Chapter 14 Transformations of random variables
14.1 Introduction
In this Section we will consider transformations of random variables. Transformations are useful for:
- Simulating random variables.
For example, computers can generate pseudo random numbers which represent draws from distribution and transformations enable us to generate random samples from a wide range of more general (and exciting) probability distributions.
- Understanding functions of random variables.
Suppose that £ is invested in an account with continuously compounding interest rate . Then the amount £ in the account after years is
We will both univariate and bivariate transformations with the methodology for bivariate transformations extending to more general multivariate transformations.
14.2 Univariate case
Suppose that is a continuous random variable with p.d.f. . Let be a continuous function, then is a continuous random variable. Our aim is to find the p.d.f. of .
We present the distribution function method which has two steps:
- Compute the c.d.f. of , that is
- Derive the p.d.f. of , , using the fact that
Square of a Standard Normal
Let . Find the p.d.f. of .
Now , so
Thus , otherwise known as a Chi-squared distribution with degree of freedom. :::
14.3 Bivariate case
Suppose that and are continuous random variables with joint p.d.f. given by . Let . We want to find the joint p.d.f. of and .
Transformation of random variables.
Let be some transformation of random variables. If is a one-to-one function and the Jacobian of is non-zero in whereif , and otherwise.
Transformation of uniforms.
Let , and suppose that and are independent. LetFind the joint p.d.f. of and .
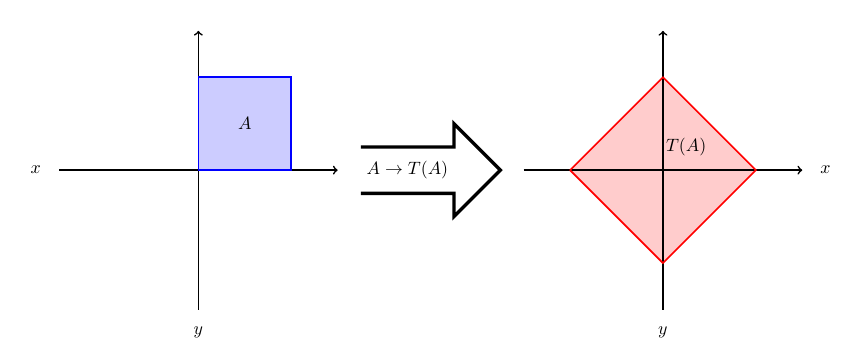
Figure 14.1: Transformation
Transformation of Exponentials.
Suppose that and are i.i.d. exponential random variables with parameter . Let and .
- Find the joint p.d.f. of and .
- Find the p.d.f. of .
Attempt Exercise 1: Transformation of Exponentials and then watch Video 22 for the solutions.
Video 22: Transformation of Exponentials
Alternatively the solutions are available:
Solution to Exercise 1
Remember from previous results that .
- Since and are i.i.d. exponential random variables with parameter , the joint p.d.f. of and is given by
Computing the Jacobian of , we get
Now,
Therefore, . Since and , Furthermore, since , then implies . Therefore,
Consequently, the joint p.d.f. of and , is given by
If either or , then .
- The p.d.f. of is the marginal p.d.f. of coming from the joint p.d.f . Therefore, for ,
So,
Figure 14.2: Plot of the p.d.f. of .
Note that one can extend the method of transformations to the case of random variables.
Student Exercise
Attempt the exercise below.
Question.
Let and be independent random variables, each having probability density function and let and .
- Find the joint probability density function of and .
- Hence derive the marginal probability density functions of and .
- Are and independent? Justify your answer.
Solution to Question.
- Let the transformation be defined by , where and . Then, and , so that
- The marginal pdf’s of and are respectively
- Clearly, does not hold for all , so and are not independent.