7.2 Answers
- Short answers:
- Since x is defined over a continuous region, X is a continuous rv.
- The cdf is found: FY(y)=∫x−∞λexp(−λt)dt=|−exp(−λt)|x0=−exp(−λx)+1 since λ>0. That is, the cdf is FY(y)=1−exp(−λx) for x>0, and FY(y)=0 for x≤0. See Fig. 7.1.
- There are two ways: ∫∞3exp(−x)dx (using the pdf), or 1−FY(3) using the cdf. Either way, Pr.
- By definition, \displaystyle E[X] = \int_0^\infty x \lambda \exp(-\lambda x)\, dx, which requires integration by parts;
then E[X] = 1/\lambda.
Also by definition, \displaystyle E[X^2] = \int_0^\infty x^2 \lambda \exp(-\lambda x)\, dx, which requires integration by parts twice; then E[X] = 2/\lambda^2. So \text{var}[X] = E[X^2] - E[X]^2 = 1/\lambda^2.
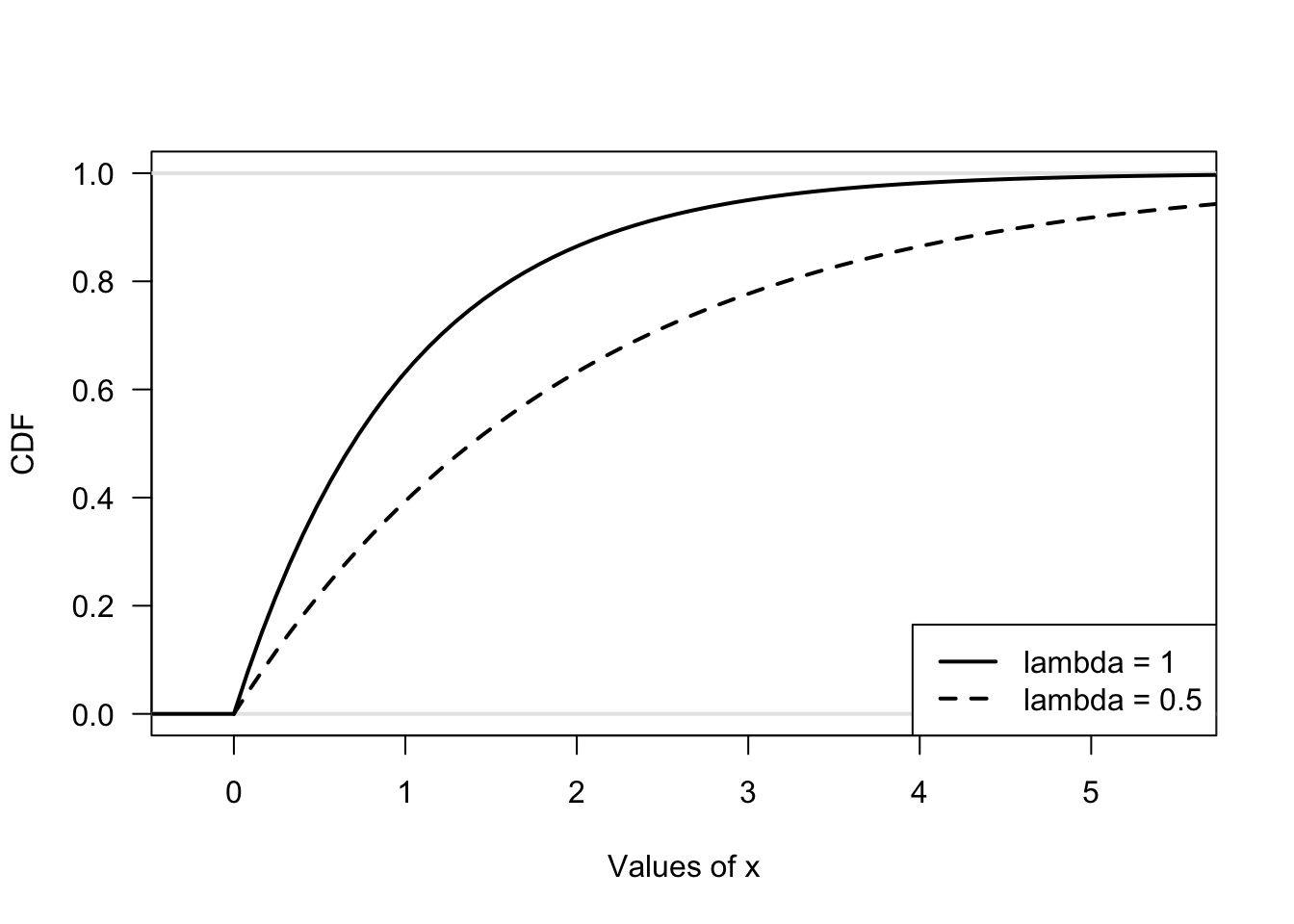
FIGURE 7.1: The CDF for Exercise 1
- Short answers:
- Since w is defined over a discrete region, W is a discrete rv.
