2 Key Statistical Concepts
2.1 Fundamental Concepts (1.2)
In introduction, we tried to convince ourselves that Statistics is indeed an exciting subject to study. Before we learn to perform statistical analysis, let’s define some fundamental Statistics terms.
Statistics: it is the art and science of collecting, analyzing, and presenting data. Statistics can be simply understood as the art and science of learning from data. Statistics provides us with tools that can help us make informed decisions. For instance, some schools have statistical models that tell them which students are at risk of dropping out, and what preventive actions are effective (See an example here). In manufacturing, Statistics is used for quality control. In Accounting, Statistics is used for frauds detection (Example: Benford’s law).
Data: simply put, data refers to any information collected for analysis.
Based on how frequent the data were collected, we can distinguish, among others:
Cross-sectional are data collected at a given point in time. Example: a survey administered at the beginning of a class.
Time series or longitudinal data data collected over several period. Example: data on stock prices, data on unemployment.
For your information, these days, there are other forms of data:
Text data: phrases, sentences used as data. Examples: State of the Union Speeches, product reviews.
Image data: Examples include pictures and videos.
Data are collected on subjects or things. Case, element, or observation are words often used to refer to the subject on which information have been collected. On non technical way, we often use the word row to refer to case, element, or observation.
Information are collected on specific aspects, or characteristics of the subject. A variable is a characteristic of the element. Examples: gender, age, weight, major, grade etc.
There are two main types of variables:
- Numerical variable (also known as Quantitative variable) refers to a characteristic that can be:
- counted (discrete variable). Example: number of siblings.
- Or measured (continuous variable). Example: height, weight, annual income.
Rule of thumb: a variable is a quantitative variable when calculating its average makes sense.
- Categorical variable (also known as qualitative variables) refers to a characteristic that describes something. Examples of categorical variables include Hair color, Gender, religious belief, belief in life after death (yes or no) etc. Categorical data can be ordinal. Example: the rating of a product.
Following is a summary flowchart of the different types of variables:
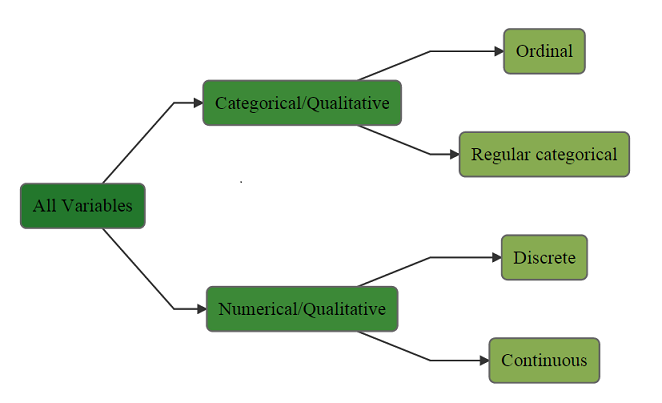
Flowchart of types of data
The distinction between categorical and quantitative variables is important because they are analyzed differently.
2.2 Data collection principles (1.3)
Depending on the research question we want to answer we will collect data on particular subjects.
- The Population is the set of all the subject of interest. Example: all the students of Econ-215, section 002 constitute a population of students taking econ-215, section 002.
Question 1. Assume a study is interested in understanding the study habits of the undergraduate students at UNL. What is the population of interest of this study?
Question 2. Assume a study is interested in understanding the academic performance of married students, in the US. What is the population of interest of this study?
Note: Each research question refers to a target population.
Often times, it is not practical to collect informations on all the subject in a population of interest; it may be too expensive, or unethical… Thus, we often rely on a sample from the population.
- A Sample refers to a subset of the population. Example: A set of 10, or 15 students constitutes a sample of econ-215, section 002 students.
Note: a valid sample must be representative of the population from which the sample was drawn. It is extremely difficult to get a representative sample of a population. We often rely on random sampling. Random sampling allows us to avoid systematic biases. The most basic random sample is called a simple random sample.
2.3 Three general steps of Statistics (1.4/1.5)
We can distinguish three general steps of Statistics:
Design: refers to planning how to obtain the data. For example, in a marketing survey, how do you select the people to survey so you will get data that provide good predictions about future sales? In social sciences, such as political science, sociology, and economics, we often rely on “observational data”, that is, data collected by monitoring what occurs. The researcher does not control anything; she just collect data on what is going on. On the contrary, sometimes, the researcher can conduct experiments. That is, the researcher can administer a treatment in some groups (randomly chosen), and administer nothing in other groups(also chosen randomly). Then collect data on what happen in both groups during and/or at the end of the experiment. The end goal is to compare the two groups to see if there are some differences. It is important to distinguish data obtained through experiments from observational data. Data obtained through a well design experiment is the gold standard.
Description (or descriptive Statistics): refers to exploring and summarizing patterns in the data.
Inference (or inference Statistics): refers to making decisions or predictions based on the data. Usually, the decision or prediction refers to a larger group of people, not merely those in the study. In other words, we infer about the general population from the observed data (or sample data).