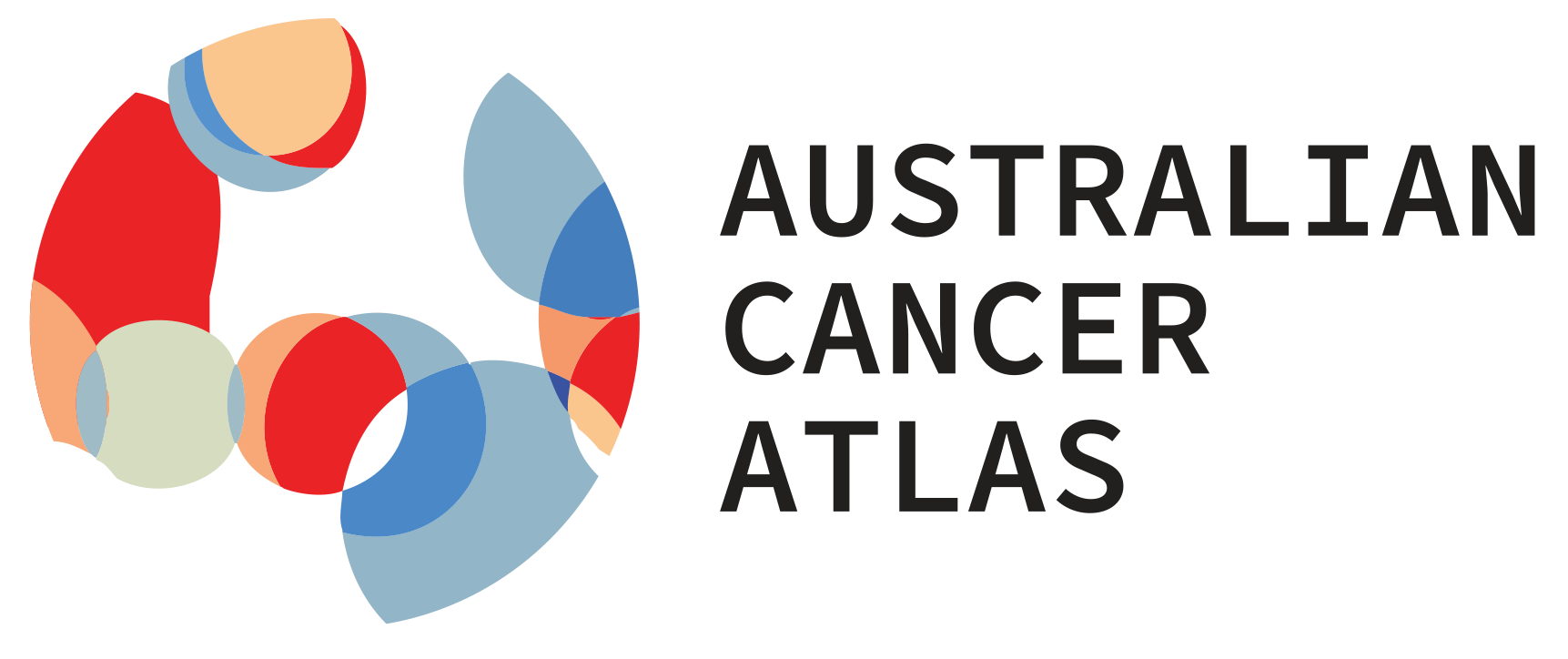
10 Cancer risk factors
10.1 What are the cancer risk factors?
In Australia, at least one in three cancers are attributable to modifiable risk factors. (Whiteman et al., 2015) The following five broad risk factor groups were chosen for the Australian Cancer Atlas: smoking, alcohol, diet, weight and physical activity. These were selected by consulting: a broad range of experts; literature, specifically evidence for casual associations with cancer (World Cancer Research Fund: Cancer risk factors) and population attributable fractions (PAF), (Whiteman et al., 2015) for cancer; and the availability of data in the 2017-18 National Health Survey. (Australian Bureau of Statistics, 2017-18) The PAF is a measure of the number of cancers attributed in a population to a specific risk factor.
A variety of possible measures and corresponding definitions for each of the five broad risk factor groups were explored, placing priority on the definitions and recommendations used in the Social Health Atlas, (Social Health Atlas) the work by Whiteman and colleagues in 2010, (Whiteman et al., 2015) Cancer Council Australia (Risk factors | Cancer Council) and those provided by Australian government agencies such as the National Health and Medical Research Council (NHMRC), (National Health and Medical Research Council, 2013a, National Health and Medical Research Council, 2013b, National Health and Medical Research Council, 2020) the Australian Institute of Health and Welfare (Australian Institute of Health and Welfare, 2019, Australian Institute of Health and Welfare, 2021) and the National Department of Health.
The alcohol, physical activity, and diet variables in the National Health Survey are based on self-reports from the week before the interview; we cannot make any claims regarding how representative of lifetime behaviour these data are.