- To be a pmf, all probabilities must be non-negative (that is, f_W(w) \ge 0 for all w, which is true), and
\sum_S f_W(w) = 1.
For the second condition, use a result from the sum of a geometric series, that \sum_0^\infty a r^k = a/(1 - r), where here a = 0.6 and r = 0.4. - The cdf is: F_W(w) = \left\{ \begin{array}{ll} 0 & \text{for $W < 1$}\\ 0.6 & \text{for $1 \le W < 2$} \\ 0.6 + (0.6 \times 0.4) & \text{for $2 \le W < 3$}\\ 0.6 + (0.6 \times 0.4) + (0.6 \times 0.4^2) & \text{for $3 \le W < 4$}\\ \end{array} \right. and a pattern can be seen to be developing. See Fig. 7.2.
- Here \Pr(W > 2) = 1 - \Pr(W = 1) - Pr(W = 2), so \Pr(W > 2) = 1 - 0.6 - (0.6\times 0.4) = 0.16,
- You will need to use some results about sums of infinite series.
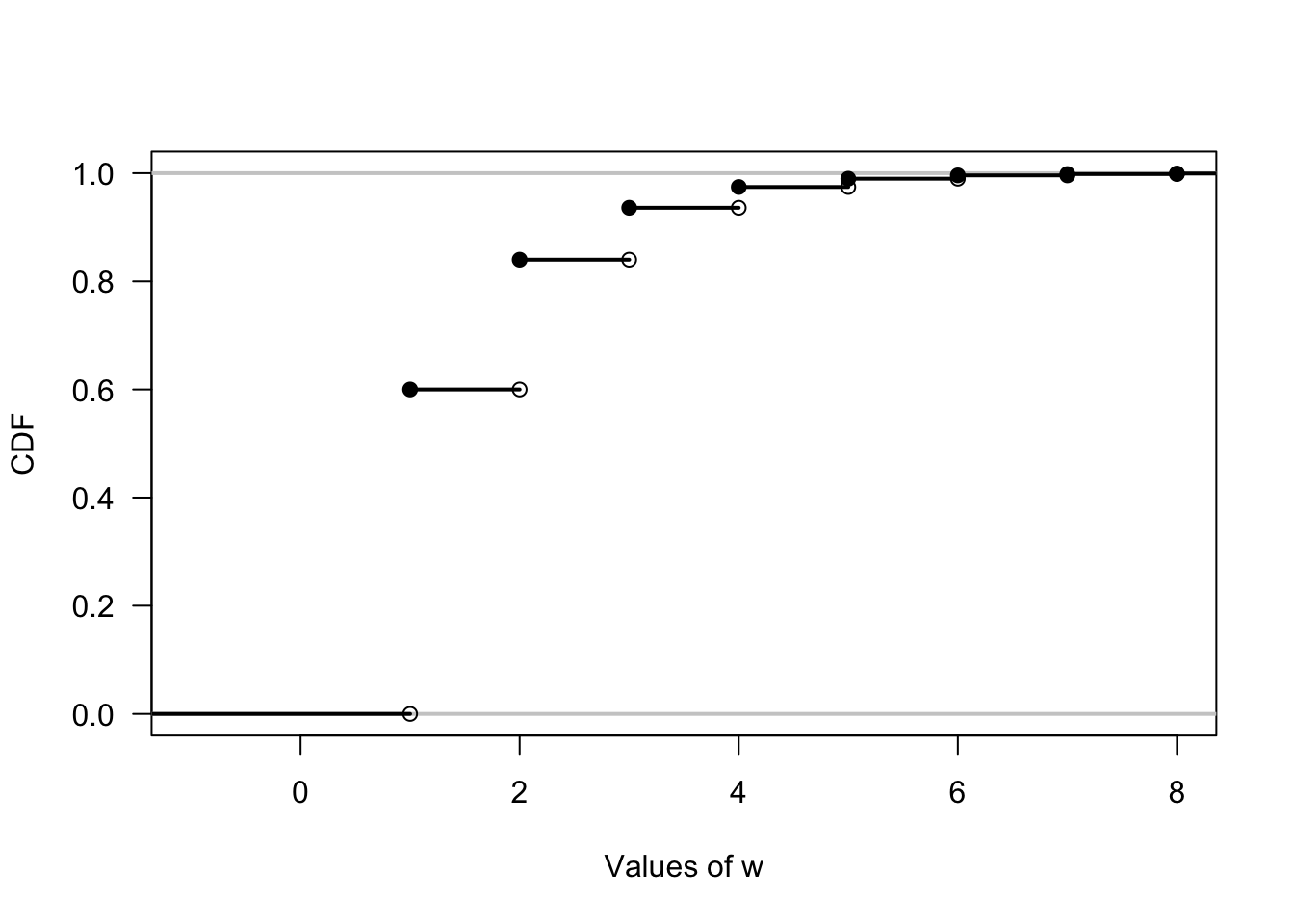
FIGURE 7.2: The CDF for Exercise 2
- Short solutions (and see Exercise 1):
- We need \int_W f_X(x)\, dx = 1, so \int_0^\infty k\exp(-x/4)\,dx = 1. Solving, k = 1/4.
- The cdf is F_X(x) = 1 - \exp(-x/4).
See Fig. 7.3. - \Pr<X \le 2) = F_X(2) = 1 - \exp(-2/4) \approx 0.393.
- \Pr(2 < X < 4) = F_X(4) - F(X(2) \approx 0.239.
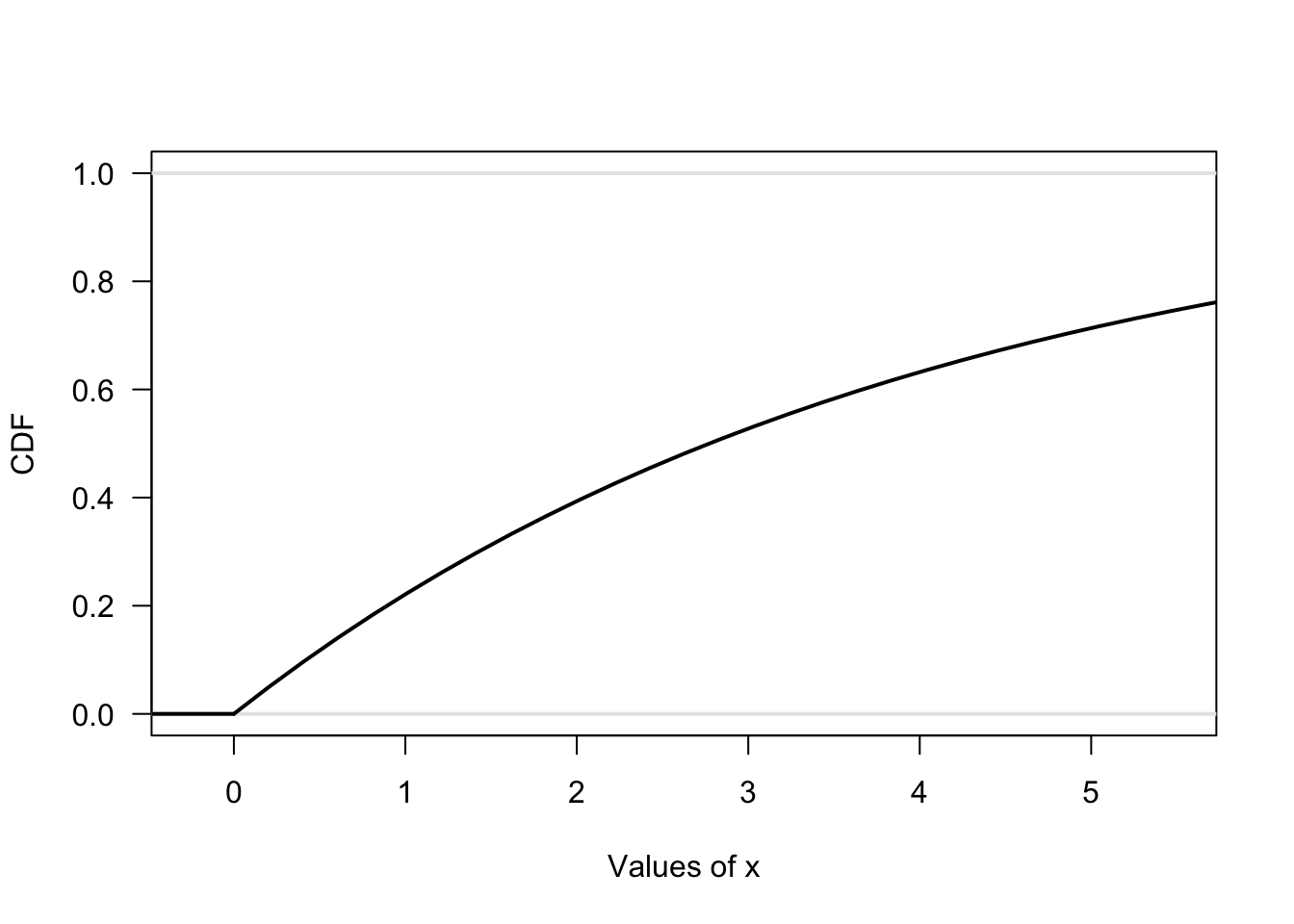
FIGURE 7.3: The CDF for Exercise 1
First we need to find the value of k, by solving 1 = k \int_0^2 \!\!\! \int_0^1 3x^2 + xy\, dx\, dy, showing that k = 1/3.
Then the marginal distribution for x is: f_X(x) = \frac{1}{3}\int_0^2 3x^2 + xy\, dy = 2x^2 + \frac{2x}{3} for 0 < x < 1.
Also, the marginal distribution for y is: f_Y(y) = \frac{1}{3}\int_0^1 3x^2 + xy\, dx = \frac{y + 2}{6} for 0 < y < 2.This question has an irregular sample space, so care is needed!
- See Fig. 7.4.
- We need to solve 1 = k \int_{z=0}^{z=1}\!\!\int_{y=0}^{y = z} y + z\, dy\, dz, when we find that k = 2.
