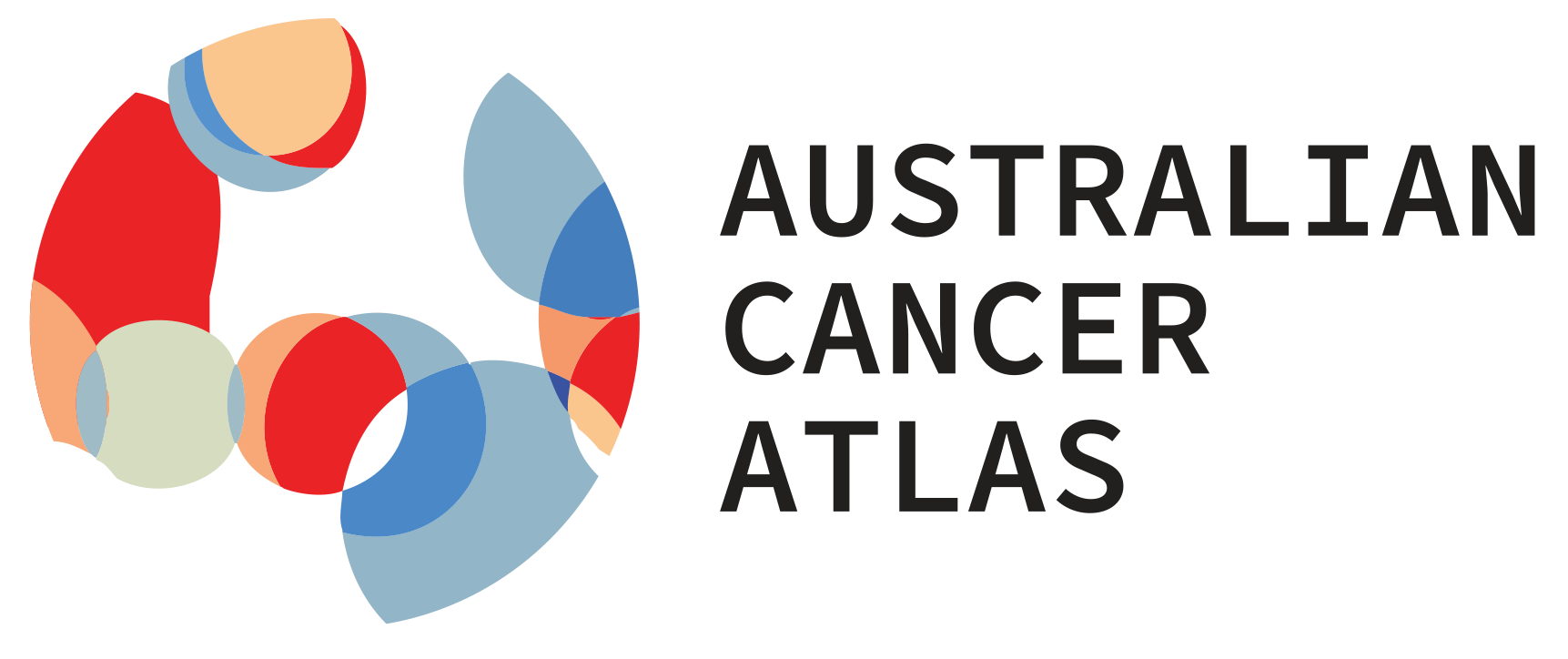
8 Cancer diagnosis
8.1 What is cancer diagnosis?
‘Cancer diagnosis’ refers to the number of new cancers diagnosed among people living in a defined geographical area within a given time period. Reporting just the geographical variation in number of cancer diagnoses can be misleading, because some areas may have many residents while others have few. For this reason, cancer diagnoses are typically reported using rates. Cancer diagnosis rates are the number of new cancers diagnosed in an area, divided by the total number of people in that area.
Cancer is more common among older people. If one geographical area has an older population on average than another, comparing rates between these areas would not be a fair comparison. Age-standardisation allows for the different age structures of the populations to be considered, so that any differences between areas are differences that cannot be explained by the different ages of the populations.
To compare the cancer diagnosis rate between areas, the cancer diagnosis rate for a specific area was compared to the Australian average. This measure is known as the standardised incidence ratio or SIR for short (Incidence is another name for diagnosis). The SIR is a number that indicates whether the cancer diagnosis burden in one area is higher or lower than expected, given the population size and age structure of that area.
In the Australian Cancer Atlas, the SIRs were calculated using Bayesian models with smoothing (Cramb et al., 2016) as described in Section 7. An SIR greater than one (shown in orange/red) means that, overall, people living in that area had a greater risk of being diagnosed with cancer than the Australian average. Conversely, values below one (shown in blue) mean that, overall, people living in the area had a lower risk of being diagnosed with cancer than the Australian average. An SIR value around one is shown in yellow, meaning it is very similar to the Australian average.