The Australian Cancer Atlas includes the following eight measures of risk factors (Table 10.1).
Table 10.1 Descriptions and definitions of the included cancer risk factors in Atlas 2.0.
Measure | Measure definition |
---|---|
Currently smoking | Those who reported to be current smokers (including daily, weekly or less than weekly), and had smoked at least 100 cigarettes in their life. These definitions were Consistent with that of the Social Health Atlas. (Social Health Atlas) |
Weight | |
Obese | Those with a measured BMI greater or equal to 30. |
Overweight/obese | Those with a measured BMI greater or equal to 25. |
Risky waist circumference |
Those with a measured waist circumference measurement of ≥94cm (men) and ≥80cm (women) (National Health and Medical Research Council, 2013b, World Cancer Research Fund/American Institute of Cancer Research, 2018, World Health Organization, 2024) |
Alcohol | |
Risky alcohol consumption | Based on self-reported alcohol consumption, those who exceeded the revised 2020 National health and Medical Research Council (NHMRC) guidelines. (National Health and Medical Research Council, 2020) The guidelines stipulate that adults should drink no more than 10 standard drinks a week and no more than 4 standard drinks on any one day. |
Physical activity | |
Inadequate activity (leisure) | Based on self-reported physical activity, those who did not meet the 2014 Department of Health physical activity guidelines, (Physical Activity Guidelines (DOH)) based on their leisure physical activity levels. |
Inadequate activity (all) | Based on self-reported physical activity, those who did not meet the 2014 DOH Physical Activity guidelines, (Physical Activity Guidelines (DOH)) based on their leisure and workplace physical activity levels. |
Diet | |
Inadequate diet | Based on self-reported diet, those who did not meet both the fruit and vegetable 2013 NHMRC Australian Dietary guidelines. (National Health and Medical Research Council, 2013a) |
10.2 Data sources
10.2.1 National Health Survey
The individual level survey data were obtained from the 2017-18 National Health Survey which is an Australia-wide population-level health survey conducted every 3-4 years by the Australian Bureau of Statistics. (Australian Bureau of Statistics, 2017-18) The survey aims to collect a variety of health data on one adult and one child (where possible) in each selected household. Households are selected using a complex multistage design. Trained Australian Bureau of Statistics interviewers conduct personal interviews with selected persons in each of the sampled households.
To allow researchers to accommodate this complex sample design, the Australian Bureau of Statistics provides survey weights, which were used in this analysis. The 2017-18 National Health Survey dataset included 17,248 sampled persons 15 years and older, with 878 persons under the age of 18. The survey provided sampled data for 1,694 SA2s across Australia, with a median and interquartile range SA2-level sample size of 8 and 5 to 13, respectively.
10.2.2 Population
Population data by SA2, five-year age group and year was obtained from the Australian Bureau of Statistics. (Australian Bureau of Statistics, 2022a) SA2-level population counts were derived by averaging across the two years (2017 and 2018).
10.2.3 Geographical areas
Of 2,288 SA2 (2016) areas in Australia that were potentially eligible for analysis after excluding 18 SA2s with no spatial information and four remote islands (see Section 4.1), any SA2 with an average annual population of less than or equal to 10 (n=67) were also excluded. This means the Australian Cancer Atlas provides modelled risk factor estimates for 2,221 SA2s across Australia.
10.3 Statistical methods
10.3.1 National summary measures
National prevalence estimates, i , for each cancer risk factor were derived using individual level survey data from the 2017-2018 National Health Survey. (Australian Bureau of Statistics, 2017-18) The Australian Bureau of Statistics provided survey weights wiq for individual q in SA2 i , which were used in the common Hajek (1971) direct estimator. (Hajek, 1971)
Σiqwiqyiqn
In the direct estimator yiq is the binary outcome for individual q in SA2 i . The formula for the approximate sampling variance of μDi is given in paper by Hogg and colleagues. (Hogg et al., 2023)
In addition to the national prevalence estimates, the estimated number of people with a specific risk factor are also reported.
10.3.2 Small area (SA2) estimates
10.3.2.1 Spatial models
Given that the data on cancer risk factors were available in survey data, methods of small area estimation were used for spatial analysis. (Rao and Molina, 2015) Specifically, we developed a new two-stage Bayesian approach to estimate small area proportions of risk factors for persons only. (Hogg et al., 2023) Given the sparsity of the survey data, estimates could not be reported for females and males separately.
The same two-stage approach was used for all eight cancer risk factors provided in the Australian Cancer Atlas. A brief overview of the approach is provided below with further details in a paper by Hogg and colleagues. (Hogg et al., 2023)
Stage 1: Individual level model
The first stage model is an individual level logistic mixed model:
yiq∼Bernoulli(πiq)
where yiq ε (0,1) is the binary value from the National Health Survey and πiq is the fitted probability that individual q in area i engages in unhealthy behaviour (yiq=1). For example, when modelling current smoking, πiq is the predicted probability that the individual is a current smoker.
