11 Imputation (Missing Data)
Imputation is a statistical procedure where you replace missing data with some reasonable values
Unit imputation = single data point
Item imputation = single feature value
Imputation is usually seen as the illegitimate child of statistical analysis. Several reasons that contribute to these negative views could be:
- Peopled hardly do imputation correctly (which will introduce bias to your estimates)
- Imputation can only be applied to a small range of problems correctly
If you have missing data on \(y\) (dependent variable), you probably would not be able to do any imputation appropriately. However, if you have certain type of missing data (e.g., non-random missing data) in the \(x\)’s variable (independent variables), then you can still salvage your collected data points with imputation.
We also need to talk why you would want to do imputation in the first place. If your purpose is inference/ explanation (valid statistical inference not optimal point prediction), then imputation would not offer much help (Rubin 1996). However, if your purpose is prediction, you would want your standard error to be reduced by including information (non-missing data) on other variables of a data point. Then imputation could be the tool that you’re looking for.
For most software packages, it will use listwise deletion or casewise deletion to have complete case analysis (analysis with only observations with all information). Not until recently that statistician can propose some methods that are a bit better than listwise deletion which are maximum likelihood and multiple imputation.
“Judging the quality of missing data procedures by their ability to recreate the individual missing values (according to hit rate, mean square error, etc) does not lead to choosing procedures that result in valid inference”, (Rubin 1996)
Missing data can make it more challenging to big datasets.
11.1 Assumptions
11.1.1 Missing Completely at Random (MCAR)
Missing Completely at Random, MCAR, means there is no relationship between the missingness of the data and any values, observed or missing. Those missing data points are a random subset of the data. There is nothing systematic going on that makes some data more likely to be missing than others.
The probability of missing data on a variable is unrelated to the value of it or to the values of any other variables in the data set.
Note: the “missingness” on Y can be correlated with the “missingness” on X We can compare the value of other variables for the observations with missing data, and observations without missing data. If we reject the t-test for mean difference, we can say there is evidence that the data are not MCAR. But we cannot say that our data are MCAR if we fail to reject the t-test.
- the propensity for a data point to be missing is completely random.
- There’s no relationship between whether a data point is missing and any values in the data set, missing or observed.
- The missing data are just a random subset of the data.
Methods include:
Universal singular value thresholding (Chatterjee 2015) (can only recover the mean, not the whole true distribution).
Softimputet: (Hastie et al. 2015) (doesn’t work well under “Limited” -missing not at random).
Synthetic nearest neighbor (Agarwal et al. 2023) (still work okay under missing not at random). Available on GitHub: syntheticNN
11.1.2 Missing at Random (MAR)
Missing at Random, MAR, means there is a systematic relationship between the propensity of missing values and the observed data, but not the missing data. Whether an observation is missing has nothing to do with the missing values, but it does have to do with the values of an individual’s observed variables. So, for example, if men are more likely to tell you their weight than women, weight is MAR.
MAR is weaker than MCAR
\[ P(Y_{missing}|Y,X)= P(Y_{missing}|X) \]
The probability of Y missing given Y and X equal to the probability of of Y missing given X. However, it is impossible to provide evidence to the MAR condition.
-
the propensity for a data point to be missing is not related to the missing data, but it is related to some of the observed data. In another word, there is a systematic relationship between the propensity of missing values and the observed data, but not the missing data.
- For example, if men are more likely to tell you their weight than women, weight is MAR
MAR requires that the cause of the missing data is unrelated to the missing values but may be related to the observed values of other variables.
MAR means that the missing values are related to observed values on other variables. As an example of CD missing data, missing income data may be unrelated to the actual income values but are related to education. Perhaps people with more education are less likely to reveal their income than those with less education
11.1.3 Ignorable
The missing data mechanism is ignorable when
- The data are MAR
- the parameters in the function of the missing data process are unrelated to the parameters (of interest) that need to be estimated.
In this case, you actually don’t need to model the missing data mechanisms unless you would like to improve on your accuracy, in which case you still need to be very rigorous about your approach to improve efficiency in your parameters.
11.1.4 Nonignorable
Missing Not at Random, MNAR, means there is a relationship between the propensity of a value to be missing and its values.
Example: people with the lowest education are missing on education or the sickest people are most likely to drop out of the study.
MNAR is called Nonignorable because the missing data mechanism itself has to be modeled as you deal with the missing data. You have to include some model for why the data are missing and what the likely values are.
Hence, in the case of nonignorable, the data are not MAR. Then, your parameters of interest will be biased if you do not model the missing data mechanism. One of the most widely used approach for nonignorable missing data is (James J. Heckman 1976)
-
Another name: Missing Not at Random (MNAR): there is a relationship between the propensity of a value to be missing and its values
- For example, people with low education will be less likely to report it
We need to model why the data are missing and what the likely values are.
the missing data mechanism is related to the missing values
It commonly occurs when people do not want to reveal something very personal or unpopular about themselves
Complete case analysis can give highly biased results for NI missing data. If proportionally more low and moderate income individuals are left in the sample because high income people are missing, an estimate of the mean income will be lower than the actual population mean.
One can use instrument that can predict the nonresponse process in outcome variable, and unrelated to the outcome of the population to correct for this missingness (but you still have to use complete cases) (B. Sun et al. 2018; E. J. Tchetgen Tchetgen and Wirth 2017)
11.2 Solutions to Missing data
11.2.1 Listwise Deletion
Also known as complete case deletion only where you only retain cases with complete data for all features.
Advantages:
Can be applied to any statistical test (SEM, multi-level regression, etc.)
In the case of MCAR, both the parameters estimates and its standard errors are unbiased.
-
In the case of MAR among independent variables (not depend on the values of dependent variables), then listwise deletion parameter estimates can still be unbiased. (Little 1992) For example, you have a model \(y=\beta_{0}+\beta_1X_1 + \beta_2X_2 +\epsilon\) if the probability of missing data on \(X1\) is independent of \(Y\), but dependent on the value of \(X1\) and \(X2\), then the model estimates are still unbiased.
- The missing data mechanism the depends on the values of the independent variables are the same as stratified sampling. And stratified sampling does not bias your estimates
- In the case of logistic regression, if the probability of missing data on any variable depends on the value of the dependent variable, but independent of the value of the independent variables, then the listwise deletion will yield biased intercept estimate, but consistent estimates of the slope and their standard errors (Vach and Vach 1994). However, logistic regression will still fail if the probability of missing data is dependent on both the value of the dependent and independent variables.
- Under regression analysis, listwise deletion is more robust than maximum likelihood and multiple imputation when MAR assumption is violated.
Disadvantages:
- It will yield a larger standard errors than other more sophisticated methods discussed later.
- If the data are not MCAR, but MAR, then your listwise deletion can yield biased estimates.
- In other cases than regression analysis, other sophisticated methods can yield better estimates compared to listwise deletion.
11.2.2 Pairwise Deletion
This method could only be used in the case of linear models such as linear regression, factor analysis, or SEM. The premise of this method based on that the coefficient estimates are calculated based on the means, standard deviations, and correlation matrix. Compared to listwise deletion, we still utilized as many correlation between variables as possible to compute the correlation matrix.
Advantages:
If the true missing data mechanism is MCAR, pair wise deletion will yield consistent estimates, and unbiased in large samples
-
Compared to listwise deletion: (Glasser 1964)
- If the correlation among variables are low, pairwise deletion is more efficient estimates than listwise
- If the correlations among variables are high, listwise deletion is more efficient than pairwise.
Disadvantages:
- If the data mechanism is MAR, pairwise deletion will yield biased estimates.
- In small sample, sometimes covariance matrix might not be positive definite, which means coefficients estimates cannot be calculated.
Note: You need to read carefully on how your software specify the sample size because it will alter the standard errors.
11.2.3 Dummy Variable Adjustment
Also known as Missing Indicator Method or Proxy Variable
Add another variable in the database to indicate whether a value is missing.
Create 2 variables
\[ D= \begin{cases} 1 & \text{data on X are missing} \\ 0 & \text{otherwise}\\ \end{cases} \]
\[ X^* = \begin{cases} X & \text{data are available} \\ c & \text{data are missing}\\ \end{cases} \]
Note: A typical choice for \(c\) is usually the mean of \(X\)
Interpretation:
- Coefficient of \(D\) is the the difference in the expected value of \(Y\) between the group with data and the group without data on \(X\).
- Coefficient of \(X^*\) is the effect of the group with data on \(Y\)
Disadvantages:
- This method yields biased estimates of the coefficient even in the case of MCAR (Jones 1996)
11.2.4 Imputation
11.2.4.1 Mean, Mode, Median Imputation
-
Bad:
- Mean imputation does not preserve the relationships among variables
- Mean imputation leads to An Underestimate of Standard Errors → you’re making Type I errors without realizing it.
- Biased estimates of variances and covariances (Haitovsky 1968)
- In high-dimensions, mean substitution cannot account for dependence structure among features.
11.2.4.2 Maximum Likelihood
When missing data are MAR and monotonic (such as in the case of panel studies), ML can be adequately in estimating coefficients.
Monotonic means that if you are missing data on X1, then that observation also has missing data on all other variables that come after it.
ML can generally handle linear models, log-linear model, but beyond that, ML still lacks both theory and software to implement.
11.2.4.2.1 Expectation-Maximization Algorithm (EM Algorithm)
An iterative process:
- Other variables are used to impute a value (Expectation).
- Check whether the value is most likely (Maximization).
- If not, it re-imputes a more likely value.
You start your regression with your estimates based on either listwise deletion or pairwise deletion. After regressing missing variables on available variables, you obtain a regression model. Plug the missing data back into the original model, with modified variances and covariances For example, if you have missing data on \(X_{ij}\) you would regress it on available data of \(X_{i(j)}\), then plug the expected value of \(X_{ij}\) back with its \(X_{ij}^2\) turn into \(X_{ij}^2 + s_{j(j)}^2\) where \(s_{j(j)}^2\) stands for the residual variance from regressing \(X_{ij}\) on \(X_{i(j)}\) With the new estimated model, you rerun the process until the estimates converge.
Advantages:
- Easy to use
- Preserves the relationship with other variables (important if you use Factor Analysis or Linear Regression later on), but best in the case of Factor Analysis, which doesn’t require standard error of individuals item.
