3 What sort of a science is Ecology?
How to cite this chapter
Elliott-Graves A. (2023) What sort of a science is ecology. In: Cousens R. D. (ed.) Effective ecology: seeking success in a hard science. CRC Press, Taylor & Francis, Milton Park, United Kingdom, pp. 33-48. Doi:10.1201/9781003314332-3
Abstract Ecologists have frequently questioned the concepts, methods and philosophical foundations of their science, often finding them wanting. In this chapter we show that these misgivings stem from a picture of science based on philosophical accounts that were popular for most of the 20th century. However, this picture is outdated, as it does not accurately describe all scientific practice, especially in sciences such as ecology that deal with complex systems. We outline some ways in which ecological systems are complex, including system heterogeneity and the entanglement of social and ethical values, and show how this affects ecological research, for example by creating difficulties for generalisations, predictions and interventions. We then provide an alternative account of science based on newer philosophical views. Rather than dismissing Ecology as an immature science, we argue that it is a challenging but ultimately rewarding science.
3.1 Ecology as a science
As the science of the struggle for existence (Cooper 2003) developed and matured into what we now recognise as Ecology, a number of new struggles emerged, this time for its practitioners rather than the organisms they studied. These struggles have plagued the discipline since its beginnings, threatening the value of its concepts and methods (Kingsland 1995). They have even cast doubt on its status as a science (see discussion in Elliott-Graves 2019).
Ecology does not fit neatly into the traditional picture of what a science ought to look like. Laws are hard to find and tend to have too many exceptions to be useful (Elliott-Graves 2019). Predictions often turn out to be inaccurate, even when established explanations of the same phenomena already exist and theoretical results often resist practical application (Shrader-Frechette and McCoy 1994). Yet the aim of this chapter is not to chastise the discipline for its failings to address these problems, rather it is to show that the struggles faced by ecologists are to a large extent due to the special type of complexity of the systems they investigate. This means that we should not expect ecological research to proceed along the lines laid out by 20th-century philosophers of science, who based most of their ideas on certain sub-disciplines of physics and chemistry (Mitchell 2000).
Of course, the existence of these problems does not mean that we should cease testing the quality of ecological research or stop striving to increase the value and usefulness of ecological concepts and methods. It simply means that the traditional philosophical framework for evaluating scientific practice will doom Ecology to failure.
This chapter highlights some key aspects of this traditional scientific philosophical framework, explains why they cannot apply to ecological research and suggests some ways to modify this picture so that it applies more fairly - but also more usefully - to ecological research. The chapter begins by outlining the way in which ecological systems are complex and then examines two ways in which complexity affects ecological research: in terms of ecologists’ ability to generate generalisations, explanations, predictions and interventions, and in terms of the role of values in ecological research. It then shows how the traditional picture of philosophy of science fails to account for the reality in Ecology; and it concludes by suggesting some ways in which these struggles can be overcome, based on existing methods already in use by ecologists.
What sort of a science is Ecology? It is a complex, challenging but ultimately rewarding science. Moreover, the ways in which it diverges from other sciences and challenges our expectations regarding scientific practice ought not to be a source of embarrassment but an opportunity to learn and revise our theoretical frameworks about scientific practice.
3.2 Ecological systems are complex
Discussions of complexity are notoriously thorny in most disciplines (Hooker 2011). The same is true in Ecology. On the one hand, there seems to be general agreement that ecological systems are quintessential examples of complex systems (Levin 1998). There seems to be a consensus that complexity is a feature of healthy and mature ecological systems, even though such systems may be susceptible to particular disturbances (Loreau et al. 2001). On the other hand, there is no single, uncontested definition of complexity in ecology (Elliott-Graves, 2023).
One characteristic that is typically associated with complexity – both within and beyond ecology – is having multiple parts (Levins 1966), though it is rarely seen as the only relevant characteristic of complexity.
The parts of an ecosystem are not just many, they also interact (Pimm 1991). Specifically, complex ecological systems display high levels of connectance (the number of interspecific interactions out of those possible) and interaction strength (the average value of the interspecific interactions in the community) (Odenbaugh 2011). Other characteristics include non-linearity or feedback, where a change in one factor can result in a disproportional change – or a complete flip - in the behaviour of the system, and path dependence, which occurs when the later states of systems depend on their previous states (Levin 1998). For example, in the colonisation of new areas, such as islands or forest patches, the final composition of the system – which species persist and in what proportion – depends on which species are the original colonisers and how they interacted with each other.
Some characteristics of complexity are important because they lead to methodological and epistemic challenges. That is, they make it difficult for ecologists to conduct scientific investigations and limit the quality or scope of the knowledge ecologists gain from these investigations (see The Challenges of Complexity, this chapter and Figure 3.1). Specifically, in the case of ecological systems, these characteristics are the existence of multiple, interacting, and diverse parts (Elliott-Graves,2023). Ecological systems are made up of a number of heterogeneous individuals of various species in variable environments, which interact with each other and with their environment in diverse and dynamic ways (Levins 1966, 1993). It is important to note that systems, in principle, can have multiple interacting parts but be homogeneous. For example, a chainmail armour suit is made up of many interacting metal rings, yet each of these rings is almost identical to the rest. It is also possible for a system to have multiple diverse parts but be homogeneous across other systems of the same type. For example, a Boeing 747 is made up of many diverse interacting parts, yet each Boeing 747 is very similar to the rest. Thus, despite its complexity, once knowledge from one Boeing 747 is acquired it can be applied to other airplanes of the same type: indeed, air safety relies on this (Elliott-Graves 2018; Matthewson 2011). In contrast, ecological systems score highly on all three characteristics: they have multiple, interacting parts, and there is great variability both within and across ecosystems. For instance, a forest and a marine ecosystem may have similar trophic levels, but they are made up of different types of species. Even two ecosystems of the same ‘type’ such as two grasslands often have different species and/or display different dynamics and interactions (Figure 3.1).
