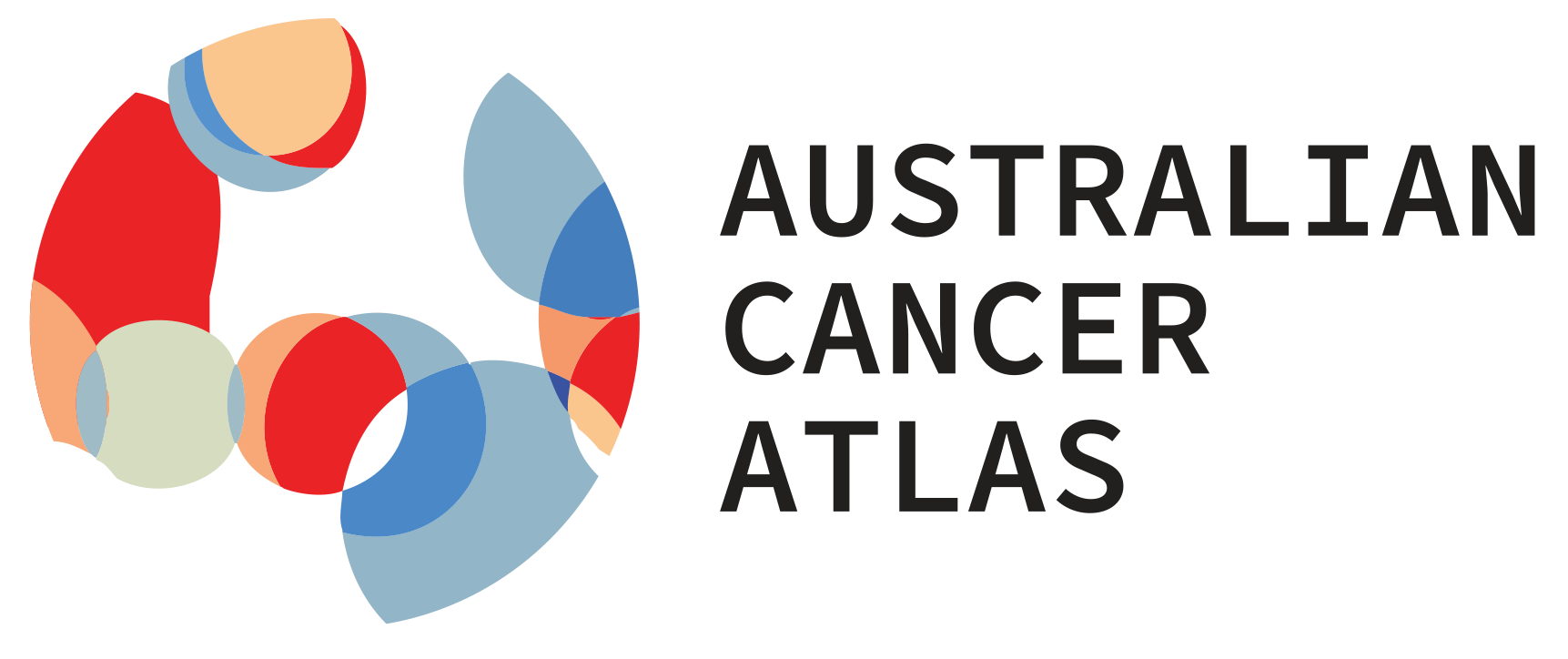
11 Cancer screening/testing
11.1 PSA testing
The PSA (prostate specific antigen) is a protein produced by the prostate – a small gland below the bladder of men. Prostate-specific antigen (PSA) testing is commonly employed on an opportunistic basis to assess the risk of prostate cancer in men who have no symptoms. However, its low positive predictive value (proportion of positive tests that are actually prostate cancer) poses challenges in correctly distinguishing between cancerous and benign conditions of the prostate.
As a result, this test is typically conducted sporadically, including in Australia, rather than being integrated into an organised, population-wide screening program. This is very different to screening programs for breast cancer, bowel cancer and cervical cancer.
In 2016, the Prostate Cancer Foundation of Australia (PCFA) and Cancer Council Australia (CCA) issued national guidelines recommending that men at average risk of prostate cancer, aged 50 to 69 years, make an informed decision to have a regular PSA test, which should be offered every two years. (PCFA and CCA, 2015) Notably, summary guidelines from the Royal Australian College of General Practitioners (RACGP) advise general practitioners that, since screening asymptomatic men with PSA is not recommended, they are not obligated to offer the test. (RACGP, 2016) The PCFA/CCA guidelines are being reviewed in 2024.
The Australian Cancer Atlas reports spatial variation in PSA testing among males aged 50-79 between 2017 and 2018.
11.1.1 Data sources
Medicare data
PSA testing data were extracted from the Medicare Benefits Schedule (MBS) data collection. Data was provided by the Commonwealth Department of Health for item number 66655 which is all about checking for prostate issues in men who do not have symptoms. The provided data included a unique (deidentified) person number, age (50-79 in 10-year age groups), month and year of test and postcode of residence at time of PSA testing.
Population data
Population data by SA2 (2016 ASGS) and 5-year age groups for males for 2018 and 2019 was obtained from the Australian Bureau of Statistics. (Australian Bureau of Statistics, 2022a)
Geographical data
Of 2,288 SA2 (2016) areas in Australia that were potentially eligible for analysis after excluding 18 SA2s with no spatial information and four remote islands (see Section 4.1), a further 99 SA2s were excluded as the average annual population of males aged 50-79 from 2017 to 2018 was less than five. This means the Australian Cancer Atlas provides modelled estimates for PSA testing for 2,189 SA2s across Australia.
