Chapter 3 Multi-Way Contingency Tables
Multi-way contingency tables are very common in practice, derived by the presence of more than two cross-classification variables.
3.1 Description
3.1.1 Three-way Tables
Consider an I×J×K contingency table (nijk) for i=1,...,I, j=1,...,J and k=1,...,K, with classification variables X (the rows), Y (the columns) and Z (the layers) respectively.
A schematic of a generic X×Y×Z contingency table of counts is shown in Figure 3.1.
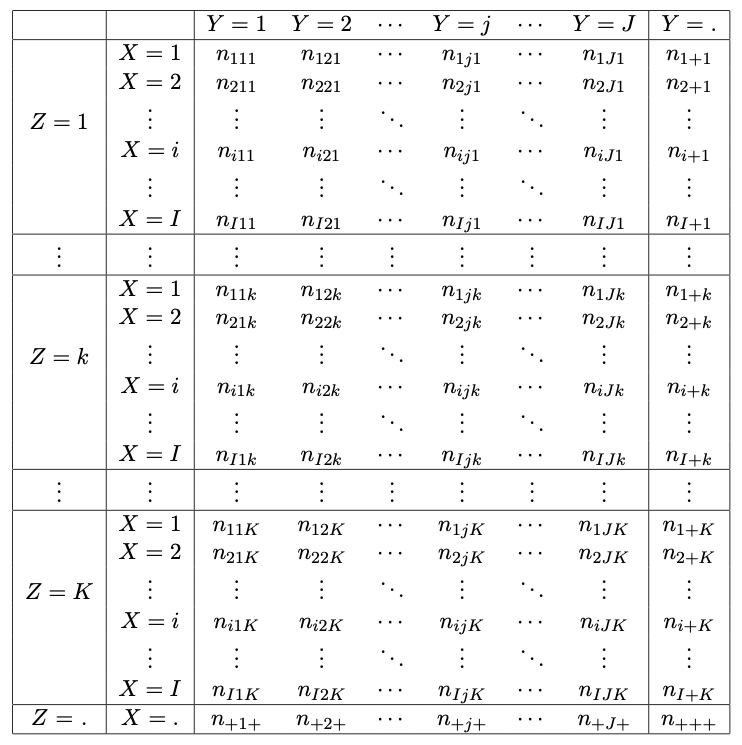
Figure 3.1: Generic I x J x K contingency table of counts.
We can define the joint probability distribution of (X,Y,Z) as πijk=P(X=i,Y=j,Z=k)
Proportions, observed and random counts are defined similarly to the I×J contingency table cases….
3.1.1.1 Example
The table in Figure 3.2 shows an example of a 3-way contingency table. This hypothetical data cross-classifies the response (Y) to a treatment drug (X) at one of two different clinics (Z).
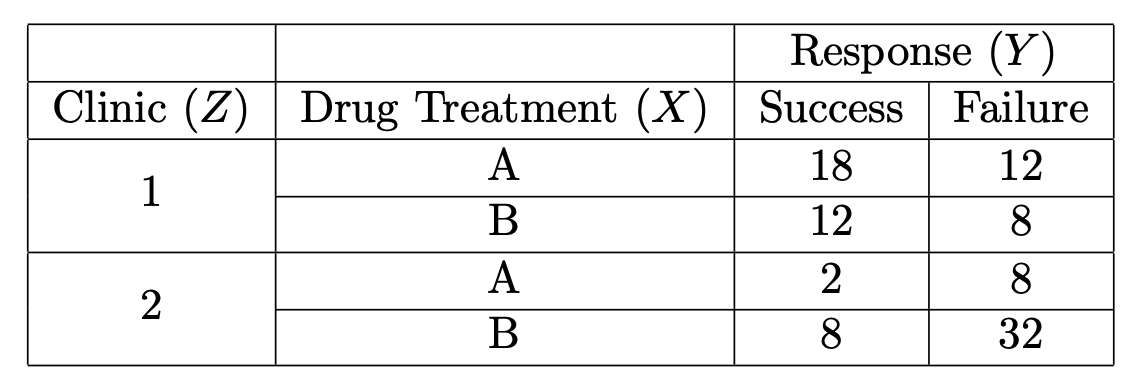
Figure 3.2: Table cross-classifying hypothetical treatment drug, response and clinic.
3.1.1.2 Partial/Conditional Tables
Partial, or conditional, tables involve fixing the category of one of the variables.
We denote the fixed variable in parentheses.
