15 Comparing quantitative data between individuals
So far, you have learnt to ask a RQ, design a study, collect the data, and describe the data. In this chapter, you will learn to compare quantitative data in different groups. You will learn to:
- compare quantitative data between individuals using the appropriate graphs.
- compare quantitative between within individuals in summary tables.
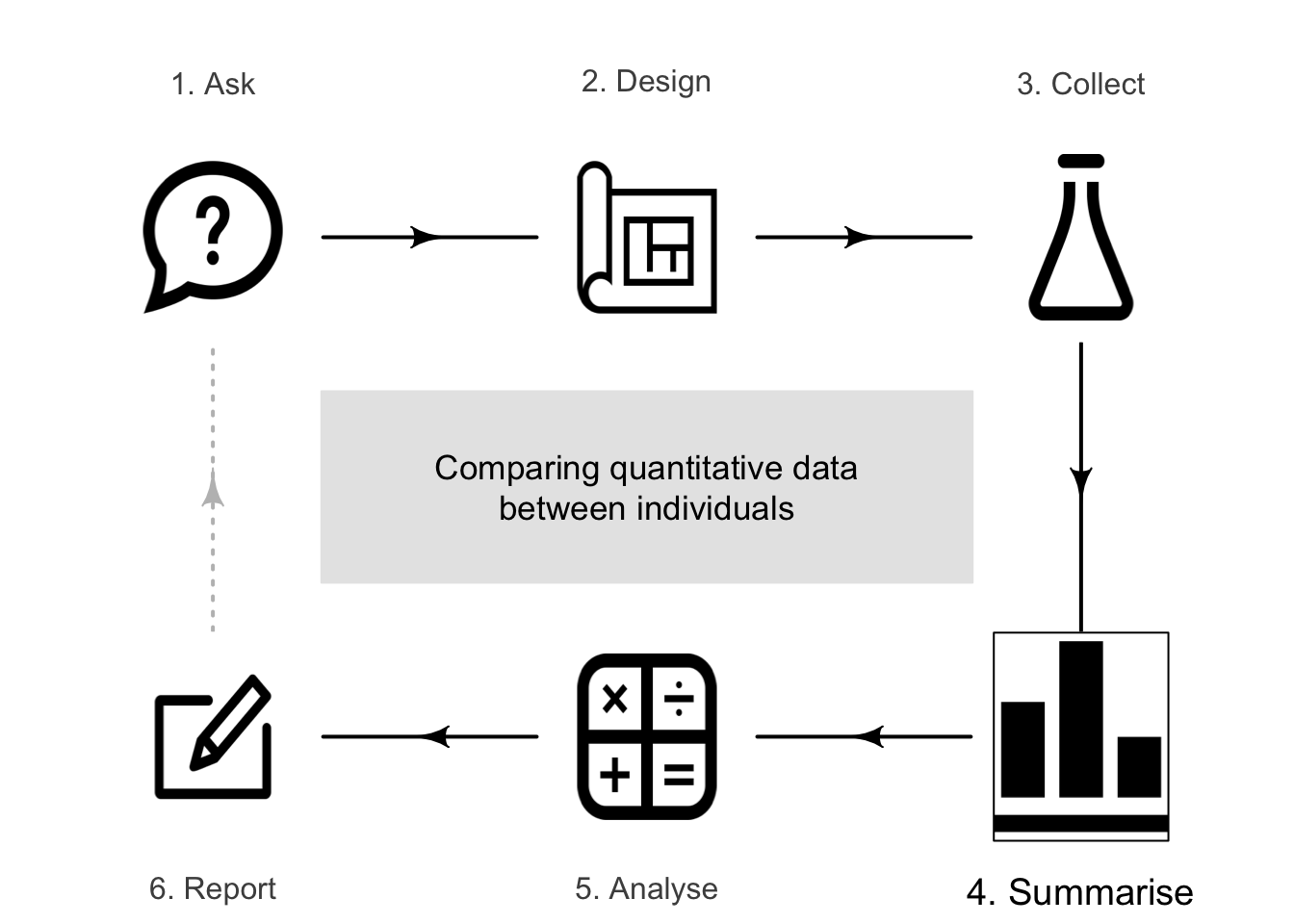
15.1 Introduction
Relational RQs compare groups. This chapter considers how to compare qualitative variables in different groups. Graphs are very useful this purpose, and a table of the numerical summaries usually are also produced.
15.2 Summarising the comparison: difference between means
The best way to compare the two groups is to summarise the difference between the two means. A numerical summary table can be constructed summarising both group, and reporting the difference between the two means.
Example 15.1 (Numerical summary table) Wright et al. (2021) recorded the number of chest-beats by gorillas (Table 15.1), for gorillas under \(20\) years old ('younger') and \(20\) years and over ('older'). For the gorilla data, the summary of the data can be tabulated as in Table 15.2. Notice that no standard deviation or sample size is provided for the difference; these make no sense.
