Welcome
This book provides a gentle introduction to data science for students of any discipline with little or no background in data analysis or computer programming. Based on notions of representation and modeling, we examine some key data types and data structures, and then learn to clean, transform, summarize and visualize data to communicate our results.
The main limitation of this book is that it focuses on rectangular data: Data that is represented in the rows and columns of tables. What may appear to be a second limitation — the absence of sophisticated modeling and machine learning methods — may actually be a feature: Rather than chasing after the latest hypes, we emphasize the importance of basic tasks, solid skills, and simple tools.
Today, students of statistics and elementary computer science are inundated by buzzword tools and complex analysis techniques. Given the ubiquity of statistical software and machine learning platforms, even novice users are tempted to use complex methods with fancy acronyms and academic pedigrees. But whenever methods and tools become opaque to us, we cannot check the validity of our results or detect and fix potential errors. Thus, our main goals here are to promote an understanding of data-related problems, introduce basic methods for solving them, and suggest ways for communicating our findings in a transparent fashion. By reflecting on the interplay between representations, tasks, and tools, this book promote data literacy and cultivate reproducible research practices that precede and enable any practical use of programming or statistics.
Please note
This course
What you know about computing other people will learn.
Don’t feel as if the key to successful computing is only in your hands.
What’s in your hands, I think and hope, is intelligence:
the ability to see the machine as more than when you were first led up to it,
that you can make it more.Alan J. Perlis (from the dedication of Abelson et al., 1996)
The current iteration of the course Introduction to Data Science (using R, ADILT) takes place at the University of Konstanz in 2023. However, as all course materials are freely available online, anyone interested in this topic is welcome to read and learn from these materials.
General information on the university’s Advanced Data and Information Literacy Track (ADILT) is available at https://www.uni-konstanz.de/adilt/.
Coordinates
- Introduction to data science 1 (ADILT, i2ds) (PSY-16620) and Introduction to data science 2 (ADILT, i2ds) (PSY-16710) at the University of Konstanz by Hansjörg Neth (h.neth@uni.kn, SPDS, office D507).
- Spring/summer 2024: Organized as block seminars.
- Course materials:
Goals
Completing this course enables students to understand, transform, analyze, and visualize data in a variety of ways. Whereas initial chapters provide an introduction to data types, data visualization, and exploratory data analysis (using base R and tidyverse packages), later chapters address more advanced issues of programming, running simulations, and predictive modeling.
The course uses the technologies and tools provided by R (R Core Team, 2023), the RStudio IDE, RMarkdown, including some key packages of the tidyverse (Wickham et al., 2019) (e.g., dplyr, ggplot2, tibble, and tidyr).
Audience and Preconditions
This course is targeted at students of all backgrounds and disciplines with a curiosity for data analysis and quantitative science. Prior familiarity with computer programming, empirical research methods or statistics is a bonus, but not a necessary condition.
More advanced students (e.g., working on their BSc/MSc thesis) should consider the course Data Science for Psychologists (PSY-15150) that proceeds at a faster pace than this introductory course (see ZEuS for details).
Requirements
In this course, we adopt an active learning and learning by doing approach. Good preparation (by working through the current topic before each session), regular attendance with active participation, and the conscientous completion of weekly exercises are essential for succeeding in this course.
Our data
Data science uses and depends on data.
Content providers want to get to know their users, companies want user feedback on their campaigns and products, governments want to know the topics, preferences, and opinions of their electorate.
Data often stems from online surveys. These have many benefits (e.g., convenience and speed, diversity) vs. some downsides (anonymity, poor data quality). Overall, the quality of data depends less on its source, but on the way in which it is collected, shared, and used.
This course and text is no exception: We would like to know who takes this course, what they wish, want, and expect, and what their background and preferences are.
Please take part in the survey to provide us with some initial data to work with.
- Link to survey to appear here.
The upshot: We can examine and use this data — your data — throughout the course, provided that its collection conforms to ethical and professional guidelines, its intended and open use is properly disclosed, and all participants volunteer to take part and understand and explicitly consent to these conditions.
Preparations
To teach and learn data science, we need some infrastructure (tools), materials (information), and agree on some rules (structure).
Software
Working through this book assumes an installation of three types of software programs:
An R engine: The R project for statistical computing (R Core Team, 2023) is the origin of all things R. A current distribution of R — e.g., R version 4.3.2 (2023-10-31) — for your machine can be downloaded from one if its mirrors.
