Week 2
# This is all copied from the "Import Data" dialog.
cows <- read_delim("input_data/cattle-NamesFemaleCalves.csv",
delim = ";", escape_double = FALSE, trim_ws = TRUE,
skip = 1)
## Rows: 592 Columns: 5
## ── Column specification ───────────────────────────────────────────────────────────────────────────────────────────────────────────────────────────────────────────────────────────────────────────────────────────────────
## Delimiter: ";"
## chr (2): Name, OwnerLanguage
## dbl (3): count, Rank, year
##
## ℹ Use `spec()` to retrieve the full column specification for this data.
## ℹ Specify the column types or set `show_col_types = FALSE` to quiet this message.
cows |>
# I only want these three names
filter(Name %in% c("Bella", "Fiona", "Tina")) |>
# This contains separate data for each language,
# but I just want the "all" data.
filter(OwnerLanguage == "__all__") |>
# I only want the data for the years before 2023
filter(year < 2023) |>
# I only want the columns Name, count, and year
select(Name, count, year) |>
# Now we put everything into ggplot!
# We add aesthetics (aes) to tell ggplot which columns
# to use for x and y, and the color
ggplot(aes(x=year, y=count, color=Name)) +
# We add a line and points
geom_line() +
geom_point() +
# We add a title and labels
labs(
title="The rise of Bella: frequency of cow names 2010-2022",
x = "Year",
y= "Total count"
)
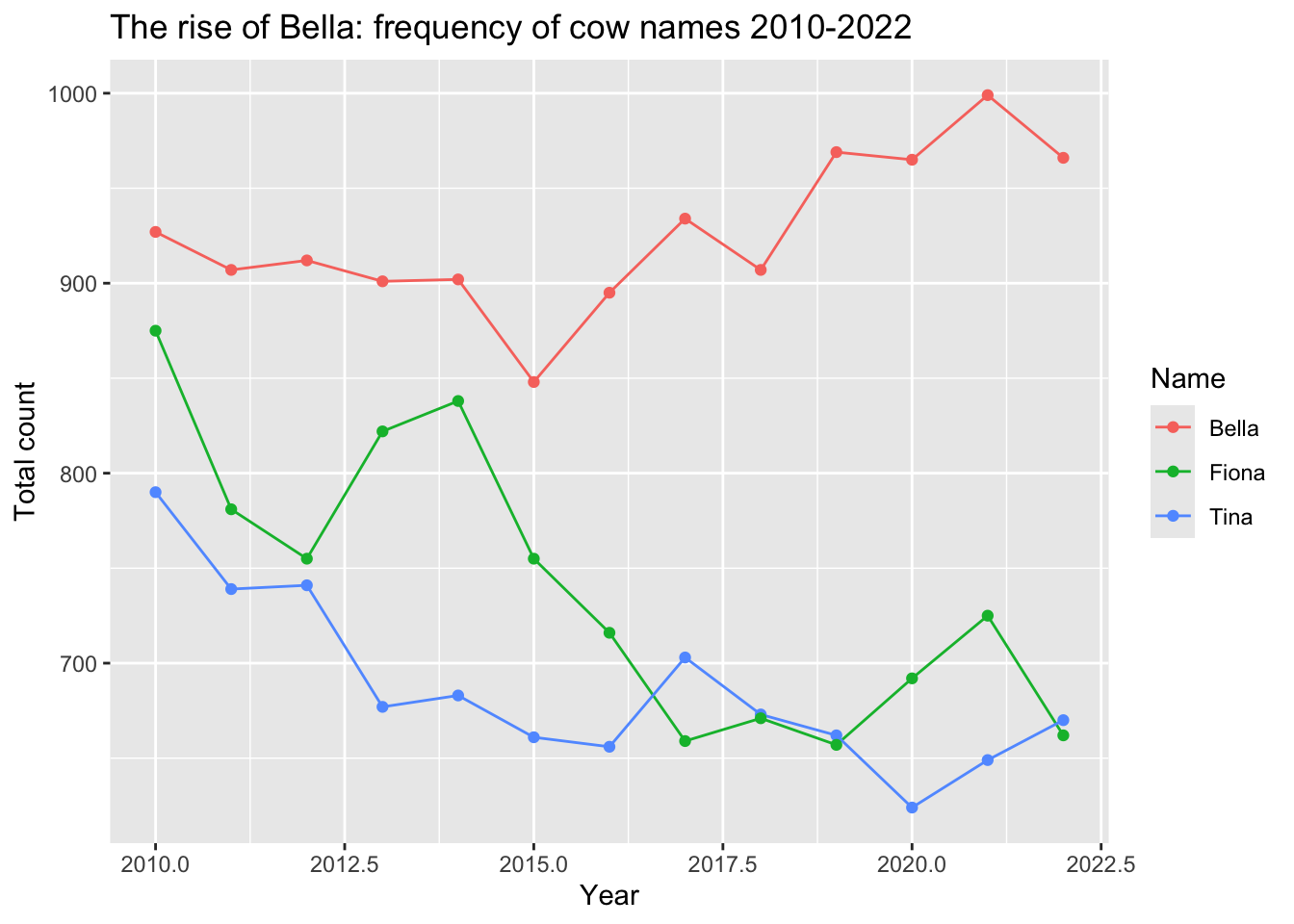
Week 4 Bonus Questions
- Between 2010 and 2020, what were the average number of children born each year in each canton?