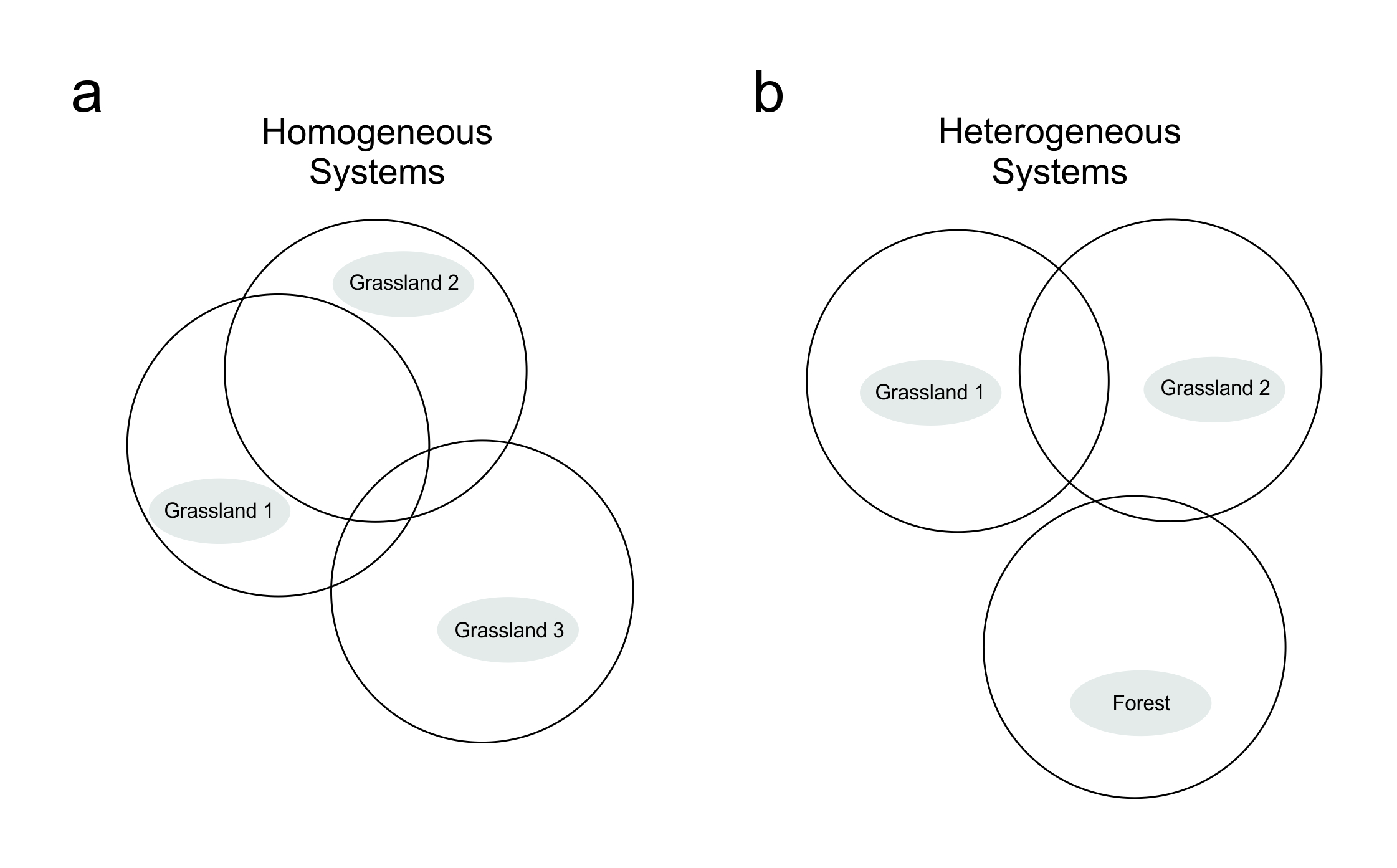
Figure 3.1 How complexity affects generalisations. In homogeneous systems (a), there are factors that are common between systems. In this example, all three grassland systems may share a certain species or dynamic, such as positive plant-soil feedback. Knowledge gained from one system may be transferred to the other systems. In heterogeneous systems (b), there are no factors common across all systems. Generalisations are limited: knowledge gained from one system does not necessarily apply to all the others – though it might apply to some.
3.3 The challenges of complexity
How do these characteristics of complexity affect ecological practice? The short answer is that they make it difficult to acquire and transfer empirical knowledge within and between ecological systems. More specifically, they create difficulties for making generalisations, explanations, predictions and interventions.
3.3.1 Generalisations
Being able to generalise beyond the results of a scientific investigation can be extremely useful. At a basic level, generalisations are practically advantageous, since they can help scientists gain insights that go beyond their current investigation. So, we can generalise the results of an experiment (where a particular phenomenon is in a sample of the population) to the larger population or maybe even the whole species. But generalisations are also useful in a deeper sense. Identifying a pattern in nature reveals information about the world. The idea is that a pattern encompasses what is common across different phenomena or systems. These common factors are thought to be the ‘real causal factors’ of the system that actually give rise to its behaviour and dynamics. In contrast, the factors that differ across systems – those that are ‘idiosyncratic’ – are thought to be ‘mere details’, or noise, in the sense that they do not contribute significantly to the dynamics or behaviour of the system. In other words, successful generalisations provide scientists with knowledge about how the world really is (Strevens 2004). This, in turn, can help scientists explain how a system or phenomenon works, as it allows scientists to ‘virtually reduce’ the complexity of a system, which makes the system easier to study. Omitting the noisy details from a system means that there are fewer factors that scientists need to take into account, hence they can study the systems as though they have fewer parts and properties. Thus, there are fewer variables and parameters to measure or calculate and fewer dynamics to identify. Moreover, there are fewer possibilities of introducing errors to the models.
Finally, identifying general patterns can help scientists form expectations about how a system or phenomenon will behave in the future. If we understand what is happening now, then we can project this information into the future. In other words, successful generalisations form the basis of explanations, predictions and interventions, three of the main goals of science.
This is all well and good, provided that the generalisations do, in fact, identify real patterns in the world. The problem is that in ecological systems, which are complex and heterogeneous, what seems like a general pattern might be restricted in time or space (Figure 3.1). In fact, patterns can break. If this is the case, then how should ecologists approach generalisations? What sorts of expectations regarding generalisations are appropriate? Should we give up on them completely or should we increase our efforts to find ecological laws, even if they are few and far between?
Prescribing a specific set of rules or actions regarding generalisation is unlikely to be useful, as each set of rules or actions will probably only work well in a small number of cases. Instead, our suggestion is to take a step back and change our overall attitude and expectations of generalisations. What does that mean? It means that we should adjust our expectations of what generalisations mean, what sort of information we can glean from them and what their role is within a scientific investigation. So, for example, we can continue to look for patterns, but not necessarily expect to find them. Even if we do find a pattern, we should expect it to break. In other words, we should expect the level of generality to be constrained to variation within particular types of phenomena, such as disturbance, plant-soil feedback or migration. A parallel suggestion is to pay more attention to testing the scope of a generalisation rather than assuming (or hoping) that a generalisation that seems to hold will continue to do so. This can be achieved in many different ways, including experiment replications, testing for model robustness and using statistical review methods such as meta-analysis.
3.3.2 Explanations, predictions and interventions
Explanation and prediction are two central goals of science (Douglas 2009b). When scientists attempt to explain, they aim to understand how and why certain phenomena occur in the way that they do, or why certain systems exhibit a type of behaviour. When scientists predict, they make claims based on knowledge of past investigations about the occurrence of an event or phenomenon in the future, or about how a system will behave in the future. In addition, successful explanations and predictions are hallmarks of ‘good science’. When scientists manage to provide explanations of phenomena and their predictions turn out to be accurate, then we have good reason to have confidence in our theories and methods (Douglas 2009b). In contrast, when explanations and predictions start to fail, then this is usually a sign that something is wrong. A plethora of failed explanations and predictions in a scientific discipline are usually treated as a sign that some fundamental assumptions, theories or methods must be abandoned or at least revised (Douglas and Magnus 2013).
