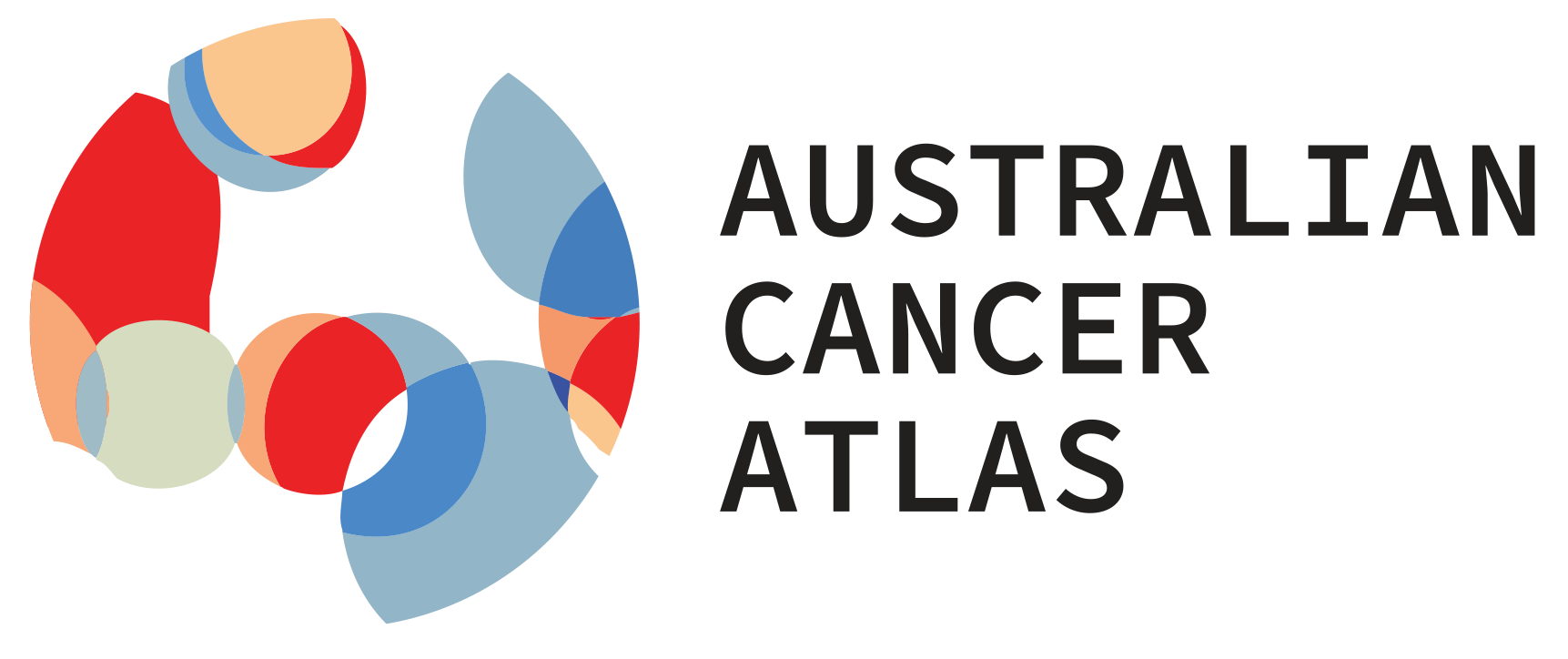
12 Hospital Treatments
The Australian Cancer Atlas report geographical patterns for three hospital treatments for prostate cancer.
12.1 What are the prostate cancer hospital treatment types?
The Australian Cancer Atlas includes details about three different interventional treatments for prostate cancer among men admitted to Australian hospitals: radical prostatectomy, low dose rate brachytherapy and high dose rate brachytherapy. It is noted that there are major treatments for prostate cancer that are not included, such as external beam radiotherapy, but population-based data across Australia on these other types of treatment are not currently available. This is a priority for further work.