It is important to note that the SIR for a specific geographical area reflects the overall result for that area. All the results presented in the Australian Cancer Atlas are about the average cancer burden for all people living within an area. Since individual people are very different, with different characteristics, lifestyles and habits, this overall result does not apply to every person living in that area.
8.2 How are cancer types defined?
Cancer is a disease caused by rapid growth and uncontrollable division of abnormal cells. Visit the Cancer Council Australia website for more information about cancer. (Cancer Council Australia: What-is-cancer)
Majority of cancer types in the Australian Cancer Atlas were defined using the International Statistical Classification of Diseases and Related Health Codes, tenth revision (ICD-10). (ICD-10) However some cancer types (such as rare cancers, rare blood cancers, soft tissue sarcomas, classic myeloproliferative neoplasms and neuro endocrine tumours) were defined using a combination of the International Classification of Diseases for Oncology, third edition (ICD-O-3) topography (site) and morphology (histology) codes. (ICD-O-3)
Below are the specific types of cancers included in the Atlas 2.0 (Table 8.1). Further information about these cancers can be found at the Cancer Council Australia website. (Cancer Council Australia: Types of Cancer)
8.2.1 Cancer type definitions
Table 8.1: Cancer types included in Australian Cancer Atlas 2.0.
Cancer description | ICD-10 code(s) |
---|---|
All malignant cancers combined | C00–C97, D45, D46, D47.1, D47.3–D47.5 |
Bladder cancer | C67 |
Bowel cancer (Colorectal cancer) | C18-C20 |
Brain cancer | C71 |
Breast cancer (Female only) | C50 |
Breast cancer in situ (Female only) | D05 |
Cervical cancer | C53 |
Classic myeloproliferative neoplasms | ICD-O-3 morphology codes: 9950, 9960-9962 (Cameron et al., 2022) |
Head and neck cancers | C00-C14, C30-C32 |
Kidney cancer | C64 |
Leukaemia | C91-C95 |
Liver cancer | C22 |
Lung cancer | C33- C34 |
Melanoma in situ | D03 |
Melanoma of the skin | C43 |
Mesothelioma | C45 |
Myeloma | C90 |
Neuroendocrine tumours | ICD-O-3 morphology codes: 8013,8040-8045, 8150-8156, 8158-8240-8249, 8345-8347, 8680-8683, 8690-8693, 8700, 9091 for all topography codes or 8510 for topography C73. (Australian Institute of Health and Welfare, 2023d) |
Non-Hodgkin Lymphoma | C82-C86 |
Oesophageal cancer | C15 |
Oral cancers | C0-C10 |
Ovarian cancer | C56 |
Pancreatic cancer | C25 |
Prostate cancer | C61 |
Rare blood cancers | See section (8.2.2) |
Rare cancers | See section (8.2.2) |
Soft tissue sarcomas | Codes in Tables 5 and 6. (Australian Institute of Health and Welfare, 2023e) |
Stomach cancer | C16 |
Testicular cancer | C62 |
Thyroid cancer | C73 |
Uterine cancer | C54-C55 |
Vulva | C51 |
8.2.2 Rare cancers
The term ‘rare cancer’ includes hundreds of different cancer types, where each individual cancer type impacts only a small number of people, the reason for calling them ‘rare’. Collectively rare cancers add up to an important health burden, about quarter of cancer diagnoses and deaths. (Dasgupta et al., 2023a)
For the Australian cancer atlas, rare cancers were defined based on a system first developed by the Surveillance of Rare Cancers in Europe. This system defines rare cancers based on incidence, that is the number of newly diagnosed cancers per year. According to this definition, a rare cancer is a cancer with a crude incidence rate of less than 6 per 100,000 persons per year. (Rare Cancers Europe) The rate is given by the number of cases within a cancer type, sex and age group combination divided by the corresponding population and then adding all the rates together.
To define rare cancers, each cancer case was first classified according to a list called the RARECANCER list. (Botta et al., 2020) This list divides cancer types based on a combination of their ICD O-3 site and morphology (the type of cell that has become cancerous, based on pathology) codes into two tiers. The first Tier (Tier-1) includes major cancer types in a clinical sense (for example “soft tissue sarcoma”) while the second Tier (Tier-2) categorises Tier-1 types into clinically distinct tumours (for example “soft tissue sarcoma of limb”). Rare cancers were then defined as Tier-2 cancers with an incidence rate of less than 6 per 100,000 persons per year. These rates were calculated based on the total population (males and females combined) except for sex- specific cancer types.
All Tier-2 cancers with the above incidence cut-off were collectively categorised as rare cancers.