Explanations and predictions are based on generalisations. Providing a scientific explanation used to be synonymous with demonstrating how a particular phenomenon is an instance of a more general pattern, while scientific theories were those that subsumed many disparate phenomena under one framework (Douglas 2009b). The simpler the theoretical framework and the greater the number of phenomena it could explain, the more powerful it was. In causal accounts of explanation such as those of Woodward (2003), generalisations form the basis for explanations, as they describe causal regularities between variables. Here, to explain an event or phenomenon is to show that it is caused by another event or phenomenon and that this relationship is invariant or stable within certain parameters. In the case of prediction, the connection to generalisations is even clearer, as a prediction is just the projection of an existing pattern into the future.
But if generalisations fail, and explanations and predictions are based on generalisations, shouldn’t we expect explanations and predictions to also fail? We should, or at the very least, we should not be surprised or demoralised when they do. For example, explanations of community structures that were based on the keystone species concept have subsequently been proven to be false, as it turns out that keystone species do not behave in the same way across different systems (Cottee-Jones and Whittaker 2012). Similarly, predictions can fail when underlying patterns change or break. Another example comes from species distribution models (SDMs), which are often used to predict the range of a species colonising a new area, by projecting variables of the species’ native region to the new area (Sobek-Swant et al. 2012). However, climate change can render these predictions inaccurate, as unexpected higher temperatures or fewer extreme cold events can increase a species’ range to regions that it would normally not be able to colonise (Cuddington et al. 2018).
Ecological research is not exclusively focused on building a body of knowledge for its own sake. Quite often, the reason for investigating a certain phenomenon, system or dynamic is so that ecologists can intervene and induce, change or stop something from occurring. In other words, ecologists often apply knowledge from their scientific investigations so as to intervene in ecological systems. Yet, as these interventions are based on knowledge of existing systems – knowledge of generalisations, explanations and predictions – when these fail then the subsequent interventions are also likely to fail. If a group of ecologists are planning an intervention to stop the spread of a potential invasive insect, they may base their intervention on the predictions of an SDM. However, if that SDM predicts a significantly smaller range of the insect’s spread than it is actually capable of (due to higher temperatures) then the subsequent intervention which focuses on the smaller range is likely to fail.
3.3.3 Scientific and ethical values
Another way in which complexity manifests in ecological research is in the context of values. Philosophers of science distinguish between epistemic and non-epistemic values. Epistemic values (sometimes called cognitive or even scientific values) are those that directly help with the attainment of knowledge, such as truth, empirical adequacy, explanatory power, simplicity, generality, theoretical unity etc. Non-epistemic values (sometimes called contextual or ethical values) are those that arise from the social and/or ethical context within which a scientific investigation takes place, such as justice, equality, conservation, diversity etc. (Reiss and Sprenger 2020). For much of the 20th and 21st centuries, there has been an ongoing debate concerning the relationship between the two types of values and the appropriate role for each type of value in scientific practice. More specifically, while there is widespread agreement that epistemic values are important for most aspects of scientific practice, there is much less agreement on the value and role of non-epistemic values in science (Douglas 2009a).
From the Enlightenment until the mid-20th Century, the received view was that non-epistemic values had little or no place in science, as they detracted from the objectivity that scientists ought to strive for at all costs (McMullin 1982). A slightly less extreme version of this view is that non-epistemic values have a role to play, but only in some aspects of science, for example, when it comes to choosing which topics to study or how to allocate funding to various disciplines or sub-disciplines within an academic institution. Still, for each scientific investigation, when it comes to testing, accepting or rejecting hypotheses, this view dictates that only epistemic values ought to play a role (Reiss and Sprenger 2020).
More recent philosophical views show that the prevailing attitude towards non-epistemic values has shifted. Several philosophers have pointed out that it is impossible to keep non-epistemic values out of science completely (Douglas 2009a). More importantly, there are cases where separating epistemic and non-epistemic values and attempting to eliminate the latter from scientific investigations can actually result in worse outcomes (i.e. inaccurate results and/or an increase in bias). For example, there is a large body of research on intellectual and physical differences between men and women that is based on assumptions of female inferiority (Kourany 2003). As long as these assumptions remained unchallenged, they were accepted as part of the scientific body of knowledge, thus perpetuating the illusion that the results of these studies were objective. Only after scientists with an explicit feminist perspective challenged these assumptions were they and the many results based on them questioned. A particularly poignant case is that of archaeological findings, where most tools that indicate advances in human evolution were immediately – and mistakenly – associated with males (Kourany 2003).
The issue of competing epistemic and non-epistemic values is particularly important in ecology, because the behaviour of ecological systems affects and is affected by human societies. This includes exploitation for resources (e.g. forests or marine ecosystems), management of biological invasions, biodiversity conservation measures and so on. Scientific investigations into these systems are inherently and inescapably ‘ethically driven’ (Justus 2021). Ethical values affect the very concepts and methods used in the investigation, which, in turn, affect how choices for various strategies are made and evaluated. In addition, the results of these investigations inform policy decisions and thus have ethical implications for human societies. For example, research on biodiversity is loaded with values. First, the concept itself is not neutral or merely descriptive, but has a positive normative connotation: biodiversity is considered to be a good thing that ought to be preserved. Second, there are different ways to conceptualise biodiversity, which affect how it is measured. Different measurements can result in very different strategies or policies regarding an area or species. Third, policies for biodiversity conservation are made in the context of economic scarcity and in competition with other policies that are often based on very different values. For instance, determining the size of a marine nature reserve will depend on which species are considered important, how many of these are also economically viable, where commercial ferry routes are located, how much funding is allocated to implementing the policy and so on.
3.4 What sort of a science Ecology is not
The intent of this chapter so far has been to highlight some ways in which the complexity of ecological systems creates conceptual and practical difficulties for ecologists. But this is only half of the story. The more interesting question is what can and should be done in light of these difficulties. This is where delving deeper into the philosophical foundations of ecology can be illuminating. We will start by sketching out the traditional picture of science, based on philosophical views of the early to mid-20th century, and show how and why it does not work well for Ecology. Luckily, there are philosophical views that can make sense of, and provide a coherent theoretical framework for ecological research.
3.4.1 The traditional picture of science
The traditional picture of science, which appears in many textbooks and scientific lore as ‘the scientific method’ is a mishmash of two philosophical theories: hypothetico-deductivism and falsificationism. There are quite a few versions of hypothetico-deductivism out there, but at its basis it is a framework for understanding how hypotheses are (or should be) tested: scientists formulate hypotheses (hence the hypothetico-) which entail certain expectations (E). The deductivism comes in because of the ‘entail’; that is, the expectations are meant to be logically deducible from the hypothesis. This gives us the logical statement: if H then E (if the hypothesis is true, then the expectation must be true). A typical example used in philosophical discussions is the hypothesis ‘All ravens are black’ which entails the expectation E (the next raven I will see will be black). Apologies to ecologists for perpetuating this example of ‘indoor ornithology’ (Goodman 1955 in Godfrey-Smith 2009), but it actually works in the ecological context. So, we can go out in the world (or our lab, where we could be waiting for the raven eggs to hatch) and observe whether E occurs. If our observations match our expectations (i.e. the next raven, and the one after that, and so on, is in fact black) then our hypothesis H must also be true. Right?