\(0.7\) | \(1.5\) | \(1.7\) | \(2.6\) | \(4.4\) | \(0.0\) | \(0.3\) | \(0.8\) | \(1.6\) |
\(0.9\) | \(1.5\) | \(1.7\) | \(3.0\) | \(4.4\) | \(0.1\) | \(0.4\) | \(0.9\) | \(4.0\) |
\(1.3\) | \(1.5\) | \(1.8\) | \(4.1\) | \(0.2\) | \(0.6\) | \(1.1\) |
(in beats per 10 h) | (in beats per 10 h) | size | |
---|---|---|---|
Younger | \(2.22\) | \(1.270\) | \(14\) |
Older | \(0.91\) | \(1.131\) | \(11\) |
Difference | \(1.31\) |
15.3 Graphs
When a quantitative variable is measured or observed in different groups (i.e., between individuals), the distribution of each variable can be graphed separately. However, graphs for comparing the quantitative variable in the groups include:
- Back-to-back stemplots: Best for small amounts of data in two groups;
- 2-D dot charts: Best choice for small to moderate amounts of data;
- Boxplots: Best choice, except for small amounts of data.
These situations have one quantitative variable being compared in different groups (defined by one qualitative variable).
15.3.1 Back-to-back stemplot
Back-to-back stemplots are two stemplots (Sect. 11.3.2) sharing the same stems; one group has the leaves emerging left-to-right from the stem, and the second group has the leaves emerging right-to-left from the stem. Back-to-back stemplots can only be used when two groups are being compared. Again, one advantage of using stemplots over other plots is that the original data are retained. Disadvantage are that only two groups can be compared, and not all data work well with stemplots.
Example 15.2 (Back-to-back stemplots) A back-to-back stemplot for comparing the chest-beating rate of gorillas (Fig. 15.1) has the leaves for younger gorillas right-to-left, and the leaves for older gorillas left-to-right, sharing the same stems. The younger gorillas have a faster chest-beating rate in general. One older gorilla has a much faster rate that the other older gorillas (a potential outlier).

FIGURE 15.1: Stemplot for the chest-beating rate for gorillas
15.3.2 2-D dot charts
A 2-dimensional (2-D) dot chart places a dot for each observation, separated for each level of the qualitative variable (also see Sect. 12.3.1). Any number of groups can be compared.
The axis displaying the counts (or percentages) should start from zero, since the distance of the dots from the axis visually implies the frequency of those observations (see Example 17.1).
Example 15.3 (Boxplots) For the chest-beating data seen in Example 15.2, a dot chart is shown in Fig. 15.2. Many observations are the same, so some points would be overplotted if points were not stacked (left panel), or jittered (right panel).
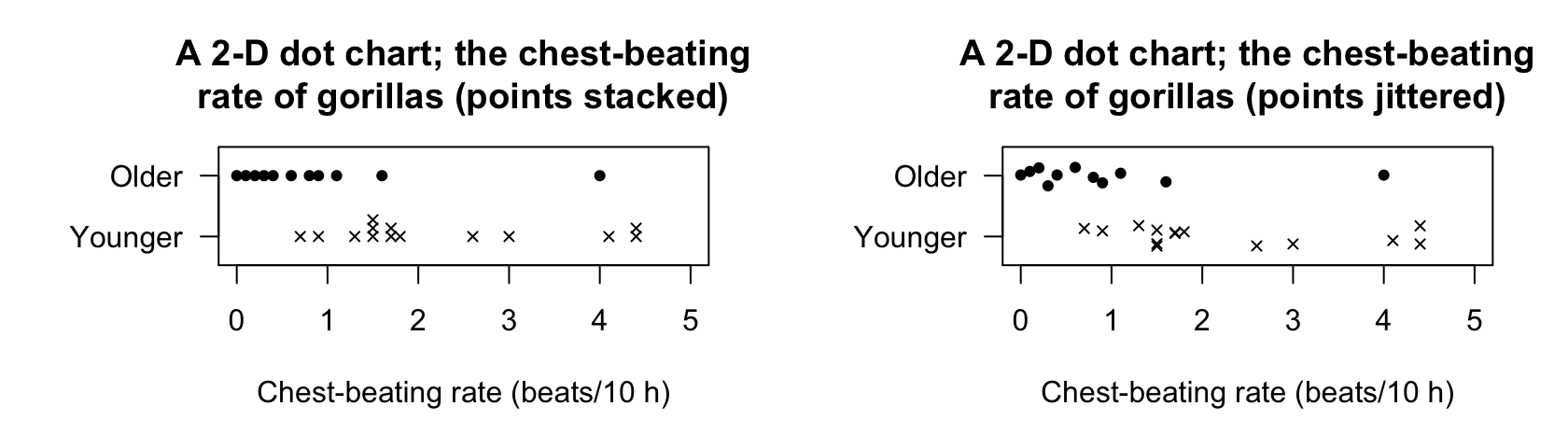
FIGURE 15.2: Two variations of a 2-D dot chart for the chest-beating data to avoid overplotting: stacking (left) and jittering (right)
15.3.3 Boxplots
A boxplot is a picture of the quantiles (Sect. 11.6.3) of each group, drawn together on the same plot (and so are sometimes called parallel boxplots). Any number of groups can be compared using a boxplot.