Geographical concordance
Residential location at time of PSA testing was available at postcode level. The geographical boundaries of postcodes themselves can be difficult to quantify and there is no direct correspondence available between postcode and SA2s. However, the Australian Bureau of Statistics has generated Postal Areas, which enable the release of Census data by areas that are as close as possible to postcodes. (Australian Bureau of Statistics, 2016c)
A probability-based geographical correspondence file was used to transform postcode information to 2016 SA2. (Australian Bureau of Statistics Geospatial Solutions, 2023) Postcodes can encompass either a whole or partial section of one or more SA2s. The correspondence file contained the proportion of population within each postcode allocated to each SA2. Using these proportions, each record was randomly allocated to a relevant SA2 according to the uniform distribution. The allocation process was repeated 50 times to incorporate the uncertainty in the postcode-to-SA2 correspondence via the probabilistic allocation of SA2s, generating 50 different allocated datasets.
11.1.2 Statistical methods
The analyses were only conducted for PSA testing data collected for males aged 50-79. Multiple PSA screening tests within a year was counted as once for that year.
11.1.2.1 National summary measures
The reported national summary measures were the total number of males aged 50-79 who had at least one PSA test during 2017-2018 and the percentage of eligible population tested.
11.1.2.2 Spatial models
For each SA2, participation in PSA testing was quantified using the indirectly age-standardised participation ratio (SPR), which is the ratio of the observed versus expected counts of males who had PSA testing from 2017 to 2018.
Following a process of model testing and evaluation, (Kohar et al., 2023) the model selected for PSA testing was the Leroux model. (Leroux et al., 2000)
The Leroux model applied a Poisson distribution with an offset of the logged expected counts in each small area. Expected counts were calculated by multiplying national age-specific rates by the age-specific population in each small area, then summing all age-specific expected counts. The expected counts and observed counts were input to the Bayesian model to calculate smoothed SPR estimates for each SA2 compared to the Australian average.
The first stage is the likelihood model for the observed count data, that is, the number of PSA tests, Yi. in area i:
Yi ∼ Poisson(Eiλi )for i=1………, N areas
where Ei is the expected number of PSA tests for each SA2 of usual residence at PSA testing and 5-year age group from ages 50 to 79 (50-54, 55-59, ……75-79). The expected number of PSA tests was calculated as the:
Ei=6∑age group=1Number of PSA tests in Australia in the age groupAustralian male population in the age groupSA2 population for the age group
where age group=1 represents ages 50-54 and age group=6 represents ages 74-79. This used data aggregated over 2 years (2017-2018).
Modelled estimates are effectively age-adjusted since Ei considers the age structure and population size.
The second stage comprises an expression for the SPR (λi), which is the key output used in the Australian Cancer Atlas.
log(λi)= β+Si
The parameter β is the intercept, while Si represents the spatial random effect for area i. The specification of these spatial random effects determines how the smoothing is performed, and together with the specification of the intercept parameter, forms the third stage of the model in the form of prior distributions.
The prior for the intercept was a weakly informative Gaussian distribution with zero mean.
β ∼ N(0,100000)
The spatial random effects Siwere modelled by the Leroux prior, (Leroux et al., 2000) which is a Gaussian distribution, and its mean is the weighted average of the neighbouring areas’ random effects (with weight ρ) and a global mean of 0 (with weight 1− ρ).
Si|S∖i ∼ N(ρΣhwihShρΣhwih+1−ρ, σ2SρΣhwih+1−ρ)
The spatial weights wih represent the spatial proximity between areas i and h, and collectively they comprise an N×N spatial adjacency matrix W. A binary weighting scheme was used, so that wih=1 if areas i and h were considered to be neighbours, and otherwise wih=0.
To complete the Bayesian model specification, the variance σ2S was also given a weakly informative prior:
σ2S ∼ InverseGamma(1, 0.01)
which is an inverse-gamma distribution with parameters shape and scale. The prior for the spatial smoothing parameter ρ was weakly informative.
ρ ∼ Uniform(0,1)
Small area-specific iterations from 50 models were combined with equal weighting, resulting in 500,000 iterations for each small area. Results are based on the median value of the estimates over these 500,000 iterations. (Kohar et al., 2023)
The Australian cancer Atlas reports the estimated smoothed SPR (relative) and modelled counts (absolute) for males who had at least one PSA test from 2017 to 2018 by SA2s across Australia.
11.1.2.3 Bayesian spatial model for PSA testing
11.1.2.3.1 Input data set
Key variables in this data set are:
Variable | Value |
---|---|
grid | A unique identification number for each small area. The numbers should be consecutive values from 1 to N, where N is the total number of areas |
observed | Observed number of males who had PSA testing |
expect | Expected count of males having PSA testing |
The data should be sorted by grid. Expected counts should be greater than zero.
11.1.2.3.2 Adjacency matrix
An adjacency matrix specifies the spatial structure of the small areas, with a unique row and column for each area. For the Australian Cancer Atlas, a first-order adjacency matrix was used, in which two areas were considered to be neighbours if they shared a common geographical boundary. Small areas without any shared boundaries (for example islands) were manually assigned at least one neighbour (usually the closest mainland area).
