3.3 Powerful pipes
Although each of the essential dplyr commands is useful, the examples above have shown that we rarely encounter them in isolation. Instead, we can think of these commands as verbs of a language for basic data manipulation.
The so-called pipe operator %>%
of the magrittr package (Bache & Wickham, 2022) allows chaining commands in which the current result is passed to the next command (from left to right). With such forward pipes, seemingly simple commands become so powerful that we will rarely use just one command.
Whereas our introduction of essential dplyr commands in Section 3.2 relied on an intuitive understanding of the pipe, we should take a brief look at how pipes work and when we should use or avoid them.
3.3.1 Ceci est un pipe
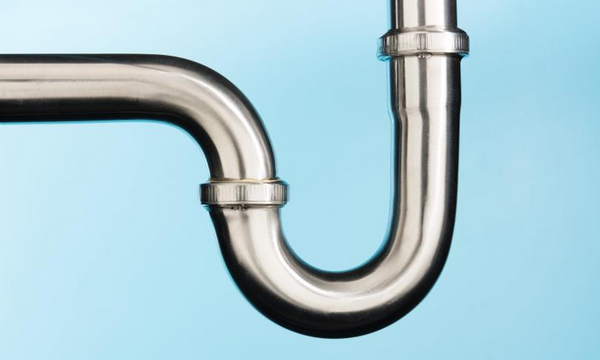
Figure 3.6: Ceci est un pipe: %>%
.
What is a pipe? While some people see pipes primarily as smoking devices, others are reminded about representational statements in art history (see Wikipedia: The treachery of images). In the applied sub-area of physics known as plumbing, a pipe is a device for directing fluid and solid substances from one location to another.
In R, the pipe is an operator that allows re-writing a nested call to multiple functions as a chain of individual functions.
Historically, the native forward pipe operator |>
of base R (introduced in R version 4.1.0, published on 2021-05-18) was preceded by the %>%
operator of the magrittr package (Bache & Wickham, 2022).
Despite some differences, both pipes allow turning a nested expression of function calls into a sequence of processing steps that is easier to understand and avoids the need for saving intermediate results.
An indicator of the pipe’s popularity is the existence of a corresponding keyboard shortcut.
When using the the RStudio IDE, typing the key combination Cmd + Shift + M
inserts the %>%
operator (or the |>
operator, when selecting “Select native pipe operator” in the Code
section of Global options
).
In the following examples, we mostly use the %>%
operator of magrittr, but most examples would be the same for the native pipe operator |>
.
Basic usage
For our present purposes, it is sufficient to think of the pipe operator %>%
as passing whatever is on its left (or left-hand-side, lhs
) to the first argument of the function on its right (or right-hand-side, rhs
):
Here, lhs
is an expression (i.e., typically an R function) that yields some value (e.g., a number, vector, or table),
and rhs
is an expression that uses this value as an input (i.e., as an argument of an R function).
This description sounds more complicated than it is in practice. Actually, we are quite familiar with R expressions that contain and combine multiple steps. But so far, we have been nesting them in arithmetic formulas (with parentheses indicating operator precedence) or in hierarchical function calls, as in:
Using the pipe operator %>%
of magrittr allows us to re-write the nested function calls into a linear chain of steps:
Thus, given three functions a()
, b()
and c()
, the following pipe would compute the result of the compound expression c(b(a(x)))
:
As the intermediate steps get longer and more complicated, we typically re-write the same pipe sequence as follows:
3.3.2 Example pipes
Whereas a description of the pipe operator may sound complicated, the underlying idea is quite simple: We often want to perform several operations in a row. This is familiar in arithmetic expressions. For instance, consider the following step-by-step instruction:
- Start with a number
x
(e.g.,x = 3
). Then,
- multiply it by 4,
- add 20 to the result,
- subtract~7 from the result, and finally
- take the result’s square root.