The distribution for each group is summarised by five numbers: the minimum value; the first quartiel (\(Q_1\)); the median (\(Q_2\)); the third quartile (\(Q_3\)); and the maximum value. Outliers, identified using the IQR rule (Sect. 11.7.2), are usually shown too. The values of \(Q_1\), the medians, and \(Q_3\) can be used to compare the distributions. Different software may use different rules for computing quartiles, and hence may produce slightly different boxplots.
The axis displaying these five numbers need not start from zero, since the distance from the axis to the these numbers do not visually imply any quantity of interest. Rather, the boxes display the values of these five numbers for each group relative to each other, which is of interest.
Boxplots summarise data with only five numbers, so detail of the distributions are lost. For this reason, boxplots are excellent for comparing distributions, but histograms are better for displaying the distribution of a single quantitative variable.
Example 15.4 (Boxplots) The boxplot for the chest-beating data (Example 15.2) is shown in Fig. 15.3. No outliers are identified for younger gorillas; one large outlier is identified for the older gorillas. The boxplot shows a distinct difference between the chest-beating rates of older and younger gorillas.
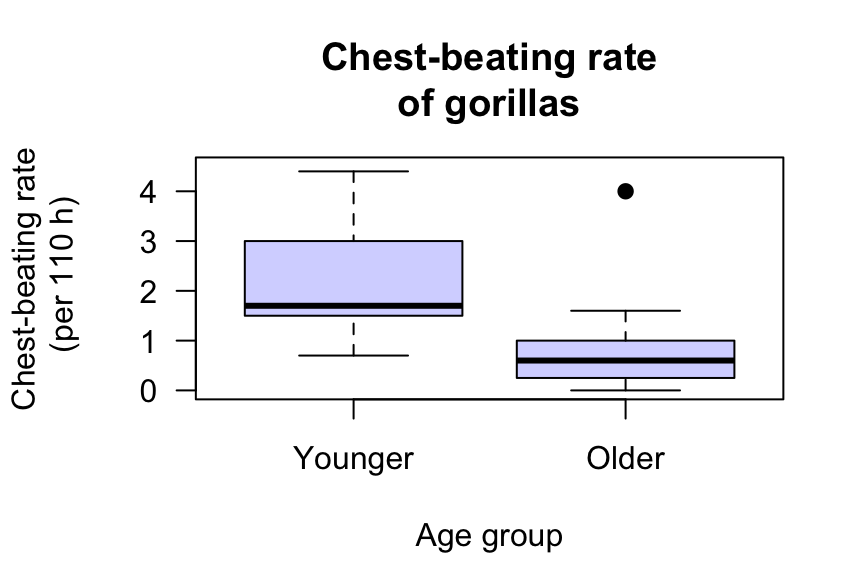
FIGURE 15.3: A boxplot for the chest-beating data
The boxplots are explained in Fig. 15.4. First focus on just the boxplot for the younger gorillas (i.e., the left box). Boxplots have five horizontal lines; from the top to the bottom of the plot:
- Top line: The fastest chest-beating rate is \(4.4\) per \(10\) h.
- Second line from top: \(75\)% of observations are smaller than about \(3\), represented by the line at the top of the central box. This is the third quartile (\(Q_3\)).
- Middle line: \(50\)% of observations are smaller than about \(1.7\), represented by the line inside the central box. This is an 'average' value, the second quartile (\(Q_2\)).
- Second line from bottom: \(25\)% of observations are smaller than about \(1.5\), represented by the line at the bottom of the central box. This is the first quartile (\(Q_1\)).
- Bottom line: The slowest chest-beating rate is \(0.7\) per \(10\) h.