births |>
filter(year >= 2010, year <= 2020) |>
group_by(canton_name, year) |>
summarise(total_births_per_year = sum(total_born)) |>
summarize(avg_born = mean(total_births_per_year))
## # A tibble: 26 × 2
## canton_name avg_born
## <chr> <dbl>
## 1 Aargau 6682.
## 2 Appenzell Ausserrhoden 545.
## 3 Appenzell Innerrhoden 175.
## 4 Basel-Landschaft 2532.
## 5 Basel-Stadt 1983.
## 6 Bern 9820.
## 7 Freiburg 3332.
## 8 Genf 5229
## 9 Glarus 382.
## 10 Graubünden 1715
## # ℹ 16 more rows
- Building off this, which years had an above average birth rate for that decade?
births |>
filter(year >= 2010, year <= 2020) |>
group_by(canton_name, year) |>
summarise(total_births_per_year = sum(total_born)) |>
summarize(year = year, total_births_per_year = total_births_per_year, avg_born = mean(total_births_per_year)) |>
mutate(above_average = total_births_per_year > avg_born) |>
filter(above_average) |>
select(canton_name, year)
## Warning: Returning more (or less) than 1 row per `summarise()` group was deprecated in dplyr 1.1.0.
## ℹ Please use `reframe()` instead.
## ℹ When switching from `summarise()` to `reframe()`, remember that `reframe()` always returns an ungrouped data frame and adjust accordingly.
## ℹ The deprecated feature was likely used in the rsconnect package.
## Please report the issue at <https://github.com/rstudio/rsconnect/issues>.
## This warning is displayed once every 8 hours.
## Call `lifecycle::last_lifecycle_warnings()` to see where this warning was generated.
## # A tibble: 154 × 2
## # Groups: canton_name [26]
## canton_name year
## <chr> <dbl>
## 1 Aargau 2014
## 2 Aargau 2015
## 3 Aargau 2016
## 4 Aargau 2017
## 5 Aargau 2018
## 6 Aargau 2019
## 7 Aargau 2020
## 8 Appenzell Ausserrhoden 2015
## 9 Appenzell Ausserrhoden 2016
## 10 Appenzell Ausserrhoden 2017
## # ℹ 144 more rows
- Here’s some data about the number of deaths in each canton. https://opendata.swiss/de/dataset/todesfalle-nach-funf-jahres-altersgruppe-geschlecht-und-kanton-1969-2023 Can you download and clean this one?