For example, the set of XY-partial tables consist of the K corresponding two-way layers, denoted as (nij(k)) for k=1,...,K.
XZ and YZ- partial tables are denoted as (ni(j)k) and (n(i)jk) respectively.
Partial/conditional probabilities: πij(k)=πij|k=P(X=i,Y=j|Z=k)=πijkπ++kk=1,...,K
Partial/conditional proportions: pij(k)=pij|k=nijkn++kk=1,...,K
3.1.1.3 Marginal Tables
Marginal tables involve summing over all possible categories of a particular variable.
We denote such summation using a + (as before).
For example, the XY- marginal table is (nij+)=(∑knijk).
XZ and YZ- marginal tables are denoted as (ni+k) and (n+jk) respectively.
Marginal probabilities: πij=P(X=i,Y=j)=πij+=K∑k=1πijk
Marginal proportions: pij=pij+=K∑k=1pijk
3.1.2 Generic Multiway Tables
A multiway I1×I2×...×Iq contingency table for variables X1,X2,...,Xq will analogously be denoted as (ni1i2...iq), il=1,...,Il, l=1,...,q.
The definition of partial and marginal tables also follow analogously.
For example, (ni1+(i3)i4(i5)) denotes the two-way partial marginal table obtained by summing over all levels/categories i2 of X2 for a fixed level/category of (or conditioning on) variables X3=i3 and X5=i5.
3.2 Odds Ratios
Conditional and marginal odds ratios can be defined for any two-way conditional or marginal probabilities table of a multi-way I1×I2×...×Iq table with Il≥2, l=1,...,q.
In this case, the conditional and marginal odds ratios are defined as odds ratios for two-way tables of size I×J.
Thus, as defined for general two-way tables in Sections 2.5 and 2.6.2, there will be a (not unique) minimal set of odds ratios of nominal, local, cumulative, or global type.
For example, for an I×J×K table, the XY local odds ratios conditional on Z are defined by rL,XYij(k)=πijkπi+1,j+1,kπi+1,j,kπi,j+1,ki=1,...,I−1j=1,...,J−1k=1,...,K and the XY-marginal local odds ratios are defined by rL,XYij=πij+πi+1,j+1,+πi+1,j,+πi,j+1,+i=1,...,I−1j=1,...,J−1
The conditional and marginal odds ratios of other types, like nominal, cumulative and global, are defined analogously.
3.3 Types of Independence
Let (nijk) be an I×J×K contingency table of observed frequencies with row, column and layer classification variables X, Y and Z respectively.
We consider various types of independence that could exist among these three variables.
3.3.1 Mutual Independence
X, Y and Z are mutually independent if and only if πijk=πi++π+j+π++ki=1,...,Ij=1,...,Jk=1,...,K
Such mutual independence can be symbolised as [X,Y,Z].
3.3.1.1 Example
Following the example of Section 3.1.1.1, mutual independence would mean that clinic, drug and response were independent of each other.
In other words, knowledge of the values of one variable doesn’t affect the probabilities of the levels of the others.
3.3.2 Joint Independence
If Y is jointly independent from X and Z (without these two being necessarily independent), then πijk=π+j+πi+ki=1,...,Ij=1,...,Jk=1,...,K
Such joint independence can be symbolised as [Y,XZ].
By symmetry, there are two more hypotheses of this type, which can be expressed in a symmetric way to Equation (3.2) for X or Z being jointly independent from the remaining two variables. These could be symbolised as [X,YZ] and [Z,XY] respectively.
3.3.3 Marginal Independence
X and Y are marginally independent (ignoring Z) if and only if πij+=πi++π+j+i=1,...,Ij=1,...,Jk=1,...,K
Here, we actually ignore Z.
Such marginal independence is symbolised [X,Y].
3.3.3.1 Example
- If Y and Z are marginally independent38 (that is [Y,Z]), then this would imply that response to treatment is not associated with the clinic attended if we ignore which drug was received.
3.3.4 Conditional Independence
Under a multinomial sampling scheme, the joint probabilities of the three-way table cells πijk can be expressed in terms of conditional probabilities as πijk=P(X=i,Y=j,Z=k)=P(Y=j|X=i,Z=k)P(X=i,Z=k)=πj|ikπi+k
X and Y are conditionally independent given Z if πij|k=πi|kπj|kk=1,...,K
We can consequently show that πj|ik=πj|k and therefore that πijk=πj|kπi+k=P(Y=j|Z=k)P(X=i,Z=k)=P(X=i,Z=k)P(Y=j,Z=k)P(Z=k)=πi+kπ+jkπ++ki=1,...,Ij=1,...,Jk=1,...,K
Note that we here assumed that Y was the response variable. The conditioning approach with X=i as response variable would also lead to Equation (3.4), which is symmetric in terms of X and Y.
