5.1 The pivotal quantity method
Definition 5.1 (Confidence interval) Let \(X\) be a rv with induced probability \(\mathbb{P}(\cdot;\theta),\) \(\theta\in \Theta,\) where \(\Theta\subset \mathbb{R}.\) Let \((X_1,\ldots,X_n)\) be a srs of \(X.\) Let \(T_1=T_1(X_1,\ldots,X_n)\) and \(T_2=T_2(X_1,\ldots,X_n)\) be two unidimensional statistics such that
\[\begin{align} \mathbb{P}(T_1\leq \theta\leq T_2;\theta)\geq 1-\alpha, \quad \forall \theta\in\Theta.\tag{5.1} \end{align}\]
Then, the interval \(\mathrm{CI}_{1-\alpha}(\theta):=[T_1(x_1,\ldots,x_n),T_2(x_1,\ldots,x_n)]\) obtained for any sample realization \((x_1,\ldots,x_n)\) is referred to as a confidence interval for \(\theta\) at the confidence level \(1-\alpha\).
The value \(\alpha\) is denoted as the significance level. \(T_1\) and \(T_2\) are known as the inferior and the superior limits of the confidence interval for \(\theta,\) respectively. Sometimes the interest lies in only one of these limits.
Definition 5.2 (Pivot) A pivot \(Z(\theta)\equiv Z(\theta;X_1,\ldots,X_n)\) is a function of the sample \((X_1,\ldots,X_n)\) and the unknown parameter \(\theta\) that is bijective in \(\theta\) and has a completely known distribution.
The pivotal quantity method for obtaining a confidence interval consists in, once fixed the significance level \(\alpha\) desired to satisfy (5.1), find a pivot \(Z(\theta)\) and, using the pivot’s distribution, select two constants \(c_1\) and \(c_2\) such that
\[\begin{align} \mathbb{P}_Z(c_1\leq Z(\theta)\leq c_2)\geq 1-\alpha. \tag{5.2} \end{align}\]
Then, solving60 for \(\theta\) in the inequalities we obtain an equivalent probability to (5.2). If \(\theta\mapsto Z(\theta)\) is increasing, then (5.2) equals
\[\begin{align*} \mathbb{P}_Z(Z^{-1}(c_1)\leq \theta \leq Z^{-1}(c_2))\geq 1-\alpha, \end{align*}\]
so \(T_1=Z^{-1}(c_1)\) and \(T_2=Z^{-1}(c_2).\) If \(Z\) is decreasing, then \(T_1=Z^{-1}(c_2)\) and \(T_2=Z^{-1}(c_1).\) In any case, \([T_1,T_2]\) is a confidence interval for \(\theta\) at confidence level \(1-\alpha.\)
Usually, the pivot \(Z(\theta)\) can be constructed from an estimator \(\hat{\theta}\) of \(\theta.\) Assume that making a transformation of the estimator \(\hat{\theta}\) that involves \(\theta\) we obtain \(\hat{\theta}'.\) If the distribution of \(\hat{\theta}'\) does not depend on \(\theta,\) then we have that \(\hat{\theta}'\) is a pivot for \(\theta.\) For this process to work, it is key that \(\hat{\theta}\) has a known distribution61 that depends on \(\theta\): otherwise the constants \(c_1\) and \(c_2\) in (5.2) are not computable in practice.
The interpretation of confidence intervals has to be done with a certain care. Notice that in (5.1) the probability operator refers to the randomness of the interval \([T_1,T_2].\) This random confidence interval is said to contain the unknown parameter \(\theta\) “with a probability of \(1-\alpha\)”. Yet, in reality, either \(\theta\) belongs or does not belong to the interval, which seems contradictory. The previous quoted statement has to be understood in the frequentist sense of probability:62 when the confidence intervals are computed independently over an increasing number of samples,63 the relative frequency of the event “\(\theta\in\mathrm{CI}_{1-\alpha}(\theta)\)” converges to \(1-\alpha.\) For example, suppose you have 100 samples generated according to a certain distribution model depending on \(\theta.\) If you compute \(\mathrm{CI}_{1-\alpha}(\theta)\) for each of the samples, then in approximately \(100(1-\alpha)\) of the samples the true parameter \(\theta\) would be actually inside the random confidence interval. This is illustrated in Figure 5.2.
