2 The evolution of ecology
How to cite this chapter
Cousens R. D. and M. R. T. Dale (2023) The evolution of ecology. In: Cousens R. D. (ed.) Effective ecology: seeking success in a hard science. CRC Press, Taylor & Francis, Milton Park, United Kingdom, pp. 13-32. Doi:10.1201/9781003314332-2
Abstract Ecology has evolved and will continue to evolve. Do we allow this evolution to occur spontaneously, or should we intervene when we identify things that need to change? In this chapter, we begin with a traditional, historical narrative of the way that Ecology has evolved over two millennia. We then consider two models for the system on which that evolution takes effect. The first is an analogy of an agent-based system, in which each individual scientist makes decisions and takes directions according to the environment they are in and their own decision criteria. The result is a set of unique trajectories of individual ecologists, each pursuing their research as their ideas develop and proliferate. The second model considers the roles of ecologists within the much wider, and multilayered, network of which they are just one part, drawing on the same network theory that is applied in research on ecological systems. That network is complex and its complexity is one feature that makes our science hard. Basic network analysis would suggest that because its function is knowledge discovery, communication and synthesis, Ecology will do better with more (and more effective) links, communication and collaboration.
2.1 Can Ecology improve?
Ecology – and by this we mean the scientific discipline of Ecology (see Box 1.1) - has achieved much since it was recognised in the mid-19th century. We understand a great deal about the interactions between organisms and their environment and the world about us.
The literature describing what we have learned is vast and ever-expanding. We are solving many practical problems and answering many fundamental questions, to a degree that satisfies the majority of ecologists (though see Chapter 10). Our contributions are increasingly appreciated by decision-makers and, although our advice is not always taken, we have succeeded in having ecological principles considered seriously by society. That seems like success, but…
Ecology remains a hard science, in which significant advances seem difficult to achieve. It is reasonable to ask whether ecology is as good as it can be and whether, or how, it can be improved.
Over the decades, there have been many criticisms of ecology (Box 2.1) and encouragement for ecology to change. Most of these criticisms have some justifiable basis, even if their importance has been hotly debated; solutions have been difficult to identify and ultimately the proposals have been either ignored or summarily dismissed.
Should we trust the existing feedback mechanisms within Ecology to optimise the discipline, maximising its effectiveness and minimising the effects of problems?
In other words: can we rely on the natural evolution of our system of doing science? If one approach to ecology, or one idea, is less effective than another, then it should decline in relative frequency over time, and the stronger the selection (the greater the difference in superiority), the more rapid the change should be. Selection of the fittest. This is how the peer review system should work: our colleagues give their views on our approaches and the consensus drives the direction of research, albeit inefficiently.
Ecologists who identify what they believe are serious flaws can do no more than to publish their concerns and arguments, then wait. No matter how strong an appeal for a paradigm shift is (Choi 2007; Rist 2013), there is no guarantee that it will be accepted (as Peters 1991 discovered: see Lawton 1991; Keddy and Weiher 1999). Scientific communities have strong inertia even in the face of overwhelming evidence - think of plate tectonics in geology, which took several decades to become widely accepted.
Can we give this evolution a helping hand, to move Ecology towards a better direction or more quickly? Having identified something new with clear benefits, can we intervene to encourage its adoption? Or something entrenched in the discipline which we would like to discourage? What would those actions be, in a system based on mutual exchange of ideas and largely without governance? Editorial boards, for example, have been unable to eliminate common statistical errors over several decades in which statisticians have drawn attention to our frequent and consistent errors.
Concerns about the way ecology is done have included:
-
dominance of descriptive studies (e.g. Whittaker 1962);
-
over-reliance on correlations as demonstrations of cause and effect (Carr et al. 2019);
-
paucity of hypotheses (Betts et al. 2021);
-
predilection for claims of confirmation (potentially resulting in ‘confirmation bias’: Brittan and Bandyopadhyay 2019);
-
failure to investigate alternative explanations (Betini et al. 2017);
-
lack of fundamental laws and testable theories (Marquet et al. 2014);
-
popularity of concepts that are inherently incapable of refutation (Scheiner 2013);
-
tendency to take up fads (Belovsky et al. 2004);
-
poor links between theoretical and empirical research (Simberloff 1981) and between pure and applied studies; and
-
and divisiveness of sub-disciplines (Dayton 1979).
(Note that one example reference has been given for each issue, even though there may be many.)
Another concern is ineffective quality control, which results in a high incidence of statistical errors (Fraser et al. 2018) and poor applications of various analytical methods (Hoekstra et al. 2012). We also see many implied criticisms, in the form of papers extolling a specific new approach and its virtues (at the expense of something else), such as calling for more interdisciplinary research (e.g. Kelly et al. 2019) or more transdisciplinary research (e.g. Marshall et al. 2018).
It is easy to list these criticisms, but it is hard to assess the extent to which they have actually detracted from the work or reduced its impact. Some of the concerns result from a mere philosophical belief that there are better ways of doing things - but without proof that progress could actually have been better (as in so-called ‘physics envy’: Nelson 2015). We can, perhaps, forgive ourselves a modicum of procedural errors in ecology, since no field of human endeavour, and no quality control system (Smith 2006), is perfect. Not every ecological study can break new ground: studies that might be dismissed as ‘busy work’ (Dayton 1979) may contribute by consolidating ideas, providing the data for meta-analyses, exploring context-specific conclusions or exploring exceptions that may cause a re-think of ideas. Indeed, any science needs a balance between advance and consolidation and there is clearly no criterion for what that balance should be.
Any discussion of change needs to be based on a thorough appreciation of how ecological research evolves. In this chapter, we will examine the evolution of ecology in three distinct ways. First, we follow a brief traditional, historical narrative of the changes that have occurred in the practice of ecological science, as ecologists have ‘gone with the flow’. With the value of hindsight, we can see how ecology has evolved to be this way – and has become this vast - because……
Next, we consider the place of individual researchers within the great, apparently unplanned effort that constitutes the entirety of the discipline. We use the analogy of an agent-based system, in which each individual makes decisions and takes directions according to the environment they are in and their own decision criteria. The result is a set of unique trajectories of individual ecologists, each pursuing their research as their ideas develop and proliferate. While the evolution of ecology may appear to be haphazard, the individuals, their efforts and their trajectories are under a form of selection pressure which must surely, over time, shape our discipline.
Finally, we consider the roles of ecologists within the much wider, and multi-layered, network of which they are just one part, drawing on the same network theory that is applied in research on ecological systems. We invite readers to apply the same principles to themselves, to better understand what they do and the way they do it.
2.2 A conventional history of Ecology
Sciences unfold upon a framework of history. In the case of most sciences, including ecology, we can trace their roots back by more than two millennia. One or two people start to ask questions about the world about them and come up with – often - naïve answers. Others come along over time, adding their new styles and ideas; the tools and procedures (such as experimentation, mathematical analysis and logic) that we use become ever more sophisticated and the answers that we reach become more persuasive. The science steadily gains in maturity and in ability. The scientific landscape upon which we can chart our activities starts to become occupied, patchily at first, but then the gaps begin to fill. Most sciences, at least in their early days, have no goals other than to gain greater understanding, so we have little idea in advance when different areas of the scientific landscape will become occupied. The scientists of the time ‘go with the flow’, following the questions and trends of the time and responding to occasional new directions. Expansion and proliferation seem to go on unabated. Inevitably, a cornucopia of information is produced, some of which allows us to make confident statements and reach generalisations while much of it - at the time - cannot be rationalised and we struggle to achieve insights. But we persist.
The pace of scientific activity also undergoes periods of increased interest and heightened recruitment of scientists, causing an inexorable increase in the cornucopial flow of information and the subsequent desire for explanations. One key aspect of what we call civilisation is that some of the people have the time to think and communicate, in the past often males in privileged positions who had the resources to do more than just survive. Some could afford travel, during which they made observations of plants, animals, geology or astronomy, which they interpreted and communicated to others. Communication led to the sharing of ideas with a common theme: to describe, classify and explain the natural world. From the ancient Greek philosophers, through the Enlightenment in Europe to the early establishment of universities, the number of people involved in science was relatively small and their capabilities were restricted.
The second half of the 20th century was a time of extraordinary growth for all sciences. Expansion of the tertiary education system produced more students, while increased government investment in research and conservation provided jobs related to ecology. Ecology became a profession rather than a pastime. There were widespread concerns about food supply (resulting in the ‘green revolution’), over-population, acid rain, eutrophication, species extinctions and land clearances for agriculture. Governments began to have policies and strategies that required ecological input - whether or not they truly embraced the need for effective action. There was a proliferation of books on ecology and new journals publishing ecological research. The ecologist’s toolbox grew to include mathematical and computer modelling, more powerful data collection and handling methods, technological advances in measurement, analytical chemistry, the use of molecular methods and so on. This growth in activity and output on many streams of enquiry, in turn, led to the development of subdisciplines in ecology, each with its own skill set, specialist knowledge, conferences, journals, jargon (and acronyms!). This diversification also brought a degree of compartmentalisation and a reduction in dialogue among some sub-disciplines. To some extent, that has been countered by the emergence of new sub-disciplines that have bridged former divides: examples would be areas of plant-animal interactions, and the ecology of global change.