The column and row number for each area corresponds to the area’s unique identification number (“grid”, as described above). The (i,h)th element of the matrix was 1 if areas i and h share a border, and 0 if i=h or if areas i and h do not share a border. The matrix must be symmetric, that is the (i,h)th element is equal to the (h,i)thelement.
11.1.2.3.3 R code
# Required libraries
library(CARBayes)
# Setting up input data for spatial model
# data$observed is the observed number of males who had PSA testing in a small area
# data$expect is the expected number of males having PSA testing based on the
# age-specific national rates applied to the age-specific
# population eligible for PSA testing within the same small area.
# data$grid is the identifier for a small area (values 1,.., N, N is the total
# number of small areas) which needs to be the same in the data file and the adjacency matrix file
file.input =
data.frame(
obs = data$observed,
expect = data$expect,
grid = data$grid)
# Model specification using the CARBayes package with the Leroux prior and a Poisson likelihood
# Default priors were used
formula <- obs ~ offset(log(expect))
MCMC <- S.CARleroux(
formula = formula,
data = file.input,
family = "poisson",
W = W, # The adjacency matrix
burnin = 50000,
n.sample = 150000,
thin = 10)
# Calculate the standardised participation ratio (SPR) for each MCMC iteration
spr.mcmc <-
MCMC$samples$fitted /
matrix(
rep(file.input$expect, nrow(MCMC$samples$fitted)),
byrow = T, ncol = ncol(MCMC$samples$fitted)
)
# Calculate median, credible interval and exceedance probability (EP) for the SPRs
SPR <-
data.frame(
grid = file.input$grid,
median = apply(spr.mcmc, 2, median),
LB = apply(spr.mcmc, 2, quantile, probs = 0.025),
UB = apply(spr.mcmc, 2, quantile, probs = 0.975),
EP = apply(spr.mcmc, 2, function(x) sum(x > 0) / nrow(spr.mcmc))
)
# Calculate median and credible interval for the modelled count estimates
modelled.counts <-
data.frame(
grid = file.input$grid,
median = apply(MCMC$samples$fitted, 2, median),
LB = apply(MCMC$samples$fitted, 2, quantile, probs = 0.025),
UB = apply(MCMC$samples$fitted, 2, quantile, probs = 0.975)
)
11.2 Breast cancer screening
Early detection of breast cancer through mammographic screening improves prognosis by providing an opportunity for early and more effective treatment. The BreastScreen Australia (BreastScreen Australia) program invites women aged 50-74 years to have a free mammogram once every two years. (Lauby-Secretan et al., 2015) Approximately 50% of Australian women aged 50-74 years participated in BreastScreen Australia in 2019 and 2020. (Australian Institute of Health and Welfare, 2023b)
The Australian Cancer Atlas reports spatial variation in breast cancer screening among females aged 50-74 years who participated in BreastScreen Australia in 2019 and 2020. Note that these data do not include screening mammograms conducted through private clinics, the number of which is not known.
11.2.1 Data sources
11.2.1.1 BreastScreen data
Breast Screen data for 2019 and 2020 was obtained via a specific data request from the Australian Institute of Health and Welfare. Data extracted included a unique (deidentified) person number, age at screening, date of first attendance (for each screening episode) and postcode of residence at time of screening.
Since residential location at time of screening through BreastScreen Australia was only available at postcode level, and a single postcode can cross multiple SA2 boundaries, a probability-based geographical correspondence was used to reallocate postcode information to 2016 SA2. (Australian Bureau of Statistics Geospatial Solutions, 2023) The correspondence proportions were adjusted to account for the fraction of the population that were eligible, using age- and sex-specific population data by SA2 and assuming that the proportion of the population eligible for the BreastScreen program was homogenous across the SA2. The allocation process was repeated 50 times to incorporate the uncertainty in the postcode-to-SA2 conversion generating 50 different allocated datasets.
11.2.1.2 Population data
Population data by SA2 (2016 ASGS) and 5-year age groups for females for 2019 and 2020 was obtained from the Australian Bureau of Statistics. (Australian Bureau of Statistics, 2022a)
11.2.1.3 Geographical data
There were 2,288 SA2 (2016) areas in Australia that were potentially eligible for analysis after excluding 18 SA2s with no spatial information (as these were for people without a fixed residential location) and four remote islands (see Section 4.1). A further, 99 SA2s were excluded as the average annual population of females aged 50-74 years old in 2019 and 2020 was less than five. Consequently, the Australian Cancer Atlas provides modelled estimates for participation in BreastScreen Australia for 2,190 SA2s across Australia.
11.2.2 Statistical methods
Reflecting the recommendations for BreastScreen, the analyses of breast screening participation included females aged 50-74 years in 2019 and 2020. For each unique identification number, only the first screening episode within the two-year period was included in the modelling.
11.2.2.1 National summary measures
The reported national summary measures were the total number of women aged 50-74 who had at least one screening episode in 2019 and 2020 and the percentage of the eligible population who participated in BreastScreen Australia.
11.2.2.2 Spatial models
For each SA2, participation in BreastScreen Australia was quantified as the modelled number of participants and the relative participation ratio (RPR), which is the area’s modelled participation rate divided by the Australian average participation rate. The BreastScreen participation models were not age-adjusted because the eligible age range is narrow, and participation should not vary by age within the eligible age range.
The model
A variety of spatial models were trialled and the model with the best fit included a binomial likelihood with the BYM2 spatial prior. (Riebler et al., 2016) The model inputs were the observed counts (Yi) and the estimated eligible resident population (popi) for each area i. The population data were averaged over the two years. The first stage of the model also included the modelled participation rate for each area (pi).