Rare cancers can also be collectively grouped into families (such as blood cancers), (Casali and Trama, 2020) based on their ICD-O-3 site and morphology codes. The Atlas reports estimates for one such family, rare blood cancers which includes all rare cancers of the blood. (DeSantis et al., 2017, Gatta et al., 2017)
8.3 Data sources
8.3.1 Cancer registry
Details about cancers diagnosed in Australia and their survival outcomes were obtained from the Australian Cancer Database. (Australian Cancer Database) This database includes all primary invasive cancer cases (excluding basal and squamous cell carcinoma of the skin) notified to each of the cancer registries across Australia. These notifications come from hospitals and pathology laboratories in all states and territories, as well as general practitioners, cancer screening registers and nursing homes in some, but not all, states, and territories. It is a legal requirement for cancer to be notified to the appropriate registry across Australia, hence cancer is one of the few diseases with almost complete national coverage.
The Cancer Data & Monitoring Unit at Australian Institute of Health and Welfare combines the information collected by each of the eight state and territory cancer registries in Australia to create the Australian Cancer Database.
The Australian Cancer Atlas reports estimates for Australians aged 15 years and over who were diagnosed with cancer from 1996 to 2019.
8.3.2 Population
Population data by SA2, five-year age group and year was obtained from the Australian Bureau of Statistics. (Australian Bureau of Statistics, 2022a)
8.3.3 Geographical areas
The data set included details on the usual area of residence at time of cancer diagnosis, based on their 2016 SA2. (Australian Bureau of Statistics, 2016a) For a small subset of records, the 2016 SA2 was unknown, but the 2011 SA2 was known. The 2011 SA2 information for these records were converted to 2016 SA2 using population-weighted geographical correspondence files. (Australian Bureau of Statistics Geospatial Solutions, 2023) Records with no SA2 information were excluded.
Cancer registries use address information of usual residence at the time of diagnosis to determine the SA2 information. The method by which each cancer registry allocates this information varies from using the longitude/latitude coordinates of the street address to geographical correspondence files. Additional manual checks and verification are conducted on an ongoing basis by registry staff to increase the completeness and accuracy of the processes.
Of 2,288 SA2 (2016) areas in Australia that were potentially eligible for analysis after excluding 18 SA2s with no spatial information and 4 remote islands (see Section 4.1), we excluded 28 SA2s that had no population and a further 22 SA2s that had fewer than five residents on average per year during 2010-2019. This means the Australian Cancer Atlas provides modelled estimates for cancer diagnosis for 2,238 SA2s across Australia.
8.3.4 Clinical characteristics
The Australian Cancer Atlas reports geographical patterns by cancer stage at diagnosis for breast, prostate and melanoma, and melanoma thickness at diagnosis across Australia. It also reports geographical patterns in diagnosis for in situ melanomas and female breast cancers.
8.3.4.1 Cancer staging
The stage at cancer diagnosis is indicative of how far a cancer has spread when first diagnosed. It is categorised into four stages of increasing severity with stage 1 being localised disease and stage 4 being more widely spread or metastatic disease. (National cancer stage at diagnosis data) A higher stage is indicative of worse survival. In Australia, stage at diagnosis data is only available for the top five cancers: melanoma, female breast, colorectal, lung and prostate cancer that were diagnosed in 2011. (National cancer stage at diagnosis data) Stage data helps to understand survival patterns and direct further research and control measures to reduce diagnosis of cancers at advanced stages.
The stage at diagnosis data was collected as part of Cancer Australia’s StaR project (StaR (Stage, Treatment and Recurrence) project) in which five cancers (colorectal, lung, melanoma, female breast and prostate) diagnosed in 2011 were staged.
Data on the “registry-derived stage at diagnosis information” was obtained for three primary invasive cancer types (ICD-10 melanoma: C43, breast: C50 and prostate C61) from the Australian Institute of Health and Welfare for year of diagnosis, 2011, by small geographical areas (SA2).
Cancer staging was performed by each state and territory cancer registry using national business rules to extract information from pathology records and assign a stage of 1 (early) to 4 (metastatic). However, low numbers (or, for prostate cancer, differing definitions) required aggregation of categories. For melanoma and breast cancer, there were two stage categories: stage 1 was considered “early” and stages 2 to 4 aggregated together were considered “advanced”. For prostate cancer, stages 1-2 were grouped together as “early” and stages 3-4 were aggregated together as “advanced”. A small number of tumours could not be staged, while an additional small number were not considered in scope when the staging was undertaken. These were excluded from the analyses.