And so, to the present. Our textbooks tell their readers what ecology is – or the authors’ impressions of what it was at the time when it was written (ecology text books have great longevity and, as a result, views persist). But ecology will not remain that way.
Ecology not only will continue to change, but it needs to change: to keep up with modern scientific techniques and advances in understanding, to fulfil the needs and expectations of society and to strive constantly to improve in quality. The modern world needs ecology as an essential science that will help us to cope with the problems that we face and create. The question we raised in the introduction to this chapter was whether ecologists as a body of scientists continue to just go with the flow. Will the ecology that our discipline evolves into in, say, 40 years’ time be the best fit-for-purpose? Or should we attempt to manage the change? Let us reflect on the evolution of ecology in a little more detail.
Elton (1927) stated that ‘Ecology is a new name for a very old subject’. Humans have long appreciated aspects of the interactions between organisms and the environment, but without the basis provided by formal study. Early people living within and relying directly on nature developed their own understanding of the natural world. This understanding was not the structured knowledge of reductionist science, with ideas rigorously expressed and tested. There are different types of knowledge and different ways of knowing (Wildman and Inayatullah 1996). Annual changes in the environment and the landscape determined the resources available to our ancestors, when and where they could be found and, as a result, how best to move around in that world. Over time, people worked out how to alter the environment and to control preferred plants and animals, making food more abundant and more accessible. They observed and they tried new techniques; those that were successful were adopted and agriculture evolved, along with the rest of the paraphernalia that goes with Homo sapiens, such as trade, technology, arts, politics, weapons, pollution and environmental change.
Ecology, the science that it is now, derives from two distinct pathways which have, to some extent, coalesced: natural history and demographic modelling.
2.2.1 Natural history
The first pathway to modern ecology can be traced back at least as far as the Greek philosophers Aristotle and Theophrastus and relates to what we might call natural science: a desire to understand the way the world is, the laws of nature.
Early philosophers certainly understood that different species inhabited different environments (later to be reflected in our concept of the niche) and responded to both climate and soil. They also saw that this knowledge could be applied for the benefit of food production. They were also aware of the diversity of organisms and the fact that some organisms were similar to others. Their attempts to understand the natural world, however, were based on simple observations, their version of logic and their attempts to draw inferences.
After a period of relative quiescence, the description of the natural world and the search for explanations were revived in the Middle Ages as travellers began to describe what they saw in different regions and to search for commonalities and for explanations for what lives where: notable among these were von Humboldt, Darwin and Wallace. Others received information and specimens from these travellers, catalogued and classified them, such as Ray and Linnaeus, building a body of knowledge capable of a more methodical (systematic) analysis. Ultimately, their activities led to the establishment of research areas familiar to ecologists today, such as biogeography, evolution, systematics, phytosociology, biodiversity and macroecology.
A great deal of what we see practised in ecology today is clearly a development of early natural history – the observation of particular organisms in their environments – but now using the methods of science to record, analyse and explain. The diversity of the natural world means that we will never be lacking for case studies that have never been investigated previously. Natural history has always fuelled ecology (Anderson 2017), providing the basic information on which ideas could be formed. It became a common amateur pastime among the middle and upper classes of Europe, reaching its height in the Victorian era. Observational natural history is still a popular pursuit, existing alongside increased public concerns about the environment and conservation. Indeed, the National Audubon Society in the USA and the Royal Society for the Protection of Birds in the UK each have over one million members. This resource has been tapped increasingly, with large numbers of observers collecting useful data combined with the spatio-temporal locations, thus acting as ‘citizen scientists’.
When Elton referred to ecology in 1927 as ‘scientific natural history’ he was referring to the fact that scientists were, by then, collecting natural history data in a quantitative and systematic way and searching for explanations and generalisations. As Simberloff (2013) observes, Elton was not using other scientific approaches in his own work, such as hypotheses and experiments, but by that time the use of experiments was firmly established (as in the work of Darwin and others: see Hector and Hooper 2002) and the formal methods of statistics were starting to become popular.
2.2.2 Demography and modelling
The second pathway to modern ecology was fuelled by an interest in animal life histories, reproductive rates, ages at death and population growth curves – things today that we might group under the term ‘demography’ (see Hutchinson 1978). This arose primarily from the study of humans: lifespans affected calculations for insurance purposes and informed economic policies – as explored by Malthus (1798) in relation to policies regarding aid to the poor. Calculations could be made using census and church records. (There were also religious discussions regarding the age of the earth (by Ussher and others) based on the Bible as a source of evidence.) It was natural to extend demographic calculations to include other animals (see Egerton’s 1968 account of van Leeuwenhoek). It became clear that there were natural checks on population growth rates that, in time, themselves became the targets of investigation.
It was but a small step from there to the use of the data to model population dynamics. The classic work on mixtures of species by Lotka and Volterra in the 1920s and Gause in the 1930s and spatial populations by Fisher in the 1930s led to modelling of ever greater complexity. Coincident with this was the development of population genetics and evolutionary theory, championed by Wright, Haldane and Fisher. Alongside these theoretical studies, there were repeated attempts to test the model predictions on experimental systems (see Chapter 8). Like other areas of research, this theoretical work expanded, became ever more complicated, bringing in new approaches and enabled by dramatic advances in computing technology. Two modern examples are the exploration of population genetics at invasion population margins and the conditions needed for persistence of competing species. There is now a vast literature on what became referred to as theoretical ecology.
The empirical side of this work expanded to include the task of measuring and comparing demographic rates (life history parameters, life table analysis) among species and trying to understand the evolutionary causes of those differences. However, it took until the 1960s and 1970s for plant ecology to adopt demographic approaches – which it did in a major way, championed by Harper. It was realised that these demographic data could be incorporated into models that would predict the likely dynamics of species under different circumstances and, through various types of sensitivity analysis, could be used to derive advice for the conservation of rare species and the management of pests.
2.2.3 Coalescence of natural history with demography and beyond
By the time that Haeckel (1866) gave ecology its name, ‘ecological’ research had already achieved momentum, following the conclusions of Darwin and Wallace. Many natural philosophers were beginning to see the powerful ramifications of natural selection for biology, philosophy, society and religion. The key concepts at the time were the ‘struggle for life’, ‘natural selection’ and ‘evolution’ (Allee et al. 1949; Egerton 2013). Haeckel’s publication was an attempt to reorganise zoology according to the ideas of Darwin, which effectively brought together the two fields of natural history and demography, expanding ecology’s capacity for investigating phenomena. And, over the next 150 years or so, the size of the cornucopia of ecological data accelerated – along with an increase in our frustration to find definitive explanations for so many different things. Ecologists came up with a plethora of concepts, through which they could assemble data, but very few laws and generalisations emerged (Lawton 1999).
Not only did the total quantity of ecological research expand, but it rapidly expanded into new areas of enquiry, fed at first by advances in chemistry, physics and biology - and by physiology in particular. In addition to populations and communities, ecologists had begun to study ecosystems – both empirically and using models. Interest in the environment had grown into a discipline in its own right and, over time, would lead to concepts such as ecosystem services and bioremediation, along with increasing interest in urban ecology and the impacts of unrelenting global change in atmospheric gases, temperatures, sea levels, land use and deforestation. Economics began to be seen as a natural partner for ecology, allowing us to explore some of the social implications of ecosystem management and providing us with a way to converse more effectively with policy-makers and managers. New techniques emerged, such as molecular ecology, offering us the possibility of answering questions that had previously been beyond our reach. Although ecologists had been working on pest and conservation issues for many years, pressure increased for ecologists to focus on useful outcomes. And we began to realise that we were missing entire pieces of the ecological jigsaw puzzle, such as the social components of systems and the social implications for our actions. So, we welcomed these into our discipline. Had ecology grown too broad?
Ecology does not just expand. During its lifetime, some of ecology’s most favoured topics for debate and research have waxed, but then waned. There are too many topics to list, but examples are (1) the individualistic continuum concept of communities, (2) the regulation of population abundance, (3) keystone species in communities and (4) species coexistence. New topics may arise due to a new concept or approach, a novel result for which interesting explanations can be explored, or simply a new technique that can be applied to a variety of study systems. Each topic can stimulate a number of activities that may last for a decade or two. Thereafter, interest declines as attention moves on to other, newer topics. A topic may reappear because advanced techniques develop, allowing us to answer previously unresolvable questions (e.g. the resurgence of interest in population genetics with gene sequencing) or because a new context arises (e.g. phenology in the context of climate change). But, overall, the picture of ecology is one of expansion. A rapid increase in knowledge calls for synthesis, particularly to assist new generations entering the discipline.