There is no single “best” treatment for prostate cancer. (Litwin and Tan, 2017) Management options include interventional treatments (such as surgery and radiation) and active surveillance (involves monitoring for disease progression while not undergoing interventional treatments). Treatment decisions depend on many factors including clinical features, patient age, general health, socio-demographics, and preferences. (Baade et al., 2012, Dasgupta et al., 2019)
Radical prostatectomy is a surgical procedure involving complete or partial removal of the prostate. It is the most common treatment for localised or locally advanced prostate cancer. Brachytherapy is a type of radiotherapy that involves implanting a sealed radioactive source near or inside the cancer. Radiation is released targeting the cancer cells with minimal exposure to the surrounding tissues and organs. (Cancer Council Australia, 2021) High dose rate brachytherapy releases radiation at a high dose for a few minutes at multiple sessions. In contrast, low dose rate brachytherapy releases radiation at a lower dose for a prolonged time (over 1-6 days).
12.2 Data sources
12.2.1 Hospital Treatment data
The National Hospital Morbidity Database (NHMD) held by the Australian Institute of Health and Welfare contains information on each episode of care for admitted patients in all Australian hospitals. The data were obtained as “separations”, or the number of episodes of care (Infobite-what-is-a-separation). Counts of separations were obtained for males aged 40 years and older who had any of the three hospital treatments. Separations from both public and private hospitals were included.
The separations were aggregated by type of hospital treatment using the Australian Classification of Health Intervention (ICD-10-AM/ACHI sixth edition) refined Diagnosis Related Groups codes (Table 12.1). Records were excluded if age or sex were not reported, if the care type was “newborn”, for posthumous organ procurement and for hospital boarders.
Table 12.1 Hospital Treatment types and codes included in Atlas 2.0.
Treatment | ICD-10-AM/ACHI codes |
---|---|
High dose rate brachytherapy | 15327-01, 15327-03, 15327-06, 15327-07, 15536-00, 15536-01, 15536-02 |
Low dose rate brachytherapy | 15327-00, 15327-02, 15327-04, 15327-05, 15338-00 |
Radical prostatectomy | 37209-00, 37210-00, 37211-00, 37209-01, 37210-01, 37211-01 |
Data were provided aggregated by procedure code, 5-year age group, financial year (defined as July 1 to June 30) and area of residence at time of hospital treatment.
12.2.2 Population data
Population data by SA2 (2016 ASGS), calendar year and 5-year age groups for males was obtained from the Australian Bureau of Statistics. (Australian Bureau of Statistics, 2022a)
12.2.3 Geographical data
There were 2,288 SA2 areas in Australia, after 18 non-spatial SA2s and four remote islands were excluded from the analysis. A further 74 were excluded as the average annual male population aged 40 or over was less than five. Hence the Australian Cancer Atlas provides modelled estimates for hospital treatment patterns for 2,214 SA2s across Australia.
Residential location at time of hospital treatment was provided by Statistical Local Area (SLA) for financial years 2001-02 to 2011-12 and, by 2011 SA2 for 2012-13 to 2016-17. Residential location was probabilistically reallocated to 2016 SA2s using population-weighted correspondence files. (Australian Bureau of Statistics Geospatial Solutions, 2023) Finally the counts were reallocated from financial to calendar year proportionately to population size, so that data were available between 2002 and 2016.
12.3 Statistical methods
12.3.1 National summary measures
The reported national summary measures were the total number of males aged 40 years and older and the percentage of the corresponding population who had each hospital treatment.
12.3.2 Spatial models
Geographical patterns for each hospital treatment are presented using indirectly age standardised separation rate ratios (SSRs) which is the ratio of the observed versus expected counts of corresponding separations from 2007 to 2016.
Following a process of model testing and evaluation, (Cameron et al., 2023) the final models were Bayesian spatial models with the Leroux prior. (Leroux et al., 2000)
For each hospital treatment, the aggregated observed counts of separations from 2007 to 2016 was modelled separately, offset by the age-adjusted corresponding expected counts. The model applied a Poisson distribution with an offset of the logged expected counts in each small area. Expected counts were based on national age-specific rates. The model gave a set of spatially smoothed area-level SSRs relative to the national average.