8.3.4.2 Melanoma tumour thickness
Australia has the highest incidence rates of melanoma in the world. (Ferlay J et al., 2024) Melanoma is a type of skin cancer that develops in melanocytes due to overexposure to the sun. Melanoma is considered the most severe type of skin cancer compared to the basal cell or squamous cell carcinomas (keratinocyte cancers) since it is most likely to spread to the other parts of the body if not detected early. The thickness of the melanoma determines the severity of the disease. Generally, a higher thickness indicates more severe disease and worse survival. Hence the early detection of melanoma is crucial for better survival. Full body clinical skin examination is associated with lower melanoma related deaths and fewer advanced stage melanomas. (Aitken et al., 2010) There’s evidence that thick melanomas are higher among low socioeconomic groups, potentially due to less frequent skin examinations. (Youl et al., 2011)
The Australian Cancer Atlas reports the geographical patterns by thickness for melanomas diagnosed from 1996 to 2019.
Melanoma tumour thickness details were extracted from the same dataset used for cancer diagnosis. The thickness groups were categorised as thin (≤1mm), and thick (>1mm). (Iannacone et al., 2015)
8.4 Statistical methods
8.4.1 National summary measures
Number of diagnoses
The total number of diagnoses by sex (males, females, persons) aged 15 years and over for the time period of analysis were reported.
Diagnosis rate (age-standardised)
Age-specific rates in 5-year age bands, starting at 15 years (i.e. 15-19, 20-24,…85+) were calculated for each cancer type and sex (males, females, persons) for Australia for the time period of analysis. These age-specific rates equal the number of cases within a cancer type, sex and age group combination divided by the corresponding population.
Age-standardised rates were then calculated by multiplying the age-specific rates by standard population weights, which for the Atlas was the Australian Standard Population, (Australian Standard Population 2001) and then adding all the rates together.
8.4.2 Spatial models
The final selected models for cancer diagnosis were Bayesian spatial models with the Leroux prior. (Leroux et al., 2000) This is one of many models designed for count data which is a specific case of the three-stage hierarchical model for the purpose of disease mapping. (Best et al., 2005) This model was chosen based on a thorough and careful process of testing and evaluation. (Cramb et al., 2018, Cramb et al., 2020) The first stage is the likelihood model for the observed count data, that is, the number of cancer diagnoses, \(Y_{i}\). In area \(i\):
\[Y_{i}\ \sim\ Poisson(E_{i}\lambda_{i})for\ i = 1\ldots\ldots\ldots,\ N\ areas\]
where \(E_{i}\) is the expected number of cancer diagnosis for each SA2 of usual residence and 5-year age group from ages 15+ (15-19, 20-24,…,85+). The expected number of diagnoses were calculated as:
\[E_{i} = \sum_{age\ group = 1}^{15}{\frac{Number\ of\ cancers\ diagnosed\ in\ Australia\ in\ the\ age\ group}{Australian\ population\ in\ the\ age\ group}SA2\ population\ for\ the\ age\ group}\]
where age group=1 represents ages 15-19 and age group=15 represents ages 85+. This used data aggregated over 10 years (2010-2019).
Modelled estimates are effectively age-adjusted since \(E_{i}\) considers the age structure and population size.
The second stage comprises an expression for the SIR (𝜆𝑖), which is the key output used in the Australian Cancer Atlas.
\[log(\lambda_{i}) = \ \beta + S_{i}\]
The parameter \(\beta\) is the intercept, while \(\text{S}_{i}\)represents the spatial random effect for area i. The specification of these spatial random effects determines how the smoothing is performed, and together with the specification of the intercept parameter, forms the third stage of the model in the form of prior distributions.
The prior for the intercept was a weakly informative Gaussian distribution with zero mean.
\[\beta\ \sim\ N(0,100000)\]
The spatial random effects \(\text{S}_{i}\) were modelled by the Leroux prior, (Leroux et al., 2000) which is a Gaussian distribution, and its mean is the weighted average of the neighbouring areas’ random effects (with weight) \(\rho\) and a global mean of 0 (with weight \(1 - \ \rho\)).
\[S_{i}|S_{\backslash i}\ \sim\ N\left( \frac{\rho\Sigma_{h}w_{ih}S_{h}}{\rho\Sigma_{h}w_{ih} + 1 - \rho},\ \frac{\sigma_{S}^{2}}{\rho\Sigma_{h}w_{ih} + 1 - \rho} \right)\]
The spatial weights \(w_{ih}\) represent the spatial proximity between areas \(i\) and \(h\), and collectively they comprise an N×N spatial adjacency matrix W. A binary weighting scheme was used, so that \(w_{ih} = 1\) if areas \(i\) and \(h\) were considered to be neighbours, and otherwise \(w_{ih} = 0\).