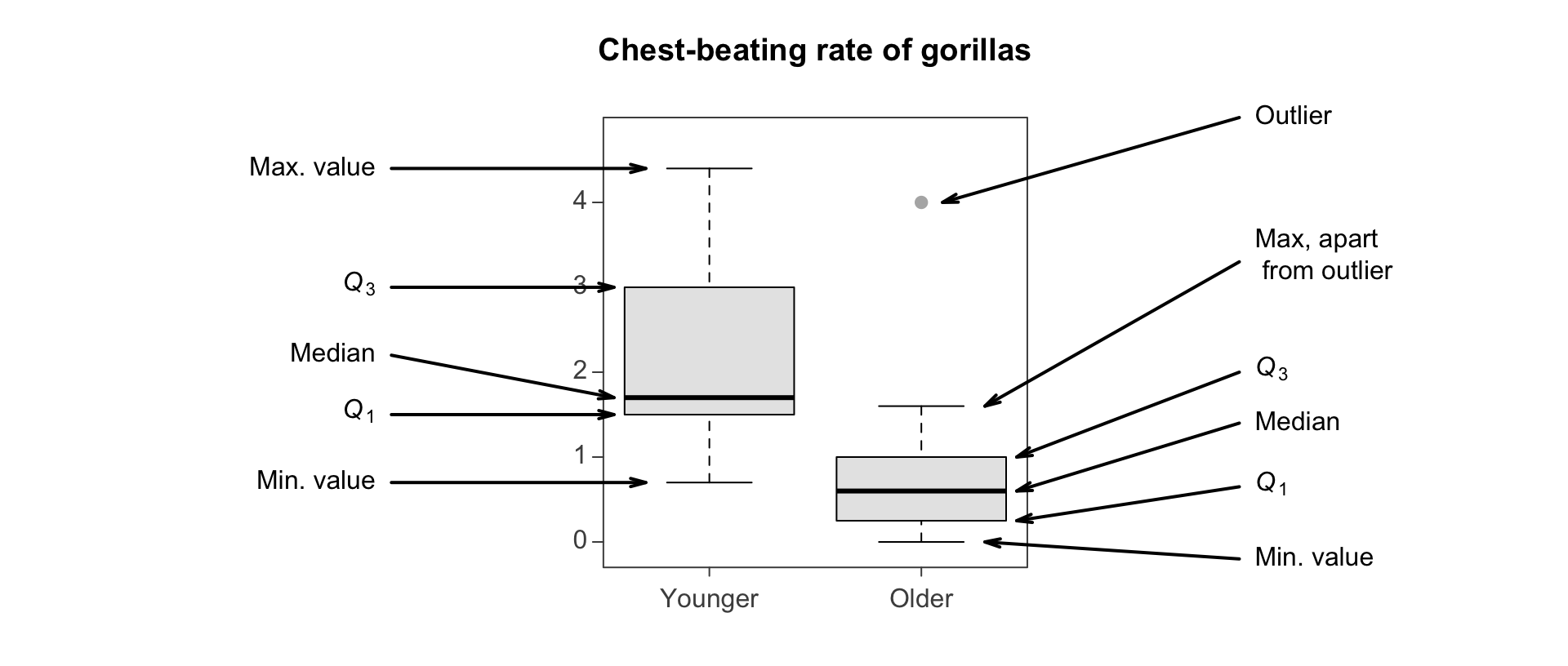
FIGURE 15.4: Explaining the boxplots for the chest-beating data
The box for the older gorillas is slightly different (Fig. 15.3): one observation is identified with a point, above the top line. Computer software has identified this observation as a potential (large) outlier using the IQR rule (Sect. 11.7.2), and has plotted this point separately.
The values of \(Q_1\), the median and \(Q_3\) are all substantially larger for the younger gorillas, suggesting that younger gorillas have, in general, faster chest-beating rates.
Example 15.5 (Boxplots) Boxplots can be plotted horizontally too, which leaves space for long labels. In Fig. 15.5 (based on Silva et al. (2016)), the three dental cements are very different regarding their push-out forces.
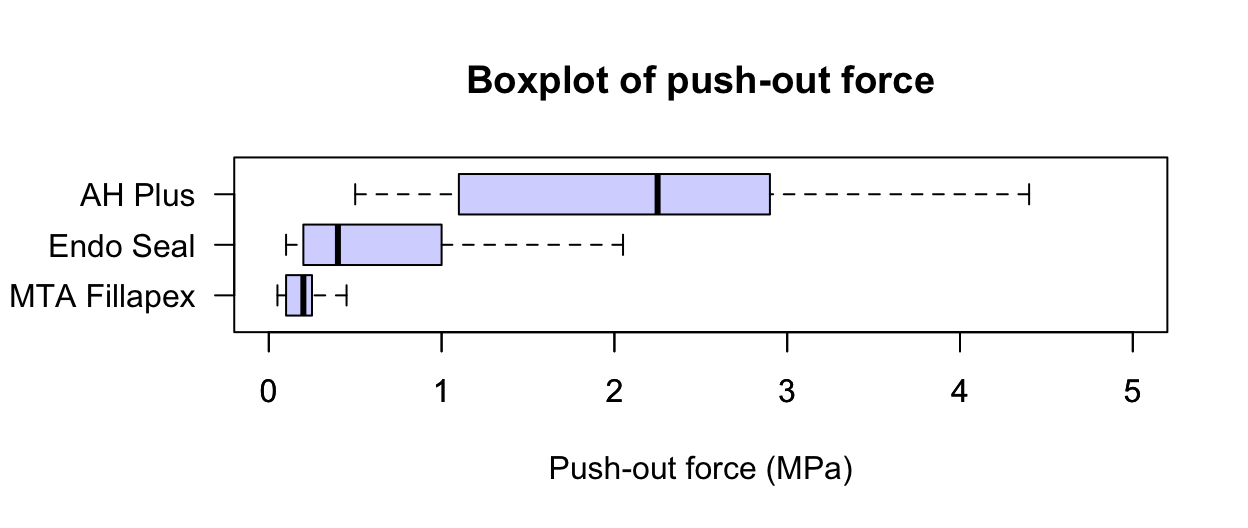
FIGURE 15.5: Comparing three push-out values for three dental cements
15.4 Example: water access
López-Serrano et al. (2022) recorded data about access to water in three rural communities in Cameroon (Sect. 11.9). One purposes of the study was to determine contributors to the incidence of diarrhoea in young children (\(85\) households had children under \(5\)).