Wrong! Deducing particular phenomena or events from generalisations that we know to be true is all well and good if we already know that the generalisation is true. The problem with hypothesis testing is that we are in the process of trying to determine whether the general hypothesis is true. So, rather than engaging in deduction, we are, in fact, engaging in induction – where we are attempting to generalise ‘upwards’ from a number of particular instances (Figure 3.2). Now, you might think that this is not a problem – scientists do it all the time – but many philosophers, most notably Hume, are inductive sceptics. They believe that we can never be 100% sure that a generalisation constructed from many observed instances holds. In other words, just because you have observed 1,000,000,000 black ravens does not mean that every single raven in all of time and space will be black. Here the reason for sticking with the example should become apparent to ecologists – it is quite easy to breed ravens of different colours, while there are also occasional ravens found with leucism (white pigmentation). So, our hypothesis cannot be confirmed. This might not be a huge deal in the case of ravens, but the worry is that the same problem (called the problem of induction) affects our ability to confirm all hypotheses. If we take the problem of induction seriously, then no experiment or model will ever be sufficient to make us certain about any hypothesis. Every time a test turns out positive (that is, it supports the hypothesis) all we know is that we have no reason to reject it.
In the mid-20th century, there were two main suggestions for how to deal with this problem. The first was to overcome the problem of induction by discovering so-called ‘laws of nature’ and the second was Popper’s falsificationism.
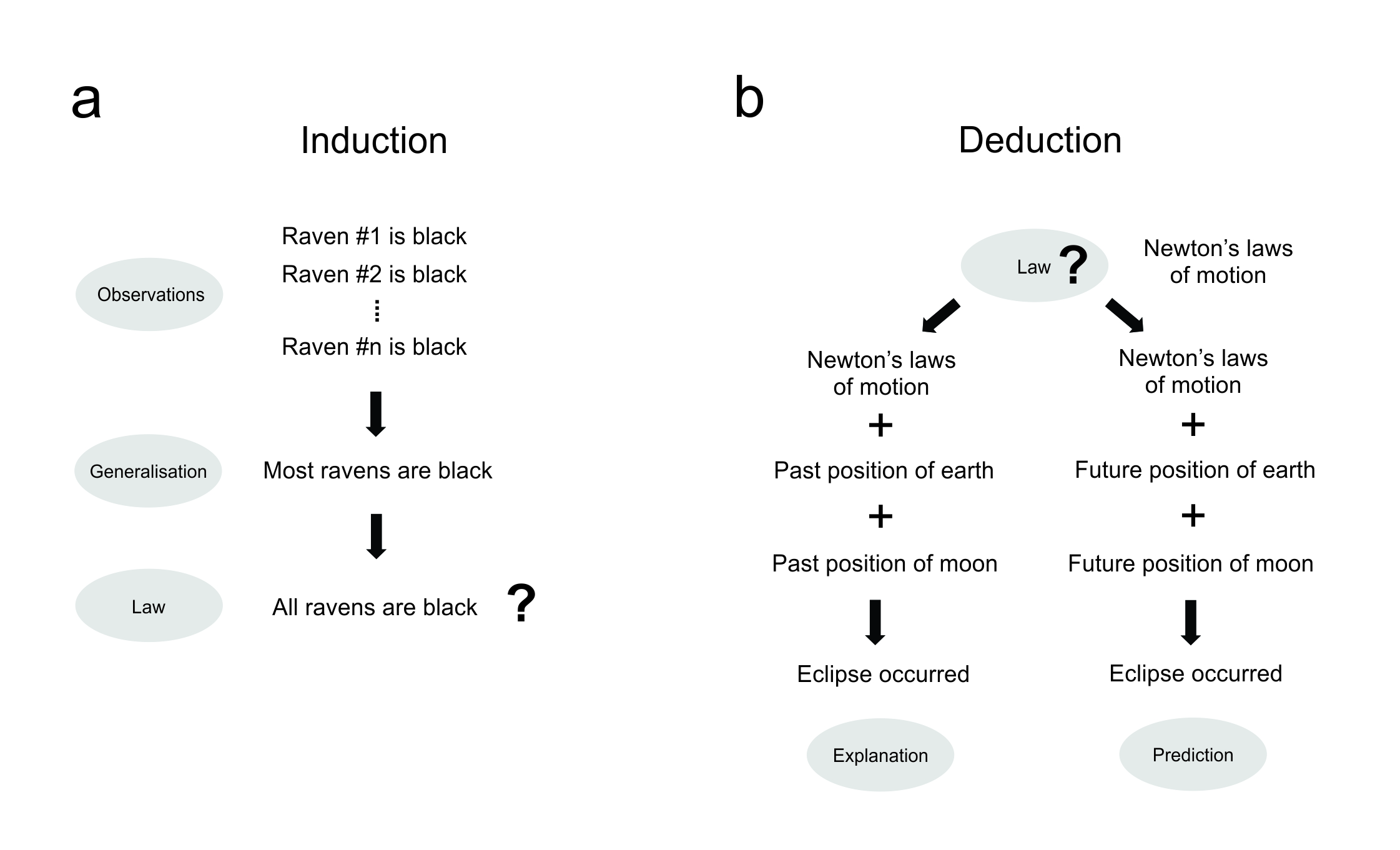
Figure 3.2 Traditional pictures of science. In induction (a), we start by making observations. When we have enough of these, we might be able to make generalisations. But is this generalisation merely accidental or a genuine law? We can never be 100% sure (the problem of induction). In deduction (b), genuine laws of nature can be used to make explanations (by showing that an event is an instance of the more general pattern described by the law) or predictions (by projecting the pattern described by the law to an event in the future). This symmetrical portrayal of explanation and prediction is called the symmetry thesis (Hempel and Oppenheim 1948). The problem is that the deductive structure only works if laws are already established. But establishing laws is difficult, due to the problem of induction.
3.4.2 Laws of nature
Laws of nature are generalisations that have three main characteristics: (1) universality (they cover all of space and time), (2) truth (they are exceptionless) and (3) natural necessity (they are not accidental) (Mitchell 2003). The first and second characteristics concern the scope of generalisations. Universal generalisations exist throughout all space and time. They are true if they hold in all instances within their defined scope. Thus, even if a generalisation is not universal, it can still be exceptionless within a specified domain. For example, it is likely that in the first few minutes after the ‘big bang’, only helium, deuterium and lithium were formed. All other elements were formed later, as stars developed (Mitchell 2003). Thus, a law stating that no uranium-235 sphere is larger than 55 kg is only applicable to the time where uranium-235 actually exists. The third characteristic aims to distinguish between generalisations that are necessary and those that are merely accidental. For example, the generalisation ‘all the pens in my drawer are blue’. There is nothing about my drawer or the nature of pens that affects whether or not the statement is, in fact, true. If it turns out to be true, then it is true accidentally (for further explanation on laws versus accidental generalisations see Box 3.1).
Why were scientists and philosophers of the past obsessed with finding laws of nature? A full answer to this question is long and complicated, but here is a short summary: laws of nature can bypass the problem of induction.