To complete the Bayesian model specification, the variance \(\sigma_{S}^{2}\) was also given a weakly informative prior:
\[\sigma_{S}^{2}\ \sim\ InverseGamma(1,\ 0.01)\]
which is an inverse-gamma distribution with parameters shape and scale. The prior for the spatial smoothing parameter \(\rho\) was weakly informative.
\[\rho\ \sim\ Uniform(0,1)\]
If \(\rho=1\), then the Leroux Conditional Autoregressive (CAR) prior (Cramb et al., 2016) reverts to the intrinsic CAR prior (i.e. complete smoothing across neighbours), whereas if \(\rho=0\) then no spatial smoothing occurs, and each area is independent of its neighbours.
The Australian Cancer Atlas reports smoothed SIRs and the modelled counts of diagnosed cases by SA2 from the fitted models, aggregated over 10 years (2010-2019). Estimates shown on maps are the median value of the posterior distribution for each SA2.
8.4.2.1 Bayesian spatial model for cancer diagnosis
8.4.2.1.1 Input data set
Key variables in this data set are:
Variable | Value |
---|---|
grid | A unique identification number for each small area. The numbers should be consecutive values from 1 to N, where N is the total number of areas |
observed | Observed number of diagnosed cancer cases |
expect | Expected count of diagnosed cancer cases |
The data should be sorted by grid. Expected counts should be greater than zero.
8.4.2.1.2 Adjacency matrix
An adjacency matrix specifies the spatial structure of the small areas, with a unique row and column for each area. For the Australian Cancer Atlas, a first-order adjacency matrix was used, in which two areas were considered to be neighbours if they shared a common geographical boundary. Small areas without any shared boundaries (for example islands) were manually assigned at least one neighbour (usually the closest mainland area).
The column and row number for each area corresponds to the area’s unique identification number (“grid”, as described above). The \((i,{h)}^{th}\) element of the matrix was 1 if areas \(i\) and \(h\) share a border, and 0 if \(i = h\) or if areas \(i\) and \(h\) do not share a border. The matrix must be symmetric, that is the \((i,{h)}^{th}\) element is equal to the \(({h,i)}^{th}\)element.
8.4.2.1.3 R code
## Required libraries
library(CARBayes)
## Setting up input data for spatial model
## data$observed is the observed number of cancer cases in a small area.
## data$expect is the expected count of cancer cases based on the
## age-specific national rates applied to the age-specific population within the same small area.
## data$grid is the identifier for a small area (values 1,.., N, N is the total
## number of small areas) which needs to be the same in the data file and the adjacency matrix file
file.input = data.frame(
obs = data$observed,
expect = data$expect,
grid = data$grid
)
# Model specification using the CARBayes package with the Leroux prior and a Poisson likelihood
# Default priors were used
formula <- obs ~ offset(log(expect))
MCMC <- S.CARleroux(
formula = formula,
data = file.input,
family = "poisson",
W = W, # The adjacency matrix
burnin = 50000,
n.sample = 150000,
thin = 10
)
# Calculate the standardised incidence ratio (SIR) for each MCMC iteration
sir.mcmc <-
MCMC$samples$fitted /
matrix(
rep(file.input$expect, nrow(MCMC$samples$fitted)),
byrow = T, ncol = ncol(MCMC$samples$fitted)
)
# Calculate median, credible interval and exceedance probability (EP) for the SIRs
SIR <-
data.frame(
grid = file.input$grid,
median = apply(sir.mcmc, 2, median),
LB = apply(sir.mcmc, 2, quantile, probs = 0.025),
UB = apply(sir.mcmc, 2, quantile, probs = 0.975),
EP = apply(sir.mcmc, 2, function(x) sum(x > 0) / nrow(sir.mcmc))
)
# Calculate median and credible interval for the modelled count estimates
modelled.counts <-
data.frame(
grid = file.input$grid,
median = apply(MCMC$samples$fitted, 2, median),
LB = apply(MCMC$samples$fitted, 2, quantile, probs = 0.025),
UB = apply(MCMC$samples$fitted, 2, quantile, probs = 0.975)
)
8.4.3 Spatiotemporal models
After an exhaustive investigation of different types of spatiotemporal models, the model of Bernardinelli and colleagues (Bernardinelli et al., 1995) was adapted for the Australian context. In the first stage of the model, the observed number of diagnoses (\(Y_{it}\)) was modelled on the expected counts (\(E_{it}\)) and the standardised incidence ratio (\(\lambda_{it}\)) for each area \(i\) and time \(t\).
\[Y_{it}\sim\ Poisson\left( E_{it}\lambda_{it} \right)\ \ \ \ for\ i = 1,\ldots,\ 2238\ areas\ and\ t = 1,\ldots,T\ time\ points\]
The expected number of diagnoses were calculated using the age distribution of the area at each time and the age-specific rates of cancer diagnoses. These age-specific rates were calculated differently for the different types of estimates, as described below, and separate models were fitted for each.