In a review of Krebs’ (1972) textbook on ecology, which was a basic text for ecology worldwide for decades, Futuyma (1973) expressed an opinion that ecology was a ‘nebulous science’ that lacked a focus and meant different things to different ecologists. That multiplicity does not, however, mean that ecology is weak.
A science that has expanded so quickly and in so many directions can be viewed from many perspectives. Just because some ecologists chose to focus on ecosystem processes, and others focus on theory, population dynamics, or conservation, it did not mean that these views were in conflict. They are just different, as we might expect to find a variety of viewpoints or areas of emphasis within the sciences of physics or biology. The diversity does, however, require that ecologists make special effort to communicate across specialisations rather than only within their own silo.
One modern trend is an increase in the proportion of ecological studies designed to investigate management issues. At the same time, there has been an acceptance that topics such as wildlife management and agriculture are bona fide fields of research for the ecologist (though barriers still exist). Although ecologists have a long history of involvement in conservation, pest management and reclamation, many prestigious journals chose to focus on ‘fundamental’ ecology. Some of the fundamental ecological work on population dynamics of interacting species, for example, was inspired and funded because of pest management, although the papers’ language did not reflect it. In contrast, there is now often a pressure from editors to comment specifically on the applied significance of research (with the risk of the applied relevance being over-stated). However, this has not necessarily resulted in an overwhelming change in what we do. Carmel et al. (2013) considered less than 20% of ecological studies as ‘problem-solving’, suggesting that despite a greater interest in applied ecology, it was far from dominant.
Another trend is the number of calls for more interdisciplinarity in ecological research (Reyers et al. 2010): for example, the human component of ecosystems and the necessary decisions requires us to consider the social systems in which scientific issues are embedded. There are some barriers to this, including the structures of our funding systems (Bromham et al. 2016) and the rewards for researchers (Goring et al. 2014). Nevertheless, Anderson et al. (2021) concluded that ‘Within eight decades, the field (ecology) has evolved from focusing on natural history and observational field studies to a multidisciplinary field more applied and quantitative in scope’ (see also Nobis and Wohlgemuth 2004).
In apparent conflict with that conclusion, Carmel et al. (2013) found that two-thirds of ecological activities were still of individual species studied using a single discipline. Descriptive studies using data exploration methods still represented over half of published papers, with only one-third experimental studies. Although that study was almost a decade before Anderson’s (but contemporary with Nobis and Wohlgemuth’s), there is little to support a conclusion that ecology has, indeed, undergone a radical change. The Anderson et al. study was a culturomic analysis based on the terminology that we use in papers and the things we say, rather than an analysis of what we actually do.
While ecology may now be more diverse (a much ‘bigger tent’), embracing more spheres of research than it once did (Craven et al. 2019) and we more readily refer in our work to papers from other disciplines (see also Gates et al. 2019), there is little evidence that we are bringing those disciplines together effectively in the performance of the research itself. We have a way to go before we can claim to be an interdisciplinary science.
2.3 Selection in science space
Ecologists are familiar with the concept of the niche as a hypervolume of many dimensions that together describe the set of conditions under which a species can exist.
We can easily extend this idea to consider the underlying conditions present in the entire universe – not just in biology - as a hyperdimensional set of physical rules and relationships that together dictate what can and cannot occur: we might call this ‘factspace’. However, the exploration of that universe, in which we attempt to develop knowledge about that factspace, is a human endeavour and therefore involves social dimensions. It is both aided and constrained by the ways in which humans think, feel and behave.
What we will call ‘science social space’ consists of many dimensions determined by all the social factors that can influence its outcomes: people, groups, communication, ecological constructs, investigative methods and so on (Box 2.2) – in addition to the characteristics of the universe.
The evolution of ecology as a discipline results from the actions of individuals, groups and organisations acting in that space, and we can consider the career of a researcher as a unique trajectory through it, though it is linked in both direct and indirect ways to the trajectories of other ecologists.
Influenced by our interests, past experiences, education, training, opportunities and relationships with others (including the norms of the discipline), we may move towards that part of science social space that is ecology. Once in that social space, our postgraduate studies continue to depend on many factors within science: marks, an inspiring lecturer, a supervisor with an available place, a scholarship; and beyond: place, attachments, hobbies, personal relationships (Manzi et al. 2019). Thereafter, the work we do, the papers that we publish, the papers we read, the conferences we attend, the places we visit and the people we meet all affect the trajectory we follow within the social subspace that is ecology.
The multidimensional science social space we explore can be defined by a number of factors. These include:
-
People: students, researchers, managers, politicians, citizens, industry directors…
-
Collectives: collaborative research teams, schools, faculties, organisations…
-
Social constructs: values, identity, attitudes, motivations, intentions, biases, emotions, norms…
-
Communications: lectures, publications, conferences, hallway conversations…
-
Ecological constructs: concepts, theories, models, diagrams…
-
Investigative methods: field observations, measurement, experiment, synthesis, meta-analysis…
-
Context: culture, place, date…
-
Resources: facilities, grants, contracts, scholarships…
- Environment: the characteristics and underlying laws of the universe which will determine organism behaviour.
One way to understand the trajectory of an ecologist in science social space involves the overt recognition of ecologists as being part of a social network, similar to the way that ecologists themselves build an agent-based model of a group of interacting animals. However, we need to incorporate individual motivations (other than merely access to resources, mates and avoidance of predation) – for example, values which may influence who people interact with and the nature of their relationships to others.
The direction of each step taken by an ecologist depends on individual motivations, values, interests, the local availability of resources (grants, students, ideas), the opportunities for resources elsewhere and the feasibility of moving from the current ‘location’ in science social space to another. Access to resources depends on the relationships (in science social space) with other individuals, who compete, stimulate and provide social context. The ability to move from one area of science to another depends on social relationships, technical and cognitive abilities and desires, the current distribution, motivations and expectations of other ecologists, and serendipitous events (such as hearing about a job application from an acquaintance).
The resulting trajectory of a researcher may not resemble a ‘simple random walk’ (Figure 2.1). Success – perhaps measured in terms of research outputs - in one region of science social space will increase the tendency to remain in the same location or to continue moving in the same direction (i.e. a correlated walk). New advances may come from breaking away from a strong paradigm and looking afresh (‘separation and reintegration’: Koestler 1959), but moving may carry a greater risk of failure to continue in a strong scientific career. Trajectories are drawn towards spatial attractors in the social landscape, such as calls by funding agencies for specific proposals, which may be influenced by broader social and cultural trends towards or away from particular ideas, such as resilience. As mentioned, popularity is also a powerful attractor. There are also barriers to movement (Börger et al. 2008), such as those imposed by employment. It is easy to appreciate, then, why ecologists become aggregated in particular regions of the scientific landscape.
As with a dispersing organism, an individual research trajectory can exhibit a trend even if it is not goal-orientated. This correlated pathway is still likely to be perturbed by the whims of reviewers, funding agencies and employers, and it may end abruptly or take a sudden turn. In a ‘correlated random walk’ through research, it may be difficult to distinguish among (1) slow but steady progress towards a major breakthrough, (2) tying up loose ends at a fine scale to complete a story or (3) being in a rut that is hard to escape.