Yi∼Binomial(pi, popi)
The RPR displayed in the maps was calculated using pi and the national participation (pAust), as shown below.
pAust=ΣiYiΣipopi
RPRi=pi/pAust
In the second stage of the model, the participation rate was modelled by an intercept (β) and a spatial random effect (Ri), using a “logit” link function.
log(pi1−pi)=β+Ri
The spatial effects were modelled by a BYM2 prior, (Riebler et al., 2016) which is a weighted average between a spatially structured effect (ϕi) and a Gaussian effect with zero mean (θi):
θi ∼ N(0, 1)
ϕi|ϕ∖i ∼ N(∑hwihϕh∑hwih, 1∑hwih)
Ri=1√τR(θi√1−ρ+ ϕi√ρ/ι)
where ι is a scaling factor calculated from the adjacency matrix, W, which is comprised of the spatial weights wih. These weights represent the spatial proximity between areas i and h. A binary weighting scheme was used, so that wih=1 if areas i and h were considered neighbours and wih=0 otherwise.
The intercept had a Gaussian prior, the precision of which was drawn from a Gamma prior.
β ∼ N(0, τβ)
τβ ∼ Gamma(1, 0.01)
Similarly, the precision on the spatial random effects also had a gamma prior.
τR ∼ Gamma(1, 0.01)
The mixing parameter had a beta prior with uniform distribution.
ρ ∼ beta(1, 1)
11.2.2.3 Bayesian spatial model for participation in the BreastScreen program
11.2.2.3.1 Input data set
Key variables in this data set are:
Variable | Value |
---|---|
grid | A unique identification number for each small area. The numbers should be consecutive values from 1 to N, where N is the total number of areas |
observed | Observed number of female BreastScreen participants aged 50 to 74 |
pop | Estimated resident female population aged 50 to 74, averaged over 2-years |
11.2.2.3.2 Adjacency matrix
An adjacency matrix specifies the spatial structure of the small areas, with a unique row and column for each area. For the Australian Cancer Atlas, a first-order adjacency matrix was used, in which two areas were considered neighbours if they shared a common geographical boundary. Small areas without any shared boundaries (for example islands) were manually assigned at least one neighbour (usually the closest mainland area).
The column and row number for each area corresponds to the area’s unique identification number (“grid”, as described above). The (i,h)th element of the matrix was 1 if areas i and h share a border, and 0 if i=h or if areas i and h do not share a border. The matrix must be symmetric, that is the (i,h)th element is equal to the (h,i)thelement.
11.2.2.3.3 R code
library(nimble)
# W is the adjacency matrix
## Setting up input data for spatial model
# The input data, 'dat.in' are as described in the above table, where
# grid is the unique area ID and corresponds to the area's row / column number in W
# observed is the number of female BreastScreen participants
# pop is estimated resident female population averaged over 2-years eligible for screening
# The number of areas (from the adjacency matrix)
N = ncol(W)
# Obtain scaling factor for BYM2 from adjacency matrix
# ICAR precision matrix
Q = apply(W, 1, sum) * diag(N) - W
# Add a small jitter for numerical stability
Q_pert <- Q + diag(N) * max(Matrix::diag(Q)) * sqrt(.Machine$double.eps)
# Compute the diagonal elements of the covariance matrix subject to the
# constraint that the entries of the ICAR sum to zero
Q_inv <- function(Q){
Sigma <- Matrix::solve(Q)
A <- matrix(1,1, NROW(Sigma))
W <- Sigma %*% t(A)
Sigma <- Sigma - W %*% solve(A %*% W) %*% Matrix::t(W)
return(Sigma)
}
Q_inv <- .Q_inv(Q_pert)
# Compute the geometric mean of the variances (diagonal of Q_inv)
scaling_factor <- exp(mean(log(Matrix::diag(Q_inv))))
# Obtain adjacency information
adj.info <- as.carAdjacency(W)
L = length(adj.info$adj)
dat.input = list(obs = dat.in$observed)
constants = list(pop = dat.in$pop,
Area = dat.in$grid,
nr = nrow(dat.in),
adj = adj.info$adj, weights = adj.info$weights, num = adj.info$num,
scaling_factor = scaling_factor, L=L
)
# Initial values
inits = list(
beta = rnorm(1),
theta = rnorm(N),
phi = rnorm(N),
tau.u = rgamma(1, 0.01),
taubeta = rgamma(1, 0.01),
rho = rbeta(1,1,1)
)
source(nimble.model.code)
Rmodel <- nimbleModel(model.code,
data = list(obs = dat.in$observed),
inits = inits,
constants = constants
)
mcmcConf <- configureMCMC(Rmodel,
monitors = c(names(inits), "p")
)
Rmcmc <- buildMCMC(mcmcConf, nchains = 1)
Cmodel <- compileNimble(Rmodel)
Cmcmc <- compileNimble(Rmcmc, project = Rmodel)
mcmc.out <- runMCMC(Cmcmc,
niter = 100000,
nburnin = 50000,
thin = 10
)
11.2.2.3.4 Nimble code
model.code <- nimbleCode({
for (idx in 1:nr) {
obs[idx] ~ dbin(p[idx], pop[idx])
logit(p[idx]) <-
beta +
delta[Area[idx]]
}
# Priors for the intercept
beta ~ dnorm(0, taubeta)
# Spatial effects
for (i in 1:nr) {
delta[i] <- (1/sqrt(tau.u))*(sqrt((1-rho))*theta [i] + sqrt(rho/scaling_factor)*phi[i])
theta[i] ~ dnorm(0, tau = 1)
}
phi[1:nr] ~ dcar_normal(adj[1:L], weights[1:L], num[1:nr], tau = 1, zero_mean = 1)
# Priors of precision parameters
taubeta ~ dgamma(1, 0.01)
# BYM2 hyperpriors
tau.u ~ dgamma(1, 0.01)
rho ~ dbeta(1, 1)
})
Computation
To incorporate the uncertainty in the postcode-to-SA2 concordance, the model was fitted separately to each of the 50 reallocated data sets (Section 11.2.1.1), resulting in 50 sets of results. The MCMC samples from the 50 models were combined, resulting in 500,000 iterations for each small area. Point estimates and uncertainty measures were based on all these 500,000 iterations.