The standardised incidence ratios (\(\lambda_{it}\)) are the key output visualised in the Atlas maps. These were calculated using the modelled counts (\({\widehat{Y}}_{it}\)) and the expected counts.
\[\lambda_{it} = {\widehat{Y}}_{it}/E_{it}\]
The standardised incidence ratios (SIRs) for the national trend were calculated similarly. For each MCMC iteration \(m\), the national SIR (\(\lambda_{Aust,t,m})\ \) for time \(t\) was calculated as below.
\[\lambda_{Aust,t,m} = \frac{\Sigma_{i}{\widehat{Y}}_{it,m}}{\Sigma_{i}E_{it}}\]
The second stage of the model describes how the standardised incidence ratios vary over time and space using a national temporal trend (\(f(t)\)), spatial effects (\(R_{i}\)) and area-specific deviations from the national trend (\(f_{i}(t)\)).
\[\log\left( \lambda_{it} \right) = \ f(t) + f_{i}(t) + R_{i}\]
Spatial effects
The spatial effects were modelled by a BYM2 prior, (Riebler et al., 2016) which is a weighted average between a spatially structured effect (\(\phi_{i}\)) and a Gaussian effect with zero mean (\(\theta_{i}\)):
\[\theta_{i}\ \sim\ N(0,\ 1)\]
\[\phi_{i}|\phi_{\backslash i}\ \sim\ N\left( \frac{\sum_{h}^{}{w_{ih}\phi_{h}}}{\sum_{h}^{}w_{ih}},\ \frac{1}{\sum_{h}^{}w_{ih}} \right)\]
\[R_{i} = \frac{1}{\sqrt{\tau_{R}}}\left( \theta_{i}\sqrt{1 - \rho} + \ \phi_{i}\sqrt{\rho/\iota} \right)\]
where \(\iota\) is a scaling factor calculated from the adjacency matrix, W, which is comprised of the spatial weights \(w_{ih}\). These weights represent the spatial proximity between areas \(i\) and \(h\). A binary weighting scheme was used, so that \(w_{ih} = 1\) if areas \(i\) and \(h\) were considered neighbours and \(w_{ih} = 0\) otherwise.
Temporal effects
The national trend and the area deviations from the national trend were modelled using splines. Splines are a set of simple lines or curves that are added together, resulting in a versatile range of possible curves. You can explore splines in the app below.
The national temporal trend was modelled by an intercept (\(\beta_{0}\)), a linear component (\(\beta_{1}\)) and a set of spline coefficients (\(b_{\kappa}\) for each knot \(\kappa = 1,\ldots,Κ\)), as follows:
\[f(t) = \ \beta_{0} + \beta_{1}X_{t} + \sum_{\kappa = 1}^{Κ}{b_{\kappa}Z_{\kappa,t}}\]
where \(X_{t}\) is the time point (normalised) and \(Z_{\kappa,\ t}\) is the spline for the \(\kappa\)th knot at time point \(t\).
The deviation for area from the national trend was defined by the splines alone.
\[f_{i}(t) = \ \sum_{\kappa = 1}^{Κ}{d_{i,\kappa}Z_{\kappa,t}}\]
For some cancer types, the temporal trend was linear and in this case the area deviation was also linear.
The types of splines used varied by cancer type. For most cancer types, thin plate splines (Crainiceanu et al., 2005) were used but for the more common cancers basis splines (Wang and Yan, 2021) tended to provide a better fit. For basis splines, in the equations above \(Κ\) is the number of basis splines.
For each combination of cancer type and sex, multiple models were fitted with different numbers of knots to identify the best fitting model. Initially, multiple numbers of knots were fitted to the national data using a temporal model (as above, but without \(R_{i}\) or \(f_{i}(t)\)) and the optimal number of knots, \(Κ_{opt}\), for each cancer type and sex was identified using the WAIC. (Watanabe, 2010) A set of candidate numbers of knots for each cancer type and sex was then defined for the spatiotemporal models as \(\left\{ 1,\ 2,\ \ Κ_{opt} - 1,\ Κ_{opt} \right\}\). In practice, \(Κ_{opt}\) was typically one or two and so only one or two knots were fitted to most cancer types. After the spatiotemporal models were fitted using this set of candidates, the best number of knots fitted to each cancer type and sex was selected using WAIC, Moran’s ST (Anderson and Ryan, 2017) of the residuals and other model fit considerations. If Moran’s ST values were large (typically, absolute value greater than 0.01) then basis spline models were fitted.