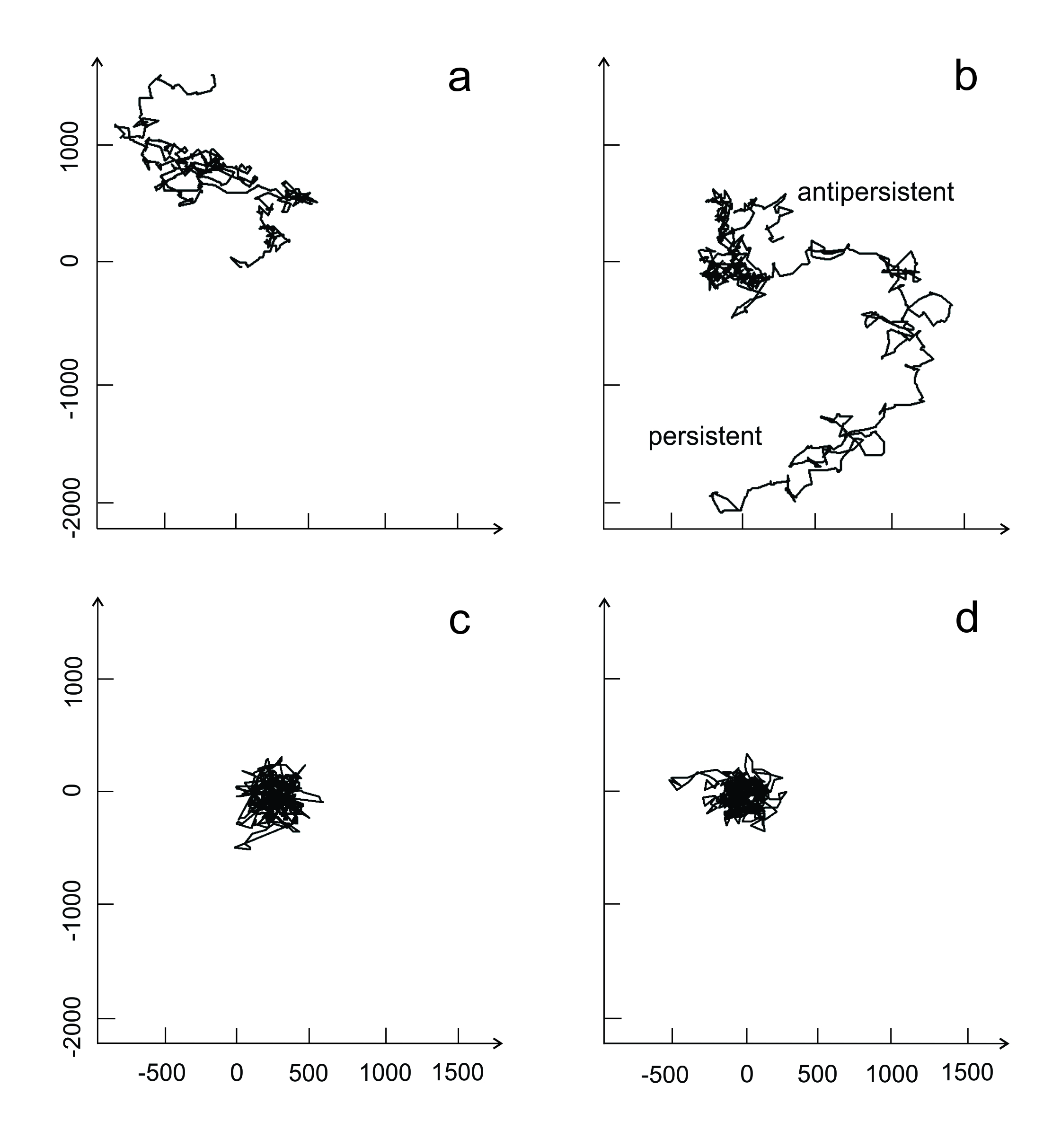
Figure 2.1 Examples of random walks of organisms varying in the constraints to movement that are incorporated. Redrawn from Börger et al. 2008. (a) Simple unconstrained random walk; (b) correlated random walks of two types; (c) simple correlated random walk with simple long-term memory; and (d) a biased random walk.
Considering this view of multiple (and connected) individual trajectories in science space, we can appreciate how selection can affect not just which scientists are successful in their careers, but the movement of a population of scientists or their ideas, causing them to congregate in particular regions, through what Richard McElreath in a lecture called the ‘population dynamics of how we discover things about the world’.
It is not just researchers who have trajectories in science space. Consider the evolution of an idea or ecological concept (and using appropriate evolutionary terms as analogies).
We start with a background of variation among ecologists and ecological concepts, reflecting a diversity of traits. Novel ‘mutations’ arise, as when a new concept, theory or technology arrives, often arising out of previous ideas by synthesis rather than novel inspiration. The success of these ideas and approaches can be considered in terms of contributions to ecologists’ ‘fitness’: our survival as scientists depends on how good we are (subject to chance events), and social biases that privilege some over others. Our best ideas proliferate (reproduce) through publication and translation of our findings to broader audiences, adoption by colleagues and civil society and the success of our students. The models that are used to explore the population genetics of organisms under different conditions can be used to investigate the development of knowledge under different sociological and management regimes and the selection pressures they impose: (McElreath and Smaldino 2015; Smaldino and McElreath 2016).
Many changes in scientific methodology can be considered adaptive in that they improve the quality (‘fitness’) of the knowledge generated, at least in the short term. Examples include the arrival of electronic data-loggers, personal computers or genetic sequencing. Other changes may be more akin to genetic ‘drift’: someone does something first and the idea catches on, not because it is especially beneficial but because it is appealing and becomes popular (Abrahamson 2009). Other changes may seem merely superficial (equivalent to changes to the phenotype rather than to the genotype), as when we re-package research to appeal to different funding agencies.
Despite the virtues of peer review, some changes may actually be ‘mal-adaptive’, in that they set the discipline back. Similarly, we may inadvertently accumulate the equivalent of ‘deleterious alleles’, the bad habits that we have failed to recognise or failed to correct (such as common statistical errors). A final comment on this analogy is that, like the evolution that we see in organisms, the evolution of ideas and knowledge in science occurs at a variable rate, perhaps resembling a series of ‘punctuated equilibria’. Periods of slow development based on ‘additive variation’ (the ‘normal science’ of Kuhn 1970) may be interspersed by occasional selection for novel ‘mutations with major effect’.
All the changes described here, the trajectories of individual researchers or of groups, and the selection and fitness of methods or ideas can be seen as movement through ‘science space’, almost always with a random component, but with constraints, so that the movement does not completely resemble the random walk as a null model. In fact, it is our contention that the trajectory of ecology as a hard science can be made more direct and more effective if we work together to change the way the science is done. The conceptual model of science space is useful for organising our thoughts, and in the next section, we describe and explore an alternative.
2.4 The evolution of Ecology as a multilayered network
Let’s start again from a different viewpoint. Many of the systems that ecologists study are best treated as networks; a network is a structure consisting of nodes (shown as points) with edges (shown as lines) joining the nodes in pairs as in Figure 2.2 (see Dale and Fortin 2021). Think of the trophic network of an ecosystem or, for greater simplicity, the mutualistic network of flowering plants and their pollinators: in Figure 2.2, the nodes are species and the edges are the pollination interactions.
Usually a network is more than a simple graph of nodes and edges because the nodes have labels or magnitudes and the edges have categories, quantitative properties of rates or capacities, or even equations describing the dynamics. A network, therefore, has properties related to composition (the identities of the nodes and the categories of interactions between them), structural properties determined by which nodes are joined by what kinds of edges, and functional properties related to the dynamics of the relationships determined by the edges and the interactions that result. A network may have dynamics on it or in it, with the structure of the network unchanged (think of trains running on a railway), but often a network has the dynamics of structural change as it evolves through time (think of a river system changing course through floods and droughts).
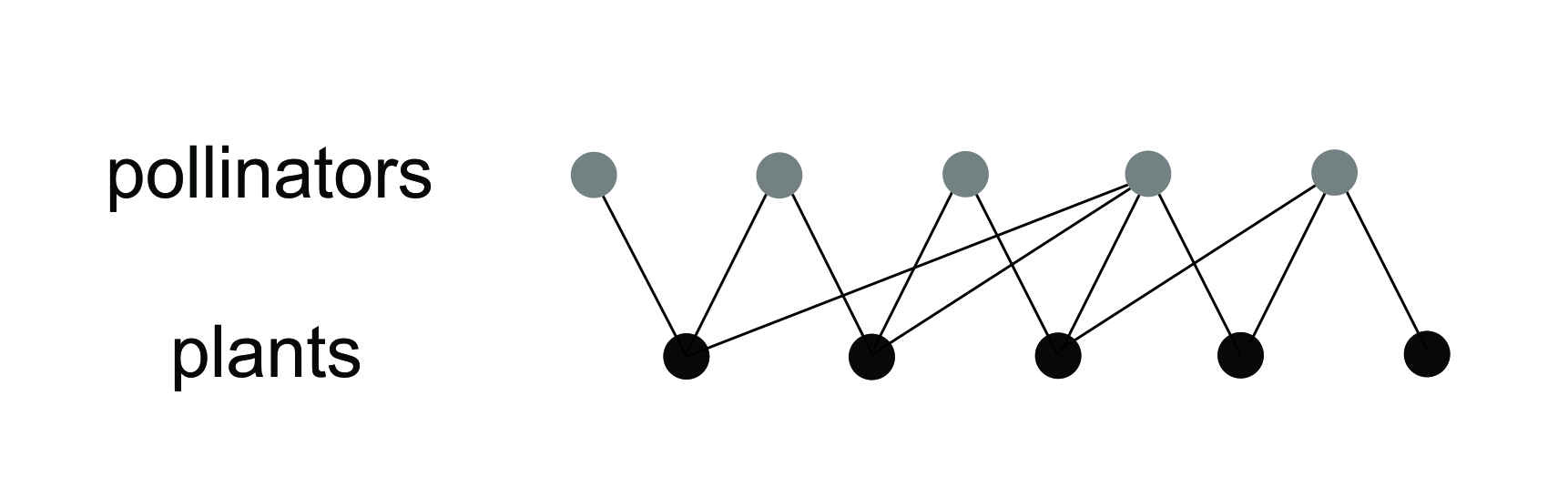
Figure 2.2 A simple ecological network of pollination interactions. Five pollinator species interact with five plant species, but different pollinators visit different plants. Modified from Dale and Fortin (2021); reprinted with permission.