This instruction can easily be translated into the following R expression:
#> [1] 5
In this expression, the order of operations is determined by parentheses, arithmetic rules (e.g., left to right, multiplying before adding and substracting, etc.), and functions. Avoiding the infix operators *
and +
, we can re-write the expression as a sequence of R functions:
#> [1] 5
The order of function application is determined by their level of encapsulation in parentheses.
The pipe operator %>%
allows us re-writing the sequence of functions as a chain:
#> [1] 5
Note that this pipe is fairly close to the step-by-step instruction above, particularly when we re-format the pipe to span multiple lines:
#> [1] 5
Thus, the pipe operator lets us express chains of function applications in a way that matches their natural language description.
The dot notation (of magrittr)
If we find the lack of an explicit representation of each step’s result on the right hand side of %>%
confusing, we can re-write the magrittr pipe as follows:
# Arithmetic pipe (using the dot notation of magrittr):
x %>% prod(., 4) %>% sum(., 20, -7) %>% sqrt(.)
#> [1] 5
Here, the dot .
of the magrittr pipe operator is a placeholder for entering the result of the left (lhs
) on the right (rhs
).
Thus, the .
represents whatever was passed (or “piped”) from the left to the right (here: the current value of x
).
The key benefit of using the dot placeholder notation is that we may occasionally want the result of the left expression to be passed to a non-first argument of the right expression. When using R for statistics, a typical example consists in passing data to a linear model:
# The linear model:
lm(Sepal.Length ~ Petal.Length, data = iris)
# can be re-written as a pipe (with dot notation):
iris %>%
lm(Sepal.Length ~ Petal.Length, data = .)
As both of these expressions effectively call the same lm()
function, they also yield the same result:
#>
#> Call:
#> lm(formula = Sepal.Length ~ Petal.Length, data = .)
#>
#> Coefficients:
#> (Intercept) Petal.Length
#> 4.3066 0.4089
By contrast, the base R pipe operator |>
does not support the dot notation:
#> Error: object '.' not found
#> Error in eval(mf, parent.frame()): object '.' not found
Another difference between both operators is that the magrittr pipe %>%
allows dropping the parentheses when calling a function with no other arguments, whereas the native pipe |>
always requires the parentheses.
(See Differences between the base R and magrittr pipes (by Hadley Wickham, 2023-04-21) for additional details.)
The pipe vs. assignment
As mentioned above, we must not confuse the pipe with R’s assignment operator <-
.
Although %>%
or |>
may look and seem somewhat similar to the assignment operator <-
(which also works as =
or ->
), they are and provide different functions.
Importantly, the pipe does not assign new objects, but rather apply a function to an existing object that then may serve as an input of another function.
The concrete input object changes every time a function is being applied and eventually results in an output object.
What happens if we tried to use a pipe to assign something to a new object y?
Assuming there is no function y()
defined in our current environment, the following code does not assign anything to y
, but instead yields an error:
# The pipe does NOT assign objects:
# ERROR with base R pipe:
x |> y
# ERROR with magrittr pipe:
x %>% y
#> Error in y: The pipe operator requires a function call as RHS (<input>:4:6)
Thus, for actually assigning the result of a pipe to an object y
, we need to use our standard assignment operator on the left (or at the beginning) of the pipe:
#> [1] 5
The following also works, but using <-
(above) is clearer than using ->
:
# Pipe and alternative assignment by `->`:
x %>%
prod(4) %>%
sum(20, -7) %>%
sqrt() -> y
y # evaluates to:
#> [1] 5
3.3.3 Evaluation
Overall, the effects of the pipe operators %>%
and |>
are similar to introducing a new mathematical notation:
They do not allow us to do anything we could not do before, but allow us re-writing chains of commands in a more natural, sequential fashion.
Essentially, embedded or nested calls of functions within functions are untangled into a linear chain of processing steps.
This only re-writes R expressions, but in a way that corresponds more closely to how we think about them (i.e., making them easier to parse and understand).