If you assume that the natural world is orderly and has patterns, then it is a good idea to identify those patterns. These patterns can then provide the basis for a number of scientific endeavours: you can explain something by showing how it fits into an existing pattern, you can predict something by projecting an existing pattern into the future and you can intervene on a system to enhance or break an existing pattern. The problem is that not all patterns are equally stable, some are contingent on other factors, some are ephemeral, while some are illusory. So, the aim of science (according to some scientists and philosophers) was to identify the most stable of these patterns – which they called laws of nature – and to distinguish them from merely accidental generalisations. In other words, what makes a law a law is that it is not arbitrary or accidental, it has some underlying reason for its existence.
Consider the following three generalisations:
(1) All spheres of uranium-235 have a diameter of less than 100 m.
(2) All spheres of gold have a diameter of less than 100 m.
(3) All the pens in my drawer are blue.
The traditional story is the following: all three of these generalisations are true, yet only the first is necessarily true. That is, there is something about uranium-235 that makes it impossible for a sphere of it to exceed 100 m. That ‘something’ is a chain reaction of nuclear fission, which spontaneously occurs once uranium-235 reaches critical mass. In contrast, there is nothing about pens or my drawer that makes it necessary that all the pens in the drawer are blue. Even if I only buy blue pens, this is still an accidental fact of the world, there is nothing about me or my pens that makes it necessary for me to have made such a decision. What about the gold? It may seem impossible for such a sphere to exist – even if there is enough gold in the world to construct such a sphere, it is extremely unlikely that you could convince everyone to give up their gold for such a project. Still, these constraints are practical, it would be possible to construct a gold sphere so large if we managed to collect enough gold.
If you are not entirely convinced that there is a strict dichotomy between laws and accidental generalisations, you are not alone. More recent philosophical views present a much more nuanced picture (Mitchell 2000). On the one hand, established examples of laws, including the uranium spheres, are in some sense contingent – the law only exists in a universe where uranium exists, and only after it has come into existence. On the other hand, many generalisations in biology, such as Mendel’s laws, are the products of evolution, and are sufficiently stable to generate explanations and predictions. While generalisations like (3) are unlikely to be useful for science, there are many modest, highly contingent generalisations that are useful. The problem with such generalisations is that they don’t neatly bypass the problem of induction. Alas! Uncertainty remains with us.
The point is that if you discover a law of nature, then you can bypass the problem of induction, by showing (deductively) that a phenomenon or event is a particular instance of the general pattern described by the law. This goes a long way in explaining a lot of scientific and philosophical practice in the subsequent years, especially the obsession with ever increasing the scope of generalisations, and the quest for discovering laws that are specific to particular disciplines such as ecology.
For many philosophers of science, laws became the basis for their scientific ‘worldview’, as they formed the basis of what counted as ‘good’ or high-quality science. For example, a popular view of scientific explanation in the mid-20th century was the so-called deductive-nomological (DN) model (also called the covering-law model), where a scientific explanation was nothing more than a deduction of a phenomenon or event from an existing law and certain antecedent conditions (Hempel and Oppenheim 1948). Many scientists took these ideas to heart and realised that finding laws of nature that were specific to one’s own discipline effectively legitimised the discipline as a true and independent science (Mayr 1996). In other words, if Ecology had its own laws, then it would definitely count as an autonomous science. The problem is that laws of nature – at least of the type that would allow for overcoming the problem of induction – remain elusive in many sciences, ecology included.
3.4.3 Ecological ‘laws’?
In ecology, various candidates for lawhood have been proposed, including exponential/logistic growth, allometric metabolic/body weight relationships and species/area relationships (Colyvan and Ginzburg 2003) . However, none of these potential laws have been proven to be universal or exceptionless (Shrader-Frechette and McCoy 1993). In addition, many of these supposed laws seem like accidental regularities rather than regularities that are necessarily true (Lange 2005). In fact, given the difficulties of generating generalisations, it seems unlikely that ecology will ever be awash with laws of nature.
There are roughly two responses to this state of affairs, both of which have been espoused by philosophers and ecologists.
The first option is to just give up on laws completely, arguing that sciences like biology and ecology just do not have the types of regularities that count as laws (see Lange 2005). Schrader-Frechette and McCoy (1993) argued that Ecology is ‘more a science of case studies and statistical regularities than a science of exceptionless, general laws’. The second option is to revise the notion of laws, so that ecological regularities make the cut. The idea is that the classic dichotomies (necessary/accidental, universal/contingent) are not useful for practicing scientists, as the point of generalisations is that they help scientists achieve various goals, such as explaining and predicting natural phenomena or intervening on the world. In this context, the most important characteristic of ecological laws is that they are invariant (or stable, or resilient – different accounts use different terms, which are not identical, but close enough for the purposes of this discussion) (Mitchell 2000).
Linquist et al. (2016) identify three dimensions of ‘resilience’. A generalisation is ‘taxonomically resilient’ if it is stable across a different number of species or higher-level taxa. ‘Habitat resilience’ occurs when a generalisation is invariant across a broad set of regions or biological contexts. ‘Spatial resilience’ occurs when a generalisation remains invariant at the scale of whole organisms, molecular systems and genomic communities. They use meta-analyses to investigate the extent to which various generalisations are resilient across these three dimensions. For example, they found that the generalisation ‘Habitat fragmentation negatively impacts pollination and reproduction in plants’ is resilient across ‘five distinct habitats and across 89 species from 49 families’. They believe that these types of generalisations qualify as laws.
It is worth noting that the only agreement, in philosophical circles, is that sciences such as biology or ecology do not have laws in the traditional sense. The jury is still out when it comes to whether we should revise our notion of laws or give up on laws in these sciences. The important point is that in the absence of traditional laws, we must find other ways in which to test the quality of ecological research.
3.4.4 Falsificationism
A different suggestion for dealing with the problem of induction is to ‘bite the bullet’ and accept that it cannot be overcome. A famous proponent of such a view was Popper. Contrary to how his views are often portrayed in scientific textbooks, Popper believed that scientists can never confirm hypotheses with 100% certainty, they can only refute hypotheses (Popper 1965; see also Godfrey-Smith 2009).
Going back to our raven example, if you observe a white raven, then you have conclusively rejected the hypothesis that all ravens are black. This notion of refutation was so important that it formed the foundation of Popper’s philosophy of science, including his view of scientific progress and his method for distinguishing science from pseudoscience. For Popper, science progressed through a continual process of conjecture (formulating hypotheses) and refutation (rejecting hypotheses). As soon as a hypothesis is rejected a new hypothesis is constructed to take its place, which is then tested until it also is rejected, and so on. There are some important implications of this view: while we can be sure that hypotheses accepted by past scientists are false, we have no reason to believe that the hypotheses we currently hold are true. It is inevitable that they too will eventually be refuted. This means that scientific knowledge is nowhere near to being foolproof. It is just as likely to be false than non-scientific knowledge.
But then, how can we distinguish between science and non-science? According to Popper, true science is the one that is most likely to turn out false. When put like that it sounds very counterintuitive, but a short explanation might help. The key difference between science and pseudoscience lies in accepting the possibility of your hypothesis being refuted. True scientists, therefore, make bold hypotheses that are falsifiable. Scientists make hypotheses that can be tested by available methods and observations that are actually possible. These hypotheses are novel (not of events that have already been observed) and precise (not vague) so that it is possible to determine when they are refuted. In contrast, pseudo-scientists construct hypotheses that are difficult to test and thus not easily refuted.