The first stage is the likelihood model for the observed count data, that is, observed count of separations \(Y_{i}\). in area \(i\):
\[Y_{i}\ \sim\ Poisson(E_{i}\lambda_{i})for\ i = 1\ldots\ldots\ldots,\ N\ areas\]
where \(E_{i}\) is the expected count. Expected counts was calculated for each area \(i\), using the Australian total count of separations and population for each five-year age group (𝑘) and the population in the SA2 at each age group.
\[E_{i} = \sum_{age\ group = k}^{K}{\frac{Total\ counts\ in\ Australia\ in\ the\ age\ group}{Australian\ male\ population\ in\ the\ age\ group}SA2\ population\ for\ the\ age\ group}\]
This used data aggregated over 10 years (2007-2016).
Modelled estimates are effectively age-adjusted since \(E_{i}\) considers the age structure and population size.
The second stage comprises an expression for the SSR (𝜆𝑖), which is the key output used in the Australian Cancer Atlas.
\[log(\lambda_{i}) = \ \beta + S_{i}\]
The parameter \(\beta\) is the intercept, while \(\text{S}_{i}\) represents the spatial random effect for area i. The specification of these spatial random effects determines how the smoothing is performed, and together with the specification of the intercept parameter, forms the third stage of the model in the form of prior distributions.
The prior for the intercept was a weakly informative Gaussian distribution with zero mean.
\[\beta\ \sim\ N(0,100000)\]
The spatial random effects \(\text{S}_{i}\)were modelled by the Leroux prior, (Leroux et al., 2000) which is a Gaussian distribution and its mean is the weighted average of the neighbouring areas’ random effects (with weight \(\rho\)) and a global mean of 0 (with weight \(1 - \ \rho\)).
\[S_{i}|S_{\backslash i}\ \sim\ N\left( \frac{\rho\Sigma_{h}w_{ih}S_{h}}{\rho\Sigma_{h}w_{ih} + 1 - \rho},\ \frac{\sigma_{S}^{2}}{\rho\Sigma_{h}w_{ih} + 1 - \rho} \right)\]
The spatial weights \(w_{ih}\) represent the spatial proximity between areas \(i\) and \(h\), and collectively they comprise an N×N spatial adjacency matrix W. A binary weighting scheme was used, so that \(w_{ih} = 1\) if areas \(i\) and \(h\) were considered to be neighbours, and otherwise \(w_{ih} = 0\).
To complete the Bayesian model specification, the variance \(\sigma_{S}^{2}\) was also given a weakly informative prior:
\[\sigma_{S}^{2}\ \sim\ InverseGamma(1,\ 0.01)\]
which is an inverse-gamma distribution with parameters shape and scale. The prior for the spatial smoothing parameter \(\rho\) was weakly informative.
\[\rho\ \sim\ Uniform(0,1)\]
The Australian Cancer Atlas reports the modelled SSR and estimated modelled counts for the three prostate cancer interventional hospital treatments by SA2s across Australia from 2018 to 2022.
12.3.2.1 Bayesian spatial model for treatment
12.3.2.1.1 Input data set
Key variables in this data set are:
Variable | Value |
---|---|
grid | A unique identification number for each small area. The numbers should be consecutive values from 1 to N, where N is the total number of areas |
observed | Observed count of separations for a treatment |
expect | Expected count of separations for a treatment |
The data should be sorted by grid. Expected counts should be greater than zero.
12.3.2.1.2 Adjacency matrix
An adjacency matrix specifies the spatial structure of the small areas, with a unique row and column for each area. For the Australian Cancer Atlas, a first-order adjacency matrix was used, in which two areas were considered to be neighbours if they shared a common geographical boundary. Small areas without any shared boundaries (for example islands) were manually assigned at least one neighbour (usually the closest mainland area).
The column and row number for each area corresponds to the area’s unique identification number (“grid”, as described above). The \((i,{h)}^{th}\) element of the matrix was 1 if areas \(i\) and \(h\) share a border, and 0 if \(i = h\) or if areas \(i\) and \(h\) do not share a border. The matrix must be symmetric, that is the \((i,{h)}^{th}\) element is equal to the \(({h,i)}^{th}\)element.
