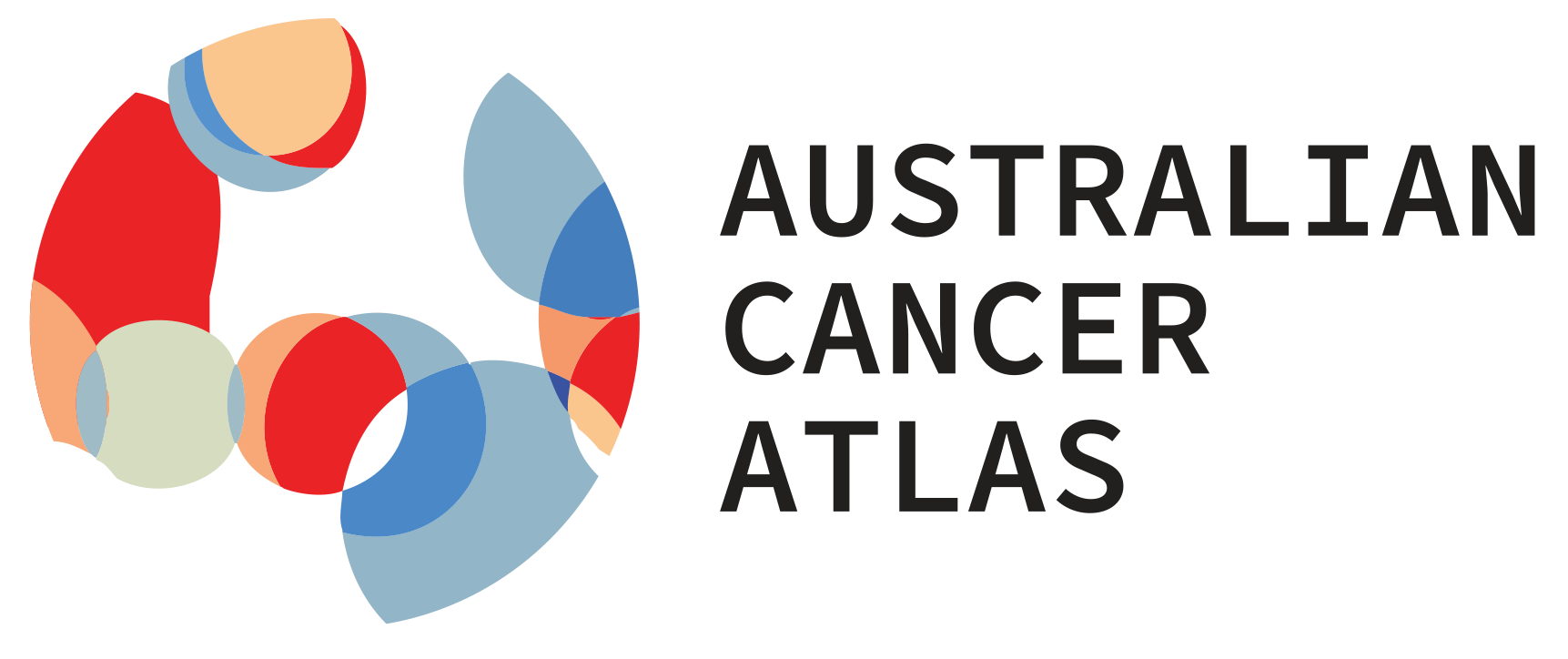
15 Limitations
Some of the limitations regarding Australian Cancer Atlas are given below:
Aggregation of data: Although more detailed address information may be collected by the data custodians (such as state and territory cancer registries, Australian Institute of Health and Welfare, Medicare Benefits Schedule), to preserve confidentiality and privacy, the most detailed geographical information able to be provided for the Australian Cancer Atlas was the Australian Bureau of Statistics SA2 classification. (Australian Bureau of Statistics, 2016a) However, even with this aggregation, there were often very small numbers of people diagnosed with cancer or for other indicators in many specific SA2s each year. This meant that we needed to aggregate several years of data to calculate estimates with sufficient reliability.
Choice of geographical area: The aggregation of the data into geographical areas and over time does have some limitations because it covers over any differences within each area and within the combined time period. Therefore, it is possible that if we chose different geographical areas, or a different time period, then the geographical patterns shown in the current Australian Cancer Atlas would be different. This is what is known as the modifiable areal unit problem (MAUP). (Wong, 2009) While we do not discount the importance of the modifiable areal unit problem in spatial analyses, the estimates supplied are at the smallest level of geography currently possible given the availability of suitable population data, maintaining sufficient area-specific numbers of cases for analyses, and the need to maintain the confidentiality of data. This meant we could not check how estimates would differ when using smaller areas.