Disadvantages:
- Standard errors of the coefficients are incorrect (biased usually downward - underestimate)
- Models with overidentification, the estimates will not be efficient
11.2.4.3 Multiple Imputation
MI is designed to use “the Bayesian model-based approach to create procedures, and the frequentist (randomization-based approach) to evaluate procedures”. (Rubin 1996)
MI estimates have the same properties as ML when the data is MAR
- Consistent
- Asymptotically efficient
- Asymptotically normal
MI can be applied to any type of model, unlike Maximum Likelihood that is only limited to a small set of models.
A drawback of MI is that it will produce slightly different estimates every time you run it. To avoid such problem, you can set seed when doing your analysis to ensure its reproducibility.
11.2.4.3.1 Single Random Imputation
Random draws form the residual distribution of each imputed variable and add those random numbers to the imputed values.
For example, if we have missing data on \(X\), and it’s MCAR, then
Regress \(X\) on \(Y\) (Listwise Deletion method) to get its residual distribution.
-
For every missing value on X, we substitute with \(\tilde{x_i}=\hat{x_i} + \rho u_i\) where
- \(u_i\) is a random draw from a standard normal distribution
- \(x_i\) is the predicted value from the regression of X and Y
- \(\rho\) is the standard deviation of the residual distribution of X regressed on Y.
However, the model you run with the imputed data still thinks that your data are collected, not imputed, which leads your standard error estimates to be too low and test statistics too high.
To address this problem, we need to repeat the imputation process which leads us to repeated imputation or multiple random imputation.
11.2.4.3.2 Repeated Imputation
“Repeated imputations are draws from the posterior predictive distribution of the missing values under a specific model , a particular Bayesian model for both the data and the missing mechanism”.(Rubin 1996)
Repeated imputation, also known as, multiple random imputation, allows us to have multiple “completed” data sets. The variability across imputations will adjust the standard errors upward.
The estimate of the standard error of \(\bar{r}\) (mean correlation estimates between X and Y) is \[ SE(\bar{r})=\sqrt{\frac{1}{M}\sum_{k}s_k^2+ (1+\frac{1}{M})(\frac{1}{M-1})\sum_{k}(r_k-\bar{r})^2} \] where M is the number of replications, \(r_k\) is the the correlation in replication k, \(s_k\) is the estimated standard error in replication k.
However, this method still considers the parameter in predicting \(\tilde{x}\) is still fixed, which means we assume that we are using the true parameters to predict \(\tilde{x}\). To overcome this challenge, we need to introduce variability into our model for \(\tilde{x}\) by treating the parameters as a random variables and use Bayesian posterior distribution of the parameters to predict the parameters.
However, if your sample is large and the proportion of missing data is small, the extra Bayesian step might not be necessary. If your sample is small or the proportion of missing data is large, the extra Bayesian step is necessary.
Two algorithms to get random draws of the regression parameters from its posterior distribution:
- Data Augmentation
- Sampling importance/resampling (SIR)
Authors have argued for SIR superiority due to its computer time (G. King et al. 2001)
11.2.4.3.2.1 Data Augmentation
Steps for data augmentation:
- Choose starting values for the parameters (e.g., for multivariate normal, choose means and covariance matrix). These values can come from previous values, expert knowledge, or from listwise deletion or pairwise deletion or EM estimation.
- Based on the current values of means and covariances calculate the coefficients estimates for the equation that variable with missing data is regressed on all other variables (or variables that you think will help predict the missing values, could also be variables that are not in the final estimation model)
- Use the estimates in step (2) to predict values for missing values. For each predicted value, add a random error from the residual normal distribution for that variable.
- From the “complete” data set, recalculate the means and covariance matrix. And take a random draw from the posterior distribution of the means and covariances with Jeffreys’ prior.
- Using the random draw from step (4), repeat step (2) to (4) until the means and covariances stabilize (converged).
The iterative process allows us to get random draws from the joint posterior distribution of both data nd parameters, given the observed data.
Rules of thumb regarding convergence:
- The higher the proportion of missing, the more iterations
- the rate of convergence for EM algorithm should be the minimum threshold for DA.
- You can also check if your distribution has been converged by diagnostic statistics Can check Bayesian Diagnostics for some introduction.
Types of chains
-
Parallel: Run a separate chain of iterations for each of data set. Different starting values are encouraged. For example, one could use bootstrap to generate different data set with replacement, and for each data set, calculate the starting values by EM estimates.
- Pro: Run faster, and less likely to have dependence in the resulting data sets.
- Con: Sometimes it will not converge
-
Sequential one long chain of data augmentation cycles. After burn-in and thinning, you will have to data sets
- Pro: Converged to the true posterior distribution is more likely.
- Con: The resulting data sets are likely to be dependent. Remedies can be thinning and burn-in.
Note on Non-normal or categorical data The normal-based methods still work well, but you will need to do some transformation. For example,
- If the data is skewed, then log-transform, then impute the exponentiate to have the missing data back to its original metric.
- If the data is proportion, logit-transform, impute, then de-transform the missing data.
If you want to impute non-linear relationship, such as interaction between 2 variables and 1 variable is categorical. You can do separate imputation for different levels of that variable separately, then combined for the final analysis.
- If all variables that have missing data are categorical, then unrestricted multinomial model or log-linear model is recommended.
- If a single categorical variable, logistic (logit) regression would be sufficient.
11.2.4.4 Nonparametric/ Semiparametric Methods
11.2.4.4.1 Hot Deck Imputation
- Used by U.S. Census Bureau for public datasets
- approximate Bayesian bootstrap
- A randomly chosen value from an individual in the sample who has similar values on other variables. In other words, find all the sample subjects who are similar on other variables, then randomly choose one of their values on the missing variable.
When we have \(n_1\) cases with complete data on \(Y\) and \(n_0\) cases with missing data on \(Y\)
- Step 1: From \(n_1\), take a random sample (with replacement) of \(n_1\) cases
- Step 2: From the retrieved sample take a random sample (with replacement) of \(n_0\) cases
- Step 3: Assign the \(n_0\) cases in step 2 to \(n_0\) missing data cases.
- Step 4: Repeat the process for every variable.
- Step 5: For multiple imputation, repeat the four steps multiple times.
Note:
If we skip step 1, it reduce variability for estimating standard errors.
-
Good:
- Constrained to only possible values.
- Since the value is picked at random, it adds some variability, which might come in handy when calculating standard errors.
Challenge: how can you define “similar” here.
11.2.4.4.2 Cold Deck Imputation
Contrary to Hot Deck, Cold Deck choose value systematically from an observation that has similar values on other variables, which remove the random variation that we want.
Donor samples of “cold-deck” imputation come from a different data set.
11.2.4.4.3 Predictive Mean Matching
Steps:
- Regress \(Y\) on \(X\) (matrix of covariates) for the \(n_1\) (i.e., non-missing cases) to get coefficients \(b\) (a \(k \times 1\) vector) and residual variance estimates \(s^2\)
- Draw randomly from the posterior predictive distribution of the residual variance (assuming a noninformative prior) by calculating \(\frac{(n_1-k)s^2}{\chi^2}\), where \(\chi^2\) is a random draw from a \(\chi^2_{n_1-k}\) and let \(s^2_{[1]}\) be an i-th random draw
- Randomly draw from the posterior distribution of the coefficients \(b\), by drawing from \(MVN(b, s^2_{[1]}(X'X)^{-1})\), where X is an \(n_1 \times k\) matrix of X values. Then we have \(b_{1}\)
- Using step 1, we can calculate standardized residuals for \(n_1\) cases: \(e_i = \frac{y_i - bx_i}{\sqrt{s^2(1-k/n_1)}}\)
- Randomly draw a sample (with replacement) of \(n_0\) from the \(n_1\) residuals in step 4
- With \(n_0\) cases, we can calculate imputed values of \(Y\): \(y_i = b_{[1]}x_i + s_{[1]}e_i\) where \(e_i\) are taken from step 5, and \(b_{[1]}\) taken from step 3, and \(s_{[1]}\) taken from step 2.
- Repeat steps 2 through 6 except for step 4.
Notes:
- can be used for multiple variables where each variable is imputed using all other variables as predictor.
- can also be used for heteroskedasticity in imputed values.