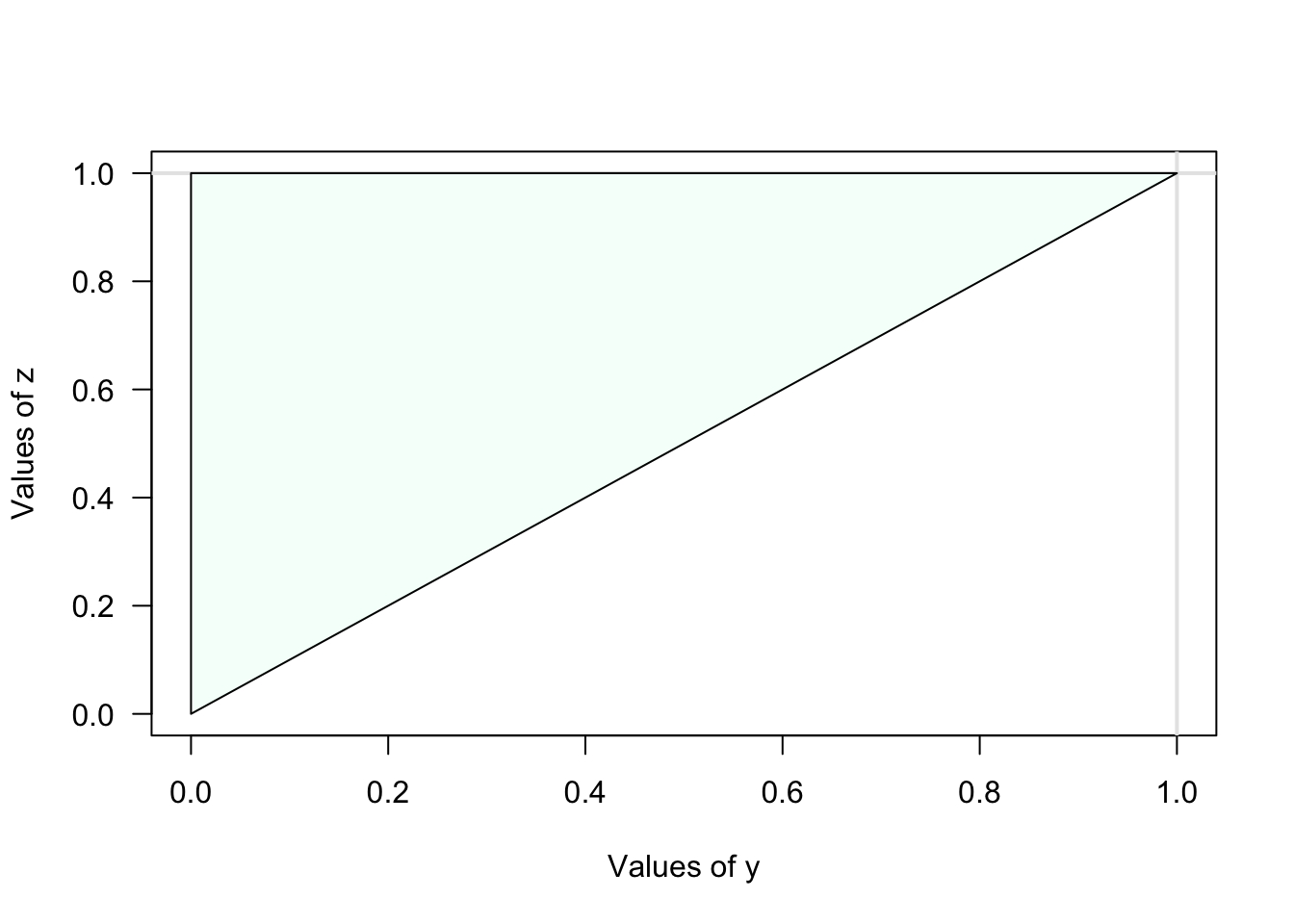
FIGURE 7.4: The sample space for Exercise 5
- Proceed: \begin{align*} \text{Var}[ \bar{X} ] &= \text{Var}[ (X_1 + X_2 + \cdots + X_n)/n]\\ &= \frac{1}{n^2} \text{Var}[ X_1 + X_2 + \cdots + X_n ]\qquad \text{(since $\text{Var}[cX] = c^2\text{Var}[X]$))}\\ &= \frac{1}{n^2} \left( \text{Var}[ X_1] + \text{Var}[X_2] + \cdots + \text{Var}[X_n] \right)\\ &= \frac{1}{n^2} (n \times \sigma^2)\qquad \text{(since $\text{Var}(X) = \sigma^2$ for all $X$)}\\ &= \frac{\sigma^2}{n}. \end{align*}