11.3 Bowel cancer screening
The National Bowel Cancer Screening Program (NBCSP, National Bowel Cancer Screening Program) aims at reducing bowel cancer related deaths by screening for early symptoms of the disease. The program sends eligible Australians aged 50-74 years old a free in-home test. The test measures presence of blood in the stool which is an early sign of bowel cancer.
The eligibility criteria for the National Bowel Cancer Screening Program include being an Australian or New Zealand citizen or permanent migrant with a current Medicare card (or registered as a Department of Veterans’ Affairs customer) in the invited age bracket and a current Australian mailing address.
Approximately 44% of eligible persons participated in the National Bowel Cancer Screening Program from 2019 to 2020. (Australian Institute of Health and Welfare, 2023a)
The Australian Cancer Atlas reports spatial variation in bowel cancer screening among persons aged 50-79 years who participated in the National Bowel Cancer Screening Program from 2019 to 2020. Note that these data do not include bowel cancer screening such as colonoscopies conducted outside the program (such as private clinics), the number of which is not known.
11.3.1 Data sources
11.3.1.1 National bowel cancer screening data
Publicly available population-level aggregated participation data for the National Bowel Cancer Screening Program, 2019-2020 was obtained from the Australian Institute of Health and Welfare. (Australian Institute of Health and Welfare, 2023f)
Data were available in two separate datasets: (a) national participation for all of Australia by sex and five-year age groups; and (b) participation for persons only by their location at the time of screening for 50-74 years combined. For each dataset, the following information was extracted: a) the number of eligible persons invited to screen (invitees) and b) the number of eligible persons who returned a completed bowel screening kit period within 2019 to 2020 or by 30 June of the following year 2021 (participants).
The reported number of eligible invitees (not participants) excluded those who deferred, opted out or skipped a screening round (from November 2019 onwards due to a colonoscopy within past two years) without completing their screening test.
11.3.1.2 Population data
Population data by SA2 (2016 ASGS) and 5-year age groups for persons for 2019 and 2020 was obtained from the Australian Bureau of Statistics. (Australian Bureau of Statistics, 2022a)
However, we also required the population by single year age groups since the National Bowel Cancer Screening Program eligibility was rolled out by single ages within each five-year age band. (Australian Institute of Health and Welfare, 2023g) We assumed that the population in each single year age group was spread equally within the relevant five-year age group.
11.3.1.3 Geographical data
Of 2,288 SA2 (2016) areas in Australia that were potentially eligible for analysis after excluding 18 non-spatial SA2s and remote islands (4), a further 50 were excluded (less than five residents annually on average). This means the Australian Cancer Atlas provides modelled estimates for bowel cancer screening through the National Bowel Cancer Screening Program for 2,238 SA2s across Australia.
Residential location when invited to screen in the National Bowel Cancer Screening Program was available at the 2016 SA2 level. SA2 areas were directly assigned to these invitees by the Australian Institute of Health and Welfare using longitude and latitude data. (Australian Institute of Health and Welfare, 2023g) For those invitees without reliable SA2 data, SA2s were assigned with a postcode to SA2 correspondence instead. Persons with unknown SA2s or small areas for which screening information was suppressed by the Australian Institute of Health and Welfare due to concerns about data quality were excluded.
11.3.2 Statistical methods
The analyses were conducted for bowel cancer screening data collected for persons aged 50-74 invited to screen through the National Bowel Cancer Screening Program in Australia from 2019 to 2020 who returned a completed screening test within that period or in first half of 2021.
11.3.2.1 National summary measure
The reported national summary measures were the total number of eligible persons aged 50-74 who participated in the National Bowel Cancer Screening Program from 2019 to 2020 and the percentage of eligible population who were screened.
11.3.2.2 Spatial models
For each SA2, participation in bowel cancer screening was quantified using the indirectly age-standardised participation ratio (SPR), which is the ratio of the observed versus expected counts of persons screened through the National Bowel Cancer Screening Program by SA2 from 2019 to 2020.
A Bayesian spatial model with the Leroux prior was used. (Leroux et al., 2000) The model applied a Poisson distribution with an offset of the logged expected counts in each small area. Expected counts were based on national age-specific rates. The expected counts and observed counts were input to the Bayesian model to calculate smoothed SPR estimates for each SA2 compared to the Australian average.
The first stage is the likelihood model for the observed count data, that is, observed count of screened persons, Yi. in area i:
Yi ∼ Poisson(Eiλi )for i=1………, N areas
where Ei is the expected count of screened persons for each SA2 of usual residence for participants in the National Bowel Cancer Screening Program when screened and 5-year age group from ages 50 to 74 (50-54, 55-59, ……75-79).