Ecologists study many kinds of natural systems that are often portrayed and studied as networks, but the approach we explore here is to consider the science of Ecology itself, including all its components, as a network. In this network of Ecology, there are nodes representing ‘things’ of different kinds (people, theories, concepts, experiments, publications…) and edges between nodes representing a range of relationships among the nodes (learning, collaboration, creation, modification, synthesis, management…). There are two main features of this representation: it is best structured as a multilayer network (see Bianconi 2018), and it is in many ways a complex multilayer network.
In many applications of networks, the nodes can be assigned to layers, whether by location or node category, producing a multilayer network which can be seen as a series of subnetworks, one on each layer (as in Figure 2.3). A review of ecological networks will show that almost all are essentially multilayer, with several interaction types, interactions that vary in space or time, or interconnected systems forming networks of networks (Pilosof et al. 2017). The multilayer property can arise in ecological networks in different ways. For example, when the same interaction network (e.g. pollination mutualism) is observed at several different times, each time period in the figure is a layer with (mostly) the same nodes in each, but with differences among the edges within each layer. The same structure may arise from studies of the same ecological interaction network at several locations (Bianconi 2018). In this kind of multilayer structure, edges between layers may indicate the persistent identity of individual nodes or something different such as causal relationships. When the layers are generated by space or time, their order is obviously of significance; in other circumstances, the order of the layers can be arbitrary or merely of convenience.
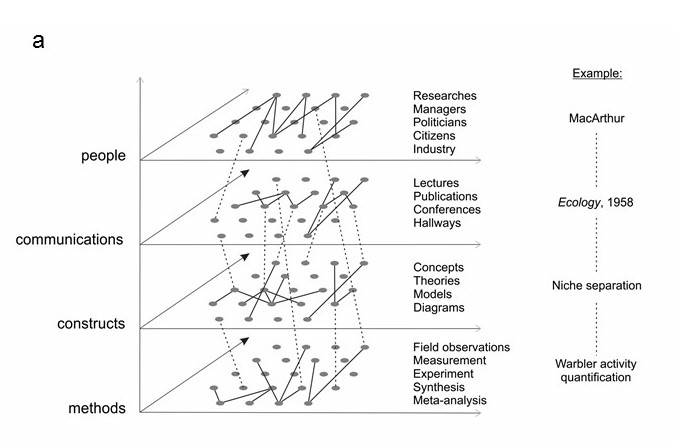
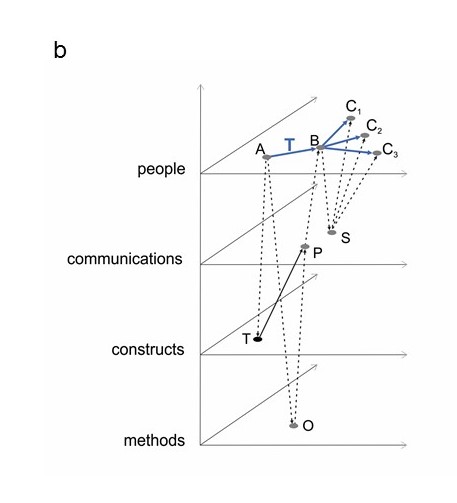
Figure 2.3 (a) The science of Ecology is depicted as a multilayer network. Each layer has its own components. Solid edges are interactions within a layer and their nature will vary depending on the layer. The dashed edges are interactions between layers and will also vary. For example, the researcher MacArthur created the publication in Ecology which described the concept of niche separation, and that concept was based on observation and quantification of warbler behaviour. (b) The layers active in the development and communication of a theory by one researcher through a publication to another researcher and thence to colleagues. See text for details.
In the network that is the science of Ecology, the nodes can be classified by their type (people, concepts, theories…) and so an obvious suggestion is to treat it as a multilayer network, with the layers defined by the categories to which the nodes belong and the kinds of interactions that occur (Figure 2.3a). Using this structure allows greater clarity in identifying the most critical nodes and interactions. For example, Figure 2.3 (b) illustrates the layers active in the development and communication of a theory by one researcher through a publication to another researcher and thence to colleagues. A and B are researchers, with three colleagues, C. The blue arrows show the communication of theory T from one person to another. The dashed arrows between layers show different effects. A develops theory T and makes observations O; T and O inform the publication P. Researcher B learns about theory T from A’s publication P and presents the ideas in seminar S. Seminar S affects the colleagues C, as they learn about theory T from it.
The idea of a science and its context as a complex network is not really new. Networks of researchers with edges defined by collaboration or co-authorship are frequently used as textbook examples of social networks (e.g. Newman 2010), usually including only the researchers as a kind of society. Halpern et al. (2020) illustrate the inverse of that approach, showing the identifiable clusters in a network of publications based on the authors.
Networks depicting subfields of a science as nodes with weighted edges between them have already appeared in the literature (e.g. Herrera 2010). Similarly, Dale and Fortin (2014, figure 12.9) used a network depiction to show the relationships among methods of spatial analysis (or among quantitative methods for network analysis (Dale and Fortin 2021, figure 5.3). Even the multilayer aspect is not new; for example, Omodei et al. (2016) used a separate layer for publications in each subfield of physics, and Meleu and Melatagia (2021) used three layers for publications, based on authors, laboratories and institutions. With layers representing time slices, Boekhout et al. (2021) studied mobility in co-authorship networks by counting identified categories of multilayer ‘temporal motifs’, which are small subgraphs of identifiable structure that are frequent structural components of the network.
The ‘concept’ or ‘construct’ layer of our multilayer figure could start as the familiar ‘concept map’ used to organise ideas in a diagram that depicts the relationships among them; it is a common device in both classroom teaching and brain-storming workshops. There is also a close relationship with the semantic networks or ‘knowledge graphs’ in information science (James 1992), originally maps of words but now used to depict networks of entities and their relationships (Kejriwal et al. 2021), perhaps embedded with their related concepts (Guan et al. 2019). The entities in a knowledge graph can include people, places, products and so on, but they are not organised into layers and the knowledge graph does not actually contain the knowledge that flows in the collection it depicts. On the other hand, in the ‘Ecology network’ shown in Figure 2.3a, paths of links joining ‘MacArthur’ and ‘island biogeography’ would both include and go through the relevant publications and communications and their contents, so that the information is actually included in the structure.
So, what does the Ecology network do? It discovers and creates knowledge through observation, experiment, analysis and synthesis, and it organises and communicates that knowledge, advancing some forms of knowledge over others depending on the social trends, values and norms at the time.
Ecology is a science that studies complex systems and complex networks, such as those just described, but it is also true to say that the science of Ecology is itself a complex multilayer network with many of the properties mentioned in Box 2.2. There are layers associated with people: researchers, managers, funders, politicians, citizen scientists… There are layers associated with the development of the science itself: observations, concepts, theories, methods, techniques, models… (Figure 2.3a). There are interactions between these two kinds of layers: publications (Figure 2.3a), presentations, conferences, courses… What about layers of resources? Consider: universities, salaries, research grants, lab facilities, field stations… Each of the layers has nodes representing the appropriate structural units, edges within layers representing intra-layer relationships and then the complex web of interactions between nodes in different layers. There is complexity also in the dynamics of the network: disorder; non-equilibrium; feedback loops; history, memory, and adaptive behaviours; and self-organisation and stability to some forms of perturbation. The balance or imbalance of these various dynamic characteristics can lead naturally to the evolution of the system through time.
All this complexity of the science itself, in addition to the complexity of the living systems which we study, is another factor that makes this science hard.
A corollary of the statement that the science of Ecology is a complex network is that it is evolving towards even greater complexity. The units in the layers become more numerous with time, and with more connections between units, whether within or between layers (see Halpern 2020, figure 5 as an example). It is easy to see that we may also acquire more layers.
Complexity is a concept that has different implications depending on the system being studied and whether the focus is on structure or on function (or on both), but there are a number of characteristics that can be associated with it (see Box 2.3).
Ladyman and Wiesner (2020) consider complex systems generally and provide the following list of ten features – under three headings - that may be either sufficient or necessary for a system to be complex:
-
Structural: Numerosity (many units: nodes or edges); disorder and diversity; nestedness and modularity.
-
Short-term dynamics: Feedback loops; non-linear responses; non-equilibrium; stable to some perturbations.
-
Long-term dynamics: Spontaneous order or self-organization; adaptive behaviour; memory of past history.
This general list in Box 2.3 can form a background for the more technical and focused discussion of ecological networks that follows. Of note is the fact that ecologists have pursued their own progression in defining measures of the complexity of networks, without relying on some pre-existing work on the topic such as Bonchev and Buck (2005). Part of the reason has to do with the focus by ecologists on the relationship between network complexity and network stability for ecological systems (but only part). Of the many measures that have been proposed for the complexity of an ecological network, a high proportion are based on or related to random walks on the network (Dale and Fortin 2021). Table 2.1 uses the categories from Box 2.3 to discuss the features of the Ecology network’s complexity:
Complexity characteristics of the evolving Ecology network.