An R interface: The RStudio IDE (by Posit) provides an integrated development environment for R.2
Additional tools: The R packages of the tidyverse (Wickham, 2023b) and ds4psy (Neth, 2023b).
To understand the differences between these components, two analogies are helpful:
R vs. RStudio: Think of the analogy of a car — engine vs. driver’s console/dashboard (means of input and output, monitoring system information).
R vs. R packages: Think of a toolbox containing a Swiss pocket knife with additional tools (see the section on Terminology).
Welcome to the R world
Once you have installed the software, take a moment to reflect on a curious fact: You just installed software that was written by hundreds of highly-trained experts, who dedicate years of their professional lives to its creation and improvement. Interestingly, you could just download their products and had to pay nothing to do so. This is possible because most R developers subscribe to an open source philosophy that was ridiculed by corporations when it started in the 1980s and 1990s, but has become one of the most powerful paradigms in software development.
But actually, you did invest time and effort to install all these programs and packages. And by doing so, you are taking first steps to join a world-wide community that shares certain interests, assumptions, and ideals. So, welcome to the R community — but be aware: Learning R can profoundly transform your life.
Working with RStudio
The distinctions between R, RStudio, and R packages are somewhat confusing at first. Thus, it is good to know that we will typically be using the RStudio IDE to interact with R and manage our library of R packages. The basic idea of an integrated development environment (IDE) is to make it easier to access and manage all R-related concerns through a single interface. So think of RStudio as your console or dashboard that allows you to monitor and control the R engine underneath.
Given its large variety of functions, the RStudio interface is divided into many sub-windows that can be arranged and expanded in various ways. At this point, we only need to distinguish between the main Editor window (typically located on the top left), the Console (for entering R commands), and a few auxiliary windows that may display outputs (e.g., a Viewer for showing visualizations) and provide information on our current Environment or the Packages available on our computer. A useful window is Help: Although its main page provides mostly links to online materials, any R package contains detailed documentations on its functions and examples that illustrate their use.
Figure 0.1 shows the Posit cheatsheet on the RStudio IDE and illustrates that there are dozens of other functions available. As you get more experienced, you will discover lots of nifty features and shortcuts. Especially foldable sections and keyboard shortcuts (see Alt + Shift + K
for an overview) can make your life in R a lot easier.