The graphs (Fig. 15.6) and summary (Table 15.3) show that households in which diarrhoea was found in the last two weeks in children had older coordinators, more people in the household, and more children under \(5\) in the household. The may be expected: older female coordinators probably have more children, have more children in the household under \(5\), and more children (and hence people) in the household in general.
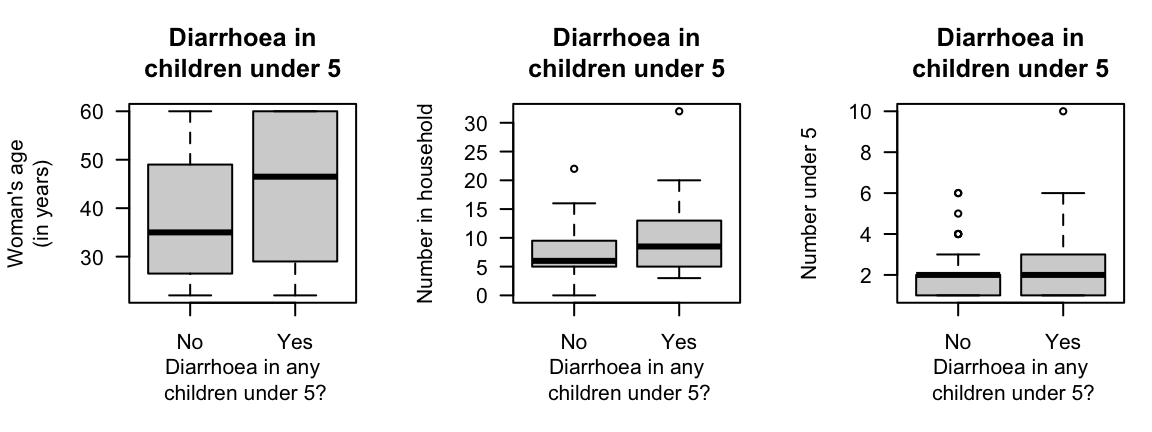
FIGURE 15.6: Some plots for the water access data in 85 households (\(59\) household reported no diarrhoea in children under \(5\); \(26\) reported diarrhoea in children under \(5\)).
\(n\) | Mean | Median | Std. dev. | IQR | |
---|---|---|---|---|---|
Woman's age | |||||
All households with children | \(85\) | \(40.2\) | \(37.0\) | \(13.90\) | \(28.00\) |
Incidents of diarrhoea | \(26\) | \(45.0\) | \(46.5\) | \(14.04\) | \(28.50\) |
No incidents of diarrhoea | \(59\) | \(38.1\) | \(35.0\) | \(13.44\) | \(22.50\) |
Household size | |||||
Difference | \(\phantom{0}6.8\) | ||||
All households with children | \(85\) | \(\phantom{0}8.4\) | \(\phantom{0}7.0\) | \(\phantom{0}4.93\) | \(\phantom{0}6.00\) |
Incidents of diarrhoea | \(26\) | \(10.5\) | \(\phantom{0}8.5\) | \(\phantom{0}6.51\) | \(\phantom{0}7.75\) |
Children under 5 in household | |||||
No incidents of diarrhoea | \(59\) | \(\phantom{0}7.5\) | \(\phantom{0}6.0\) | \(\phantom{0}3.78\) | \(\phantom{0}4.50\) |
Difference | \(\phantom{0}2.9\) | ||||
All households with children | \(85\) | \(\phantom{0}2.2\) | \(\phantom{0}2.0\) | \(\phantom{0}1.56\) | \(\phantom{0}2.00\) |
Incidents of diarrhoea | \(26\) | \(\phantom{0}2.8\) | \(\phantom{0}2.0\) | \(\phantom{0}2.01\) | \(\phantom{0}1.75\) |
No incidents of diarrhoea | \(59\) | \(\phantom{0}1.9\) | \(\phantom{0}2.0\) | \(\phantom{0}1.26\) | \(\phantom{0}1.00\) |
Difference | \(\phantom{0}0.8\) |
15.5 Chapter summary
Quantitative data can be compared between different groups (between individuals comparisons) using a back-to-back stemplot, boxplot or \(2\)-D dot chart. A summary table should show the numerical summaries for the quantitative variable at each comparison and the between-group differences.