Structural
Network size (‘Numerosity’)
Ecology is home to many thousands of ecologists, publications, ideas, projects, approaches, outcomes. Need we say more? Disorder and diversity
What organisation there is in Ecology is not externally imposed but developed within, in response to changing conditions and its history, local and global. The flexibility of disorder is obvious in every layer as well as in the relationships between layers (see Ohlmann et al. 2019).
A key form of diversity in ecological networks is the existence of indirect interactions (see Figure 2.4). Familiar examples may be the ‘trophic cascade’ or ‘apparent competition’. In essence, a network edge can act as a node in a subsequent level of interactions. This form of diversity in ecological networks is one critical factor in how ecological networks function, but it is also a factor in what makes our science hard. In the network that is Ecology, the same phenomenon is very common: direct interactions between pairs of nodes can be modified by the effects of other nodes in the network or by edges, so that network edges act as nodes for the next level of modification. The network that is Ecology has the potential for hierarchies of indirect interactions of various degrees or levels. Clearly this form of diversity contributes greatly to its complexity and also to the detail of how it can evolve.
Nestedness and modularity
All four layers depicted in Figure 2.3a will be modular with clusters of researchers, and publications and overlapping subnetworks of closely related concepts and closely related methodological approaches. There will also be tight inter-layer clustering of researchers with their favourite methods and concepts and then papers and presentations that result (see Costa et al. 2007).
Short-term Dynamics
Feedback loops, positive and negative
As with diversity, feedback loops can be found in every layer as well as among layers. Some of the feedback loops are negative, providing controls to processes and increasing stability, and some are positive, enhancing or reinforcing change. For example, an over-production of PhDs in one subfield may supress employment opportunities and thus its perceived attractiveness to future students. On the positive side, research avenues that succeed can provide increased resources of many kinds that enable further potentially successful research.
Non-linear responses
Equal step incremental changes may produce small effects over a certain range but the same size of change may cause a break-point in behaviour, a flash of insight, or a paradigm shift, depending on the layer in question.
Non-equilibrium
Instability and tipping points are found in the structure of Ecology as well as in the natural phenomena it studies. Small changes that made big differences include the understanding of genetics and heredity, and the development of digital computers.
Robustness, stable to some perturbations
New ideas may destabilise old concept maps or may be absorbed into the existing framework depending on the conflicts or extensions they create (see Barrat et al. 2008). The diversity of all elements of the structure may or may not enhance the stability of the network’s function and we can speculate whether positive interactions such as collaboration enhance both stability and the function.
Long-term dynamics
Spontaneous order or self-organisation
External forces obviously affect the development of the science, but most of the changes as it has evolved through time have been driven by nodes and their interactions both within and between layers. New or refined techniques affect how research is done, the concepts that are generated and the questions that may be asked or answered.
Adaptive behaviour
Having noted that much of the change we can observe in the evolving network of Ecology is self-generated, we also need to acknowledge that its behaviour can also be adaptive, changing in response to prevailing conditions (see Gross and Blasius 2008). This becomes clear in the changing focus of attention from natural processes in natural systems such as disturbance and succession, to landscape fragmentation and conservation, to climate change and the Anthropocene.
History and memory
Nodes and the intra-layer edges in the communications layer of the network will be temporal in nature and thus the history aspect of the structure can be explicit. The elements in other layers may be closely tied to a flow of historical development and evolution. Memory can be found in all layers, and it would be interesting, if possible, to contrast the lines of memory in the layer of concepts or constructs with the memory made explicit in the communications layer. The memory shown by citations may sometimes prove to be too short-sighted. Knowledge requires the system’s memory, but it is imperfect.
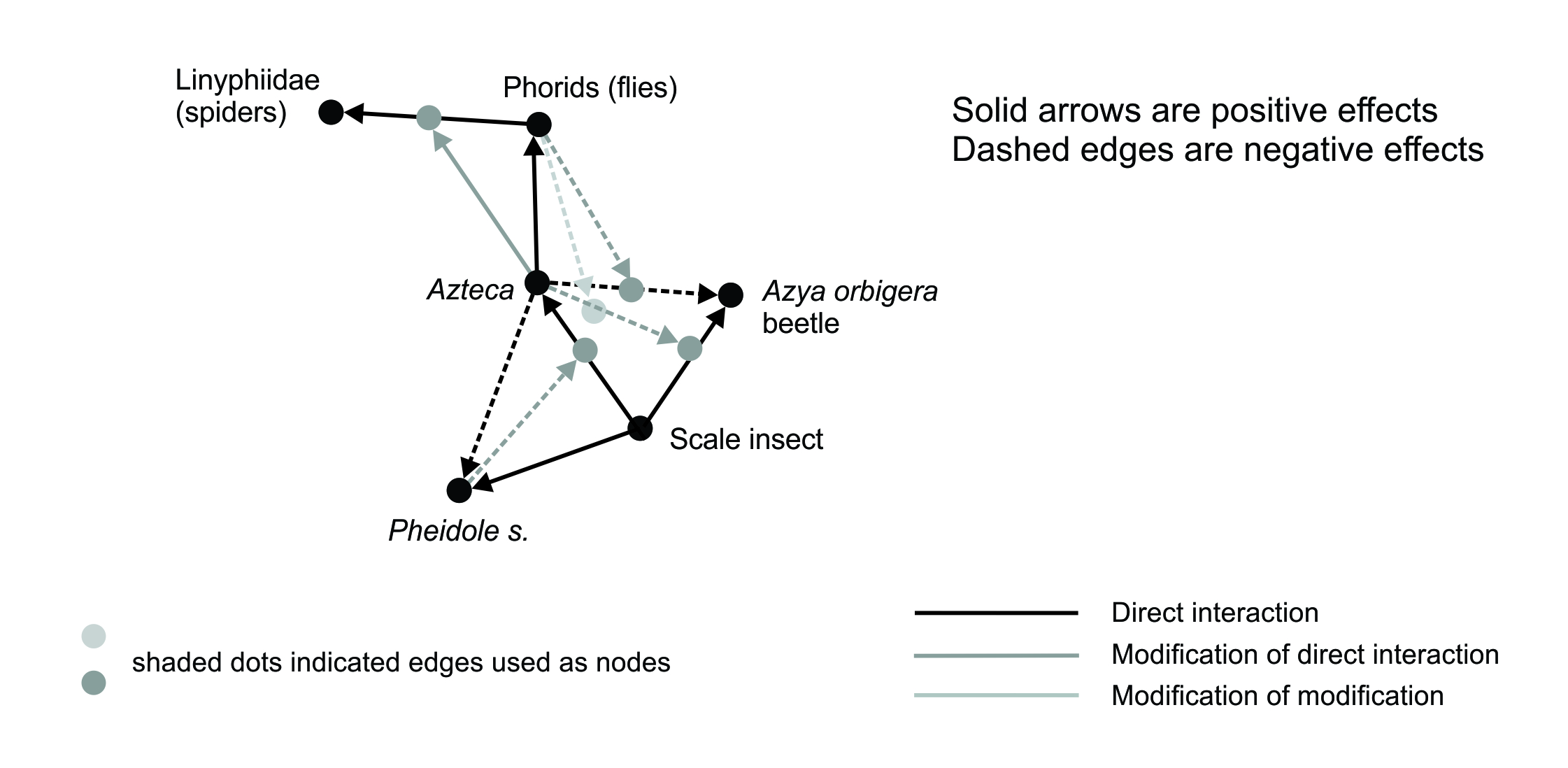
Figure 2.4 Indirect effects in an ecological network: two levels of modifications of ecological interactions. Part of a coffee agro-ecosystem, showing a hierarchy of interaction modifications: edges at one level become nodes for edges at the next. Azteca and Pheidole are ants. Modified from Dale and Fortin 2021; reprinted with permission.
The challenge of the network model is to make sense of its complexity, the opportunities for synthesis as well as critique and critical thinking. Complexity of our systems is one factor that makes the science hard. We already are familiar with how to understand some of the characteristics of a single layer (e.g. the studies of co-authorship relations and evolution such as Barabási et al. 2001), but we need to extend this to the many layers and the edges between them. There are at least two features of the multilayer networks that will be helpful in dealing with the potentially overwhelming complexity. The first is the existence of small structurally defined subgraphs, motifs or graphlets, as an aid to summarising small-scale or local aspects of structure and its consistency. This is complemented by the tendency for many of the individual layers to have structural hierarchies of nestedness or modules (or both). The second is the redundancy of information in separate layers. For almost any network, the density of edges (‘connectance’) has implications for its function but usually ‘connectivity’ (how difficult it is to disconnect) is more critical to function and resilience. Connectivity may manifest itself in the form of redundancy of information in different layers. For example, the relationships among concepts in a cluster centred on niche separation will also be reflected in the relationships such as paper citation among publications in the ‘communications’ layer, and in the clustering of like-minded authors in the ‘people’ layer. De Domenico et al. (2014) describe a method to aggregate the information in different layers, which was designed for multiplex networks (the same nodes in all layers), but may work for multilayer networks with different node sets in different layers.
