27.6 Making decisions with P-values
P-values tells us the likelihood of observing the sample statistic (or something more extreme), based on the assumption about the population parameter being true. In this context, the P-value tells us the likelihood of observing the value of ˉx (or something more extreme), just through sampling variation (chance) if μ=37. The P-value is a probability, albeit a probability of something quite specific, so it is a value between 0 and 1. Then:
‘Big’ P-values mean that the sample statistic (i.e., ˉx) could reasonably have occurred through sampling variation, if the assumption about the parameter (stated in H0) was true (Fig. 27.7, top panel): The data do not contradict the assumption (H0).
‘Small’ P-values mean that the sample statistic (i.e., ˉx) is unlikely to have occurred through sampling variation, if the assumption about the parameter (stated in H0) was true: (Fig. 27.7, bottom panel): The data contradict the assumption.
What is meant by ‘small’ and ‘big?’ It is arbitrary: no definitive rules exist. Commonly, a P-value smaller than 1% (that is, smaller than 0.01) is usually considered ‘small,’ and a P-value larger than 10% (that is, larger than 0.10) is usually considered ‘big.’ Between the values of 1% and 10% is often a ‘grey area.’
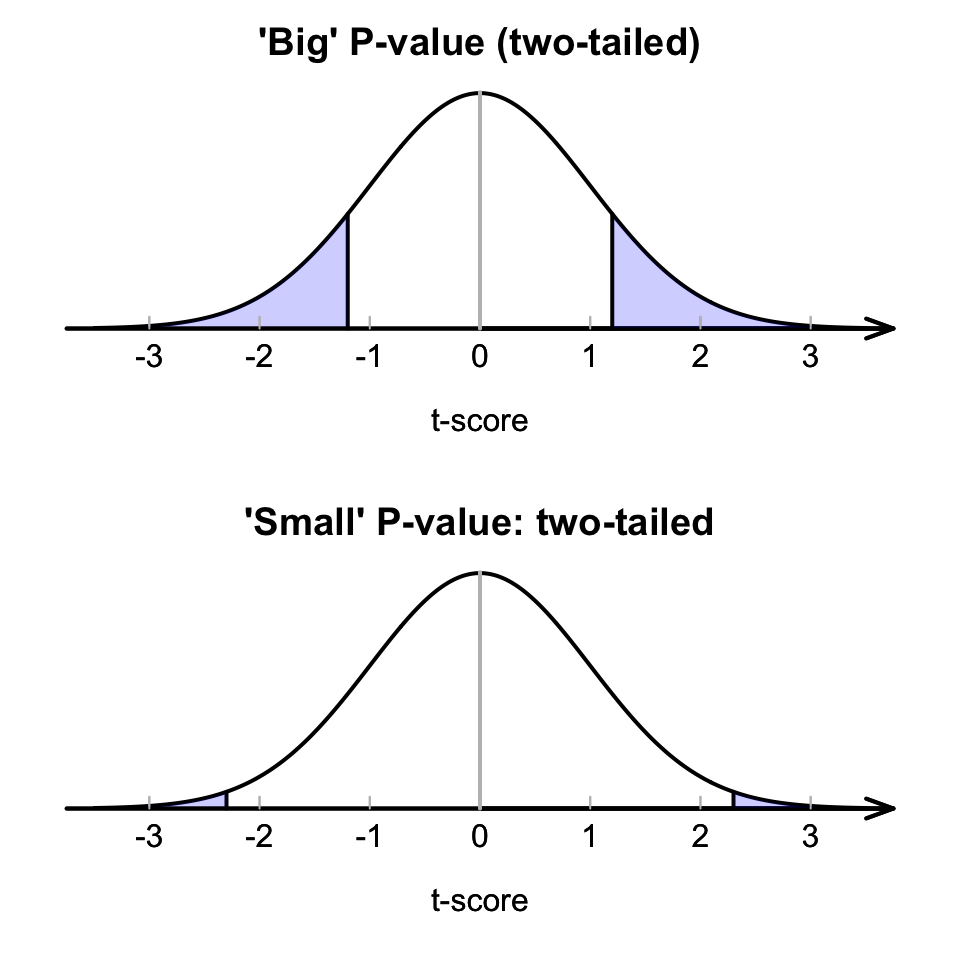
FIGURE 27.7: A picture of large (top) and small (bottom) P-value situations
Traditionally, a P-value is ‘small’ if it is less than 5% (less than 0.05), and ‘big’ if greater than 5% (greater than 0.05). However, again this is arbitrary, and binary decision making (either big or small) is unreasonable. More reasonably, P-values should be interpreted as providing varying strength of evidence in support of the alternative hypothesis H1 (Table 27.1. These are not definitive, but are only guidelines. Of course, conclusions should be written in the context of the problem.
If the P-value is… | Write the conclusion as… |
---|---|
Larger than 0.10 | Insufficient evidence to support H1 |
Between 0.05 and 0.10 | Slight evidence to support H1 |
Between 0.01 and 0.05 | Moderate evidence to support H1 |
Between 0.001 and 0.01 | Strong evidence to support H1 |
Smaller than 0.001 | Very strong evidence to support H1 |
For one-tailed tests, the P-value is half the value of the two-tailed P-value.
SPSS always produces two-tailed P-values, usually calls them ‘Significance values,’ and labels them asSig.
,
and sometimes explicitly notes that they are two-tailed.
For the body-temperature data then, where P<0.001, the P-value is very small, so there is very strong evidence that the population mean body temperature is not 37.0∘C.