15.6 Quick review questions
Are the following statements true or false?
- A boxplot is an appropriate graph for comparing a quantitative variable in two or more groups.
- A back-to-back stemplot is an appropriate graph for comparing a quantitative variable in two or more groups.
- A case-profile plot is an appropriate graph for comparing a quantitative variable in two or more groups.
- When comparing a quantitative variable in two or more groups, the sample size for the difference should be included
15.7 Exercises
Answers to odd-numbered exercises are available in App. E.
Exercise 15.1 Hale et al. (2009) studied different engineering project delivery methods (Fig. 15.7, left panel). The grey, horizontal line is where the projected costs are the same as the actual cost.
What does the plot reveal about the two methods?
Exercise 15.2 [Dataset: AISsub
]
Telford and Cunningham (1991) studied athletes at the Australian Institute of Sport (AIS).
Numerous physical and blood measurements were taken from high performance athletes.
Figure 15.7 (right panel) compares the heights of females in two similar sports: basketball and netball.
(Netball was derived from basketball.)
How would you interpret the graph, regarding the heights of the athletes in the two sports?
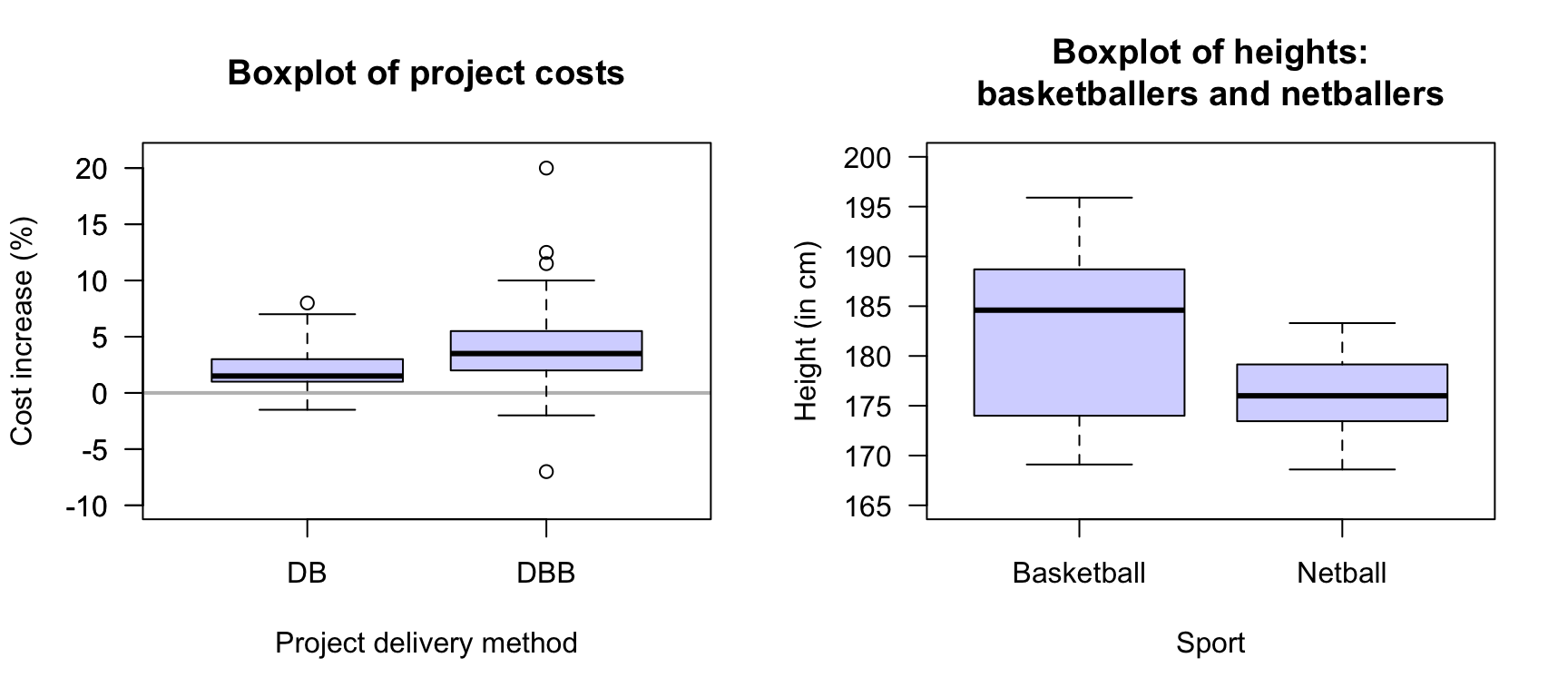
FIGURE 15.7: Left: cost increases for two different building project delivery methods (the grey, horizontal line is where the projected costs are the same as the actual cost). Right: the heights of female basketball and netball players attending the AIS.
Exercise 15.3 Match the histograms with the corresponding boxplots in the activity below.