12.3.2.1.3 R code
## Required libraries
library(CARBayes)
## Setting up input data for spatial model
## data$observed is the observed number of separations for a treatment in a small area.
## data$expect is the expected number of separations for the same treatment based on the
# age-specific national rates applied to the age-specific population eligible for treatment within a small area.
## data$grid is the identifier for a small area (values 1,.., N, N is the total number
# of small areas) which needs to be the same in the data file and the adjacency matrix file
file.input =
data.frame(
obs = data$observed,
expect = data$expect,
grid = data$grid
)
# Model specification using the CARBayes package with the Leroux prior and a Poisson likelihood
# Default priors were used
formula <- obs ~ offset(log(expect))
MCMC <- S.CARleroux(
formula = formula,
data = file.input,
family = "poisson",
W = W, # The adjacency matrix
burnin = 50000,
n.sample = 150000,
thin = 10)
# Calculate the standardised separation rate ratio (SSR) for each MCMC iteration
spr.mcmc <-
MCMC$samples$fitted /
matrix(
rep(file.input$expect, nrow(MCMC$samples$fitted)),
byrow = T, ncol = ncol(MCMC$samples$fitted)
)
# Calculate median, credible interval and exceedance probability (EP) for the SSRs
SSR <-
data.frame(
grid = file.input$grid,
median = apply(ssr.mcmc, 2, median),
LB = apply(ssr.mcmc, 2, quantile, probs = 0.025),
UB = apply(ssr.mcmc, 2, quantile, probs = 0.975),
EP = apply(ssr.mcmc, 2, function(x) sum(x > 0) / nrow(ssr.mcmc))
)
# Calculate median and credible interval for the modelled count estimates
modelled.counts <-
data.frame(
grid = file.input$grid,
median = apply(MCMC$samples$fitted, 2, median),
LB = apply(MCMC$samples$fitted, 2, quantile, probs = 0.025),
UB = apply(MCMC$samples$fitted, 2, quantile, probs = 0.975)
)
12.3.3 Spatiotemporal models
After an exhaustive investigation of different types of spatiotemporal models, the model of Bernardinelli and colleagues (Bernardinelli et al., 1995) was adapted for the Australian context. In the first stage of the model, the observed number of separations for a given type of hospital treatment (\(Y_{it}\)) was modelled on the expected counts (\(E_{it}\)) and the standardised separation rate ratio (\(\lambda_{it}\)) for each area \(i\) and time \(t\).
\[Y_{it}\sim\ Poisson\left( E_{it}\lambda_{it} \right)\ \ \ \ for\ i = 1,\ldots,\ 2214\ areas\ and\ t = 1,\ldots,T\ time\ points\]
The expected number of separations were calculated using the age distribution of the area at each time and the age-specific rates of separations. The age-specific rates were calculated differently for the different types of estimates, as described below, and separate models were fitted for each.
The standardised separation rate ratios (\(\lambda_{it}\)) are the key output visualised in the Atlas maps. These were calculated using the modelled counts (\({\widehat{Y}}_{it}\)) and the expected counts.
\[\lambda_{it} = {\widehat{Y}}_{it}/E_{it}\]
The second stage of the model describes how the standardised separation rate ratios vary over time and space using a national temporal trend (\(f(t)\)), spatial effects (\(R_{i}\)) and area-specific deviations from the national trend (\(f_{i}(t)\)).
\[\log\left( \lambda_{it} \right) = \ f(t) + f_{i}(t) + R_{i}\]
Spatial effects
The spatial effects were modelled by a BYM2 prior, (Riebler et al., 2016) which is a weighted average between a spatially structured effect (\(\phi_{i}\)) and a Gaussian effect with zero mean (\(\theta_{i}\)):
\[\theta_{i}\ \sim\ N(0,\ 1)\]
\[\phi_{i}|\phi_{\backslash i}\ \sim\ N\left( \frac{\sum_{h}^{}{w_{ih}\phi_{h}}}{\sum_{h}^{}w_{ih}},\ \frac{1}{\sum_{h}^{}w_{ih}} \right)\]
\[R_{i} = \frac{1}{\sqrt{\tau_{R}}}\left( \theta_{i}\sqrt{1 - \rho} + \ \phi_{i}\sqrt{\rho/\iota} \right)\]
where \(\iota\) is a scaling factor calculated from the adjacency matrix, W, which is comprised of the spatial weights \(w_{ih}\). These weights represent the spatial proximity between areas \(i\) and \(h\). A binary weighting scheme was used, so that \(w_{ih} = 1\) if areas \(i\) and \(h\) were considered neighbours and \(w_{ih} = 0\) otherwise.
