4.4 Assumptions of the model
Some probabilistic assumptions are required for performing inference on the model parameters \boldsymbol{\beta} from the sample (\mathbf{X}_1, Y_1),\ldots,(\mathbf{X}_n, Y_n). These assumptions are somehow simpler than the ones for linear regression.
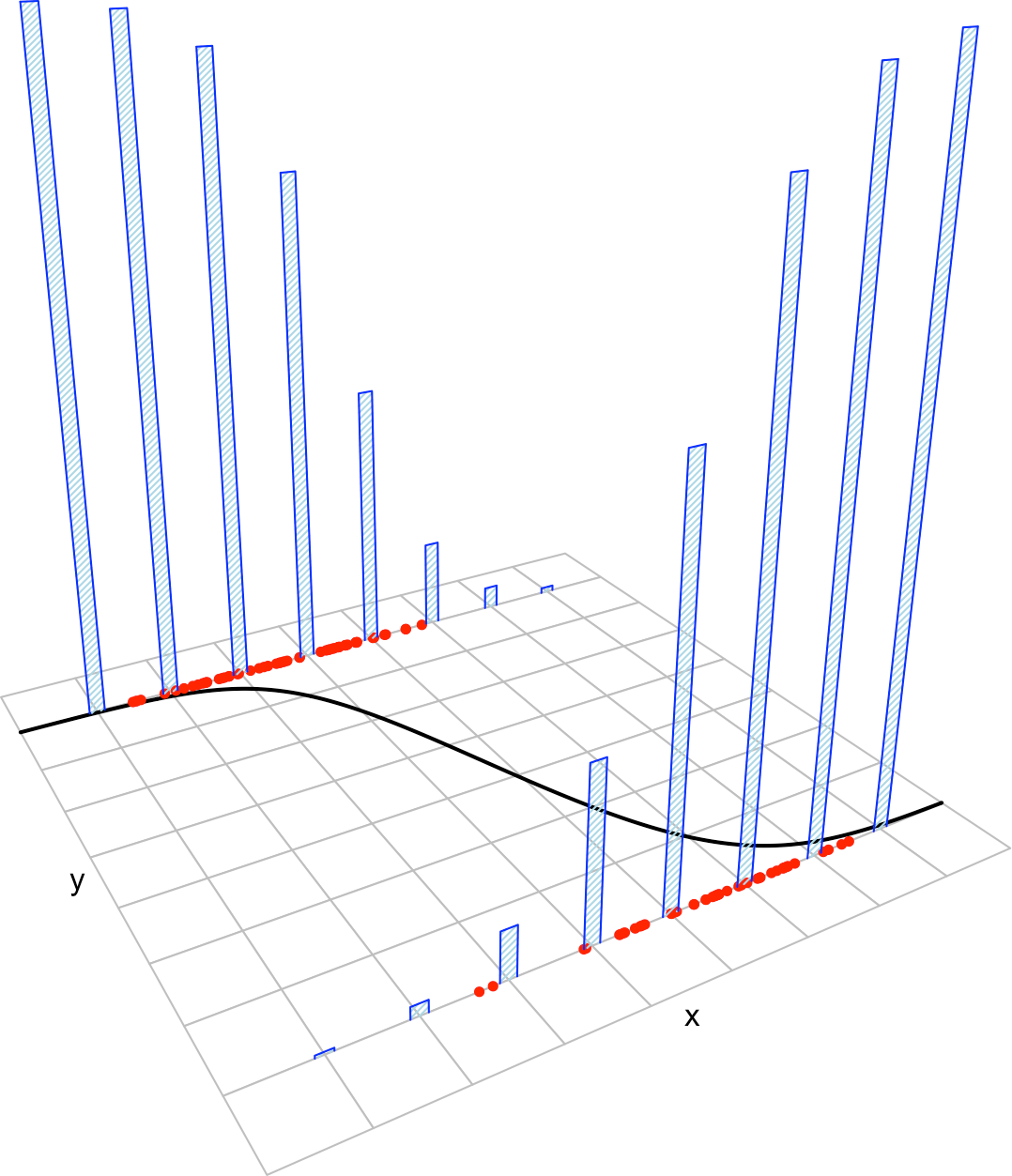
Figure 4.7: The key concepts of the logistic model.
The assumptions of the logistic model are the following:
- Linearity in the logit30: \mathrm{logit}(p(\mathbf{x}))=\log\frac{ p(\mathbf{x})}{1-p(\mathbf{x})}=\beta_0+\beta_1x_1+\cdots+\beta_kx_k.
- Binariness: Y_1,\ldots,Y_n are binary variables.
- Independence: Y_1,\ldots,Y_n are independent.
A good one-line summary of the logistic model is the following (independence is assumed) \begin{align} Y|(X_1=x_1,\ldots,X_k=x_k)&\sim\mathrm{Ber}\left(\mathrm{logistic}(\beta_0+\beta_1x_1+\cdots+\beta_kx_k)\right)\nonumber\\ &=\mathrm{Ber}\left(\frac{1}{1+e^{-(\beta_0+\beta_1x_1+\cdots+\beta_kx_k)}}\right).\tag{4.9} \end{align}
There are three important points of the linear model assumptions missing in the ones for the logistic model:
- Why is homoscedasticity not required? As seen in the previous section, Bernoulli variables are determined only by the probability of success, in this case p(\mathbf{x}). That determines also the variance, which is variable, so there is heteroskedasticity. In the linear model, we have to control \sigma^2 explicitly due to the higher flexibility of the normal.
- Where are the errors? The errors played a fundamental role in the linear model assumptions, but are not employed in logistic regression. The errors are not fundamental for building the linear model but just a helpful concept related to least squares. The linear model can be constructed without errors as (3.5), which has a logistic analogous in (4.9).
- Why is normality not present? A normal distribution is not adequate to replace the Bernoulli distribution in (4.9) since the response Y has to be binary and the Normal or other continuous distribution would put yield illegal values for Y.
Recall that:
- Nothing is said about the distribution of X_1,\ldots,X_k. They could be deterministic or random. They could be discrete or continuous.
- X_1,\ldots,X_k are not required to be independent between them.
An equivalent way of stating this assumption is p(\mathbf{x})=\mathrm{logistic}(\beta_0+\beta_1x_1+\cdots+\beta_kx_k).↩︎