Piped expressions are particularly useful when generating and transforming data objects (e.g., vectors or tables) by a series of functions that all share the same type of inputs and outputs (e.g., vectors or tables). As using the pipe avoids the need for saving intermediate objects, it makes complex sequences of function calls easier to construct, write, and understand.
While using pipes can add convenience and reduce complexity, these benefits also have some costs.
From an applied perspective, a key requirement for using the pipe is that we must be aware of the data structures serving as inputs and outputs at each step.
More importantly, piping functions implies that we do not need a record of all intermediate results.
Whenever the results of intermediate steps are required later, we still have to assign them to corresponding objects.
From a programming perspective, enabling piped expressions requires that R functions accept some key input as their first argument (unless we use the .
notation of magrittr).
Fortunately, most R functions are written in just this way, but it is something to keep in mind when writing our own functions.
When (not) to pipe
Stringing together several dplyr commands allows slicing and dicing data (tibbles or data frames) in a step-wise fashion to run non-trivial data analyses on the fly. As such pipes do not only work with dplyr verbs, but with all R packages that define functions in which key data structures appear as the first argument of its functions, we will soon solve quite sophisticated tasks by chaining together simple commands. For instance, pipes allow selecting and sorting sub-groups of data, computing descriptive statistics (e.g., counts, mean, median, standard deviation, etc.), and provide details about specific variables (e.g., describe their statistics and visualize them) by a sequence of simple commands.
Unfortunately, not all functions in R can be used with the pipe.
While the tools in the tidyverse are designed to support piping (see The tidy tools manifesto, e.g., by evaluating vignette('manifesto')
), there are many functions in R that do not.
And before getting overly enthusiastic about pipes, we should realize that they are fundamentally linear and typically link one input to one output.
Thus, pipes are wonderful tools whenever we start with some data table and incrementally transform it to obtain some specific output object (e.g., some resulting value, table, or graph). However, when we are solving tasks that routinely involve multiple inputs or multiple outputs, pipes are probably not the best tools to use.
In summary, pipes are great tools for transforming vectors or data tables, but we mostly use them for linear sequences of tidyverse commands that have one input and one output. Let’s practice them further by draining our sws
data table by various pipes.
Practice
Try to answer each of the following questions by a pipe of dplyr commands:
What is the number and mean height and mass of individuals from Tatooine by species and gender?
Which humans are more than 5cm taller than the average human overall?
Which humans are more than 5cm taller than the average human of their own gender?
Solution
Here are possible ways of answering these questions:
# What is the number and mean height and mass of individuals
# from Tatooine (filter) by species and gender (groups):
sws %>%
filter(homeworld == "Tatooine") %>%
group_by(species, gender) %>%
summarise(count = n(),
mn_height = mean(height),
mn_mass = mean(mass, na.rm = TRUE)
)
# Which humans are more than 5cm taller than
# the average human overall (filter humans,
# then compute mean and test for taller individuals):
sws %>%
filter(species == "Human") %>%
mutate(mn_height = mean(height, na.rm = TRUE),
taller = height > mn_height + 5) %>%
filter(taller == TRUE)
# Which humans (filter) are more than 5cm taller
# than the average human of their own gender
# (first group by gender, then mutate):
sws %>%
filter(species == "Human") %>%
group_by(gender) %>%
mutate(mn_height_2 = mean(height, na.rm = TRUE),
taller_2 = height > mn_height_2 + 5) %>%
filter(taller_2 == TRUE)
3.3.4 More about pipes
Additional details and opinions on the pipe operator are available at the following links:
- see
vignette('magrittr')
of the magrittr package (Bache & Wickham, 2022)
- read Chapter 18: Pipes of the r4ds textbook
- for programming with pipes, see this discussion at stackoverflow
For comparisons between the base R pipe |>
and the magrittr pipe, see:
- New features in R 4.1.0 (by Russ Hyde, 2021-05-17)
- The new R pipe (by Elio Campitelli, 2021-05-25)
- Differences between the base R and magrittr pipes (by Hadley Wickham, 2023-04-21)