Example from Statistics Globe
set.seed(918273) # Seed
N <- 3000 # Sample size
y <- round(runif(N,-10, 10)) # Target variable Y
x1 <- y + round(runif(N, 0, 50)) # Auxiliary variable 1
x2 <- round(y + 0.25 * x1 + rnorm(N,-3, 15)) # Auxiliary variable 2
x3 <- round(0.1 * x1 + rpois(N, 2)) # Auxiliary variable 3
# (categorical variable)
x4 <- as.factor(round(0.02 * y + runif(N))) # Auxiliary variable 4
# Insert 20% missing data in Y
y[rbinom(N, 1, 0.2) == 1] <- NA
data <- data.frame(y, x1, x2, x3, x4) # Store data in dataset
head(data) # First 6 rows of our data
#> y x1 x2 x3 x4
#> 1 8 38 -3 6 1
#> 2 1 50 -9 5 0
#> 3 5 43 20 5 1
#> 4 NA 9 13 3 0
#> 5 -4 40 -10 6 0
#> 6 NA 29 -6 5 1
library("mice") # Load mice package
##### Impute data via predictive mean matching (single imputation)#####
imp_single <- mice(data, m = 1, method = "pmm") # Impute missing values
#>
#> iter imp variable
#> 1 1 y
#> 2 1 y
#> 3 1 y
#> 4 1 y
#> 5 1 y
data_imp_single <- complete(imp_single) # Store imputed data
# head(data_imp_single)
# Since single imputation underestiamtes stnadard errors,
# we use multiple imputaiton
##### Predictive mean matching (multiple imputation) #####
# Impute missing values multiple times
imp_multi <- mice(data, m = 5, method = "pmm")
#>
#> iter imp variable
#> 1 1 y
#> 1 2 y
#> 1 3 y
#> 1 4 y
#> 1 5 y
#> 2 1 y
#> 2 2 y
#> 2 3 y
#> 2 4 y
#> 2 5 y
#> 3 1 y
#> 3 2 y
#> 3 3 y
#> 3 4 y
#> 3 5 y
#> 4 1 y
#> 4 2 y
#> 4 3 y
#> 4 4 y
#> 4 5 y
#> 5 1 y
#> 5 2 y
#> 5 3 y
#> 5 4 y
#> 5 5 y
data_imp_multi_all <-
# Store multiply imputed data
complete(imp_multi,
"repeated",
include = TRUE)
data_imp_multi <-
# Combine imputed Y and X1-X4 (for convenience)
data.frame(data_imp_multi_all[, 1:6], data[, 2:5])
head(data_imp_multi)
#> y.0 y.1 y.2 y.3 y.4 y.5 x1 x2 x3 x4
#> 1 8 8 8 8 8 8 38 -3 6 1
#> 2 1 1 1 1 1 1 50 -9 5 0
#> 3 5 5 5 5 5 5 43 20 5 1
#> 4 NA 1 -2 -4 9 -8 9 13 3 0
#> 5 -4 -4 -4 -4 -4 -4 40 -10 6 0
#> 6 NA 4 7 7 6 0 29 -6 5 1
Example from UCLA Statistical Consulting
library(mice)
library(VIM)
library(lattice)
library(ggplot2)
## set observations to NA
anscombe <- within(anscombe, {
y1[1:3] <- NA
y4[3:5] <- NA
})
## view
head(anscombe)
#> x1 x2 x3 x4 y1 y2 y3 y4
#> 1 10 10 10 8 NA 9.14 7.46 6.58
#> 2 8 8 8 8 NA 8.14 6.77 5.76
#> 3 13 13 13 8 NA 8.74 12.74 NA
#> 4 9 9 9 8 8.81 8.77 7.11 NA
#> 5 11 11 11 8 8.33 9.26 7.81 NA
#> 6 14 14 14 8 9.96 8.10 8.84 7.04
## check missing data patterns
md.pattern(anscombe)
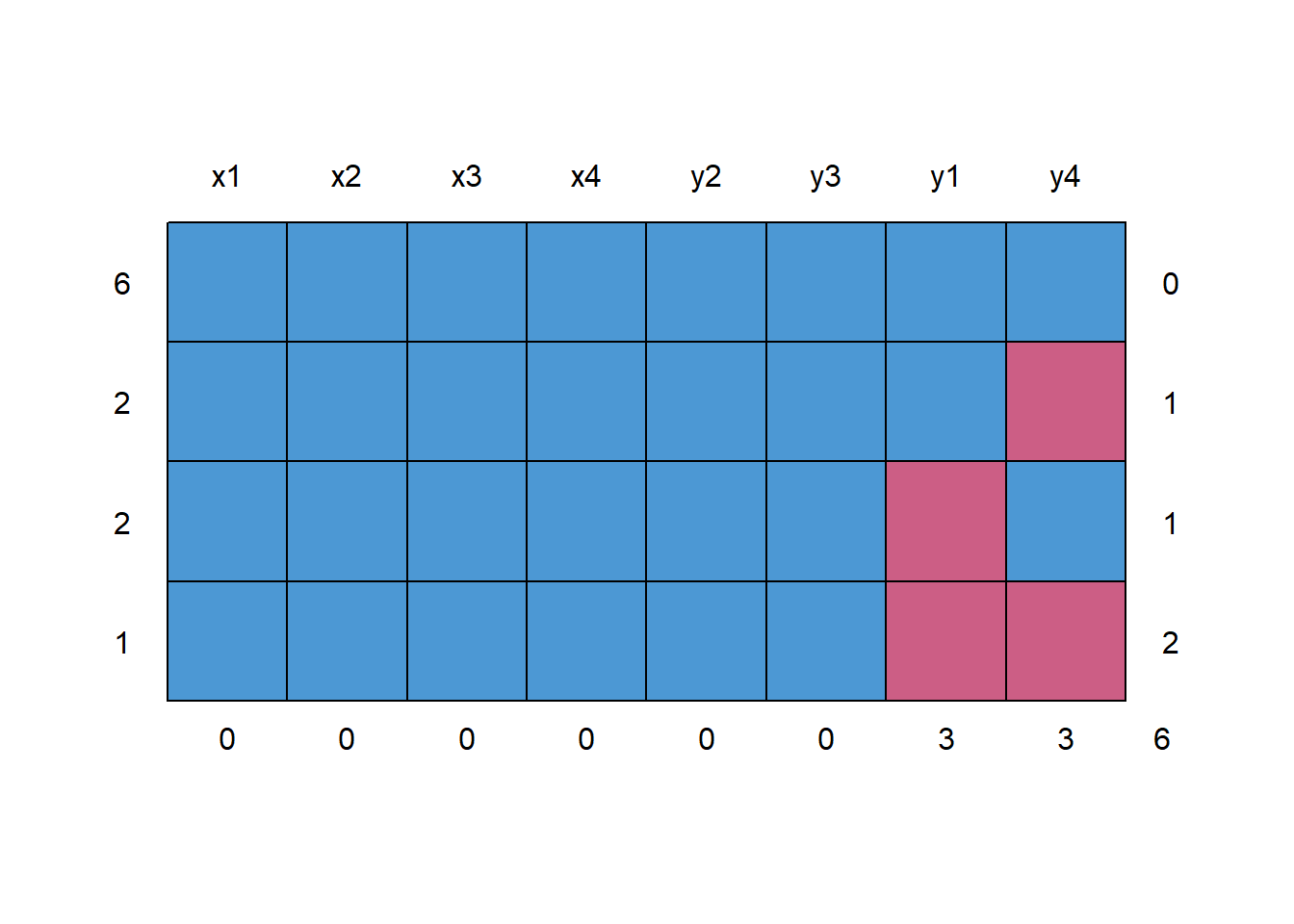
#> x1 x2 x3 x4 y2 y3 y1 y4
#> 6 1 1 1 1 1 1 1 1 0
#> 2 1 1 1 1 1 1 1 0 1
#> 2 1 1 1 1 1 1 0 1 1
#> 1 1 1 1 1 1 1 0 0 2
#> 0 0 0 0 0 0 3 3 6
## Number of observations per patterns for all pairs of variables
p <- md.pairs(anscombe)
p
#> $rr
#> x1 x2 x3 x4 y1 y2 y3 y4
#> x1 11 11 11 11 8 11 11 8
#> x2 11 11 11 11 8 11 11 8
#> x3 11 11 11 11 8 11 11 8
#> x4 11 11 11 11 8 11 11 8
#> y1 8 8 8 8 8 8 8 6
#> y2 11 11 11 11 8 11 11 8
#> y3 11 11 11 11 8 11 11 8
#> y4 8 8 8 8 6 8 8 8
#>
#> $rm
#> x1 x2 x3 x4 y1 y2 y3 y4
#> x1 0 0 0 0 3 0 0 3
#> x2 0 0 0 0 3 0 0 3
#> x3 0 0 0 0 3 0 0 3
#> x4 0 0 0 0 3 0 0 3
#> y1 0 0 0 0 0 0 0 2
#> y2 0 0 0 0 3 0 0 3
#> y3 0 0 0 0 3 0 0 3
#> y4 0 0 0 0 2 0 0 0
#>
#> $mr
#> x1 x2 x3 x4 y1 y2 y3 y4
#> x1 0 0 0 0 0 0 0 0
#> x2 0 0 0 0 0 0 0 0
#> x3 0 0 0 0 0 0 0 0
#> x4 0 0 0 0 0 0 0 0
#> y1 3 3 3 3 0 3 3 2
#> y2 0 0 0 0 0 0 0 0
#> y3 0 0 0 0 0 0 0 0
#> y4 3 3 3 3 2 3 3 0
#>
#> $mm
#> x1 x2 x3 x4 y1 y2 y3 y4
#> x1 0 0 0 0 0 0 0 0
#> x2 0 0 0 0 0 0 0 0
#> x3 0 0 0 0 0 0 0 0
#> x4 0 0 0 0 0 0 0 0
#> y1 0 0 0 0 3 0 0 1
#> y2 0 0 0 0 0 0 0 0
#> y3 0 0 0 0 0 0 0 0
#> y4 0 0 0 0 1 0 0 3
-
rr
= number of observations where both pairs of values are observed -
rm
= the number of observations where both variables are missing values -
mr
= the number of observations where the first variable’s value (e.g. the row variable) is observed and second (or column) variable is missing -
mm
= the number of observations where the second variable’s value (e.g. the col variable) is observed and first (or row) variable is missing
## Margin plot of y1 and y4
marginplot(anscombe[c(5, 8)], col = c("blue", "red", "orange"))
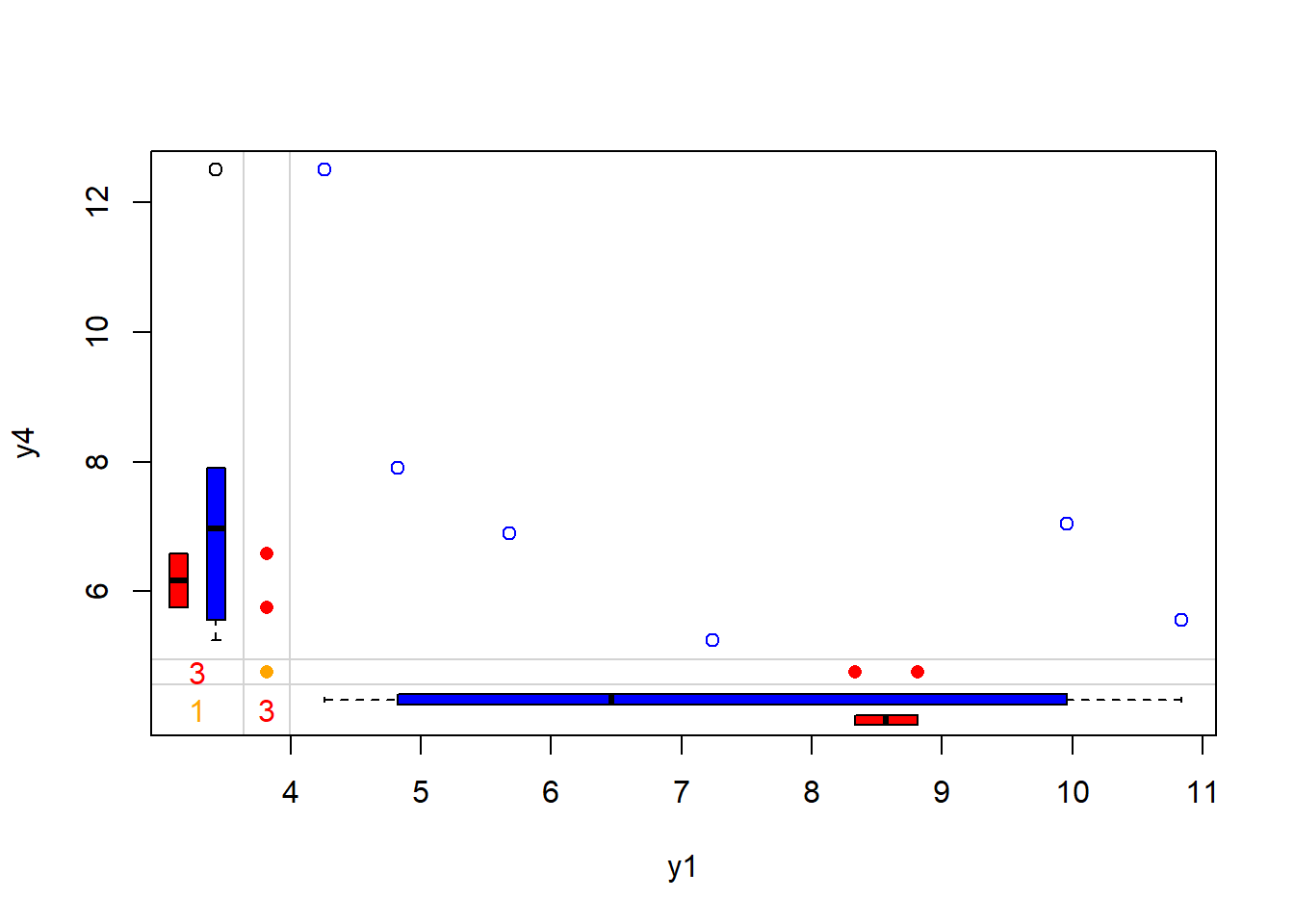
## 5 imputations for all missing values
imp1 <- mice(anscombe, m = 5)
#>
#> iter imp variable
#> 1 1 y1 y4
#> 1 2 y1 y4
#> 1 3 y1 y4
#> 1 4 y1 y4
#> 1 5 y1 y4
#> 2 1 y1 y4
#> 2 2 y1 y4
#> 2 3 y1 y4
#> 2 4 y1 y4
#> 2 5 y1 y4
#> 3 1 y1 y4
#> 3 2 y1 y4
#> 3 3 y1 y4
#> 3 4 y1 y4
#> 3 5 y1 y4
#> 4 1 y1 y4
#> 4 2 y1 y4
#> 4 3 y1 y4
#> 4 4 y1 y4
#> 4 5 y1 y4
#> 5 1 y1 y4
#> 5 2 y1 y4
#> 5 3 y1 y4
#> 5 4 y1 y4
#> 5 5 y1 y4
## linear regression for each imputed data set - 5 regression are run
fitm <- with(imp1, lm(y1 ~ y4 + x1))
summary(fitm)
#> # A tibble: 15 × 6
#> term estimate std.error statistic p.value nobs
#> <chr> <dbl> <dbl> <dbl> <dbl> <int>
#> 1 (Intercept) 8.60 2.67 3.23 0.0121 11
#> 2 y4 -0.533 0.251 -2.12 0.0667 11
#> 3 x1 0.334 0.155 2.16 0.0628 11
#> 4 (Intercept) 4.19 2.93 1.43 0.190 11
#> 5 y4 -0.213 0.273 -0.782 0.457 11
#> 6 x1 0.510 0.167 3.05 0.0159 11
#> 7 (Intercept) 6.51 2.35 2.77 0.0244 11
#> 8 y4 -0.347 0.215 -1.62 0.145 11
#> 9 x1 0.395 0.132 3.00 0.0169 11
#> 10 (Intercept) 5.48 3.02 1.81 0.107 11
#> 11 y4 -0.316 0.282 -1.12 0.295 11
#> 12 x1 0.486 0.173 2.81 0.0230 11
#> 13 (Intercept) 7.12 1.81 3.92 0.00439 11
#> 14 y4 -0.436 0.173 -2.53 0.0355 11
#> 15 x1 0.425 0.102 4.18 0.00308 11
## pool coefficients and standard errors across all 5 regression models
pool(fitm)
#> Class: mipo m = 5
#> term m estimate ubar b t dfcom df
#> 1 (Intercept) 5 6.3808015 6.72703243 2.785088109 10.06913816 8 3.902859
#> 2 y4 5 -0.3690455 0.05860053 0.014674911 0.07621042 8 4.716160