Temporal effects
The national trend and the area deviations from the national trend were modelled using basis splines. Splines are a set of simple lines or curves that are added together, resulting in a versatile range of possible curves. You can explore splines in the app below. Basis splines (Wang and Yan, 2021) were found to provide the best model fit according to the Moran’s ST of the residuals. (Anderson and Ryan, 2017)
The national temporal trend was modelled by an intercept (\(\beta\)) and a set of spline coefficients (\(b_{\kappa}\) for each basis \(\kappa = 1,\ldots,Κ\)), as follows:
\[f(t) = \beta + \sum_{\kappa = 1}^{Κ}{b_{\kappa}Z_{\kappa,t}}\]
where \(X_{t}\) is the time point (normalised) and \(Z_{\kappa,\ t}\) is the spline for the \(\kappa\)th basis at time point \(t\).
The deviation for area from the national trend was defined by the splines alone:
\[f_{i}(t) = \ \sum_{\kappa = 1}^{Κ}{d_{i,\kappa}Z_{\kappa,t}}\]
For each type of hospital treatment, multiple models were fitted with differing numbers of knots to identify the best fitting model. Initially, multiple numbers of knots were fitted to the national data using a temporal model (as above, but without \(R_{i}\) or \(f_{i}(t)\)) and the optimal number of knots (and the corresponding number of bases, \(Κ_{opt}\)), for each type of hospital treatment was identified using the WAIC. (Watanabe, 2010) A set of candidate numbers of bases for each type of hospital treatment was then defined for the spatiotemporal models as \(\left\{ 1,\ 2,\ \ Κ_{opt} - 1,\ Κ_{opt} \right\}\). After the spatiotemporal models were fitted using this set of candidates, the best number of knots fitted to each type of hospital treatment was selected using WAIC, Moran’s ST of the residuals and other model fit considerations.
Other priors and hyperpriors
The intercepts and coefficients were given Gaussian priors, each with unique precisions. For example, \(\beta_{0}\) and \(\beta_{1}\) were independently sampled, as shown below.
\[\beta_{0},\ \beta_{1}\ \sim\ N(0,\ \tau_{\beta})\]
\[\tau_{\beta}\ \sim\ Gamma(1,\ 0.01)\]
Similarly, the precision on the spatial random effects also had a gamma prior.
\[\tau_{R}\ \sim\ Gamma(1,\ 0.01)\]
The mixing parameter was given a flat beta prior.
\[\rho\ \sim\ beta(1,\ 1)\]
Calculating the expected counts
For “Changes over time for each geographic area”, the age-specific rates (\(r_{k}\)) were calculated using data aggregated across the entire period, as shown below.
\[r_{k} = \frac{number\ of\ separations\ in\ Australia\ in\ age\ group\ k}{number\ of\ people\ in\ Australia\ in\ age\ group\ k}\]
The expected counts used the \(r_{k}\) and the population residing in area \(i\) at time \(t\) in age group \(k\):
\[E_{it} = \ \sum_{k = 1}^{15}{r_{k}\ \times \ (number\ of\ people\ in\ area\ i\ at\ time\ t\ in\ age\ group\ k})\]
where \(k\) = 1 represents ages 15-19 and \(k\) = 15 represents ages 85+.
