Chapter 4 Estimation methods
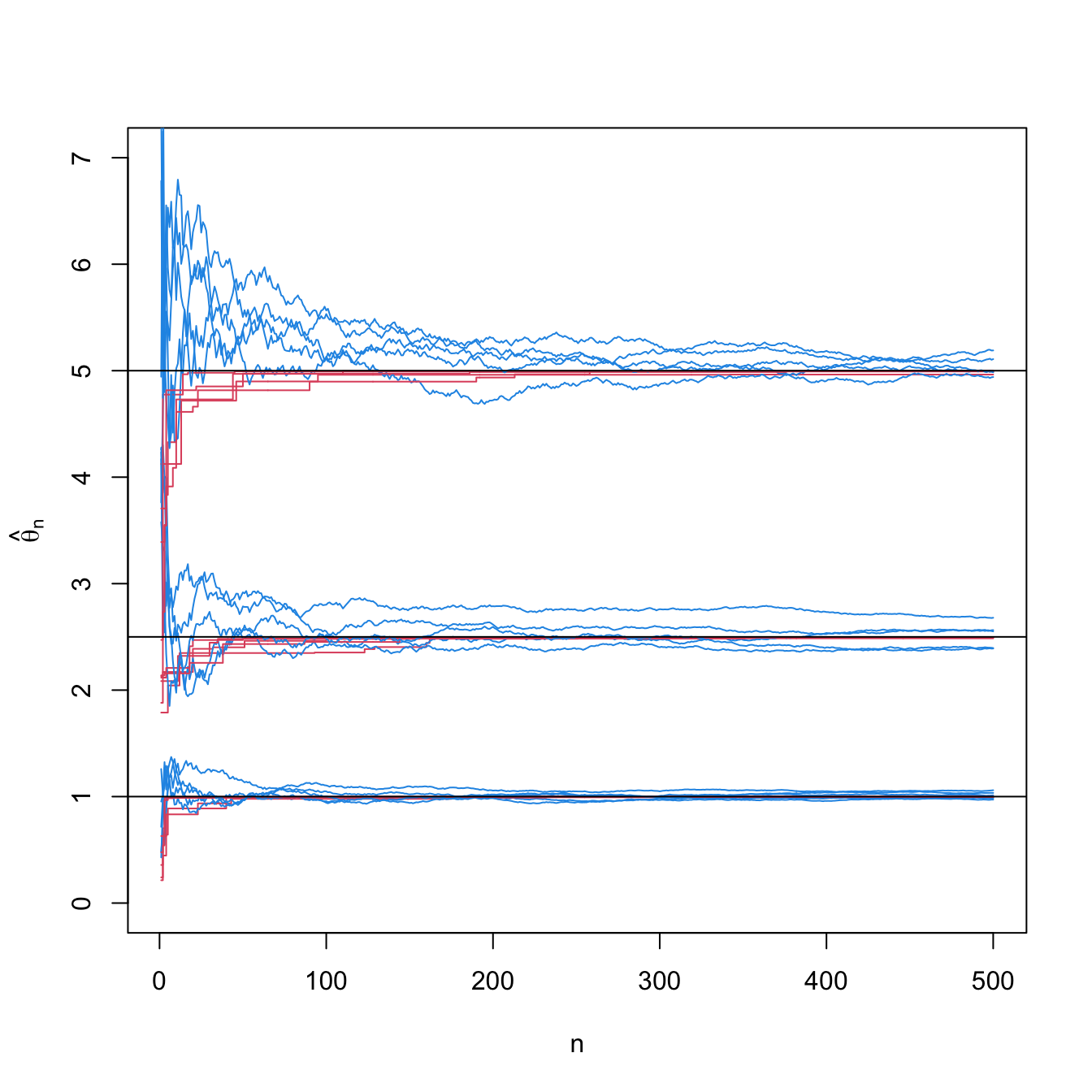
Figure 4.1: Convergence of the moment estimator ˆθMM (blue) and maximum likelihood estimator ˆθMLE (red) for θ in a U(0,θ) population. For θ=1,2.5,5, N=5 srs’s of sizes increasing until n=500 were simulated. The black horizontal lines represent the values of θ. The maximum likelihood estimator is more efficient than the moment estimator, as evidenced by the faster convergence of the former.
This chapter is devoted to arguably the two most popular approaches in statistical inference for estimating one or more parameters: the method of moments and maximum likelihood.
In this chapter we start embracing the more realistic case of estimating several parameters of the population, as opposed to the uniparameter view we have been mainly following so far.