2.5 Evolving space for evolving Ecology
If Ecology is treated as a multilayer network, as the science evolves, the network evolves in a non-random way, based on the mutual influence of adjacent nodes, rather like the diffusion of information through the edges.
We might imagine at first that this network is embedded in the same n-dimensional contextual space of time, physical space, conditions, and resources, but unlike our individual researcher described in Selection in Science Space in this chapter, the Ecology network is not in that same ‘science space’; in fact, it is conceptually difficult to see how it could be. The layers constrain the structure although we draw them as parallel planes. Similarly, the indirect interactions among k species won’t fit into the k-dimensional species density space. Fortunately, this is not a problem because any graph or network creates its own space (Dale and Fortin 2021). This means that as the network evolves, so does the space within which it is embedded. The co-evolution of the embedding space is an important aspect of spontaneous order or self-organisation recognised as a key feature of complex systems. That, in turn, will affect our view of what the evolution of this network is like; it is definitely not like a line of organisms evolving in response to their environment and interactions.
We suggest that this approach resembles using structure as surrogate for a process like diffusion and using a conceptual process like diffusion to understand the structure. As often in the study of graphs, this ties closely with the concept of random walks or constrained random walks (on a graph or in an n-dimensional space), as discussed previously with respect to a researcher exploring science space.
2.6 So what?
Viewing the science of Ecology as a dynamic multilayer network, rather than as an evolving organism, allows more independence to different parts of the endeavour as it develops and helps explain why the science is hard.
However, this view also recognises that indirect influences flow through the network between different and distant projects, whether as directed communication or as a random walk of chance exchanges. The model makes possible the recognition of the different roles of elements in different layers, and of different roles within a single layer (e.g. student versus administrator) while providing scope for the interactions of units within and between layers.
Of course, the network model makes a convenient and helpful metaphor for our science, but someone will undoubtedly ask whether the network might actually be created. The answer is ‘yes’, at least for parts of it, and the knowledge graph approach is proposed as a potential summary of the discipline’s state (Popping 2007). This can be done by applying and developing methods based on those already in existence to create knowledge graphs from very large data. In parallel with our comment about networks (they are used and studied by ecologists, and the science of Ecology is one itself), knowledge graphs have been fundamental to organising and representing scientific information in a structured manner (Kejriwal et al. 2021) and the science of Ecology can also be portrayed as one. Time will tell…
The network model also enables us to appreciate how the diverse parts of ecology and the system of scientific research interact, but also how change might occur within the discipline. In the early decades of the science, there were few practitioners, large regions of science space had yet to be explored and the discipline network had yet to mature. Not to devalue the contributions of our predecessors, but it was relatively easy for the ideas of a few researchers to be adopted and dominate the discipline. Change seems to have occurred readily. Now, with a more established multilayer structure and a greater volume and diversity of research activity, it is much harder for the ideas of even the most innovative of ecologists to percolate through and to have influence over the whole discipline; influence tends to be localised within subdisciplines. This contributes to the frustration of those who believe that there are fundamental flaws in the way all ecology is done and see the need for wholesale change.
From here onwards, change may be slow and limited in scope. Those calling for paradigm shifts by their colleagues, even within a subdiscipline, cannot expect immediate uptake; complex multilayer networks have their own dynamics.
This is not to suggest that change is impossible, just difficult. Changes to nodes and links within the multilayer network can produce worthwhile benefits: examples frequently discussed include changes to research grant allocation systems (Fang and Casadevall 2016), the adoption of interdisciplinary and transdisciplinary approaches (Kelly et al. 2019; Marshall et al. 2018), and the establishment of mechanisms for synthesis and debate (Halpern et al. 2020; Cousens 2017) workshops and working groups. Improvements at the level of individual ecologists through modified education, training and quality-assurance systems will be beneficial (to address known common errors and weaknesses).
The multilayered network that is Ecology contains little in the way of overall governance or management. No node has individual responsibility for improving the whole network. As scientists, we all share that responsibility but as individuals have little power or authority. So, we must encourage the academy to take up the best suggestions: waving flags, writing papers, introducing local innovations and hoping that they catch on.
In this chapter we have presented two views or two models of what ecology is like and how it evolves - both useful, both interesting. The first model, of selection acting on individual ecologists, emphasises the importance of the traits and context of the researcher and their research projects in multidimensional science space. The second model presents Ecology as a multilayer network that defines its own space. That network is complex and that complexity is one feature that makes our science hard. The network model emphasises the diversity of nodes and their relationships, accommodating the frequency and importance of indirect interactions, and the potential for hierarchies of indirect interactions of various levels. Basic network analysis would suggest that because its function is knowledge discovery, communication and synthesis, it will do better with more links, more communication and more collaboration.
2.7 References
Abrahamson, E. 2009. Necessary conditions for the study of fads and fashions in science. Scandinavian Journal of Management 25:235-239. doi:10.1016/j.scaman.2009.03.005
Allee, W. C., A. E. Emerson, O. Park, et al. 1949. Principles of animal ecology. Philadelphia: W.B. Saunders.
Anderson, J. G. T. 2017. Why ecology needs natural history. American Scientist 105:290-297. doi:10.1511/2017.105.5.290
Anderson, S. C., P. R. Elsen, B. B. Hughes, et al. 2021. Trends in ecology and conservation over eight decades. Frontiers in Ecology and the Environment 19:274-282. doi:10.1002/fee.2320
Barabási, A. -L., H. Jeong, Z. Néda, et al. 2001. Evolution of the social network of scientific collaborations. arXiv:cond-mat/0104162v1. doi:10.48550/arXiv.cond-mat/0104162
Barrat, A., Barthélemy, M., and A. Vespignani. 2008. Dynamical processes on complex networks. Cambridge: Cambridge University Press. doi:10.1017/CBO9780511791383
Belovsky, G. E., D. B. Botkin, T. A. Crowl, et al. 2004. Ten suggestions to strengthen the science of ecology. BioScience 54:345–351. doi:10.1641/0006-3568(2004)054[0345:TSTSTS]2.0.CO;2
Betini, G. S., T. Avgar, and J. M. Fryxell. 2017. Why are we not evaluating multiple competing hypotheses in ecology and evolution? Royal Society Open Science 4:160756. doi:10.1098/rsos.160756
Betts M. G., A. S. Hadley, D. W. Frey, et al. 2021. When are hypotheses useful in ecology and evolution? Ecology and Evolution 11:5762-5776. doi:10.1002/ece3.7365
Bianconi, G. 2018. Multilayer networks: Structure and function. Oxford: Oxford University Press. doi:10.1093/oso/9780198753919.001.0001
Boekhout, H. D., V. A. Traag, and F. W. Takes. 2021. Investigating scientific mobility in co-authorship networks using multilayer temporal motifs. Network Science 9:354-386. doi:10.1017/nws.2021.12
Bonchev, D., and G. A. Buck. 2005. Quantitative measures of network complexity. In: Complexity in chemistry, biology, and ecology, eds D. Bonchev and D. H. Rouvray, D.H. (eds), 191-235. Springer: Boston. doi:10.1007/0-387-25871-X_5
Börger, L., B. D. Dalziel, and J. M. Fryxell. 2008. Are there general mechanisms of animal home range behaviour? A review and prospects for future research. Ecology Letters 11:637-650. doi:10.1111/j.1461-0248.2008.01182.x
Brittan, G., and P. S. Bandyopadhyay. 2019. Ecology, evidence, and objectivity: In search of a bias-free methodology. Frontiers in Ecology and Evolution 7:399. doi:10.3389/fevo.2019.00399
Bromham, L., R. Dinnage, and X. Hua. 2016. Interdisciplinary research has consistently lower funding success. Nature 534:684–687. doi:10.1038/nature18315
Carmel, Y., R. Kent, A. Bar-Massada, et al. 2013. Trends in ecological research during the last three decades – A systematic review. PLoS ONE 8:e59813. doi:10.1371/journal.pone.0059813
Carr, A., C. Diener, N. S. Baliga, and S. M. Gibbons. 2019. Use and abuse of correlation analyses in microbial ecology. ISME Journal 13:2647–2655. doi:10.1038/s41396-019-0459-z
Choi, Y. D. 2007. Restoration ecology to the future: A call for new paradigm. Restoration Ecology 15:351-353. doi:10.1111/j.1526-100X.2007.00224.x
Costa, L. S. da F., F. A. Rodrigues, G. Travieso, and P. R. Villas Boas. 2007. Characterization of complex networks: A survey of measurements. Advances in Physics 56:167-242. doi:10.1080/00018730601170527
Cousens, R. (2017) Do we argue enough in ecology? Bulletin of the British Ecological Society 48:58-61.