But don’t let the abundance of options overwhelm you — I have yet to meet a person who needs or uses all of them.
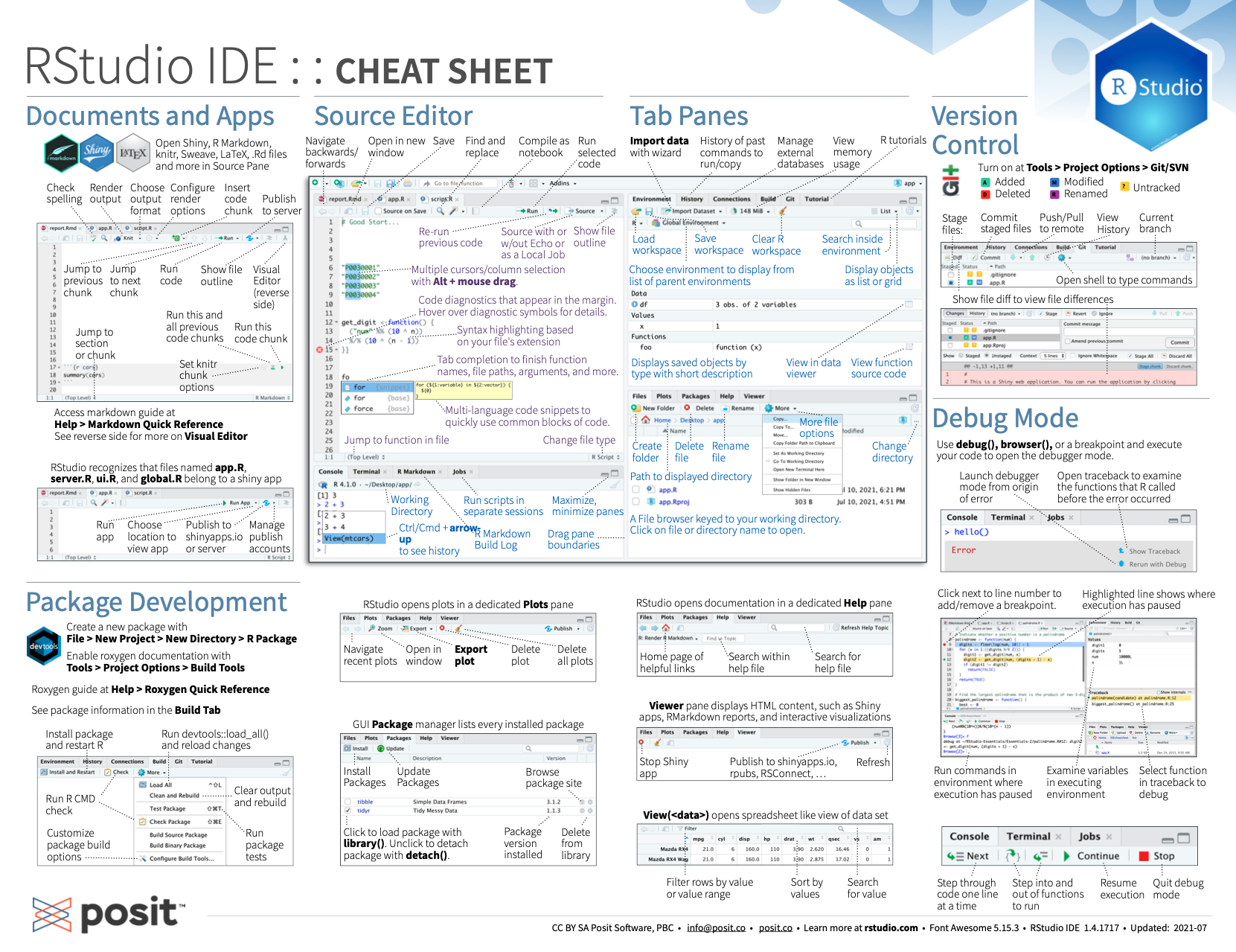
Figure 0.1: The RStudio IDE cheat sheet (from Posit cheatsheets).
A very useful feature of the RStudio IDE is that collections of files can be combined into projects. For instance, it makes sense to store everything related to this course in a dedicated directory on your hard drive (e.g., in a folder “i2ds”) and create an RStudio project (also named i2ds) that uses this directory as its root. An immediate benefit of using projects is that your entire workflow gets more organized.3
Readings
This i2ds book is still being written and revised and is likely to change frequently. Although it provides a useful and growing collection of new materials, it will remain fragmentary for the forseeable future. Fortunately, we can rely on other books for specific topics and materials. For instance, the more stable textbook Data Science for Psychologists (Neth, 2023a) caters to the special needs of social science students:
- Neth, H. (2023). ds4psy: Data Science for Psychologists.
Social Psychology and Decision Sciences, University of Konstanz, Germany.
Textbook and R package (version 1.0.0, September 15, 2023).
Retrieved from https://bookdown.org/hneth/ds4psy/.
doi 10.5281/zenodo.7229812
- The R package ds4psy (Neth, 2023b) is available at https://CRAN.R-project.org/package=ds4psy.
Relation to ds4psy
Relying on the ds4psy textbook makes sense for many topics, as the overlap of important concepts and tools is substantial. Rather than repeating entire sections here, we will avoid redundancies by providing links whenever appropriate.
At the same time, the goals and focus of the present i2ds course is more general. Beyond addressing a wider scope of target audiences (including students of all disciplines), we also dedicate more space and time to reflections and critical thinking. Whereas the ds4psy book and course essentially covers a topic and R package per chapter, this book contains shorter and more limited chapters, but combines them into larger parts.
Whereas most other books on data science may dive deeper into technical details (of R and R packages), this text provides more background information and puts many concepts into a theoretical perspective (known as ecological rationality, see Chapter 1). On the surface, this may seem like covering less content or proceeding at a slower pace. However, we believe that reflecting on conceptual foundations is a worthwhile investment. By opting for a two-semester curriculum (e.g., basic skills and tools vs. applications), we aim to promote a deeper understanding of data science.
Some distinguishing features of the i2ds book and course include:
- starting with literate programming and reproducible research (and using R Markdown)
- reflecting on the nature of representations, visualizations, etc.