#> 3 x1 5 0.4301588 0.02191260 0.004980516 0.02788922 8 4.856052
#> riv lambda fmi
#> 1 0.4968172 0.3319158 0.5254832
#> 2 0.3005074 0.2310693 0.4303733
#> 3 0.2727480 0.2142985 0.4143230
## output parameter estimates
summary(pool(fitm))
#> term estimate std.error statistic df p.value
#> 1 (Intercept) 6.3808015 3.1731905 2.010847 3.902859 0.11643863
#> 2 y4 -0.3690455 0.2760624 -1.336819 4.716160 0.24213491
#> 3 x1 0.4301588 0.1670007 2.575791 4.856052 0.05107581
11.2.4.4.4 Stochastic Imputation
Regression imputation + random residual = Stochastic Imputation
Most multiple imputation is based off of some form of stochastic regression imputation.
Good:
- Has all the advantage of Regression Imputation
- and also has the random components
Bad:
- might lead to implausible values (e.g. negative values)
- can’t handle heteroskadastic data
Note
Multiple Imputation usually based on some form of stochastic regression imputation.
# Income data
set.seed(91919) # Set seed
N <- 1000 # Sample size
income <- round(rnorm(N, 0, 500)) # Create some synthetic income data
income[income < 0] <- income[income < 0] * (- 1)
x1 <- income + rnorm(N, 1000, 1500) # Auxiliary variables
x2 <- income + rnorm(N, - 5000, 2000)
income[rbinom(N, 1, 0.1) == 1] <- NA # Create 10% missingness in income
data_inc_miss <- data.frame(income, x1, x2)
Single stochastic regression imputation
imp_inc_sri <- mice(data_inc_miss, method = "norm.nob", m = 1)
#>
#> iter imp variable
#> 1 1 income
#> 2 1 income
#> 3 1 income
#> 4 1 income
#> 5 1 income
data_inc_sri <- complete(imp_inc_sri)
Single predictive mean matching
imp_inc_pmm <- mice(data_inc_miss, method = "pmm", m = 1)
#>
#> iter imp variable
#> 1 1 income
#> 2 1 income
#> 3 1 income
#> 4 1 income
#> 5 1 income
data_inc_pmm <- complete(imp_inc_pmm)
Stochastic regression imputation contains negative values
data_inc_sri$income[data_inc_sri$income < 0]
#> [1] -66.055957 -96.980053 -28.921432 -4.175686 -54.480798 -27.207102
#> [7] -143.603500 -80.960488
# No values below 0
data_inc_pmm$income[data_inc_pmm$income < 0]
#> numeric(0)
Evidence for heteroskadastic data
# Heteroscedastic data
set.seed(654654) # Set seed
N <- 1:5000 # Sample size
a <- 0
b <- 1
sigma2 <- N^2
eps <- rnorm(N, mean = 0, sd = sqrt(sigma2))
y <- a + b * N + eps # Heteroscedastic variable
x <- 30 * N + rnorm(N[length(N)], 1000, 200) # Correlated variable
y[rbinom(N[length(N)], 1, 0.3) == 1] <- NA # 30% missing
data_het_miss <- data.frame(y, x)
Single stochastic regression imputation
imp_het_sri <- mice(data_het_miss, method = "norm.nob", m = 1)
#>
#> iter imp variable
#> 1 1 y
#> 2 1 y
#> 3 1 y
#> 4 1 y
#> 5 1 y
data_het_sri <- complete(imp_het_sri)
Single predictive mean matching
imp_het_pmm <- mice(data_het_miss, method = "pmm", m = 1)
#>
#> iter imp variable
#> 1 1 y
#> 2 1 y
#> 3 1 y
#> 4 1 y
#> 5 1 y
data_het_pmm <- complete(imp_het_pmm)
Comparison between predictive mean matching and stochastic regression imputation
par(mfrow = c(1, 2)) # Both plots in one graphic
# Plot of observed values
plot(x[!is.na(data_het_sri$y)],
data_het_sri$y[!is.na(data_het_sri$y)],
main = "",
xlab = "X",
ylab = "Y")
# Plot of missing values
points(x[is.na(y)], data_het_sri$y[is.na(y)],
col = "red")
# Title of plot
title("Stochastic Regression Imputation",
line = 0.5)
# Regression line
abline(lm(y ~ x, data_het_sri),
col = "#1b98e0", lwd = 2.5)
# Legend
legend(
"topleft",
c("Observed Values", "Imputed Values", "Regression Y ~ X"),
pch = c(1, 1, NA),
lty = c(NA, NA, 1),
col = c("black", "red", "#1b98e0")
)
# Plot of observed values
plot(x[!is.na(data_het_pmm$y)],
data_het_pmm$y[!is.na(data_het_pmm$y)],
main = "",
xlab = "X",
ylab = "Y")
# Plot of missing values
points(x[is.na(y)], data_het_pmm$y[is.na(y)],
col = "red")
# Title of plot
title("Predictive Mean Matching",
line = 0.5)
abline(lm(y ~ x, data_het_pmm),
col = "#1b98e0", lwd = 2.5)
# Legend
legend(
"topleft",
c("Observed Values", "Imputed Values", "Regression Y ~ X"),
pch = c(1, 1, NA),
lty = c(NA, NA, 1),
col = c("black", "red", "#1b98e0")
)
mtext(
"Imputation of Heteroscedastic Data",
# Main title of plot
side = 3,
line = -1.5,
outer = TRUE,
cex = 2
)
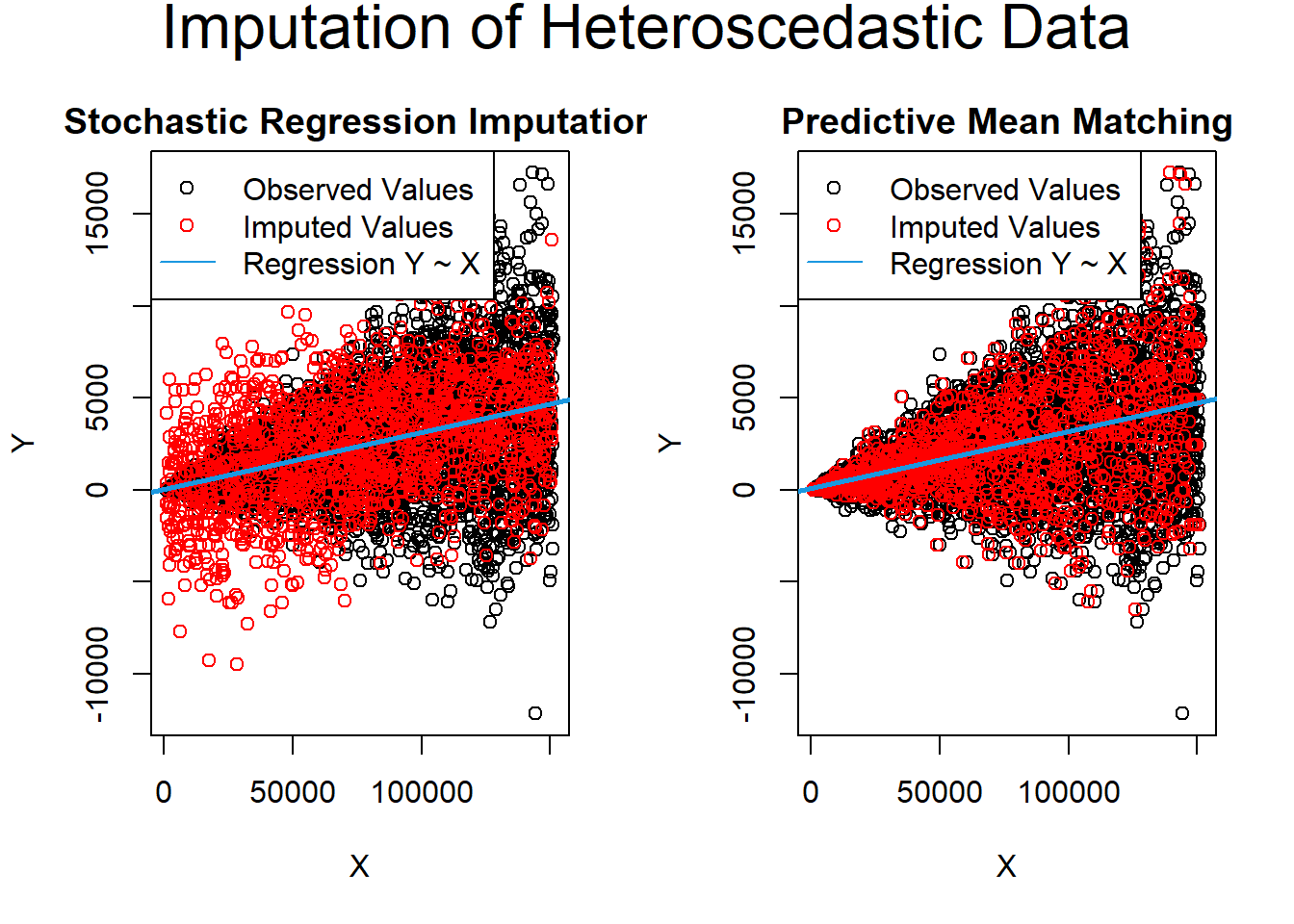
11.2.4.5 Regression Imputation
Also known as conditional mean imputation Missing value is based (regress) on other variables.
-
Good:
Maintain the relationship with other variables (i.e., preserve dependence structure among features, unlike 11.2.4.1).
-
If the data are MCAR, least-squares coefficients estimates will be consistent, and approximately unbiased in large samples (Gourieroux and Monfort 1981)
- Can have improvement on efficiency by using weighted least squares (Beale and Little 1975) or generalized least squares (Gourieroux and Monfort 1981).
-
Bad:
- No variability left. treated data as if they were collected.
- Underestimate the standard errors and overestimate test statistics
11.2.4.6 Interpolation and Extrapolation
An estimated value from other observations from the same individual. It usually only works in longitudinal data.
11.2.4.7 K-nearest neighbor (KNN) imputation
The above methods are model-based imputation (regression).
This is an example of neighbor-based imputation (K-nearest neighbor).
For every observation that needs to be imputed, the algorithm identifies ‘k’ closest observations based on some types distance (e.g., Euclidean) and computes the weighted average (weighted based on distance) of these ‘k’ obs.
For a discrete variable, it uses the most frequent value among the k nearest neighbors.
- Distance metrics: Hamming distance.
For a continuous variable, it uses the mean or mode.
-
Distance metrics:
- Euclidean
- Mahalanobis
- Manhattan