Craven, D., M. Winter, K. Hotzel, et al. 2019. Evolution of interdisciplinarity in biodiversity science. Ecology and Evolution 9:6744-6755. doi:10.1002/ece3.5244
Dale, M. R. T, and M. -J. Fortin. 2014. Spatial analysis: A guide for ecologists, 2nd edition. Cambridge: Cambridge University Press. doi:10.1017/CBO9780511978913
Dale, M. R. T., and M. -J. Fortin. 2021. Quantitative analysis of ecological networks. Cambridge: Cambridge University Press. doi:10.1017/9781108649018
Dayton, P. K. 1979. Ecology: a science and a religion. In Ecological processes in coastal and marine systems, ed. R. J. Livingstone, 3-18. New York: Plenum Press.
De Domenico, M., V. Nicosia, A. Arenas, and V. Latora. 2014. Structural reducibility of multilayer networks. Nature Communications 6:6864. doi:10.1038/ncomms7864
Egerton, F. N. 1968. Leeuwenhoek as a founder of animal demography. Journal of the History of Biology 1:1–22.
Egerton, F. N. 2013. History of ecological sciences, Part 47: Ernst Haeckel’s ecology. Bulletin of the Ecological Society of America 94:222-244. doi:10.1890/0012-9623-94.3.222
Elton, C. 1927 Animal ecology. London: Sidgwick and Jackson.
Fang, F. C., and Casadevall, A. 2016. Research funding: the case for a modified lottery. Mbio 7:e00422‐16. doi:10.1128/mBio. 00422–16
Fraser, H., T. Parker, S. Nakagawa, A. Barnett, and F. Fidler. 2018. Questionable research practices in ecology and evolution. PLoS One 13:e0200303. doi:10.1371/journal.pone.0200303
Futuyma, D. 1973. Ecology. The experimental analysis of distribution and abundance. Quarterly Review of Biology 48:47-48.
Gates, A. J., Q. Ke, O. Varol, and A. -L. Barabási. 2019. Nature’s reach: narrow work has broad impact. Nature 575:32-34. doi:10.1038/d41586-019-03308-7
Goring, S. J., K. C. Weathers,W. K. Dodds, et al. 2014. Improving the culture of interdisciplinary collaboration in ecology by expanding measures of success. Frontiers in Ecology and the Environment 12:39-47. doi:10.1890/120370
Gross, T., and B. Blasius. 2008. Adaptive coevolutionary networks: a review. Journal of the Royal Society Interface 5:259-271. doi:10.1098/rsif.2007.1229
Guan, N., D. Song, and L. Liao. 2019. Knowledge graph embedding with concepts. Knowledge Based Systems 64:38-44. doi:10.1016/j.knosys.2018.10.008
Haeckel, E. 1866. Generelle morphologie der organismen. Berlin: G. Reimer.
Halpern, B. S., E. Berlow, R. Williams, E. T. Boer et al., 2020. Ecological synthesis and its role in advancing knowledge. BioScience 70:1005-1014. doi:10.1093/biosci/biaa105
Hector, A., and R. Hooper. 2002. Darwin and the first ecological experiment. Science 295:639-640. doi:10.1126/science.1064815
Herrera, M., Roberts, D. C., and N. Gulbahce. 2010. Mapping the evolution of scientific fields. PLoS ONE 5:e10355. doi:10.1371/journal.pone.0010355
Hoekstra, R., H. A. L. Kiers, and A. Johnson. 2012. Are assumptions of well-known statistical techniques checked, and why (not)? Frontiers in Psychology 3:137. doi:10.3389/fpsyg.2012.00137
Hutchinson, G. E. 1978. An introduction to population ecology. New Haven: Yale University Press.
James, P. 1992. Knowledge graphs. In Linguistic Instruments in Knowledge Engineering, eds R. P. Van de Riet and R. A. Meersman, 97-117. Amsterdam: Elsevier.
Keddy, P., and E. Weiher. 1999. The scope and goals of research on assembly rules. In Ecological assembly rules: Perspectives, advances, retreats, eds E. Weiher, and P. Keddy, 1-20. Cambridge: Cambridge University Press.
Kejriwal, M., Knoblock, C. A., and P. Szekely. 2021. Knowledge graphs: Fundamentals, techniques, and applications. Cambridge MA: MIT Press.
Kelly, R., M. Mackay, K. L. Nash, et al. 2019. Ten tips for developing interdisciplinary socio-ecological researchers. Socio-Ecological Practice Research 1:149–161. doi:10.1007/s42532-019-00018-2
Koestler, A. 1959. The sleepwalkers: A history of man’s changing vision of the universe. London: Hutchinson.
Krebs, C. J. 1972. Ecology: The experimental analysis of distribution and abundance. New York: Harper and Row.
Kuhn, T. S. 1970. Logic of discovery or psychology of research. In Criticism and the growth of knowledge, eds I. Lakatos and A. Musgrave, 1-23. Cambridge: Cambridge University Press.
Ladyman, J., and K. Wiesner. 2020. What is a complex system? New Haven: Yale University Press. doi:10.12987/yale/9780300251104.001.0001
Lawton, J. 1991. Predictable plots - A critique for ecology by R. H. Peters. Nature 354:444.
Lawton, J. H. 1999. Are there general laws in ecology? Oikos 84:177-192.
Malthus, T. R. 1798. An essay on the principle of population as it affects the future improvement of society, with remarks on the speculations of Mr. Goodwin, M. Condorcet and other writers. London: J. Johnson.
Manzi M, D. Ojeda, and Hawkins R. 2019. Enough wandering around!’: Life trajectories, mobility, and place making in neoliberal academia. The Professional Geographer 71:355-363. doi:10.1080/00330124.2018.1531036
Marquet, P. A., A. P. Allen, J. H. Brown, et al. 2014. On theory in ecology. BioScience 64:701-710. doi:10.1093/biosci/biu098
Marshall, F., J. Dolley, and R. Priya. 2018. Transdisciplinary research as transformative space making for sustainability: Enhancing propoor transformative agency in periurban contexts. Ecology and Society 23:8. doi:10.5751/ES-10249-230308
McElreath, R., and P. E. Smaldino. 2015. Replication, communication, and the population dynamics of scientific discovery. PLoS ONE 10:e0136088. doi:10.1371/journal.pone.0136088
Meleu, G. R., and P. Y. Melatagia. 2021. The structure of co-publications multilayer network. Computational Social Networks 8:8. doi:10.1186/s40649-021-00089-w
Nelson, R. R. 2015. Physics envy: Get over it! Issues in Science and Technology 31.
Newman, M. E. J. 2010. Networks: An introduction. Oxford: Oxford University Press. doi:10.1093/acprof:oso/9780199206650.001.0001
Nobis, M., and T. Wohlgemuth. 2004. Trend words in ecological core journals over the last 25 years (1978–2002). Oikos 106:411-421. doi:10.1111/j.0030-1299.2004.13496.x
Ohlmann, M., V. Miele, S. Dray, et al. 2019. Diversity indices for ecological networks: a unifying framework using Hill numbers. Ecological Letters 22:737-747. doi: 10.1111/ele.13221
Omodei, E., M. De Domenico, and A. Arenas. 2016. Evaluating the impact of interdisciplinary research: a multilayer network approach. Network Science 5:235-246. doi:10.1017/nws.2016.15
Peters, R. F. 1991. A critique for ecology. Cambridge: Cambridge University Press.
Pilosof, S., M. A. Porter, M. Pascual, and S. Kéfi. 2017. The multilayer nature of ecological networks. Nature Ecology and Evolution 1:0101. doi:10.1038/s41559-017-0101
Popping, R. 2007. Text analysis for knowledge graphs. Quantity and Quality 41:691-709. doi:10.1007/s11135-006-9020-z
Reyers, B., D. Roux, and P. O’Farrell. 2010 Can ecosystem services lead ecology on a transdisciplinary pathway? Environmental Conservation 37:501-511. doi:10.1017/S0376892910000846
Rist, L., A. Felton, L. Samuelsson, C. Sandström, and O. Rosvall. 2013. A new paradigm for adaptive management. Ecology and Society 18:63. doi:10.5751/ES-06183-180463
Scheiner, S. M. 2013. The ecological literature, an idea‐free distribution. Ecology Letters 16:1421-1423. doi:10.1111/ele.12196
Simberloff, D. 1981. The sick science of ecology. Eidema 1:49–54.
Simberloff, D. 2013. Charles Elton. In Oxford Bibliographies New York: Oxford University Press. doi:10.1093/OBO/9780199830060-0090
Smaldino, P.E., and R. McElreath. 2016. The natural selection of bad science. Royal Society Open Science 3:160384. doi:10.1098/rsos.160384
Smith, R. 2006. Peer review: a flawed process at the heart of science and journals. Journal of the Royal Society of Medicine 99:178-182. doi:10.1258/jrsm.99.4.178
Whittaker, R. H. 1962. Classification of natural communities. Botanical Review 28:1-239.
Wildman, P., and S. Inayatullah. 1996. Ways of knowing, culture, communication and the pedagogies of the future. Futures 28:723-740.