The second stage comprises an expression for the SPR (λi), which is the key output used in the Australian Cancer Atlas.
log(λi)= β+Si
The parameter β is the intercept, while Si represents the spatial random effect for area i. The specification of these spatial random effects determines how the smoothing is performed, and together with the specification of the intercept parameter, forms the third stage of the model in the form of prior distributions.
The prior for the intercept was a weakly informative Gaussian distribution with zero mean.
β ∼ N(0,100000)
The spatial random effects Siwere modelled by the Leroux prior, (Leroux et al., 2000) which is a Gaussian distribution, and its mean is the weighted average of the neighbouring areas’ random effects (with weight ρ) and a global mean of 0 (with weight 1− ρ).
Si|S∖i ∼ N(ρΣhwihShρΣhwih+1−ρ, σ2SρΣhwih+1−ρ)
The spatial weights wih represent the spatial proximity between areas i and h, and collectively they comprise an N×N spatial adjacency matrix W. A binary weighting scheme was used, so that wih=1 if areas i and h were considered to be neighbours, and otherwise wih=0.
To complete the Bayesian model specification, the variance σ2S was also given a weakly informative prior:
σ2S ∼ InverseGamma(1, 0.01)
which is an inverse-gamma distribution with parameters shape and scale. The prior for the spatial smoothing parameter ρ was weakly informative.
ρ ∼ Uniform(0,1)
The Australian Cancer Atlas reports the modelled SPR and estimated modelled counts for persons who had a bowel cancer screening test through the National Bowel Cancer Screening Program by SA2s across Australia from 2019 to 2020.
11.3.2.3 Bayesian spatial model for bowel cancer screening
11.3.2.3.1 Input data set
Key variables in this data set are:
Variable | Value |
---|---|
grid | A unique identification number for each small area. The numbers should be consecutive values from 1 to N, where N is the total number of areas |
observed | Observed number of persons who had bowel cancer screening |
expect | Expected count of persons having bowel cancer screening |
The data should be sorted by grid. Expected counts should be greater than zero.
11.3.2.3.2 Adjacency matrix
An adjacency matrix specifies the spatial structure of the small areas, with a unique row and column for each area. For the Australian Cancer Atlas, a first-order adjacency matrix was used, in which two areas were considered to be neighbours if they shared a common geographical boundary. Small areas without any shared boundaries (for example islands) were manually assigned at least one neighbour (usually the closest mainland area).
The column and row number for each area corresponds to the area’s unique identification number (“grid”, as described above). The (i,h)th element of the matrix was 1 if areas i and h share a border, and 0 if i=h or if areas i and h do not share a border. The matrix must be symmetric, that is the (i,h)th element is equal to the (h,i)thelement.
11.3.2.3.3 R code
## Required libraries
library(CARBayes)
## Setting up input data for spatial model
## data$observed is the observed number of persons who had bowel cancer screening in a small area.
## data$expect is the expected number of persons having bowel cancer screening based
# on the age-specific national rates applied to the age-specific population eligible
# for screening within the same small area.
## data$grid is the identifier for a small area (values 1,.., N, N is the total number
# of small areas) which needs to be the same in the data file and the adjacency matrix file.
file.input =
data.frame(
obs = data$observed,
expect = data$expect,
grid = data$grid)
# Model specification using the CARBayes package with the Leroux prior and a Poisson likelihood
# Default priors were used
formula <- obs ~ offset(log(expect))
MCMC <- S.CARleroux(
formula = formula,
data = file.input,
family = "poisson",
W = W, # The adjacency matrix
burnin = 50000,
n.sample = 150000,
thin = 10
)
# Calculate the standardised participation ratio (SPR) for each MCMC iteration
spr.mcmc <-
MCMC$samples$fitted /
matrix(
rep(file.input$expect, nrow(MCMC$samples$fitted)),
byrow = T, ncol = ncol(MCMC$samples$fitted)
)
# Calculate median, credible interval and exceedance probability (EP) for the SPRs
SPR <-
data.frame(
grid = file.input$grid,
median = apply(spr.mcmc, 2, median),
LB = apply(spr.mcmc, 2, quantile, probs = 0.025),
UB = apply(spr.mcmc, 2, quantile, probs = 0.975),
EP = apply(spr.mcmc, 2, function(x) sum(x > 0) / nrow(spr.mcmc))
)
# Calculate median and credible interval for the modelled count estimates
modelled.counts <-
data.frame(
grid = file.input$grid,
median = apply(MCMC$samples$fitted, 2, median),
LB = apply(MCMC$samples$fitted, 2, quantile, probs = 0.025),
UB = apply(MCMC$samples$fitted, 2, quantile, probs = 0.975)
)
Calculating the expected counts
The expected counts of persons screened was calculated as shown below.
Ei,50−74=(K∑kRateAustraliak ×Inviteesik)/100
The expected counts in each SA2 (i) for 2019 to 2020 was calculated by multiplying the age-specific national participation rate (per 100 population) (RateAustraliak) by the age-specific eligible population (Inviteesik) for each five-year age group k (50-54, 55-59, 60-64, 65-69, 70-74).
