28.3 Restructuring: subsetting, grouping, aggregating
Some subsets are investigated more than others. It depends on the aims of the study. Subsetting involves selecting the relevant variables and cases. Large datasets can be heterogeneous with cases being of many distinct kinds that should be analysed separately. Subsetting overlaps with excluding errors, outliers or other problematic cases.
In studying how gold medal performances had changed over the years at the Olympics, only the subset of athletics and swimming events were considered. Weightlifting events could have been studied too, but many Olympic disciplines—e.g., gymnastics, boxing, diving—are not readily comparable over time.
Subsetting selects part of a dataset to be analysed. Grouping splits a dataset or subset into groups to be compared. In Chapter 12 results for two groups, those getting a treatment for their psoriasis and those receiving a placebo, were compared. In Chapter 14 species of Darwin’s finches were compared on one island, and one species was compared across different islands. In Chapter 18 three species of Penguins were compared. In Chapter 20 subsets of swimming performances were chosen and then compared by stroke, distance, and swimmer’s sex. In the Comrades Marathon dataset the numbers running were grouped by sex and age and compared over time in Figure 21.2, shown here in reduced size as Figure 28.4.
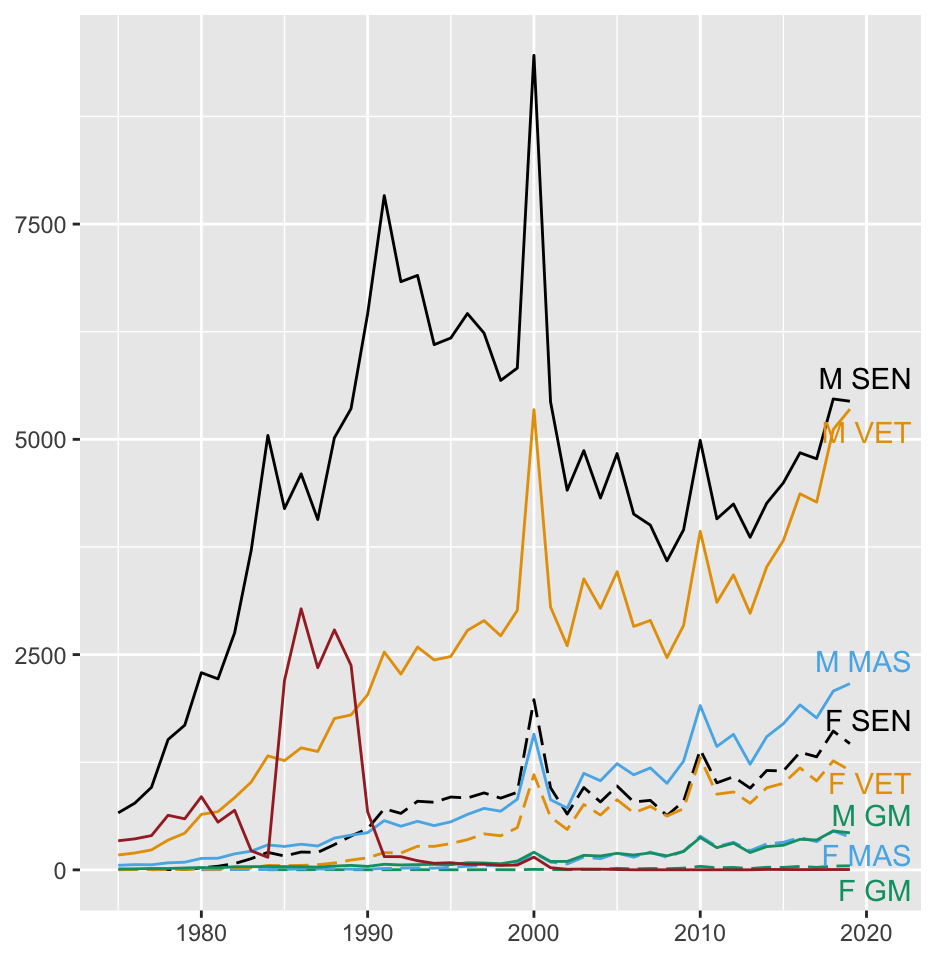
Figure 28.4: Numbers of finishers of the Comrades Ultramarathon by age group and sex from 1975 to 2019
Statistics are summaries based on aggregations. They depend on how data have been subset, grouped or aggregated. In the analysis of the 2021 German election in Chapter 26, results could be studied by individual constituency, by Bundesland, by groups of Bundesländer (such as the former East and West), or by the whole country. It is relatively easy to keep track of such hierarchical or nested structures. If there are several categorical variables with no hierarchical structure, as in the study of fuel efficiency of cars in the US in Chapter 17, there are many possible summarising levels. Just considering two of the classifying variables could lead to statistics for vehicle classes within manufacturer or for manufacturers within vehicle class or for vehicle classes and manufacturers.
Further flexibility arises when there are variables with many categories. Analyses may be simplified by combining smaller categories, as was done in Chapters 8, 15, 17, 25, and 26. Different analysts will have different opinions on how small small should be.