Exercise 15.4 Gatti et al. (2013) studied the productivity of construction workers, recording (among other things) the rate at which concrete panels could be installed by workers. Data for three different female workers in the study are shown in Table 15.4. Construct the boxplot comparing the three workers. What does it tell you?
Worker 1 | Worker 2 | Worker 3 | |
---|---|---|---|
Mean | \(1.24\) | \(1.73\) | \(1.36\) |
Minimum | \(0.59\) | \(1.13\) | \(0.86\) |
1st quartile | \(0.88\) | \(1.51\) | \(1.16\) |
Median | \(1.35\) | \(1.70\) | \(1.38\) |
3rd quartile | \(1.49\) | \(1.91\) | \(1.58\) |
Maximum | \(1.88\) | \(3.00\) | \(2.17\) |
Range | \(1.28\) | \(1.87\) | \(1.31\) |
Exercise 15.5 In a study of the temperature in offices, Paul and Taylor (2008) compared the temperature in three offices (during working hours) at Charles Sturt University (Australia); the data are summarised in Table 15.5. Using this information, draw the boxplot comparing the three offices. What do we learn from this graph?
Office A | Office B | Office C | |
---|---|---|---|
Mean | \(24.1\) | \(25.3\) | \(25.7\) |
Minimum | \(16.4\) | \(15.9\) | \(20.1\) |
\(Q_1\) | \(22.8\) | \(23.8\) | \(24.6\) |
Median | \(24.4\) | \(25.5\) | \(26.1\) |
\(Q_3\) | \(25.5\) | \(26.9\) | \(27.2\) |
Maximum | \(27.4\) | \(31.0\) | \(30.3\) |
Exercise 15.6 Swinnen et al. (2018) studied the use of ankle-foot orthoses in children with cerebral palsy. Table 10.3 describe the \(15\) subjects. (GMFCS is an ordinal variable used to describe the impact of cerebral palsy on their motor function: the Gross Motor Function Classification System.)
Sketch a graphs to explore the relationship between the children's weight and GMGCS.
Exercise 15.7 [Dataset: NHANES
]
Consider this RQ:
Among Americans, is the mean direct HDL cholesterol different for current smokers and non-smokers?
Data to answer this RQ is available from the American National Health and Nutrition Examination Survey (NHANES) (Pruim 2015). Use the software output (Fig. 15.8) to construct an appropriate table showing the numerical summary relevant to the RQ.

FIGURE 15.8: Software output for the NHANES data
Exercise 15.8 [Dataset: ForwardFall
]
Wojcik et al. (1999) compared the lean-forward angle in younger and older women.
An elaborate set-up was constructed to measure this angle, using a harnesses.
Consider the RQ:
Among healthy women, what is difference between the mean lean-forward angle for younger women compared to older women?
The data are shown in Table 15.6.
- What is an appropriate graph to display the data?
- Construct an appropriate numerical summary from the software output (Fig. 15.9).
\(29\) | \(34\) | \(33\) | \(27\) | \(28\) | \(18\) | \(15\) | \(23\) | \(13\) | \(12\) |
\(32\) | \(31\) | \(34\) | \(32\) | \(27\) |

FIGURE 15.9: Software output for the lean-forward angles data
Exercise 15.9 [Dataset: Speed
]
Ma et al. (2019) studied adding additional signage to reduce vehicle speeds on freeway exit ramps.
At one site (Ningxuan Freeway), speeds were recorded for \(38\) vehicles before the extra signage was added, and then for \(41\) different vehicles after the extra signage was added
(data below).
The researchers are hoping that the addition of extra signage will reduce the mean speed of the vehicles. The RQ is:
At this freeway exit, how much is the mean vehicle speed reduced after extra signage is added?
- Using the software output in Fig. 15.10, summarise the data numerically, then construct a summary table.
- Use a computer to produce a boxplot of the data.

FIGURE 15.10: Software output for the speed data
Exercise 15.10 [Dataset: Deceleration
]
Ma et al. (2019) studied adding additional signage to reduce vehicle speeds on freeway exit ramps.
At one site (Ningxuan Freeway), speeds were recorded at various points on the freeway exit for \(38\) vehicles before the extra signage was added, and then for \(41\) vehicles after the extra signage was added.
From this data, the deceleration of each vehicle was determined (data below) as the vehicle left the \(120\) km/h speed zone and approached the \(80\) km/hr speed zone. Use the data, and the summary in Table 15.7, to address this RQ:
At this freeway exit, what is the difference between the mean vehicle deceleration, comparing the times before the extra signage is added and after extra signage is added?
In this context, the researchers are hoping that the extra signage might cause cars to slow down faster (i.e., they will decelerate more, on average, after adding the extra signage).
- Construct a numerical summary table for the data.
- Use software to construct a boxplot of the data.