The age-specific national participation rate (Rate_Australiak) for each five-year age group k was defined as the ratio of the total number of participants (CountAustralia_s) to the total number of eligible persons invited to screen (invitees) from 2019 to 2020, where s is the single year of age in each five-year age group. The age-specific eligible population was estimated by multiplying the SA2-specific eligible population by the proportion of eligible population in that age group for all of Australia. Further adjustments were made to account for the effect of the discrepancy between the eligible population and the eligible screening population. (Dasgupta et al., 2023b)
11.4 Cervical screening
The National Cervical Screening Program (NCSP, National Cervical Screening Program) aims at reducing illness and deaths from cervical cancer by detecting and potentially treating pre-cancerous abnormalities early before progressing to cervical cancer. Cervical screening involves a five-yearly human papillomavirus (HPV) based test that looks for oncogenic (cancer causing) HPV among eligible women aged 25-74 years. (Australian Institute of Health and Welfare, 2023c) Only women who have an intact cervix (that is those who have not had a hysterectomy) are eligible.
Data on the number of eligible Australian women in the targeted population who were involved in the National Cervical Screening Program over a five-year reporting period is available for two measures. The first termed ‘participation’ is restricted to only screening HPV tests, whereas second termed ‘coverage’ is not restricted in this way and includes all HPV or liquid-based cytology (LBC) tests for any reason (including primary or follow up screening and diagnostic investigations). While both these measures are valid indicators of participation in cervical screening in Australia, coverage is a better indicator of overall participation, as it represents the number of eligible women who have had any cervical screening test. (Australian Institute of Health and Welfare, 2023c) It is therefore appropriate for comparisons within Australia if overall involvement in cervical screening is the measure of interest. Hence it was used as the measure of cervical screening participation for Atlas 2.0.
Approximately 68% of eligible women had a screening HPV test with 77% overall participation in cervical screening from 2018 to 2022. (Australian Institute of Health and Welfare, 2023c)
The Australian Cancer Atlas reports spatial variation in cervical screening among females aged 25-74 years who were eligible for the National Cervical Cancer Screening Program from 2018 to 2022.
11.4.1 Data sources
11.4.1.1 National cervical screening data
Publicly available population-level aggregated recruitment data for the National Cervical Screening Program between 2018 and 2022 was obtained from the Australian Institute of Health and Welfare. (Australian Institute of Health and Welfare, 2023h)
Data were available in two separate datasets: (a) national participation for all of Australia by five-year age groups; and (b) participation by residential location at the time of cervical testing for females aged 25-74 years combined. For each dataset, the following information was extracted: a) the number of eligible population and b) the number of eligible females who had any cervical screening test.
Eligible population was the average Australian Bureau of Statistics estimated population for females aged 25-74 between 2018 to 2022, adjusted to exclude the estimated number of females who had a hysterectomy (using age-specific hysterectomy fractions derived from the National Hospital Morbidity Database). (Australian Institute of Health and Welfare, 2023c)
Note the available participation data excluded those currently participating in an ongoing clinical trial (“Compass”). This affects Victoria more than other jurisdictions. (Australian Institute of Health and Welfare, 2023c)
11.4.1.2 Population data
Population data by SA2 (2016 ASGS) and 5-year age groups for females from 2018 to 2022 was obtained from the Australian Bureau of Statistics. (Australian Bureau of Statistics, 2022a) Population estimates were adjusted to remove the estimated number of females who underwent a hysterectomy, based on published age-specific National Hysterectomy Fractions. (Australian Institute of Health and Welfare, 2023c)
11.4.1.3 Geographical data
Of 2,288 SA2 (2016) areas in Australia that were potentially eligible for analysis after excluding 18 non-spatial SA2s and remote islands (4), a further 77 were excluded as the average annual population of females aged 25-74 years old between 2018 and 2022 was less than five. This means the Australian Cancer Atlas provides modelled estimates for cervical screening for 2,211 SA2s across Australia.
Residential location at time of cervical screening was available at the 2016 SA2 level. SA2 areas were directly assigned by the Australian Institute of Health and Welfare using longitude and latitude data. (Australian Institute of Health and Welfare, 2023g) For records without reliable SA2 data, SA2s were assigned with a postcode to SA2 correspondence instead. Records with unknown SA2s or small areas for which screening information was suppressed by the Australian Institute of Health and Welfare due to concerns about data quality were excluded.
11.4.2 Statistical methods
The analyses were conducted for cervical tests among females aged 25-74 from 2018 to 2022.
11.4.2.1 National summary measure
The reported national summary measures were the total number of females aged 25-74 years who had cervical screening between 2018 to 2022 and the percentage of eligible population who were screened.
11.4.2.2 Spatial models
For each SA2, cervical screening participation was quantified using the indirectly age-standardised participation ratio (SPR), which is the ratio of the observed versus expected counts of screened women by SA2 from 2018 to 2022.
A Bayesian spatial model with the Leroux prior was used. (Leroux et al., 2000) The model applied a Poisson distribution with an offset of the logged expected counts in each small area. Expected counts were based on national age-specific rates. The expected counts and observed counts were input to the Bayesian model to calculate smoothed SPR estimates for each SA2 compared to the Australian average.
The first stage is the likelihood model for the observed count data, that is, observed count of screened women, Yi. in area i:
Yi ∼ Poisson(Eiλi )for i=1………, N areas
where Ei is the expected count of screened women for each SA2 of usual residence at cervical screening and 5-year age group from ages 25 to 74 (25-29, 30-34……75-79).
The second stage comprises an expression for the SPR (λi), which is the key output used in the Australian Cancer Atlas.
log(λi)= β+Si
The parameter β is the intercept, while Si represents the spatial random effect for area i. The specification of these spatial random effects determines how the smoothing is performed, and together with the specification of the intercept parameter, forms the third stage of the model in the form of prior distributions.