11.2.4.9 Matrix Completion
Impute items missing at random while accounting for dependence between features by using principal components, which is known as matrix completion (James et al. 2013, Sec 12.3)
Consider an \(n \times p\) feature matrix, \(\mathbf{X}\), with element \(x_{ij}\), some of which are missing.
Similar to 22.2, we can approximate the matrix \(\mathbf{X}\) in terms of its leading PCs.
We consider the \(M\) principal components that optimize
\[ \underset{\mathbf{A} \in \mathbb{R}^{n \times M}, \mathbf{B} \in \mathbb{R}^{p \times M}}{\operatorname{min}} \left\{ \sum_{(i,j) \in \mathcal{O}} (x_{ij} - \sum_{m=1}^M a_{im}b_{jm})^2 \right\} \]
where \(\mathcal{O}\) is the set of all observed pairs indices \((i,j)\), a subset of the possible \(n \times p\) pairs
Once this minimization is solved,
One can impute a missing observation, \(x_{ij}\), with \(\hat{x}_{ij} = \sum_{m=1}^M \hat{a}_{im}\hat{b}_{jm}\) where \(\hat{a}_{im}, \hat{b}_{jm}\) are the \((i,m)\) and \((j.m)\) elements, respectively, of the matrices \(\hat{\mathbf{A}}\) and \(\hat{\mathbf{B}}\) from the minimization, and
One can approximately recover the \(M\) principal component scores and loadings, as we did when the data were complete
The challenge here is to solve this minimization problem: the eigen-decomposition non longer applies (as in 22.2
Hence, we have to use iterative algorithm (James et al. 2013 Alg 12.1)
- Create a complete data matrix \(\tilde{\mathbf{X}}\) of dimension \(n \times p\) of which the \((i,j)\) element equals
\[ \tilde{x}_{ij} = \begin{cases} x_{ij} & \text{if } (i,j) \in \mathcal{O} \\ \bar{x}_{j} & \text{if } (i,j) \notin \mathcal{O} \end{cases} \]
where \(\bar{x}_j\) is the average of the observed values for the \(j\)th variable in the incomplete data matrix \(\mathbf{X}\)
\(\mathcal{O}\) indexes the observations that are observed in \(\mathbf{X}\)
- Repeat these 3 steps until some objectives are met
a. Solve
\[ \underset{\mathbf{A} \in R^{n \times M}, \mathbf{B} \in R^{p \times M}}{\operatorname{min}} \{ \sum_{(i,j) \in \mathcal{O}} (x_{ij} - \sum_{m=1}^M a_{im}b_{jm})^2 \} \]
by computing the principal components of \(\tilde{\mathbf{X}}\)
b. For each element \((i,j) \notin \mathcal{O}\), set \(\tilde{x}_{ij} \leftarrow \sum_{m=1}^M \hat{a}_{im}\hat{b}_{jm}\)
c. Compute the objective
\[ \sum_{(i,j \in \mathcal{O})} (x_{ij} - \sum_{m=1}^M \hat{a}_{im} \hat{b}_{jm})^2 \]
- Return the estimated missing entries \(\tilde{x}_{ij}, (i,j) \notin \mathcal{O}\)
11.3 Criteria for Choosing an Effective Approach
Criteria for an ideal technique in treating missing data:
- Unbiased parameter estimates
- Adequate power
- Accurate standard errors (p-values, confidence intervals)
The Multiple Imputation and Full Information Maximum Likelihood are the the most ideal candidate. Single imputation will generally lead to underestimation of standard errors.
11.4 Another Perspective
Model bias can arisen from various factors including:
- Imputation method
- Missing data mechanism (MCAR vs. MAR)
- Proportion of the missing data
- Information available in the data set
Since the imputed observations are themselves estimates, their values have corresponding random error. But when you put in that estimate as a data point, your software doesn’t know that. So it overlooks the extra source of error, resulting in too-small standard errors and too-small p-values. So multiple imputation comes up with multiple estimates.
Because multiple imputation have a random component, the multiple estimates are slightly different. This re-introduces some variation that your software can incorporate in order to give your model accurate estimates of standard error. Multiple imputation was a huge breakthrough in statistics about 20 years ago. It solves a lot of problems with missing data (though, unfortunately not all) and if done well, leads to unbiased parameter estimates and accurate standard errors. If your rate of missing data is very, very small (2-3%) it doesn’t matter what technique you use.
Remember that there are three goals of multiple imputation, or any missing data technique:
- Unbiased parameter estimates in the final analysis (regression coefficients, group means, odds ratios, etc.)
- accurate standard errors of those parameter estimates, and therefore, accurate p-values in the analysis
- adequate power to find meaningful parameter values significant.
Hence,
Don’t round off imputations for dummy variables. Many common imputation techniques, like MCMC, require normally distributed variables. Suggestions for imputing categorical variables were to dummy code them, impute them, then round off imputed values to 0 or 1. Recent research, however, has found that rounding off imputed values actually leads to biased parameter estimates in the analysis model. You actually get better results by leaving the imputed values at impossible values, even though it’s counter-intuitive.
Don’t transform skewed variables. Likewise, when you transform a variable to meet normality assumptions before imputing, you not only are changing the distribution of that variable but the relationship between that variable and the others you use to impute. Doing so can lead to imputing outliers, creating more bias than just imputing the skewed variable.
Use more imputations. The advice for years has been that 5-10 imputations are adequate. And while this is true for unbiasedness, you can get inconsistent results if you run the multiple imputation more than once. (Bodner 2008) recommends having as many imputations as the percentage of missing data. Since running more imputations isn’t any more work for the data analyst, there’s no reason not to.