Other priors and hyperpriors
The intercepts and coefficients were given Gaussian priors, each with unique precisions. For example, \(\beta_{0}\) and \(\beta_{1}\) were independently sampled.
\[\beta_{0},\ \beta_{1}\ \sim\ N(0,\ \tau_{\beta})\]
\[\tau_{\beta}\ \sim\ Gamma(1,\ 0.01)\]
Similarly, the precision on the spatial random effects also had a gamma prior.
\[\tau_{R}\ \sim\ Gamma(1,\ 0.01)\]
The mixing parameter was given a flat beta prior.
\[\rho\ \sim\ beta(1,\ 1)\]
Calculating the expected counts
For “Changes over time for each geographical area”, the age-specific rates (\(r_{k}\)) were calculated using data aggregated across the entire period.
\[r_{k} = \frac{number\ of\ cancers\ diagnosed\ in\ Australia\ in\ age\ group\ k}{number\ of\ people\ in\ Australia\ in\ age\ group\ k}\]
The expected counts used the \(r_{k}\) and the population residing in area \(i\) at time \(t\) in age group \(k\):
\[E_{it} = \ \sum_{k = 1}^{15}{r_{k}\ \times \ (number\ of\ people\ in\ area\ i\ at\ time\ t\ in\ age\ group\ k})\]
where \(k\) = 1 represents ages 15-19 and \(k\) = 15 represents ages 85+.
For “Geographical patterns (separate time periods)”, the age-specific rates (\(r_{kt}\)) were calculated for each time point.
\[r_{kt} = \frac{number\ of\ cancers\ diagnosed\ in\ Australia\ in\ age\ group\ k\ at\ time\ t}{number\ of\ people\ in\ Australia\ in\ age\ group\ k\ at\ time\ t}\]
\[E_{it} = \ \sum_{k = 1}^{15}{r_{kt}\ \times \ (number\ of\ people\ in\ area\ i\ at\ time\ t\ in\ age\ group\ k})\]
8.4.3.1 Bayesian spatiotemporal models for cancer diagnosis
8.4.3.1.1 Input data set
Each row of the input data set represented a unique time point and small area.
Variable | Value |
---|---|
idarea | A unique identification number for each small area. The numbers should be consecutive values from 1 to N, where N is the total number of areas |
yeargrp | The time point (year or year group) |
observed | Observed number of diagnosed cancer cases |
expect | Expected count of diagnosed cancer cases |
The expected count should be greater than zero. Because area-level population sizes change over time, there were some areas with an expected count of zero. The expected counts for these areas were set to a nominal value of 0.001 times the smallest non-zero expected count in the dataset, as shown in the R code below.
8.4.3.1.2 Adjacency matrix
An adjacency matrix specifies the spatial structure of the small areas, with a unique row and column for each area. For the Australian Cancer Atlas, a first-order adjacency matrix was used, in which two areas were considered neighbours if they shared a common geographical boundary. Small areas without any shared boundaries (for example islands) were manually assigned at least one neighbour (usually the closest mainland area).
The column and row number for each area corresponds to the area’s unique identification number (“idarea”, as described above). The \((i,{h)}^{th}\) element of the matrix was 1 if areas \(i\) and \(h\) share a border, and 0 if \(i = h\) or if areas \(i\) and \(h\) do not share a border. The matrix must be symmetric, that is the \((i,{h)}^{th}\) element is equal to the \(({h,i)}^{th}\)element.
8.4.3.1.3 R code
library(nimble)
# W is the adjacency matrix
## Setting up input data for spatiotemporal model
# The input data, 'dat.in' are as described in the above table, where
# idarea is the unique area ID and corresponds to the area's row / column number in W
# yeargrp is the time point
# observed is the observed number of diagnoses for that area and time point
# expect is the expected number of diagnoses for that area and time point
# num.knots is the number of knots to be fitted. This code assumes that num.knots > 1
# The number of areas (from the adjacency matrix)
N = ncol(W)
# Obtain scaling factor for BYM2 from adjacency matrix
# ICAR precision matrix
Q = apply(W, 1, sum) * diag(N) - W