The prior for the intercept was a weakly informative Gaussian distribution with zero mean.
β ∼ N(0,100000)
The spatial random effects Siwere modelled by the Leroux prior, (Leroux et al., 2000) which is a Gaussian distribution, and its mean is the weighted average of the neighbouring areas’ random effects (with weight ρ) and a global mean of 0 (with weight 1− ρ).
Si|S∖i ∼ N(ρΣhwihShρΣhwih+1−ρ, σ2SρΣhwih+1−ρ)
The spatial weights wih represent the spatial proximity between areas i and h, and collectively they comprise an N×N spatial adjacency matrix W. A binary weighting scheme was used, so that wih=1 if areas i and h were considered to be neighbours, and otherwise wih=0.
To complete the Bayesian model specification, the variance σ2S was also given a weakly informative prior:
σ2S ∼ InverseGamma(1, 0.01)
which is an inverse-gamma distribution with parameters shape and scale. The prior for the spatial smoothing parameter ρ was weakly informative.
ρ ∼ Uniform(0,1)
The Australian Cancer Atlas reports the modelled SPR and estimated modelled counts for females who underwent cervical screening by SA2s across Australia from 2018 to 2022.
11.4.2.3 Bayesian spatial model for cervical screening
11.4.2.3.1 Input data set
Key variables in this data set are:
Variable | Value |
---|---|
grid | A unique identification number for each small area. The numbers should be consecutive values from 1 to N, where N is the total number of areas |
observed | Observed number of females who had cervical screening |
expect | Expected count of females having cervical screening |
The data should be sorted by grid. Expected counts should be greater than zero.
11.4.2.3.2 Adjacency matrix
An adjacency matrix specifies the spatial structure of the small areas, with a unique row and column for each area. For the Australian Cancer Atlas, a first-order adjacency matrix was used, in which two areas were considered to be neighbours if they shared a common geographical boundary. Small areas without any shared boundaries (for example islands) were manually assigned at least one neighbour (usually the closest mainland area).
The column and row number for each area corresponds to the area’s unique identification number (“grid”, as described above). The (i,h)th element of the matrix was 1 if areas i and h share a border, and 0 if i=h or if areas i and h do not share a border. The matrix must be symmetric, that is the (i,h)th element is equal to the (h,i)thelement.
11.4.2.3.3 R code
## Required libraries
library(CARBayes)
## Setting up input data for spatial model
## data$observed is the observed number of females who had cervical screening in a small area.
## data$expect is the expected number of females having cervical screening based
# on the age-specific national rates applied to the age-specific population
# eligible for screening within the same small area.
## data$grid is the identifier for a small area (values 1,.., N, N is the total number
# of small areas) which needs to be the same in the data file and the adjacency matrix file.
file.input =
data.frame(
obs = data$observed,
expect = data$expect,
grid = data$grid)
# Model specification using the CARBayes package with the Leroux prior and a Poisson likelihood
# Default priors were used
formula <- obs ~ offset(log(expect))
MCMC <- S.CARleroux(
formula = formula,
data = file.input,
family = "poisson",
W = W, # The adjacency matrix
burnin = 50000,
n.sample = 150000,
thin = 10)
# Calculate the standardised participation ratio (SPR) for each MCMC iteration
spr.mcmc <-
MCMC$samples$fitted /
matrix(
rep(file.input$expect, nrow(MCMC$samples$fitted)),
byrow = T, ncol = ncol(MCMC$samples$fitted)
)
# Calculate median, credible interval and exceedance probability (EP) for the SPRs
SPR <-
data.frame(
grid = file.input$grid,
median = apply(spr.mcmc, 2, median),
LB = apply(spr.mcmc, 2, quantile, probs = 0.025),
UB = apply(spr.mcmc, 2, quantile, probs = 0.975),
EP = apply(spr.mcmc, 2, function(x) sum(x > 0) / nrow(spr.mcmc))
)
# Calculate median and credible interval for the modelled count estimates
modelled.counts <-
data.frame(
grid = file.input$grid,
median = apply(MCMC$samples$fitted, 2, median),
LB = apply(MCMC$samples$fitted, 2, quantile, probs = 0.025),
UB = apply(MCMC$samples$fitted, 2, quantile, probs = 0.975)
)
Calculating the expected counts
Expected counts of women was calculated as shown below.
Ei, 25−74=(K∑kRateAustraliak ×Eligible Womenik)/100
Expected counts of women in each SA2 (i) between 2018-2022 was calculated by multiplying the age-specific national participation rate (per 100 population) (RateAustraliak) by the age-specific eligible population (Eligible Womenik) for each five-year age group k (20-24, 25-29, 30-34, 35-39, 40-44, 45-49, 50-54, 55-59, 60-64, 65-69, 70-74).
The age-specific national participation rate (RateAustraliak) for each five-year age group k was defined as the ratio of the total number of participants (CountAustralia_k) to the corresponding population of eligible women from 2018-2022. The age-specific eligible population was estimated by multiplying the SA2-specific eligible population by the proportion of eligible women in that age group for all of Australia. Further adjustments were made to account for any potential discrepancies between the total estimated eligible population and the reported eligible population in the data from the Australian Institute of Health and Welfare.
Note that direct comparisons of cervical screening participation between the states and territories of Australia are not advised, due to the substantial differences that exist between the jurisdictions, including population, area, geographical structure, policies, and other factors. (Australian Institute of Health and Welfare, 2023c)