For “Geographical patterns (separate time periods)”, the age-specific rates (\(r_{kt}\)) were calculated for each time point, as shown below.
\[r_{kt} = \frac{number\ of\ separations\ in\ Australia\ in\ age\ group\ k\ at\ time\ t}{number\ of\ people\ in\ Australia\ in\ age\ group\ k\ at\ time\ t}\]
\[E_{it} = \ \sum_{k = 1}^{15}{r_{kt}\ \times \ (number\ of\ people\ in\ area\ i\ at\ time\ t\ in\ age\ group\ k})\]
12.3.3.1 Bayesian spatiotemporal model for treatment
12.3.3.1.1 Input data set
Each row of the input data set represented a unique time point and small area.
Variable | Value |
---|---|
idarea | A unique identification number for each small area. The numbers should be consecutive values from 1 to N, where N is the total number of areas |
yeargrp | The time point (year or year group) |
observed | Observed count of separations for a treatment |
expect | Expected count of separations for a treatment |
The expected count should be greater than zero. Because area-level population sizes change over time, there were some areas with an expected count of zero. The expected counts for these areas were set to a nominal value of 0.001 times the smallest non-zero expected count in the data set, as shown in the R code below.
12.3.3.1.2 Adjacency matrix
An adjacency matrix specifies the spatial structure of the small areas, with a unique row and column for each area. For the Australian Cancer Atlas, a first-order adjacency matrix was used, in which two areas were considered neighbours if they shared a common geographical boundary. Small areas without any shared boundaries (for example islands) were manually assigned at least one neighbour (usually the closest mainland area).
The column and row number for each area corresponds to the area’s unique identification number (“grid”, as described above). The \((i,{h)}^{th}\) element of the matrix was 1 if areas \(i\) and \(h\) share a border, and 0 if \(i = h\) or if areas \(i\) and \(h\) do not share a border. The matrix must be symmetric, that is the \((i,{h)}^{th}\) element is equal to the \(({h,i)}^{th}\)element.
12.3.3.1.3 R code
library(nimble)
library(splines2)
# W is the adjacency matrix
## Setting up input data for spatiotemporal model
# The input data, 'dat.in' are as described in the above table, where
# idarea is the unique area ID and corresponds to the area's row / column number in W
# yeargrp is the time point
# observed is the observed number of separations for that treatment, area, and time point
# expect is the expected number of separations for that treatment, area, and time point
# num.knots is the number of knots to be fitted. This code assumes that num.knots > 1
# The number of areas (from the adjacency matrix)
N = ncol(W)
# Obtain scaling factor for BYM2 from adjacency matrix
# ICAR precision matrix
Q = apply(W, 1, sum) * diag(N) - W
# Add a small jitter for numerical stability
Q_pert <- Q + diag(N) * max(Matrix::diag(Q)) * sqrt(.Machine$double.eps)
# Compute the diagonal elements of the covariance matrix subject to the
# constraint that the entries of the ICAR sum to zero
Q_inv <- function(Q){
Sigma <- Matrix::solve(Q)
A <- matrix(1,1, NROW(Sigma))
W <- Sigma %*% t(A)
Sigma <- Sigma - W %*% solve(A %*% W) %*% Matrix::t(W)
return(Sigma)
}
Q_inv <- .Q_inv(Q_pert)
# Compute the geometric mean of the variances (diagonal of Q_inv)
scaling_factor <- exp(mean(log(Matrix::diag(Q_inv))))