# Add a small jitter for numerical stability
Q_pert <- Q + diag(N) * max(Matrix::diag(Q)) * sqrt(.Machine$double.eps)
# Compute the diagonal elements of the covariance matrix subject to the
# constraint that the entries of the ICAR sum to zero
Q_inv <- function(Q){
Sigma <- Matrix::solve(Q)
A <- matrix(1,1, NROW(Sigma))
W <- Sigma %*% t(A)
Sigma <- Sigma - W %*% solve(A %*% W) %*% Matrix::t(W)
return(Sigma)
}
Q_inv <- .Q_inv(Q_pert)
# Compute the geometric mean of the variances (diagonal of Q_inv)
scaling.factor <- exp(mean(log(Matrix::diag(Q_inv))))
# Obtain adjacency information
adj.info <- as.carAdjacency(W)
L = length(adj.info$adj)
# Correct the expected counts so all are greater than zero
if (any(dat.in$expect == 0)) dat.in$expect[dat.in$expect == 0] = min(dat.in$expect[dat.in$expect != 0]) / 1000
# Get standardised time variable
time.ids <- sort(unique(dat.in$yeargrp))
dat.in$idtime <- match(dat.in$yeargrp, time.ids)
X = (dat.in$idtime - mean(dat.in$idtime)) / sd(dat.in$idtime)
# Get spline design matrix
knots <- quantile(X, seq(0, 1, length=(num.knots+2))[-c(1,(num.knots+2))])
Z_K <- (abs(outer(X, knots,"-")))^3
OMEGA_all <- (abs(outer(knots, knots, "-")))^3
svd.OMEGA_all <- svd(OMEGA_all)
sqrt.OMEGA_all <- t(svd.OMEGA_all$v %*%
(t(svd.OMEGA_all$u)*sqrt(svd.OMEGA_all$d)))
Z <- t(solve(sqrt.OMEGA_all,t(Z_K)))
constants <- list(n = nrow(dat.in),
expect = dat.in$expect,
X = X, Z = Z, num.knots = num.knots,
Area = dat.in$idarea,
nAreas = N, scaling.factor = scaling.factor,
adj = adj.info$adj, weights = adj.info$weights, num = adj.info$num, L=L
)
# Initial values
inits <- list(
beta = rnorm(2),
b = rnorm(num.knots),
d = matrix(rnorm(num.knots*N), ncol = num.knots),
theta = rnorm(N),
phi = rnorm(N),
taub = rgamma(1,1,0.1),
tau.u = rgamma(1,1,0.1),
taud = rgamma(1,1,0.1),
taubeta = rgamma(1,1,0.1),
rho = rbeta(1,1,1)
)
# Set initial values for d to sum to zero for all knots, k
for (k in 1:ncol(inits$d)) inits$d[,k] = inits$d[,k] - mean(inits$d[,k])
source(nimble.model.code)
# Parameters to monitor in Nimble
parameters2 <- grep("^delta|^d$|theta|phi", names(inits), value = T)
parameters <- c(setdiff(names(inits), parameters2), "mu")
Rmodel <- nimbleModel(model.code,
data = list(obs = dat.in$observed),
inits = inits,
constants = constants
)
mcmcConf <- configureMCMC(Rmodel,
monitors = parameters,
monitors2 = parameters2
)
# Place block sampler on national trend parameters
tmp = c("beta[1]", "beta[2]", paste0("b[", 1:num.knots, "]"))
mcmcConf$removeSamplers(tmp)
mcmcConf$removeSamplers(tmp)
mcmcConf$addSampler(target = tmp,
type = "RW_block")
Rmcmc <- buildMCMC(mcmcConf, nchains = 1)
Cmodel <- compileNimble(Rmodel)
Cmcmc <- compileNimble(Rmcmc, project = Rmodel)
mcmc.out <- runMCMC(Cmcmc,
niter = 100000,
nburnin = 50000,
thin = 5,
thin2 = 50
)
### Nimble code
library(nimble)
model.code <- nimbleCode({
for (idx in 1:n) {
obs[idx] ~ dpois(mu[idx])
log(mu[idx]) <- log(expect[idx]) +
beta[1] +
beta[2] * X[idx] +
delta[Area[idx]] +
b[1] * Z[idx,1] +
b[2] * Z[idx,2] +
d[Area[idx],1] * Z[idx, 1] +
d[Area[idx],2] * Z[idx,2]
}
# Priors for the national temporal curve
for (k in 1:num.knots) { b[k] ~ dnorm(0, taub) }
for (be in 1:2) { beta[be] ~ dnorm(0, taubeta) }
# Prior for the spline coefficients for the areal deviations from the national temporal curve
for (i in 1:nAreas) {
for (k in 1:num.knots) {
d[i,k] ~ dnorm(0, taud)
}
}
# Spatial effects
for (i in 1:nAreas) {
delta[i] <- (1/sqrt(tau.u))*(sqrt((1-rho))*theta[i] + sqrt(rho/scaling.factor)*phi[i])
theta[i] ~ dnorm(0, tau = 1)
}
phi[1:nAreas] ~ dcar_normal(adj[1:L], weights[1:L], num[1:nAreas], tau = 1, zero_mean = 1)
# Priors on precision parameters
taub ~ dgamma(1, 0.01)
taubeta ~ dgamma(1, 0.01)
taud ~ dgamma(1, 0.01)
# BYM2 hyperpriors
tau.u ~ dgamma(1, 0.01)
rho ~ dbeta(1, 1)
})