This conditional independence of X and Y given Z can be symbolised as [XZ,YZ].
The hypotheses of conditional independence [XY,YZ] and [XY,XZ] are formed analogously to Equation (3.4).
3.3.4.1 Example
If Y and Z are conditionally independent given X (that is, [XY,XZ]), this implies that response to treatment is independent of clinic attended given knowledge of which drug was received.
3.3.4.2 Odds Ratios
Under conditional independence of X and Y given Z ([XZ,YZ]), the XZ odds ratios conditional on Y are equal to the XZ marginal odds ratios, that is39 rXZi(j)k=rXZiki=1,...,I−1j=1,...,Jk=1,...,K−1 In other words, the marginal and conditional XZ associations coincide.
By symmetry, we also have that rYZ(i)jk=rYZjki=1,...,Ij=1,...,J−1k=1,...,K−1 that is, the marginal and conditional YZ associations coincide.
However, the XY marginal and conditional associations do not coincide, that is: rXYij(k)≠rXYij in general.
Such arguments for [XY,YZ] and [XY,XZ] are analogous.
3.3.5 Conditional and Marginal Independence
Important: Conditional independence does not imply marginal independence, and marginal independence does not imply conditional independence.
3.3.5.1 Example
3.3.5.1.1 Marginal but not Conditional Independence
Suppose response Y and clinic Z are marginally independent (ignoring treatment drug X). However, there may be a conditional association between response to treatment Y and clinic attended Z on the drug received X.
Example potential explanation40: some clinics may be better prepared to care for subjects on some treatment drugs than others, but without knowledge of the treatment drug received, neither clinic is more associated with a successful response.
3.3.5.1.2 Conditional but not Marginal Independence
Suppose Y and Z are conditionally independent given X (that is, [XY,XZ]), then this implies that response to treatment is independent of clinic attended given knowledge of which drug was received. However, there may be a marginal association between response to treatment Y and clinic attended Z if we ignore which treatment drug X was received.
Example potential explanation: Given knowledge of the treatment drug, it does not matter which clinic the subject attends. However, without knowledge of the treatment drug, one clinic may be more associated with a successful response (perhaps because their stock of the more successful drug is greater…).
3.3.6 Homogeneous Associations
Homogeneous associations (also known as no three-factor interactions) mean that the conditional relationship between any pair of variables given the third one is the same at each level of the third variable; but not necessarily independent.
This relation implies that if we know all two-way tables between the three variables, we have sufficient information to compute (πijk).
However, there are no separable closed-form estimates for the expected joint probabilities (ˆπijk), hence maximum likelihood estimates must be computed by an iterative procedure such as Iterative Proportional Fitting or Newton-Raphson.
Such homogeneous associations are symbolised [XY,XZ,YZ].
3.3.6.1 Odds Ratios
Homogeneous associations can be thought of in terms of conditional odds ratios as follows:
the XY partial odds ratios at each level of Z are identical: rXYij(k)=rXY,⋆ij
the XZ partial odds ratios at each level of Y are identical: rXZi(j)k=rXZ,⋆ik
the YZ partial odds ratios at each level of X are identical: rYZ(i)jk=rYZ,⋆jk
Note that rXY,⋆ij,rXZ,⋆ik,rYZ,⋆jk are not necessarily the same as the corresponding marginal odds ratios rXYij,rXZik,rYZjk.
3.3.6.2 Example
The treatment response and treatment drug have the same association for each clinic.
More precisely, we have rXYA,S,(k)=rXY,⋆A,S⟺πA,S,(k)πA,F,(k)=rXY,⋆A,SπB,S,(k)πB,F,(k)k=1,2 which means that each drug has a different odds of success depending on the clinic, however, the odds of treatment success of drug A are a fixed constant rXY,⋆A,S greater than the odds of treatment success of drug B, regardless of the clinic.
3.3.7 Tests for Independence
Marginal independence (Equation (3.3)) can be tested using the test for independence presented in Section 2.4.3.1 applied on the corresponding two-way marginal table.
Hypotheses of the independence statements defined by Equations (3.1), (3.2) and (3.4) could be tested analogously using the relevant marginal counts.