Accuracy of addresses: For cancer diagnosis and survival, the aggregation of cancer address information into SA2 areas was made by the respective state- and territory-based cancer registries. No details on the specific quality of this aggregation were available, with different methods being used. It remains possible that some misclassification of SA2s has occurred in these cancer data, for example if patients provided a PO Box rather than street address information, or the location of a hospital was provided rather than a residential address. These limitations are also relevant for cancer screening or testing and hospital treatment data.
For bowel cancer and cervical screening, SA2 geographical area data were directly assigned based on longitude and latitude information at time of screening. For people without reliable address of residence, the postcodes of usual residence were used and assigned to an SA2. As postcodes can cross boundaries of SA2s, geographical mapping based on postcode correspondences are likely to be less accurate.
For breast cancer screening and PSA testing, data were only available by postcode of usual residence at time of screening or testing. Individuals were reallocated from postcode to SA2 using a correspondence matrix that is based on the whole resident population, however, the screening and testing data only include a proportion of the population. For example, only females are screened for breast cancer and the eligible age group is restricted. Postcodes are designed for delivering mail and not for statistical analyses, so they may group together areas with very different sex or age distributions. The methods used account for some of the uncertainty in reallocating from postcode to SA2. However, the reallocation method essentially applies some spatially smoothing to the data before the models were fitted.
The hospital treatment data was converted from 2011 to 2016 SA2s using population-weighted geographical correspondences, that were based on persons and for all age groups. Hence, they may not be representative of specific age groups or population sub-groups.
Areas with extreme estimates (cancer diagnosis and survival): When examining the initial spatial estimates for cancer diagnosis, we identified some problematic areas, where a specific area would have a markedly high estimate, while its neighbouring areas had correspondingly low estimates. When this occurred consistently across multiple sex/cancer combinations, and the ratio of differences was substantial, some modification of the area assignment was performed after consulting with the relevant data custodians. Cases were redistributed on a population-basis, considering broad age categories, sex, and cancer type. For Atlas 2.0, the only SA2 which was impacted was Charles (NT), for which cases were redistributed to its four neighbouring SA2s (East Side, Flynn (NT), Larapinta, Mount Johns). Full details of these modifications are available on request.
Exposure differences: Estimates are for the residential address at time of diagnosis. It is unknown how long the patient may have been living there, or whether they continue to live there throughout their cancer treatment. People often commute or travel each day, and where someone lives may not be where they were exposed. Most cancers have a long lag time between exposure to a carcinogen and developing cancer, so maps in the Atlas cannot be used to identify areas with high risk factor exposure.
Clinical characteristics: Information on stage at diagnosis was only available for year 2011 and for a limited number of cancers.
Bowel cancer & cervical screening data: Direct comparisons between the states and territories of Australia are not advised, due to the substantial differences that exist between the jurisdictions, including population, area, geographical structure, policies, and other factors. In addition, changes in screening patterns over time cannot be estimated for these two indicators as data are currently only available for a single five-year reporting period for cervical cancer and the eligibility criteria for bowel cancer screening has changed. (Dasgupta et al., 2023b)
Breast & bowel cancer screening data: Data on breast or bowel cancer screening outside the national screening programs were not available.
Prostate cancer hospital treatment data: Hospital separations data included interventional treatments only and not other types of treatment such as active surveillance, watchful waiting, external beam radiotherapy, androgen deprivation therapy, chemotherapy, or combination therapy. (Cameron et al., 2023) Notably there were no data on external beam radiation therapy, the second most common form of active treatment for prostate cancer. Overall, these gaps in the data may explain why interventional treatment rates are apparently low in some areas, particularly for radical prostatectomy.
Self-reported data: Most risk factor estimates were based on self-reported data and as such are subject to biases.
Risk factor data: Due to the lag time between exposure (to a risk factor) and a cancer diagnosis, which can be more than 40 years, the estimates here cannot be used to establish area-level associations between risk factors and cancer incidence. Moreover, as these estimates are derived from cross-sectional data at a particular time point, they cannot be used to infer lifetime risky behaviour or investigate possible causal relationships with cancer incidence or survival. (Hogg et al., 2023)
The cancer risk factor estimates can be used to understand current prevalence, and to highlight areas with the greatest potential for local interventions.
Spatiotemporal patterns: Because of internal migration and changes in the geographics of socioeconomic disadvantage and remoteness; changes in geographical patterns of cancer diagnosis or survival over time and the impact of remoteness or socioeconomic status on these patterns should be interpreted cautiously.