download.file("https://dam-api.bfs.admin.ch/hub/api/dam/assets/32007751/master", "input_data/deaths.csv")
deaths <- read_csv("input_data/deaths.csv")
## Rows: 89100 Columns: 5
## ── Column specification ───────────────────────────────────────────────────────────────────────────────────────────────────────────────────────────────────────────────────────────────────────────────────────────────────
## Delimiter: ","
## chr (3): CANTON, AGE, SEX
## dbl (2): YEAR, OBS_VALUE
##
## ℹ Use `spec()` to retrieve the full column specification for this data.
## ℹ Specify the column types or set `show_col_types = FALSE` to quiet this message.
colnames(deaths) <- c("year", "canton", "age", "sex", "total_deaths")
deaths <- deaths |>
filter(age == "_T") |>
filter(canton != "CH") |>
filter(sex == "T") |>
select(-age, -sex)
deaths
## # A tibble: 1,430 × 3
## year canton total_deaths
## <dbl> <chr> <dbl>
## 1 1969 1 9846
## 2 1969 2 8836
## 3 1969 3 2405
## 4 1969 4 283
## 5 1969 5 830
## 6 1969 6 231
## 7 1969 7 201
## 8 1969 8 431
## 9 1969 9 526
## 10 1969 10 1728
## # ℹ 1,420 more rows
- Simplify the births data into just number of births by year. Then join this data with the deaths data. What was the total population change in each canton?
births <- births |>
group_by(year, canton_number, canton_name) |>
summarize(total_births = sum(total_born))
births
## # A tibble: 1,430 × 4
## # Groups: year, canton_number [1,430]
## year canton_number canton_name total_births
## <dbl> <chr> <chr> <dbl>
## 1 1969 1 Zürich 16638
## 2 1969 10 Freiburg 3187
## 3 1969 11 Solothurn 3759
## 4 1969 12 Basel-Stadt 2833
## 5 1969 13 Basel-Landschaft 3958
## 6 1969 14 Schaffhausen 1170
## 7 1969 15 Appenzell Ausserrhoden 774
## 8 1969 16 Appenzell Innerrhoden 274
## 9 1969 17 St. Gallen 7229
## 10 1969 18 Graubünden 2819
## # ℹ 1,420 more rows
deaths <- deaths |>
rename(canton_number = canton)
birth_and_deaths <- births |>
left_join(deaths)
## Joining with `by = join_by(year, canton_number)`
## # A tibble: 1,430 × 5
## # Groups: year, canton_number [1,430]
## year canton_number canton_name total_births total_deaths
## <dbl> <chr> <chr> <dbl> <dbl>
## 1 1969 1 Zürich 16638 9846
## 2 1969 10 Freiburg 3187 1728
## 3 1969 11 Solothurn 3759 1907
## 4 1969 12 Basel-Stadt 2833 2398
## 5 1969 13 Basel-Landschaft 3958 1491
## 6 1969 14 Schaffhausen 1170 755
## 7 1969 15 Appenzell Ausserrhoden 774 656
## 8 1969 16 Appenzell Innerrhoden 274 192
## 9 1969 17 St. Gallen 7229 3726
## 10 1969 18 Graubünden 2819 1570
## # ℹ 1,420 more rows
birth_and_deaths <- birth_and_deaths |>
mutate(total_population_change = total_births - total_deaths)
birth_and_deaths
## # A tibble: 1,430 × 6
## # Groups: year, canton_number [1,430]
## year canton_number canton_name total_births total_deaths total_population_change
## <dbl> <chr> <chr> <dbl> <dbl> <dbl>
## 1 1969 1 Zürich 16638 9846 6792
## 2 1969 10 Freiburg 3187 1728 1459
## 3 1969 11 Solothurn 3759 1907 1852
## 4 1969 12 Basel-Stadt 2833 2398 435
## 5 1969 13 Basel-Landschaft 3958 1491 2467
## 6 1969 14 Schaffhausen 1170 755 415
## 7 1969 15 Appenzell Ausserrhoden 774 656 118
## 8 1969 16 Appenzell Innerrhoden 274 192 82
## 9 1969 17 St. Gallen 7229 3726 3503
## 10 1969 18 Graubünden 2819 1570 1249
## # ℹ 1,420 more rows
birth_and_deaths |>
filter(year == 2000) |>
filter(canton_name == "Zürich") |>
select(total_population_change)
## Adding missing grouping variables: `year`, `canton_number`
## # A tibble: 1 × 3
## # Groups: year, canton_number [1]
## year canton_number total_population_change
## <dbl> <chr> <dbl>
## 1 2000 1 2485
- Make a plot of the total births and deaths in Basel-Stadt between 1970 and 2000.
