z, 1 - data$Z)
# Generate outcome variable (Y) with true treatment effect
# True effect of D on Y is 3
data$Y <- 5 + 3 * data$D + rnorm(n, mean = 0, sd = 2)
# Estimate Two-Stage Least Squares (2SLS)
iv_model <- ivreg(Y ~ D | Z, data = data)
summary(iv_model)
#>
#> Call:
#> ivreg(formula = Y ~ D | Z, data = data)
#>
#> Residuals:
#> Min 1Q Median 3Q Max
#> -5.497 -1.344 0.018 1.303 5.493
#>
#> Coefficients:
#> Estimate Std. Error t value Pr(>|t|)
#> (Intercept) 5.1285 0.1861 27.557 <2e-16 ***
#> D 2.7487 0.3072 8.949 <2e-16 ***
#>
#> Diagnostic tests:
#> df1 df2 statistic p-value
#> Weak instruments 1 498 263.54 <2e-16 ***
#> Wu-Hausman 1 497 0.19 0.663
#> Sargan 0 NA NA NA
#> ---
#> Signif. codes: 0 '***' 0.001 '**' 0.01 '*' 0.05 '.' 0.1 ' ' 1
#>
#> Residual standard error: 2.017 on 498 degrees of freedom
#> Multiple R-Squared: 0.2997, Adjusted R-squared: 0.2983
#> Wald test: 80.08 on 1 and 498 DF, p-value: < 2.2e-16
The first-stage regression estimates how strongly Z affects D (compliance).
The second-stage regression estimates the true causal effect of D on Y.
If interference is present, the standard IV method may be biased. Researchers should explore network-based randomization or spatial models.