- devoting two early sessions on R basics (base R)
- devoting four sessions on visualization (including a chapter on color)
- using data from our own online survey in many chapters (yet todo)
- covering a range of applications (in later chapters and parts)
Other textbooks
Several additional sources are freely available online:
The textbooks R for Data Science and its 2nd edition (Wickham, Çetinkaya-Rundel, et al., 2023; Wickham & Grolemund, 2017) provide a classic, but more technical and tidyverse-centric introduction to data science:
Wickham, H., & Grolemund, G. (2017). R for data science: Import, tidy, transform, visualize, and model data. Sebastopol, Canada: O’Reilly Media, Inc. [Available at http://r4ds.had.co.nz.]
Wickham, H., Cetinkaya-Rundel, M. & Grolemund, G. (2023). R for data science: Import, tidy, transform, visualize, and model data (2nd edition). Sebastopol, Canada: O’Reilly Media, Inc. [Available at https://r4ds.hadley.nz/.]
Where it makes sense, we will point at chapters of related textbooks (e.g., Baumer et al., 2021):
- Baumer, B. S., Kaplan, D. T., & Horton, N. J. (2020).
Modern Data Science with R (2nd ed.).
CRC Press, Taylor & Francis Group, Boca Raton/London/New York.
[Available at https://beanumber.github.io/mdsr2e/.]
Ismay, C., & Kim, A. Y. (2020). Statistical inference via data science: A ModernDive into R and the tidyverse.
[Available at https://moderndive.com.]Zumel, N., Mount, J., & Porzak, J. (2014). Practical data science with R. Shelter Island, NY: Manning.
Literate programming
This book assumes that sound thinking and solid methodological skills are conditions for creative work in both scientific and applied disciplines. A key virtue to be learned and exercised on the path from novice to expert is that of transparent communication.
Beyond knowledge on using R and various R packages for analyzing data, one of the most important skills conveyed in this course — and one that remains relevant far beyond the topic of data science — is called literate programming (Knuth, 1984): Literate programming is a paradigm for working with computers that concerns how we write both text and computer code. Its basic goal is to design a system that enables distinctions between data types (e.g., between text and code) in order to treat them appropriately (e.g., typeset text and evaluate code), and ultimately merge their results (e.g., computations, text, figures, and tables). Conceptually, a key idea when engaging with literate programming is to separate form from content — and prioritizing content over form while creating new material. (See Wikipedia: Literate programming for background information.)
Practically, adopting this paradigm requires some skills and tools that may seem awkward and unfamiliar at first, but are easily acquired — and likely to change our lives by enabling countless opportunities.4 In this course, the main tool that allows weaving text and code together in a single document is R Markdown (which is maintained by the developers of the RStudio IDE mentioned above).
For our purposes, literate programming will allow us to take a step towards reproducible research (see Section 1.3). As a first approximation, reproducible research is a methodology to ensure that others can understand and re-create a document. Thus, reproducible research practices and promotes transparency in science. For instance, when analyzing a set of data or completing exercises on some topic, we will learn to create files that explicate all intermediate steps and can be transformed into a variety of output formats. Given the right tools, making research reproducible is not difficult, but requires an initial effort and regular practice. To turn a good idea into a habit, we will start using corresponding tools in our very first session. As we will see in Chapter 1, distinguishing between content and form makes it easy to create well-formatted documents for a variety of purposes. This will enable students not only to submit exercise solutions, but also to write research reports, blogs, and academic theses.5
ADILT denotes the Advanced Data and Information Literacy Track at the University of Konstanz.↩︎
Installing RStudio typically provides many additional R packages. Two packages we will use extensively are knitr (Xie, 2023) and rmarkdown (Allaire et al., 2024).↩︎
See the introductory chapters of R for Data Science, ideally in its 2nd edition (Wickham, Çetinkaya-Rundel, et al., 2023; Wickham & Grolemund, 2017) for short, but helpful instructions on organizing your workflow with the RStudio IDE — especially the “Workflow” chapters basics (Chapter 3), style (Chapter 5), scripts and projects (Chapter 7), and getting help (Chapter 9).↩︎
For instance, this site and many other books, reports, and websites are created within this paradigm.↩︎
For concrete instructions on combining text and code in R, see Section 1.3.3 and Chapter 27: R Markdown of the r4ds textbook (Wickham & Grolemund, 2017), or Appendix F: Using R Markdown of the ds4psy textbook (Neth, 2023a).↩︎