3.4.5 Falsification and prediction
Unfortunately, refutation has its own set of problems. The main issue is the so-called Duhem-Quine problem, or holism about testing (Godfrey-Smith 2009) that no hypothesis is tested in isolation but is embedded in a network of additional assumptions (often called auxiliary assumptions). These are assumptions about our instruments of measurement or observation, the circumstances in which the observation is made, the reliability of existing data or records and so on (Godfrey-Smith 2009). This means that the test of the hypothesis is actually a test of the conjunction of the hypothesis and all the auxiliary assumptions (some of which we might not even be aware of). So, if the expectation (E) turns out to be false, we can be sure that something in the whole conjunction is false, but we cannot be sure whether it is the hypothesis or one or more of the auxiliary assumptions.
Imagine that you are conducting a field experiment in some alpine meadows, examining why a particular butterfly population has dramatic fluctuations in population size. You want to test the hypothesis that abnormally warm temperatures in November cause the population to drop. So, you go out, set up your thermometers in July and regularly check them to see that they are working. As it turns out, the November temperatures were abnormally warm, but the butterfly population did not drop (in fact, it grew to higher levels than previous years, which had normal temperatures in November). Now, it could be that abnormally warm November temperatures actually do not have an effect on the butterfly populations. However, a number of other factors could be at play. Maybe mistakes were made in counting the butterfly population (this year, or in previous years) so that this year’s population was estimated to be larger than it actually was, or that populations in previous years were estimated to be smaller than they actually were. Alternatively, the thermometers could be faulty, calibrated wrongly or overheating because of the (atypical) lack of snow cover. Another issue could be that warm temperatures do cause population decline, but only in the presence (or absence) of other factors that you have not yet identified. The list of plausible alternative explanations can go on and on. The point is that at least without additional tests, we cannot be sure whether we should consider the hypothesis refuted.
Most scientists and philosophers nowadays do not believe that this problem is insurmountable, provided that predictions turn out to be true enough, often enough. What exactly counts as true enough and often enough tends to incur some level of debate, but usually certain norms emerge within a particular discipline about when scientists ought to be worried about the quality of predictions. The problem is that in sciences that study complex systems, the success rate of predictions is much lower than it is in other sciences (for the reasons explained in the section on The Challenges of Complexity). In Ecology, this has been an ongoing thorn in the side of many prominent ecologists, who with some regularity write papers lamenting the generalisability of ecological theories and the success rate of predictions (two of the most famous of these are Peters 1991, Lawton 1999). Moreover, given the outlook of laws and generalisations in ecology, it seems unlikely that the success rate of predictions is going to increase any time soon. So, does this mean that Ecology is at best an immature science and at worst just a lower quality science?
The answer is no! Ecology is a science that deals with systems that are complex – this complexity was not taken into account by the 20th-century philosophers who constructed the received views about science. In fact, the traditional picture of science has been extensively questioned and criticised. We will examine this critique in the next section and see whether this alternative view better captures ecological reality.
3.4.6 Objectivity
In the section Scientific and Ethical Values we outlined the difference between epistemic and non-epistemic values and showed how these values are intertwined in ecological research. We also highlighted the relationship between debates about values and objectivity. In this section, we will delve deeper into this discussion and show that two traditional views on objectivity do not work for ecological research.
To recap, the received view of values in science from the Enlightenment until the mid-20th century was that only epistemic values have a place in scientific investigations, while allowing non-epistemic values into science would result in a failure of objectivity, which would necessarily undermine scientific results. However, this view was successfully challenged by many scientists, historians and philosophers, starting with Kuhn in The Structure of Scientific Revolutions (1962). Kuhn was a physicist, historian and philosopher of science, who (correctly) pointed out that the picture of science painted by his predecessors was implausible and inaccurate (Okasha 2002). Scientists were not hyper-rational creatures who acquired knowledge and resolved any disputes by dispassionately observing ‘neutral’ (unbiased) facts. Despite scientists’ best intentions, facts, data and phenomena are ‘theory laden’, that is, identifying, observing and interpreting these facts, data and phenomena are affected by each scientist’s background beliefs.
So far, none of this is hugely controversial. As discussed earlier, in many sciences, especially those like ecology which deal with complex systems and whose results have implications beyond academia, epistemic and non-epistemic values are inextricably intertwined. Moreover, assuming that the status quo is free from non-epistemic values can decrease the overall objectivity of an investigation by obscuring or completely masking underlying biases.
However, Kuhn’s (1962) argument can be interpreted in a much stronger way, namely as an argument that science is an entirely irrational activity, where any notion of truth is relative to a particular ‘paradigm’, where a paradigm is a set of theoretical and methodological assumptions that a group of scientists accept at a given time (Okasha 2002). This sparked a movement in some academic circles (such as the strong program in sociology and cultural relativism in anthropology) which denied any role for objectivity, truth and rationality in science (Okasha 2002). The tone in which the book is written is admittedly quite radical, but Kuhn himself disagreed with this interpretation of his work (Godfrey-Smith 2009). He subsequently tried to clarify his views and rein in the most extreme interpretations, but it was too late.
So, we are now left with two extreme views. The first, where science is fully objective and scientists hyper-rational, is unattainable, while the second, where science is fully subjective and scientists irrational, is undesirable. Yet there is a middle ground. Accepting that scientists are not maximally objective does not mean that they are entirely irrational. Accepting that established theories can be problematic does not mean that we cannot distinguish between higher and lower quality research.
A similar point can be made with respect to values. Non-epistemic values can be important does not mean that they always are important, nor that they should be prioritised over epistemic values (Justus 2021). The point is rather to recognise that they may have an effect and that this effect is not necessarily problematic. Adopting a more open attitude to the existence and potential of non-epistemic values can sometimes help our scientific endeavours, by highlighting a potential source of bias, or by helping to counteract another hidden source of bias.
3.5 So what?
In this chapter we have painted a few pictures of science that do not accurately capture Ecology. Ecology is not a hyper-rational, fully objective science based on exceptionless laws of nature, whose hypotheses are constantly tested through precise predictions. Still, this does not mean that ecology is wholly unobjective, irrational and untestable. Not all ecological research is of equally high quality, and it is important to have standards to distinguish between high and low quality research – yet these standards are not to be found in (outdated) 20th-century philosophical writings. So, what can ecologists do in light of these issues? We end the chapter with some suggestions. These are not intended as specific methodological prescriptions, but more abstract recommendations for our attitudes and expectations regarding concepts and methods in ecological research.
3.5.1 Modest expectations
The first suggestion is to change our attitude and expectations regarding generalisations. In other words, rather than assuming that an observed pattern holds widely, we should focus time and resources for probing, exploring and testing whether a generalisation is possible and what its scope and limitations are. This may seem like a small point, but it is actually very important.