We do not consider these tests, but defer to log-linear models (soon!).
A specific test of independence of XY at each level of Z for 2×2×K tables is presented in Section 3.3.10.
3.3.8 Summary of Relationships
We present a summary of which independence relationships can be implied from which others, and which can’t, in Figure 3.3.
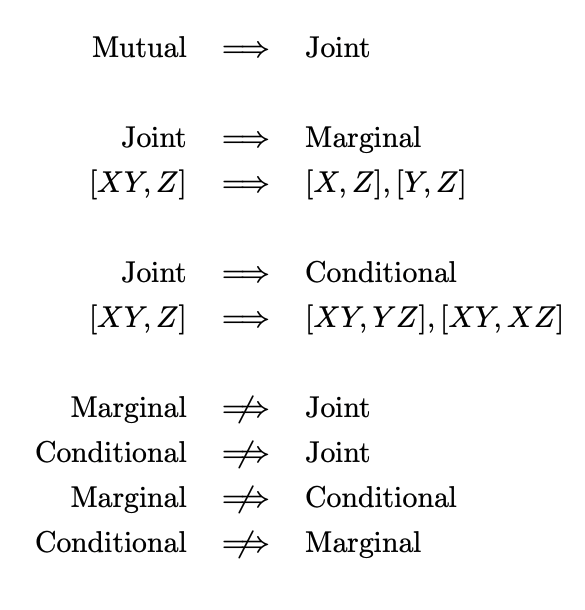
Figure 3.3: Summary of relationships between independencies.
3.3.9 Multi-way Tables
- Analogous definitions of the various types of independence exist for general multi-way tables.
3.3.10 Mantel-Haenszel Test for 2×2×K Tables
We will discuss the particular case of X and Y being binary variables that are cross-classified across the K layers of a variable Z, forming K 2×2 partial tables nij(k),k=1,...,K.
The Mantel-Haenszel Test is for testing the conditional independence of X and Y given Z for these 2×2×K tables, that is, it considers the hypotheses H0:X,Yare independent conditional on the level of Z.H1:X,Yare not independent conditional on the level of Z. or in other words41 H0:r12(k)=1,for allk=1,...,KH1:r12(k)≠1,for somek=1,...,K
The Mantel-Haenszel Test conditions on the row and column marginals of each of the K partial tables.
Under H0, every partial table has that n11k follows a hypergeometric distribution42 Hg(N=n++k,M=n1,+,k,q=n+,1,k)43, and thus has mean and variance ˆE11k=n1+kn+1kn++kˆσ211k=n1+kn2+kn+1kn+2kn2++k(n++k−1)
∑kn11k therefore has mean ∑kˆE11k and variance ∑kˆσ211k, since the values of n11k are independent of each other (having conditioned on Z=k).
The Mantel–Haenszel test statistic is defined as44 TMH=[∑k(n11k−ˆE11k)]2∑kˆσ211k
TMH is asymptotically χ21 under H0.
If TMH(obs) is the observed value of the test statistic for a particular case, then the p-value is P(χ21>TMH(obs)).
When the XY association is similar across the partial tables, then the test is more powerful.
It loses in power when the underlying associations vary across the layers, especially when they are of different direction, since the differences n11k−ˆE11k will then cancel out in the sum of the statistic given by Equation (3.6).
ignoring X↩︎
Note that we don’t superscript L or G here, as the result holds for both. Q3-2 involves showing that Equation (3.5) holds for local odds ratios.↩︎
Note that this is precisely what this is - a potential explanation - it would be incorrect to conclude that this is definitely the reason for the hypothesised independence scenarios. We all know (I hope…) that (or in this case, ) .↩︎
Note that we revert back to the r12 notation here since each of the K layers is a 2×2 table.↩︎
Why hypergeometric? Well, for any 2×2 table we have an assumed total of N=n++k items. We condition on row and column margins, so we assume knowledge of ni,+,k and n+,j,k. In that population, we know that M=n1,+,k of these items are such that i=1. If the two variables X and Y are conditionally independent given Z, then we could view N1,1,k to be the result of picking q=n+,1,k items (those going into column 1) randomly from N=n++k, and calculating how many of those are from row 1 (given that we know that there are M=n1,+,k items out of the N that will go into row 1 in total). Therefore N1,1,k∼Hg(N=n++k,M=n1,+,k,q=n+,1,k)↩︎
Note that the square is outside of the summation.↩︎