Mean | Std. dev. | Sample size | Std. error | |
---|---|---|---|---|
After | \(\phantom{0}0.0745\) | \(0.0494\) | \(38\) | \(0.0080\) |
Before | \(\phantom{0}0.0765\) | \(0.0521\) | \(41\) | \(0.0081\) |
Difference | \(\phantom{0}0.0020\) | \(0.0114\) |
Exercise 15.11 [Dataset: Typing
]
The Typing
dataset contains information about the typing speed and accuracy for students, from an online typing test (Pinet et al. 2022).
The four variables include are: typing speed (mTS
), typing accuracy (mAcc
), age (Age
), and sex (Sex
) for \(1301\) students.
- Produce appropriate numerical summaries for the quantitative variables.
- Produce appropriate numerical summaries for comparing the quantitative variables for different values of the qualitative variable.
- What do you learn from these numerical summaries?
Exercise 15.12 Lunn and McNeil (1991; Hand et al. 1996) compared the dimensions of jellyfish at two sites at Hawkesbury River, NSW (Dangar Island; Salamander Bay) to determine the difference between the jellyfish at each site. A histogram of the breadth of jellyfish at Dangar Island Bay is shown in Fig. 15.11 (left panel).
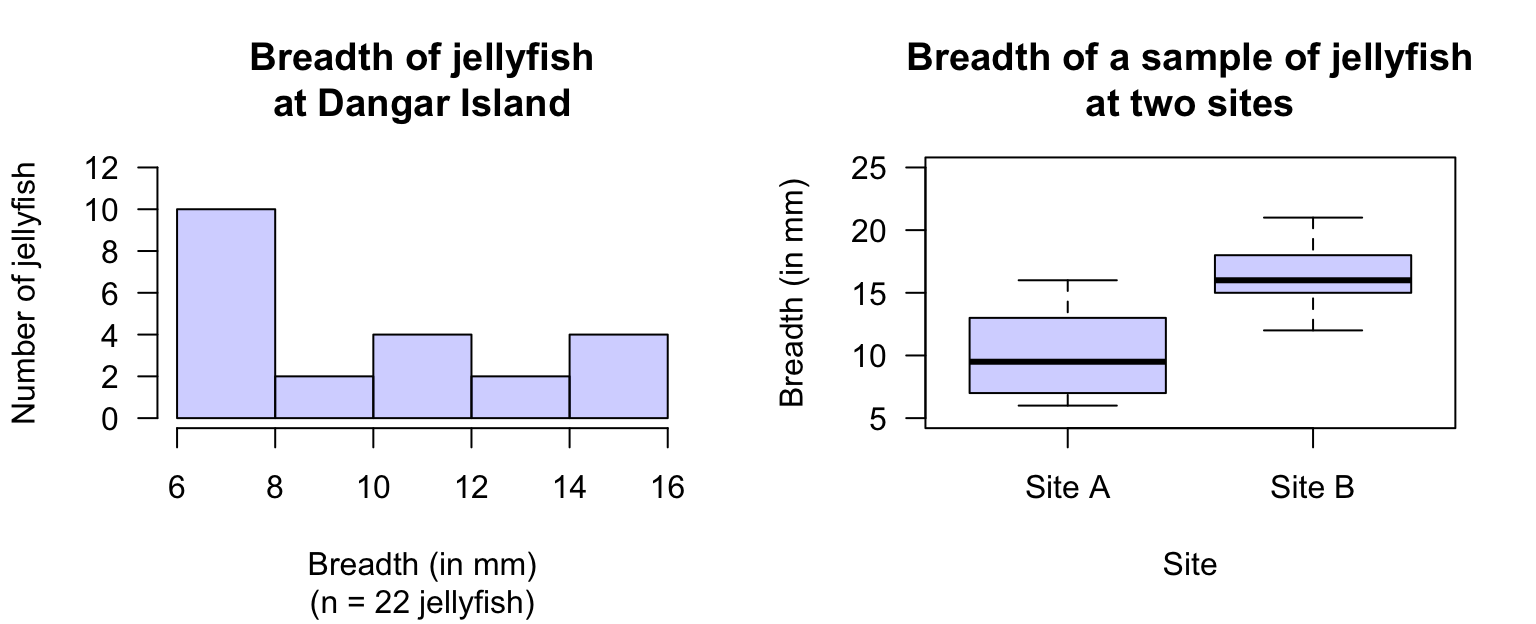
FIGURE 15.11: Left: A histogram of the breadth of jellyfish at Dangar Island. Right: A boxplot of the breadth of jellyfish at two sites.
- Two students are arguing about the median breadth. Who is correct?
Student 1 says:
The bars in the histogram have heights of \(10\), \(2\), \(4\), \(2\) and \(4\). When these numbers are put in order, they are: \(2\), \(2\), \(4\), \(4\), \(10\). The median breadth is the median of these numbers, so the median breadth is the middle one: \(4\) mm is the median.
Student 2 responds:
You have the correct answer, but for the wrong reason! There are five bars, and the middle bar is the third bar. Since the third bar has a height of \(4\), the median breadth is \(4\) mm.