birth_and_deaths |>
filter(canton_name == "Basel-Stadt") |>
select(year, canton_name, total_births, total_deaths) |>
pivot_longer(cols = c(total_births, total_deaths), names_to = "event", values_to = "total") |>
ggplot(aes(x = year, y = total, color = event)) +
geom_line()
## Adding missing grouping variables: `canton_number`
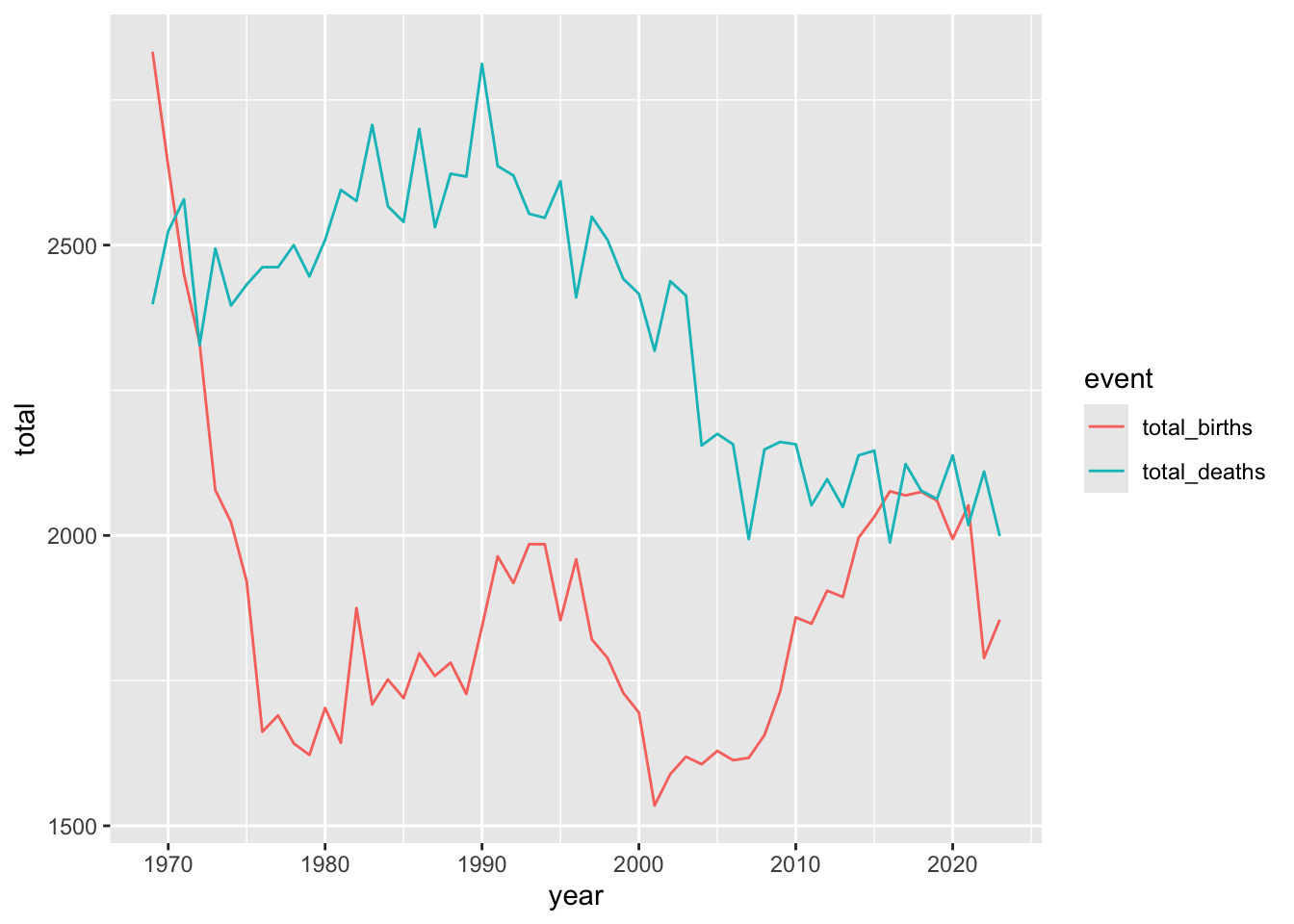