Create multiplicative terms before imputing. When the analysis model contains a multiplicative term, like an interaction term or a quadratic, create the multiplicative terms first, then impute. Imputing first, and then creating the multiplicative terms actually biases the regression parameters of the multiplicative term (Von Hippel 2009)
11.5 Diagnosing the Mechanism
11.5.1 MAR vs. MNAR
The only true way to distinguish between MNAR and MAR is to measure some of that missing data.
It’s a common practice among professional surveyors to, for example, follow-up on a paper survey with phone calls to a group of the non-respondents and ask a few key survey items. This allows you to compare respondents to non-respondents.
If their responses on those key items differ by very much, that’s good evidence that the data are MNAR.
However in most missing data situations, we can’t get a hold of the missing data. So while we can’t test it directly, we can examine patterns in the data get an idea of what’s the most likely mechanism.
The first thing in diagnosing randomness of the missing data is to use your substantive scientific knowledge of the data and your field. The more sensitive the issue, the less likely people are to tell you. They’re not going to tell you as much about their cocaine usage as they are about their phone usage.
Likewise, many fields have common research situations in which non-ignorable data is common. Educate yourself in your field’s literature.
11.5.2 MCAR vs. MAR
There is a very useful test for MCAR, Little’s test.
A second technique is to create dummy variables for whether a variable is missing.
1 = missing 0 = observed
You can then run t-tests and chi-square tests between this variable and other variables in the data set to see if the missingness on this variable is related to the values of other variables.
For example, if women really are less likely to tell you their weight than men, a chi-square test will tell you that the percentage of missing data on the weight variable is higher for women than men.
11.6 Application
library(visdat)
library(naniar)
library(ggplot2)
vis_miss()
ggplot(data, aes(x, y)) +
geom_miss_point() +
facet_wrap( ~ group)
gg_miss_var(data, facet = group)
gg_miss_upset(data)
gg_miss_fct(x = variable1, fct = variable2)
Read more on The Missing Book by Nicholas Tierney & Allison Horst
How many imputation:
Usually 5. (unless you have extremely high portion of missing, in which case you probably need to check your data again)
According to Rubin, the relative efficiency of an estimate based on \(m\) imputations to infinity imputation is approximately
\[ (1+\frac{\lambda}{m})^{-1} \]
where \(\lambda\) is the rate of missing data
Example 50% of missing data means an estimate based on 5 imputation has standard deviation that is only 5% wider compared to an estimate based on infinity imputation
(\(\sqrt{1+0.5/5}=1.049\))
library(missForest)
#load data
data <- iris
#Generate 10% missing values at Random
set.seed(1)
iris.mis <- prodNA(iris, noNA = 0.1)
#remove categorical variables
iris.mis.cat <- iris.mis
iris.mis <- subset(iris.mis, select = -c(Species))
11.6.1 Imputation with mean / median / mode
# whole data set
e1071::impute(iris.mis, what = "mean") # replace with mean
e1071::impute(iris.mis, what = "median") # replace with median
# by variables
Hmisc::impute(iris.mis$Sepal.Length, mean) # mean
Hmisc::impute(iris.mis$Sepal.Length, median) # median
Hmisc::impute(iris.mis$Sepal.Length, 0) # replace specific number
check accuracy