Changing our attitude and expectations towards generalisations is a key step in recognising the potential of generalisations in ecology. We should not be dismissive or embarrassed when generalisations turn out to be limited in scope or break down completely. We should not even expect many generalisations to actually exist in complex, heterogeneous systems. This does not mean that we should stop trying to identify them. It means that we should value them for what they are when we do find them. Examples of successful and useful generalisations where the scope is limited to variation within particular types of phenomena include disturbance (Peters et al. 2006), plant-soil feedback (Casper and Castelli 2007) and migration (Kelly and Horton 2016).
3.5.2 Methodological pluralism
The second suggestion is to make wider use of the methodological toolkit that ecologists have at their disposal.
There are numerous ongoing debates in Ecology concerning the best method to use for a set of issues or phenomena. One example is the debate concerning optimal model complexity, where one camp argues for using simple models with few parameters and the other argues for using more complex models that incorporate a larger number of parameters and dynamics (Evans et al. 2013; Levins 1966). However, rather than assuming that a single model or method will be superior, ecologists could adopt a more pluralistic strategy.
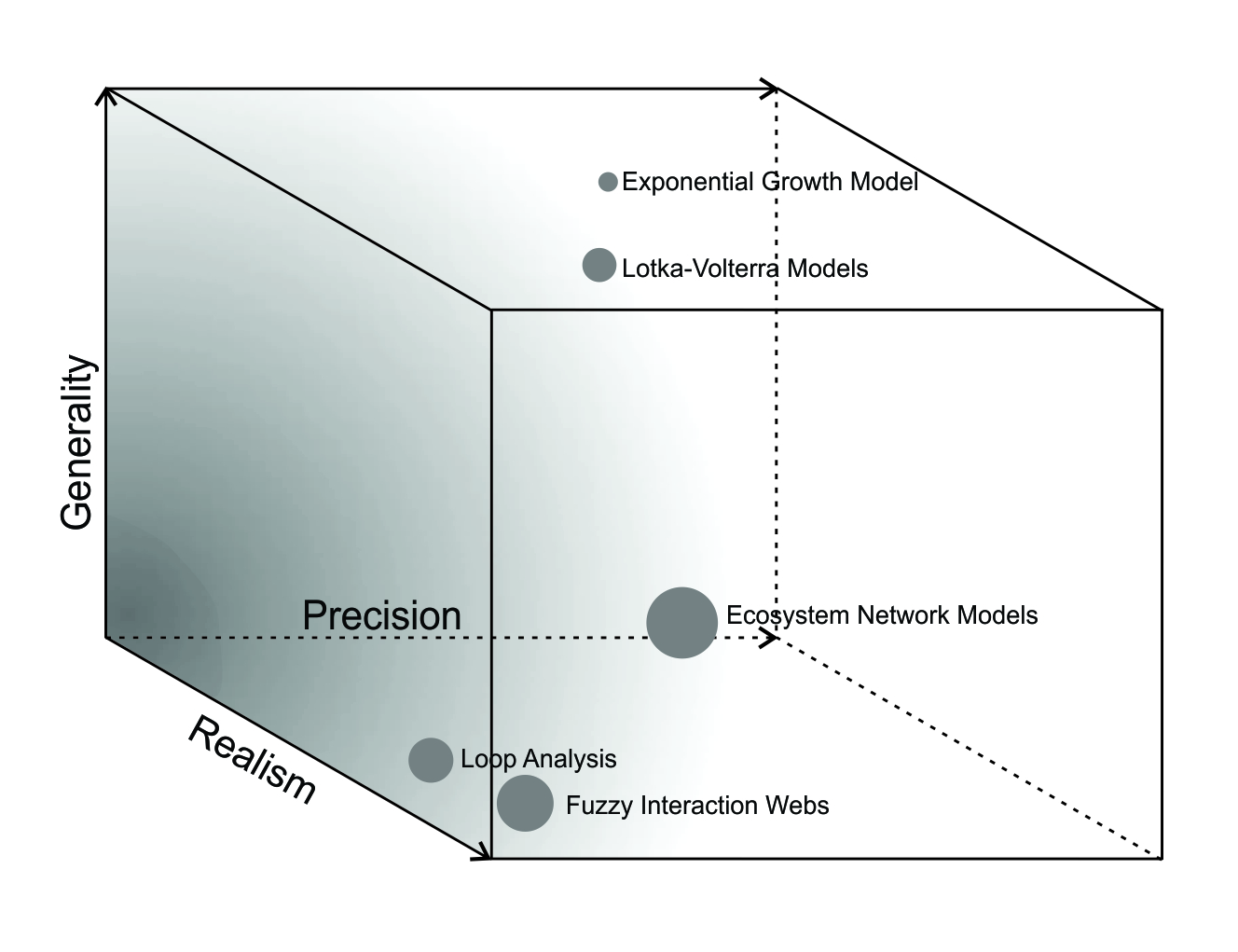
Figure 3.3 Methodological pluralism. Ecologists do not need to limit themselves to one type of approach, even in a single investigation. Models can differ in terms of generality (how many systems they apply to), realism (how many real-world parameters they contain) and precision (how finely specified their predictions are). The size of each circle represents how far along the realism axis a model is (larger circles are more realistic models). Using different models allows us to explore more of the space of possibility. Moreover, if these different models are independent, i.e. not based on the same assumptions, then if their outputs converge we can be more confident that the results are accurate (inferential robustness). Based on Levins (1966).
This pluralism can be achieved at two levels. At the basic level, it is important to recognize that each investigation occurs in a different context with different goals (Figure 3.3). Thus, in some cases generalisation will be more important, while in others predictive accuracy will be more important – each of these goals might render a different method optimal, such as models that aim at identifying a pattern versus models that aim at predicting within a particular system (Justus 2021; Levins 1966). Here are three examples:• The exponential growth model (general and precise but not realistic) applies to many systems but is not very realistic. All populations would grow exponentially if there were no other factors limiting them, but many such factors are present in the real world.
• Ecosystem network models (realistic and precise but not general) incorporate many factors present in the real world, such as many of the ecosystem’s populations and the interactions between them, but they are built each time for a particular system.
• Loop analysis (realistic and general but not precise) can incorporate many factors present in the real world and apply to many systems in the world, but their results (predictions) are not finely specified.
At a deeper level, ecologists can adopt a pluralistic strategy within a particular investigation. Here, the idea is that scientists should use multiple models to investigate a particular phenomenon (Reiners and Lockwood 2010). As long as the models are independent from each other, then they can cancel out, or at least mitigate, each other’s errors and biases. If we are lucky, the model outputs will converge, yielding inferential robustness, which can increase our confidence in the models (Weisberg 2006). In Levins’s words, ‘truth is at the intersection of independent lies’ (Levins 1966).
In short, ecology is not an immature science; it is a difficult science. Ecological systems are surprising, irregular and mercurial. The scientists who manage to get information about them should be commended in their endeavours, even if they suffer setbacks and complications. Luckily, ecologists already possess the concepts and methods necessary to achieve high-quality research, though they may not always recognise it.