The first stage model was completed by defining a linear predictor for logit(πiq). This included both individual level categorical and area level covariates as fixed effects. Complex unstructured individual and SA2 level random effects were also applied. The Bernoulli likelihood was weighted by the sample weights to ensure the predictions were approximately unbiased under the sample design. (Parker et al., 2019) An age by sex interaction was included in the first stage model, so the predicted probabilities were adjusted for age and sex.
All fixed effects were given N(0,22) prior distributions and a student-t(0,22,df=3) prior distribution was used for the intercept. Given the model’s complexity, N+(0,12) prior distributions were used for the standard deviation of the random effects.
Stage 2: Area level model
After fitting the first stage model, the fitted probabilities, πiq, were used to derive the SA2 level stage 1 proportion estimates, μS1i, and sampling variances, ψS1i. The Hajek estimator and its corresponding sampling variance estimator were used to derive these quantities using each posterior draw of πiq; thus, approximating the posterior distribution.
The stage 1 estimates were only available for sampled SA2s. The sample size and population of each sampled SA2 was accommodated into the model via the sampling variances.
To enable the use of a Gaussian distribution, the S1 estimates, and sampling variances were logit transformed, producing θS1i and τS1i, respectively. To propagate the uncertainty of the first stage model to the second stage model, a subset of the posterior draws of θS1i were fed as input to the stage 2 model. Thus, the stage 2 model likelihood was:
θS1i∼N(logit(μi),σ2i)
where σ2i captured both the S1 sampling variance, τS1i, and the posterior uncertainty in θS1i. The final proportion estimate for area i was the posterior distribution of μi.
The second stage model was complete by defining a linear predictor for logit (μi), which used several unique components. It incorporated deciles of the Index of Relative Socio-Economic Disadvantage (Australian Bureau of Statistics, 2018b) and remoteness as fixed effects. Furthermore, it used six principal components as fixed effects, with their coefficients varying based on remoteness. These principal components were derived from 84 demographic variables from the 2016 census. (Australian Bureau of Statistics, 2016b)
In addition, primary health network (Department of Health and Aged Care, 2023) level risk factor prevalence estimates from the Social Health Atlas were included as covariates. To provide smoothing and accommodate spatial dependence, a BYM2 spatial random effect (Riebler et al., 2016) was included at the SA2 level. An unstructured random effect was also included at the Statistical Area 3 (SA3) level.
All fixed effects were given N(0,22) prior distributions, a student-t(0,22,df=3) prior distribution was used for the intercept and a N+(0,22) prior for the standard deviation of the random effects. The mixing parameter in the BYM2 random effect was given a Uniform (0,1) prior.
Validation
The small area estimates were validated using both internal and external methods. Internal validation involved a fully Bayesian benchmarking procedure. (Zhang and Bryant, 2020) Benchmarking was used to ensure a level of agreement between direct and modelled estimates at a high geographical aggregation, where the 2017-18 National Health Survey was designed to provide reliable estimates. By applying a fully Bayesian benchmarking approach, the uncertainty in the final proportion estimates reflected the additional information introduced with benchmarking.
Two benchmarks were enforced: “state” and “major-by-state”. The “state” benchmark had seven groups which were composed of the states and territories of Australia (except the Northern Territory). The “major-by-state” benchmark had 12 groups, composed of the interaction of the states and territories of Australia (except the Northern Territory) and dichotomous remoteness (major city versus non-major city).
External validation was performed by comparing the results to those from the Social Health Atlas and the overall trends from other Australian health surveys conducted on specific sub-populations (e.g., states).
10.3.2.2 Reported estimates
The key output from the two-stage model that was used in the Australian Cancer Atlas was the posterior median of the relative ratios, which were derived for each posterior draw of μi by dividing by the national prevalence estimate. Relative ratios indicated whether the prevalence estimate for a SA2 was higher or lower than the national prevalence estimate.
In addition, the Atlas reported the modelled number of people with a specific risk factor (absolute measures) which was the posterior median of multiplying the posterior draws of μi by the corresponding SA2 population.
10.3.2.3 Computation of risk factor models
Bayesian spatial models were fitted using the R package rstan Version 2.26.11. (R Interface to Stan) The stan code for the stage 1 and stage 2 models can be found on GitHub. (Jamie Hogg-ACA riskfactors)
The stage 1 models had a burn-in of 1,000 iterations and were then monitored another 1,000 iterations for each of four chains. A random subset of 500 iterations were then fed from the stage 1 to the stage 2 model. Each of the MCMC chains for the stage 2 model (4 chains) had a burn-in of 3,000 iterations followed by an additional 3,000 iterations, keeping every second iteration, resulting in 6, 000 total iterations.
10.3.3 Confidence (‘uncertainty’) of modelled estimates
The confidence of the modelled estimates accommodated the sample design, spatial autocorrelation, benchmarking, and sparsity of the given risk factors. The uncertainty of the estimates was captured via the dispersion of the approximate posterior distributions for each area’s relative ratio and modelled population count.