# library(DMwR2)
# actuals <- iris$Sepal.Width[is.na(iris.mis$Sepal.Width)]
# predicteds <- rep(mean(iris$Sepal.Width, na.rm=T), length(actuals))
# regr.eval(actuals, predicteds)
11.6.2 KNN
# library(DMwR2)
# # iris.mis[,!names(iris.mis) %in% c("Sepal.Length")]
# # data should be this line. But since knn cant work with 3 or less variables,
# # we need to use at least 4 variables.
#
# # knn is not appropriate for categorical variables
# knnOutput <-
# knnImputation(data = iris.mis.cat,
# #k = 10,
# meth = "median" # could use "median" or "weighAvg")
# # should exclude the dependent variable: Sepal.Length
# anyNA(knnOutput)
# library(DMwR2)
# actuals <- iris$Sepal.Width[is.na(iris.mis$Sepal.Width)]
# predicteds <- knnOutput[is.na(iris.mis$Sepal.Width), "Sepal.Width"]
# regr.eval(actuals, predicteds)
Compared to MAPE (mean absolute percentage error) of mean imputation, we see almost always see improvements.
11.6.3 rpart
For categorical (factor) variables, rpart
can handle
library(rpart)
class_mod <-
rpart(
Species ~ . - Sepal.Length,
data = iris.mis.cat[!is.na(iris.mis.cat$Species),],
method = "class",
na.action = na.omit
) # since Species is a factor, and exclude dependent variable "Sepal.Length"
anova_mod <-
rpart(
Sepal.Width ~ . - Sepal.Length,
data = iris.mis[!is.na(iris.mis$Sepal.Width),],
method = "anova",
na.action = na.omit
) # since Sepal.Width is numeric.
species_pred <-
predict(class_mod, iris.mis.cat[is.na(iris.mis.cat$Species),])
width_pred <-
predict(anova_mod, iris.mis[is.na(iris.mis$Sepal.Width),])
11.6.4 MICE (Multivariate Imputation via Chained Equations)
Assumption: data are MAR
It imputes data per variable by specifying an imputation model for each variable
Example
We have \(X_1, X_2,..,X_k\). If \(X_1\) has missing data, then it is regressed on the rest of the variables. Same procedure applies if \(X_2\) has missing data. Then, predicted values are used in place of missing values.
By default,
- Continuous variables use linear regression.
- Categorical Variables use logistic regression.
Methods in MICE:
- PMM (Predictive Mean Matching) – For numeric variables
-
logreg
(Logistic Regression) – For Binary Variables( with 2 levels) -
polyreg
(Bayesian polytomous regression) – For Factor Variables (>= 2 levels) - Proportional odds model (ordered, >= 2 levels)
# load package
library(mice)
library(VIM)
# check missing values
md.pattern(iris.mis)
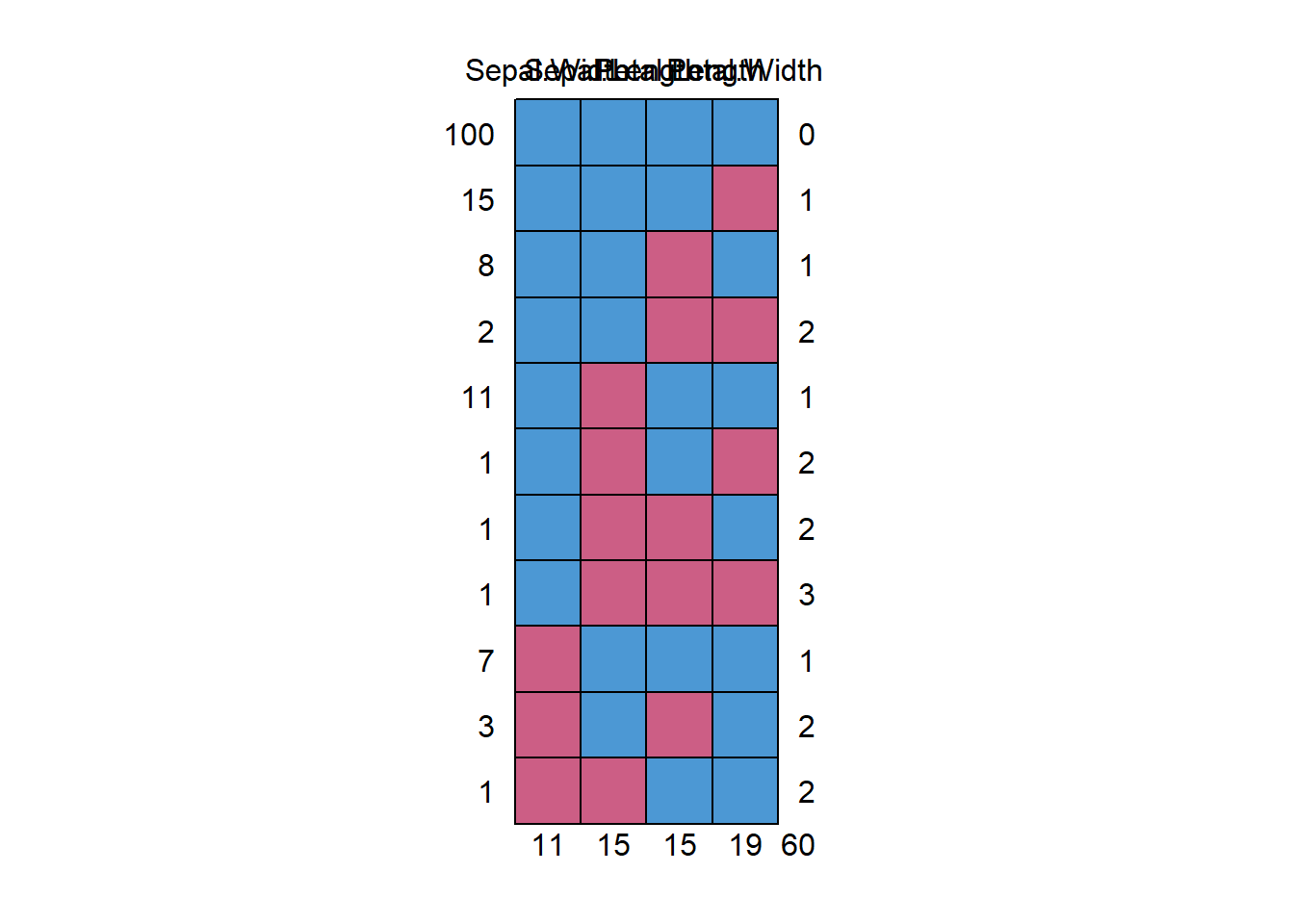
#> Sepal.Width Sepal.Length Petal.Length Petal.Width
#> 100 1 1 1 1 0
#> 15 1 1 1 0 1
#> 8 1 1 0 1 1
#> 2 1 1 0 0 2
#> 11 1 0 1 1 1
#> 1 1 0 1 0 2
#> 1 1 0 0 1 2
#> 1 1 0 0 0 3
#> 7 0 1 1 1 1
#> 3 0 1 0 1 2
#> 1 0 0 1 1 2
#> 11 15 15 19 60
#plot the missing values
aggr(
iris.mis,
col = mdc(1:2),
numbers = TRUE,
sortVars = TRUE,
labels = names(iris.mis),
cex.axis = .7,
gap = 3,
ylab = c("Proportion of missingness", "Missingness Pattern")
)
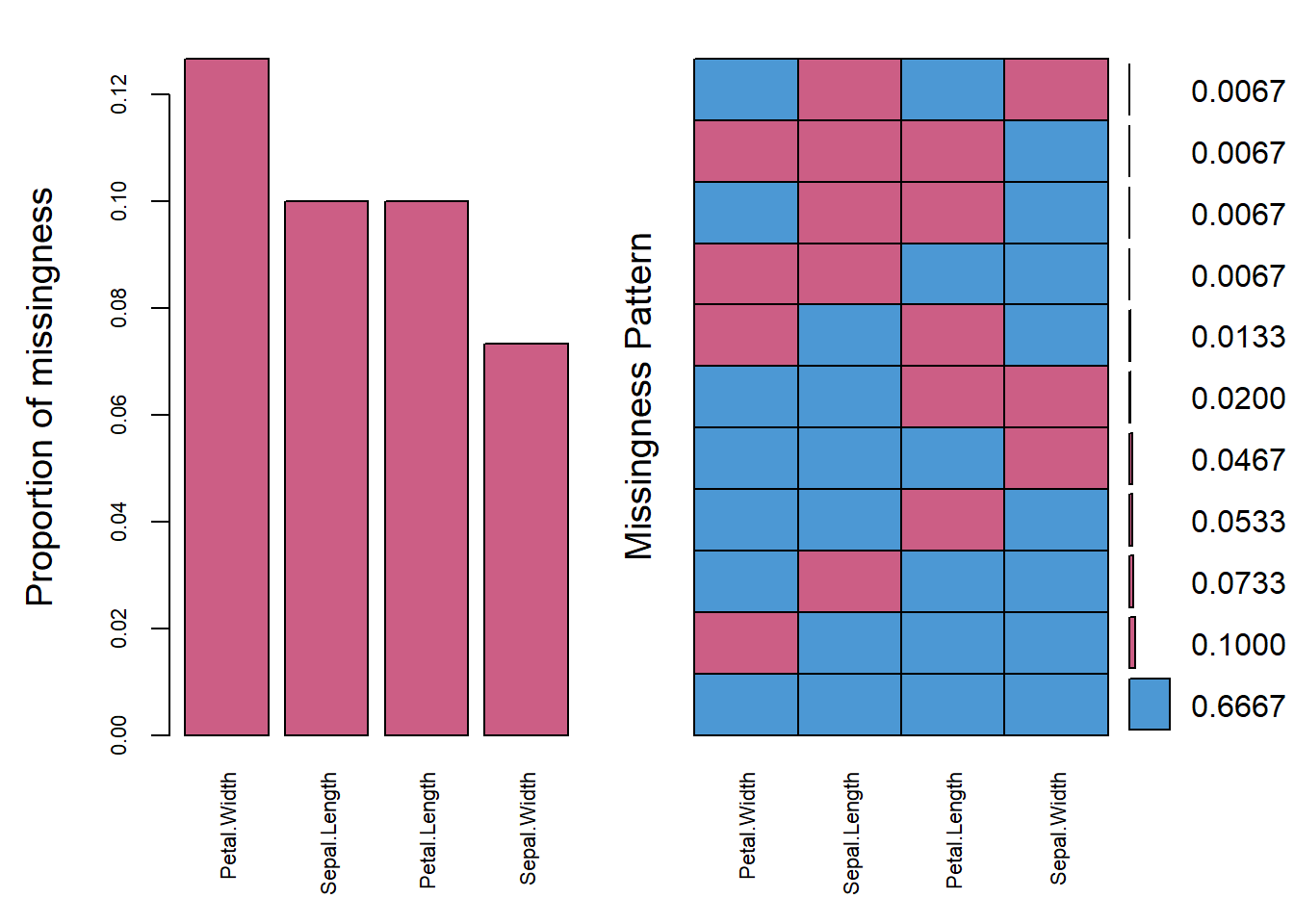
#>
#> Variables sorted by number of missings:
#> Variable Count
#> Petal.Width 0.12666667
#> Sepal.Length 0.10000000
#> Petal.Length 0.10000000
#> Sepal.Width 0.07333333
mice_plot <- aggr(
iris.mis,
col = c('navyblue', 'yellow'),
numbers = TRUE,
sortVars = TRUE,
labels = names(iris.mis),
cex.axis = .7,
gap = 3,
ylab = c("Missing data", "Pattern")
)
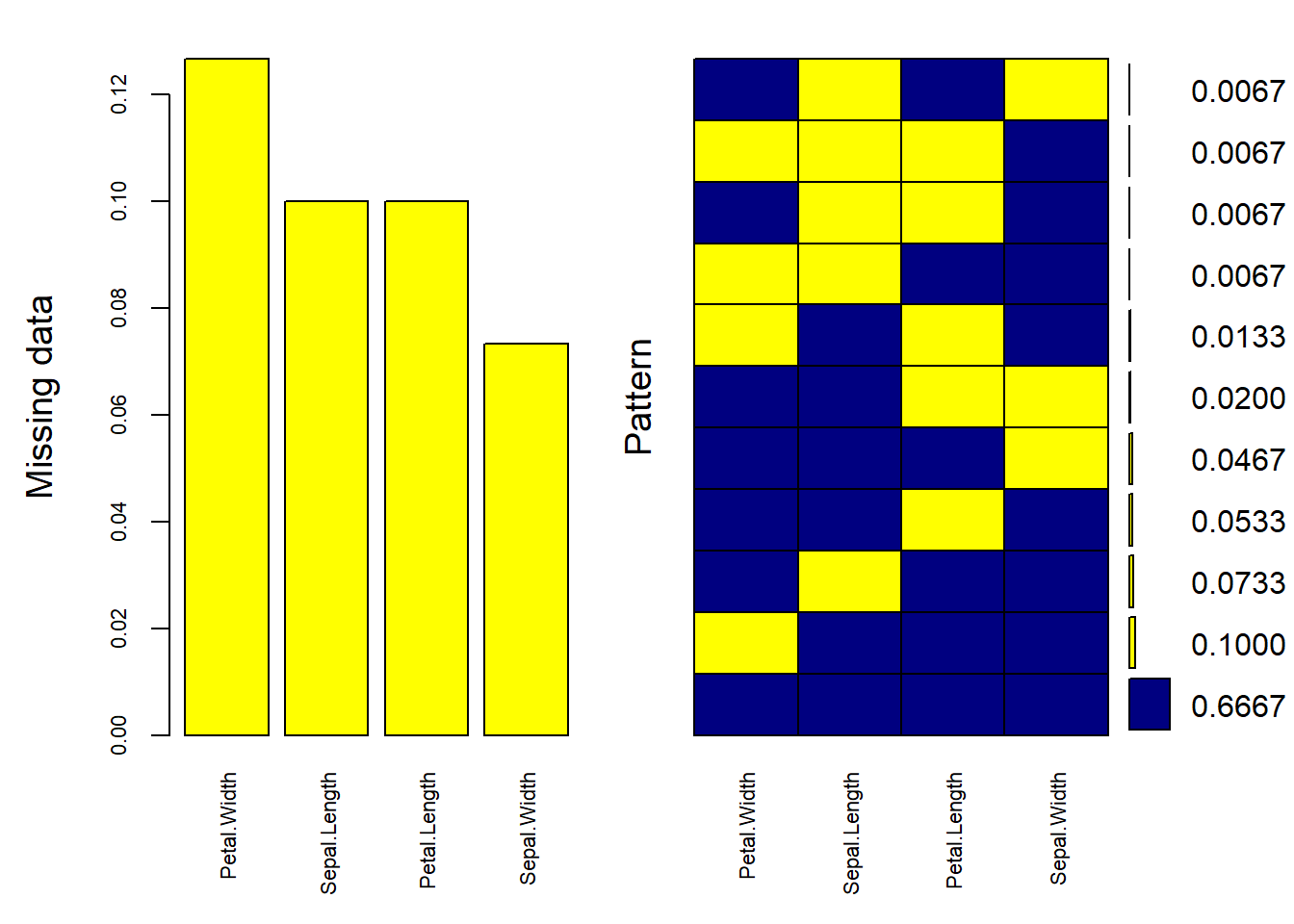
#>
#> Variables sorted by number of missings:
#> Variable Count
#> Petal.Width 0.12666667
#> Sepal.Length 0.10000000
#> Petal.Length 0.10000000
#> Sepal.Width 0.07333333
Impute Data
imputed_Data <-
mice(
iris.mis,
m = 5, # number of imputed datasets
maxit = 50, # number of iterations taken to impute missing values
method = 'pmm', # method used in imputation.