# Obtain adjacency information
adj.info <- as.carAdjacency(W)
L = length(adj.info$adj)
# Correct the expected counts so all are greater than zero
if (any(dat.in$expect == 0)) dat.in$expect[dat.in$expect == 0] = min(dat.in$expect[dat.in$expect != 0]) / 1000
# Get standardised time variable
time.ids <- sort(unique(dat.in$yeargrp))
dat.in$idtime <- match(dat.in$yeargrp, time.ids)
X = (dat.in$idtime - mean(dat.in$idtime)) / sd(dat.in$idtime)
## National spline
inc = 1/(num.knots+1)
knots <- quantile(X, probs = seq(inc, 1-inc, inc))
# creating the B-splines
Z <- bspline(X,
knots = knots, degree = 3,
Intercept = FALSE)
K <- ncol(Z)
## Area spline
num.knots_area_dev == 2
inc = 1/(num.knots_area_dev+1)
knots <- quantile(X, probs = seq(inc, 1-inc, inc))
# creating the B-splines
Z_area_dev <- bspline(X,
knots = knots, degree = 3,
Intercept = FALSE)
K_area_dev <- ncol(Z_area_dev)
constants <- list(n = nrow(dat.in),
expect = dat.in$expect,
Z = Z, Z_area_dev = Z_area_dev,
K = K, K_area_dev = K_area_dev,
Area = dat.in$idarea,
nAreas = N, scaling.factor = scaling.factor,
adj = adj.info$adj, weights = adj.info$weights, num = adj.info$num, L=L
)
# Initial values
inits <- list(
beta = rnorm(1),
b = rnorm(K),
d = matrix(rnorm(K_area_dev*N), ncol = K_area_dev),
theta = rnorm(N),
phi = rnorm(N),
taub = rgamma(1,1,0.1),
tau.u = rgamma(1,1,0.1),
taud = rgamma(1,1,0.1),
taubeta = rgamma(1,1,0.1),
rho = rbeta(1,1,1)
)
# Set initial values for d to sum to zero for all knots, k
for (k in 1:ncol(inits$d)) inits$d[,k] = inits$d[,k] - mean(inits$d[,k])
source(nimble.model.code)
# Parameters to monitor in Nimble
parameters2 <- grep("^delta|^d$|theta|phi", names(inits), value = T)
parameters <- c(setdiff(names(inits), parameters2), "mu")
Rmodel <- nimbleModel(model.code,
data = list(obs = dat.in$observed),
inits = inits,
constants = constants
)
mcmcConf <- configureMCMC(Rmodel,
monitors = parameters,
monitors2 = parameters2
)
# Place block sampler on national trend parameters
tmp = c(paste0("b[", 1:num.knots, "]"), "beta")
mcmcConf$removeSamplers(tmp)
mcmcConf$removeSamplers(tmp)
mcmcConf$addSampler(target = tmp,
type = "RW_block"
)
Rmcmc <- buildMCMC(mcmcConf, nchains = 1)
Cmodel <- compileNimble(Rmodel)
Cmcmc <- compileNimble(Rmcmc, project = Rmodel)
mcmc.out <- runMCMC(Cmcmc,
niter = 100000,
nburnin = 50000,
thin = 5,
thin2 = 50
)
12.3.3.1.4 Nimble code
library(nimble)
model.code <- nimbleCode({
for (idx in 1:n) {
obs[idx] ~ dpois(mu[idx])
log(mu[idx]) <- log(expect[idx]) +
delta[Area[idx]] +
beta+
b[1] * Z[idx,1] +
b[2] * Z[idx,2] +
b[3] * Z[idx,3] +
b[4] * Z[idx,4] +
b[5] * Z[idx,5] +
d[Area[idx],1] * Z[idx, 1] +
d[Area[idx],2] * Z[idx,2]
d[Area[idx],3] * Z[idx,3]
d[Area[idx],4] * Z[idx,4]
d[Area[idx],5] * Z[idx,5]
}
# Priors for the national temporal curve
for (k in 1:num.knots) { b[k] ~ dnorm(0, taub) }
beta ~ dnorm(0, taubeta)
# Prior for the spline coefficients for the areal deviations from the national temporal curve
for (i in 1:nAreas) {
for (k in 1:K_area_dev) {
d[i,k] ~ dnorm(0, taud)
}
}
# Spatial effects
for (i in 1:nAreas) {
delta[i] <- (1/sqrt(tau.u))*(sqrt((1-rho))*theta[i] + sqrt(rho/scaling.factor)*phi[i])
theta[i] ~ dnorm(0, tau = 1)
}
phi[1:nAreas] ~ dcar_normal(adj[1:L], weights[1:L], num[1:nAreas], tau = 1, zero_mean = 1)
# Priors on precision parameters
taub ~ dgamma(1, 0.01)
taubeta ~ dgamma(1, 0.01)
taud ~ dgamma(1, 0.01)
# BYM2 hyperpriors
tau.u ~ dgamma(1, 0.01)
rho ~ dbeta(1, 1)
})