3.6 References
Casper, B. B., and J. P. Castelli. 2007. Evaluating plant-soil feedback together with competition in a serpentine grassland. Ecology Letters 10:394–400. doi:10.1111/j.1461-0248.2007.01030.x
Colyvan, M., and L. R. Ginzburg. 2003. Laws of nature and laws of ecology. Oikos 101:649–653. doi:10.1034/j.1600-0706.2003.12349.x
Cooper, G. J. (ed.). 2003. The science of the struggle for existence: On the foundations of ecology. Cambridge: Cambridge University Press. doi:10.1017/CBO9780511720154.002
Cottee-Jones, H. E. W., and R. J. Whittaker. 2012. Perspective: The keystone species concept: A critical appraisal. Frontiers of Biogeography 4:117–127. doi:10.21425/F5FBG12533
Cuddington, K., S. Sobek-Swant, J. C. Crosthwaite, et al. 2018. Probability of emerald ash borer impact for Canadian cities and North America: A mechanistic model. Biological Invasions 20:2661–2677. doi:10.1007/s10530-018-1725-0
Douglas, H. 2009a. Science, policy, and the value-free ideal. Pittsburgh: University of Pittsburgh Press. doi:10.2307/j.ctt6wrc78
Douglas, H. 2009b. Reintroducing prediction to explanation. Philosophy of Science 76:444–463. doi:10.1086/648111
Douglas, H., and P. D. Magnus. 2013. State of the field: Why novel prediction matters. Studies in History and Philosophy of Science 44:580–589. doi:10.1016/j.shpsa.2013.04.001
Elliott-Graves, A. 2018. Generality and causal interdependence in ecology. Philosophy of Science 85:1102–1114. doi:10.1086/699698
Elliott-Graves, A. 2019. The future of predictive ecology. Philosophical Topics 47:65–82. doi:10.5840/philtopics20194714
Elliott-Graves, A. (2023) Ecological complexity. Cambridge: Cambridge University Press
Evans, M. R., V. Grimm, K. Johst, et al. 2013. Do simple models lead to generality in ecology? Trends in Ecology and Evolution 28:578–583. doi:10.1016/j.tree.2013.05.022
Godfrey-Smith, P. 2009. Theory and reality. Chicago: University of Chicago Press.
Goodman, N. 1955. Fact, fiction & forecast. Cambridge, MA: Harvard University Press.
Hempel, C. G., and P. Oppenheim. 1948. Studies in the logic of explanation. Philosophy of Science 15:135–175. doi:10.1086/286983
Hooker, C. (ed.). 2011. Philosophy of complex systems. North Holland. doi:10.1016/C2009-0-06625-2
Justus, J. 2021. The philosophy of ecology: An introduction. Cambridge: Cambridge University Press. doi:10.1017/9781139626941
Kelly, J. F., and K. G. Horton. 2016. Toward a predictive macrosystems framework for migration ecology. Global Ecology and Biogeography 25:1159-1165. doi:10.1111/geb.12473
Kingsland, S. 1995. Modeling nature. Chicago: University of Chicago Press.
Kourany, J. 2003. A philosophy of science for the twenty-first century. Philosophy of Science 70:1-14. doi:10.1086/367864
Kuhn, T. S. 1962. The structure of scientific revolutions. Chicago: University of Chicago Press.
Lange, M. 2005. Ecological laws: What would they be and why would they matter? Oikos 110:394–403. doi:10.1111/j.0030-1299.2005.14110.x
Lawton, J. H. 1999. Are there general laws in ecology? Oikos 84:177–192.
Levin, S. A. 1998. Ecosystems and the biosphere as complex adaptive systems. Ecosystems 1:431–436. doi:10.1007/s100219900037
Levins, R. 1966. The strategy of model building in population biology. American Scientist 54:421–431. doi:10.2307/27836590
Levins, R. 1993. A response to Orzack and Sober: Formal analysis and the fluidity of science. The Quarterly Review of Biology 68:547–555. doi:10.1086/418302
Linquist, S., T. R. Gregory, T. A. Elliott, et al. 2016. Yes! There are resilient generalizations (or ‘laws’) in ecology. The Quarterly Review of Biology 91:119–131. doi:10.1086/686809
Loreau, M., S. Naeem, P. Inchausti, P., et al. 2001. Biodiversity and ecosystem functioning: Current knowledge and future challenges. Science 294:804–808. doi:10.1126/science.1064088
Matthewson, J. 2011. Trade-offs in model-building: A more target-oriented approach. Studies in History and Philosophy of Science Part A 42:324–333. doi:10.1016/j.shpsa.2010.11.040
Mayr, E. 1996. The autonomy of biology: The position of biology among the sciences. The Quarterly Review of Biology 71:97-106.
McMullin, E. 1982. Values in science. PSA: Proceedings of the Biennial Meeting of the Philosophy of Science Association 1982 2:2–28. doi:10.1086/psaprocbienmeetp.1982.2.192409
Mitchell, S. D. 2000. Dimensions of scientific law. Philosophy of Science 67:242–265. doi: 10.1086/392774
Mitchell, S. D. 2003. Biological complexity and integrative pluralism. Cambridge: Cambridge University Press. doi:10.1017/CBO9780511802683
Odenbaugh, J. 2011. Complex ecological systems. In Philosophy of complex systems, ed. C. Hooker, 421–431. Elsevier. doi:10.1016/B978-0-444-52076-0.50015-8
Okasha, S. 2002. Philosophy of science: A very short introduction. Oxford: Oxford University Press.
Peters, D. P. C., B. T. Bestelmeyer, and J. E. Herrick. 2006. Disentangling complex landscapes: New insights into arid and semiarid system dynamics. BioScience 56:491-501. doi:10.1641/0006-3568(2006)56[491:DCLNII]2.0.CO;2
Peters, R. H. 1991. A critique for ecology. Cambridge: Cambridge University Press.
Pimm, S. L. 1991. The balance of nature? Chicago: University of Chicago Press.
Popper, K. R. 1959. The logic of scientific discovery. New York: Basic Books.
Reiss, J., and J. Sprenger. 2020. Scientific objectivity. In The Stanford Encyclopedia of Philosophy (Winter 2020 Edition), ed. E. N. Zalta. https://plato.stanford.edu/archives/win2020/entries/scientific-objectivity/ accessed 26 September 2022.
Reiners, W. A., and J. A. Lockwood. 2020. Philosophical foundations for the practices of ecology. Cambridge: Cambridge University Press.
Shrader-Frechette, K., and E. D. McCoy.1994. Applied ecology and the logic of case studies. Philosophy of Science 61:228–249.
Shrader-Frechette, K. S., and E. D. McCoy. 1993. Method in ecology: Strategies for conservation. Cambridge: Cambridge University Press.
Sobek-Swant, S., D. A. Kluza, K. Cuddington, and D. B. Lyons. 2012. Potential distribution of emerald ash borer: What can we learn from ecological niche models using Maxent and GARP? Forest Ecology and Management 281:23–31. doi:10.1016/j.foreco.2012.06.017
Strevens, M. 2004. The causal and unification approaches to explanation unified - causally. Noûs 38:154–176. doi: 10.1111/j.1468-0068.2004.00466.x
Weisberg, M. 2006. Robustness analysis. Philosophy of Science 73:730–742. doi:10.1086/518628
Woodward, J. 2003. Making things happen: A theory of causal explanation. Oxford: Oxford University Press. doi:10.1093/0195155270.001.0001