# Here, we used predictive mean matching
# other methods can be
# "pmm": Predictive mean matching
# "midastouch" : weighted predictive mean matching
# "sample": Random sample from observed values
# "cart": classification and regression trees
# "rf": random forest imputations.
# "2lonly.pmm": Level-2 class predictive mean matching
# Other methods based on whether variables are
# (1) numeric, (2) binary, (3) ordered, (4), unordered
seed = 500
)
summary(imputed_Data)
#> Class: mids
#> Number of multiple imputations: 5
#> Imputation methods:
#> Sepal.Length Sepal.Width Petal.Length Petal.Width
#> "pmm" "pmm" "pmm" "pmm"
#> PredictorMatrix:
#> Sepal.Length Sepal.Width Petal.Length Petal.Width
#> Sepal.Length 0 1 1 1
#> Sepal.Width 1 0 1 1
#> Petal.Length 1 1 0 1
#> Petal.Width 1 1 1 0
#make a density plot
densityplot(imputed_Data)
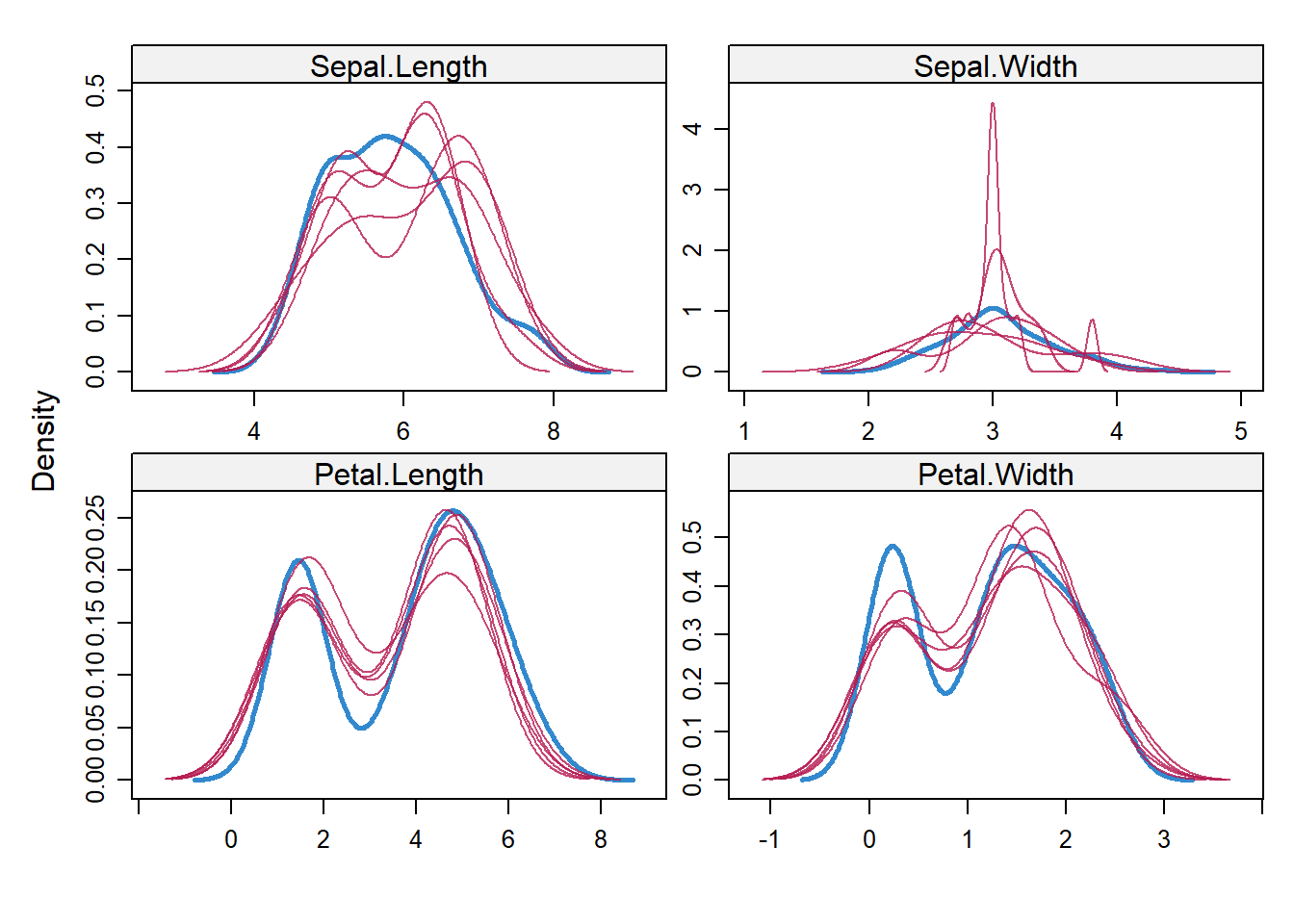
#the red (imputed values) should be similar to the blue (observed)
Check imputed dataset
Regression model using imputed datasets
# regression model
fit <-
with(data = imputed_Data,
exp = lm(Sepal.Width ~ Sepal.Length + Petal.Width))
#combine results of all 5 models
combine <- pool(fit)
summary(combine)
#> term estimate std.error statistic df p.value
#> 1 (Intercept) 1.8963130 0.32453912 5.843095 131.0856 3.838556e-08
#> 2 Sepal.Length 0.2974293 0.06679204 4.453066 130.2103 1.802241e-05
#> 3 Petal.Width -0.4811603 0.07376809 -6.522608 108.8253 2.243032e-09
11.6.5 Amelia
- Use bootstrap based EMB algorithm (faster and robust to impute many variables including cross sectional, time series data etc)
- Use parallel imputation feature using multicore CPUs.
Assumptions
- All variables follow Multivariate Normal Distribution (MVN). Hence, this package works best when data is MVN, or transformation to normality.
- Missing data is Missing at Random (MAR)
Steps:
- m bootstrap samples and applies EMB algorithm to each sample. Then we have m different estimates of mean and variances.
- the first set of estimates are used to impute first set of missing values using regression, then second set of estimates are used for second set and so on.
However, Amelia is different from MICE
- MICE imputes data on variable by variable basis whereas MVN uses a joint modeling approach based on multivariate normal distribution.
- MICE can handle different types of variables while the variables in MVN need to be normally distributed or transformed to approximate normality.
- MICE can manage imputation of variables defined on a subset of data whereas MVN cannot.
- idvars – keep all ID variables and other variables which you don’t want to impute
- noms – keep nominal variables here
#specify columns and run amelia
amelia_fit <-
amelia(iris.mis,
m = 5,
parallel = "multicore",
noms = "Species")
#> -- Imputation 1 --
#>
#> 1 2 3 4 5 6 7 8
#>
#> -- Imputation 2 --
#>
#> 1 2 3 4 5 6 7 8
#>
#> -- Imputation 3 --
#>
#> 1 2 3 4 5
#>
#> -- Imputation 4 --
#>
#> 1 2 3 4 5 6 7
#>
#> -- Imputation 5 --
#>
#> 1 2 3 4 5 6 7
# access imputed outputs
# amelia_fit$imputations[[1]]
11.6.6 missForest
- an implementation of random forest algorithm (a non parametric imputation method applicable to various variable types). Hence, no assumption about function form of f. Instead, it tries to estimate f such that it can be as close to the data points as possible.
- builds a random forest model for each variable. Then it uses the model to predict missing values in the variable with the help of observed values.
- It yields out of bag imputation error estimate. Moreover, it provides high level of control on imputation process.
- Since bagging works well on categorical variable too, we don’t need to remove them here.
library(missForest)
#impute missing values, using all parameters as default values
iris.imp <- missForest(iris.mis)
# check imputed values
# iris.imp$ximp
- check imputation error
- NRMSE is normalized mean squared error. It is used to represent error derived from imputing continuous values.
- PFC (proportion of falsely classified) is used to represent error derived from imputing categorical values.
iris.imp$OOBerror
#> NRMSE PFC
#> 0.13631893 0.04477612
#comparing actual data accuracy
iris.err <- mixError(iris.imp$ximp, iris.mis, iris)
iris.err
#> NRMSE PFC
#> 0.1501524 0.0625000
This means categorical variables are imputed with 5% error and continuous variables are imputed with 14% error.
This can be improved by tuning the values of mtry
and ntree
parameter.
-
mtry
refers to the number of variables being randomly sampled at each split. -
ntree
refers to number of trees to grow in the forest.
11.6.7 Hmisc
-
impute()
function imputes missing value using user defined statistical method (mean, max, mean). It’s default is median.
-
aregImpute()
allows mean imputation using additive regression, bootstrapping, and predictive mean matching.
- In bootstrapping, different bootstrap resamples are used for each of multiple imputations. Then, a flexible additive model (non parametric regression method) is fitted on samples taken with replacements from original data and missing values (acts as dependent variable) are predicted using non-missing values (independent variable).
- it uses predictive mean matching (default) to impute missing values. Predictive mean matching works well for continuous and categorical (binary & multi-level) without the need for computing residuals and maximum likelihood fit.
Note
- For predicting categorical variables, Fisher’s optimum scoring method is used.
-
Hmisc
automatically recognizes the variables types and uses bootstrap sample and predictive mean matching to impute missing values. -
missForest
can outperformHmisc
if the observed variables have sufficient information.
Assumption
- linearity in the variables being predicted.
library(Hmisc)
# impute with mean value
iris.mis$imputed_age <- with(iris.mis, impute(Sepal.Length, mean))
# impute with random value
iris.mis$imputed_age2 <-
with(iris.mis, impute(Sepal.Length, 'random'))
# could also use min, max, median to impute missing value
# using argImpute
impute_arg <-
# argImpute() automatically identifies the variable type
# and treats them accordingly.
aregImpute(
~ Sepal.Length + Sepal.Width + Petal.Length + Petal.Width +
Species,
data = iris.mis,
n.impute = 5
)
#> Iteration 1
Iteration 2
Iteration 3
Iteration 4
Iteration 5
Iteration 6
Iteration 7
Iteration 8
impute_arg # R-squares are for predicted missing values.
#>
#> Multiple Imputation using Bootstrap and PMM
#>
#> aregImpute(formula = ~Sepal.Length + Sepal.Width + Petal.Length +
#> Petal.Width + Species, data = iris.mis, n.impute = 5)
#>
#> n: 150 p: 5 Imputations: 5 nk: 3
#>
#> Number of NAs:
#> Sepal.Length Sepal.Width Petal.Length Petal.Width Species
#> 11 11 13 24 16
#>
#> type d.f.
#> Sepal.Length s 2
#> Sepal.Width s 2
#> Petal.Length s 2
#> Petal.Width s 2
#> Species c 2
#>
#> Transformation of Target Variables Forced to be Linear
#>
#> R-squares for Predicting Non-Missing Values for Each Variable
#> Using Last Imputations of Predictors
#> Sepal.Length Sepal.Width Petal.Length Petal.Width Species
#> 0.907 0.660 0.978 0.963 0.993
# check imputed variable Sepal.Length
impute_arg$imputed$Sepal.Length
#> [,1] [,2] [,3] [,4] [,5]
#> 19 5.2 5.2 5.2 5.8 5.7
#> 21 5.1 5.0 5.1 5.7 5.4
#> 31 4.8 5.0 5.2 5.0 4.8
#> 35 4.6 4.9 4.9 4.9 4.8
#> 49 5.0 5.1 5.1 5.1 5.1
#> 62 6.2 5.7 6.0 6.4 5.6
#> 65 5.5 5.5 5.2 5.8 5.5
#> 67 6.5 5.8 5.8 6.3 6.5
#> 82 5.2 5.1 5.7 5.8 5.5
#> 113 6.4 6.5 7.4 7.2 6.3
#> 122 6.2 5.8 5.5 5.8 6.7
11.6.8 mi
- allows graphical diagnostics of imputation models and convergence of imputation process.
- uses Bayesian version of regression models to handle issue of separation.
- automatically detects irregularities in data (e.g., high collinearity among variables).
- adds noise to imputation process to solve the problem of additive constraints.