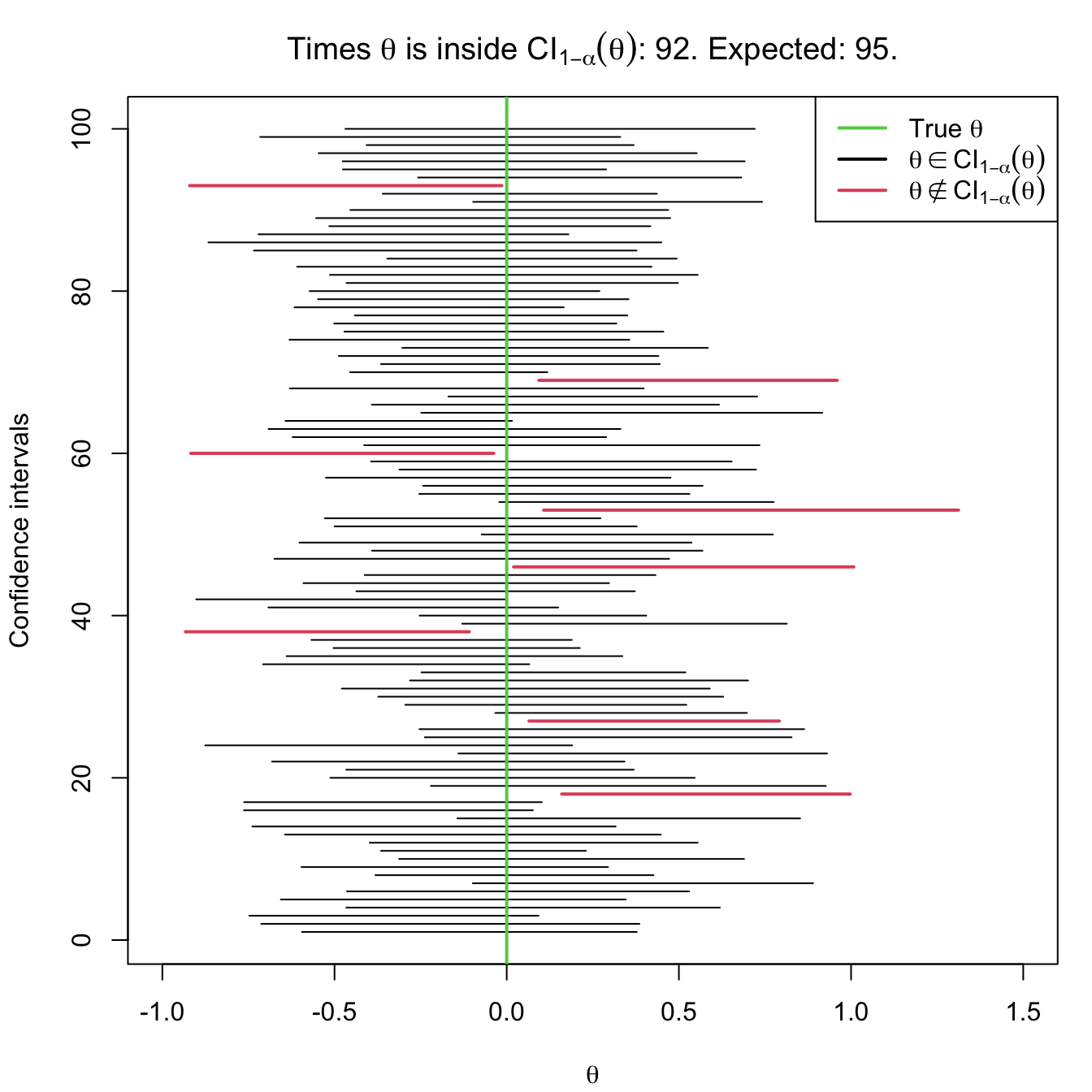
Figure 5.2: Illustration of the randomness of the confidence interval for \(\theta\) at the \(1-\alpha\) confidence. The plot shows 100 random confidence intervals for \(\theta,\) computed from 100 random samples generated by the same distribution model (depending on \(\theta\)).
Example 5.1 Assume that we have a single observation \(X\) of a \(\mathrm{Exp}(1/\theta)\) rv. Employ \(X\) to construct a confidence interval for \(\theta\) with a confidence level \(0.90.\)
We have a srs of size one and we need to find a pivot for \(\theta,\) that is, a function of \(X\) and \(\theta\) whose distribution is completely known. The pdf and the mgf of \(X\) are given by
\[\begin{align*} f_X(x)=\frac{1}{\theta}e^{-x/\theta}, \quad x>0, \quad m_X(s)=(1-\theta s)^{-1}. \end{align*}\]
Then, taking \(Z=X/\theta,\) the mgf of \(Z\) is
\[\begin{align*} m_Z(s)=m_{X/\theta}(s)=m_X(s/\theta)=\left(1-\theta\frac{s}{\theta}\right)^{-1}=(1-s)^{-1}. \end{align*}\]
Therefore, \(m_Z\) does not depend on \(\theta\) and, in addition, is the mgf of a rv \(\mathrm{Exp}(1)\) with pdf
\[\begin{align*} f_Z(z)=e^{-z}, \quad z> 0. \end{align*}\]
Then, we need to find two constants \(c_1\) and \(c_2\) such that
\[\begin{align*} \mathbb{P}(c_1\leq Z\leq c_2)\geq 0.90. \end{align*}\]
We know that
\[\begin{align*} \mathbb{P}(Z\leq c_1)&=\int_{0}^{c_1} e^{-z}\,\mathrm{d}z=1-e^{-c_1},\\ \mathbb{P}(Z\geq c_2)&=\int_{c_2}^{\infty} e^{-z}\,\mathrm{d}z=e^{-c_2}. \end{align*}\]
Splitting the probability \(0.10\) evenly in two,64 then
\[\begin{align*} 1-e^{-c_1}=0.05, \quad e^{-c_2}=0.05. \end{align*}\]
Solving for the \(c_1\) and \(c_2,\) we obtain
\[\begin{align*} c_1=-\log(0.95)\approx0.051,\quad c_2=-\log(0.05)\approx2.996. \end{align*}\]
Therefore, it is verified
\[\begin{align*} \mathbb{P}(c_1\leq X/\theta\leq c_2)= 0.9. \end{align*}\]
Solving \(\theta\) from the inequalities, we have
\[\begin{align*} \mathbb{P}(X/2.996\leq \theta\leq X/0.051)\approx 0.9, \end{align*}\]
so the confidence interval for \(\theta\) at significance level \(0.10\) is
\[\begin{align*} \mathrm{CI}_{0.90}(\theta)\approx[X/2.996,X/0.051]. \end{align*}\]
Therefore, it is key that \(Z\) is bijective in \(\theta\) to be invertible.↩︎
If the distribution of \(\hat{\theta}\) is only known asymptotically, then one can build an asymptotic confidence interval through the pivot method; see Section 5.4.↩︎
For fixed sample size! The \(n\) does not change. What is repeated is the extraction of new samples.↩︎
Other splittings are possible.↩︎