7 Lecture 5 - 22/12/2020
In this lecture we will learn how to use the ggplot2
library to produce very nice plots.
7.1 The ggplot2
library
The ggplot2
is part of the tidyverse
collection of packages. The grammar of graphics plot (ggplot) is an alternative to standard R functions for plotting; see here for the ggplot2 website. In Figure ?? we have some examples of plot (simple scatterplot, scatterplot with legend and boxplots) produced using standard R code and the ggplot2
library.
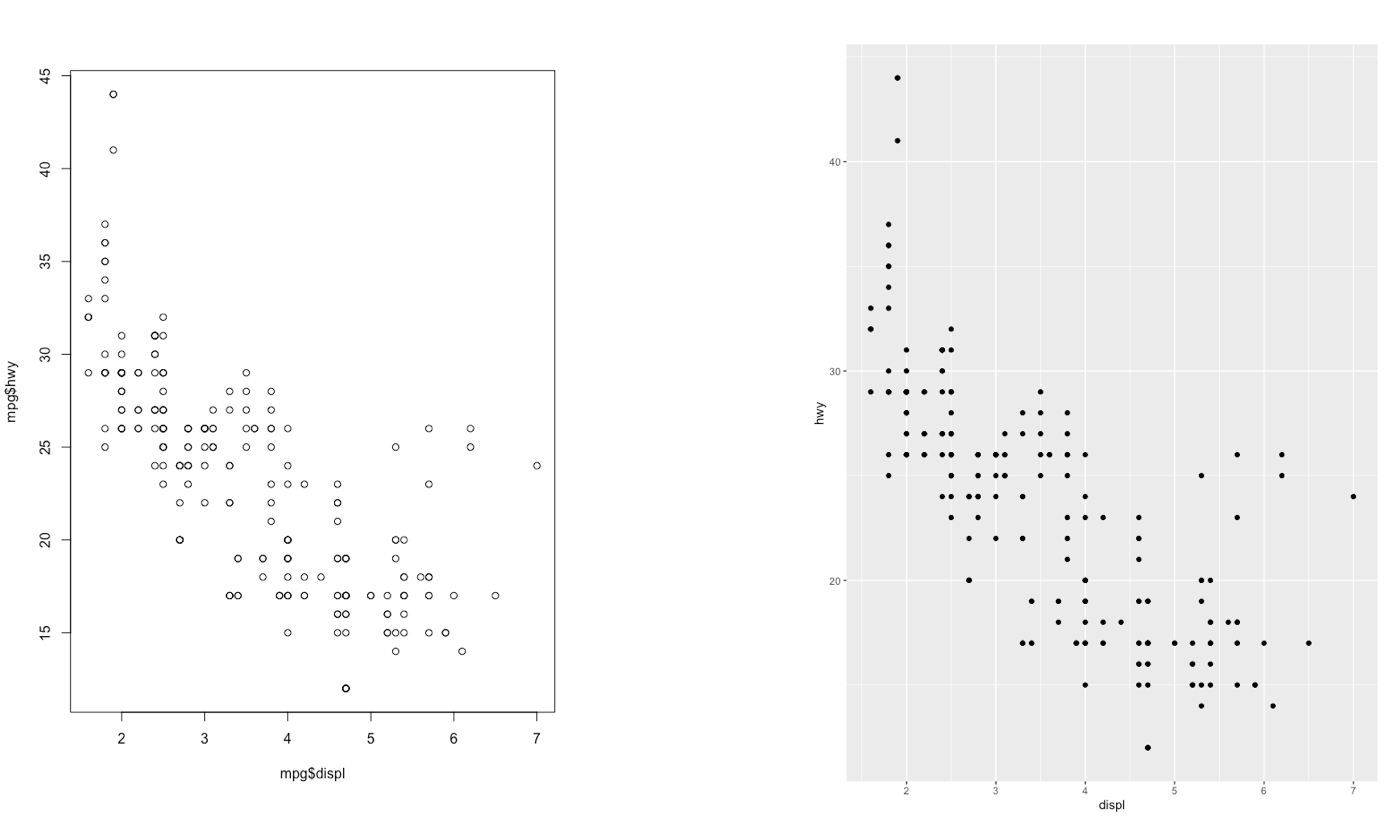
Figure 7.1: Comparison between standard and ggplot2 plots
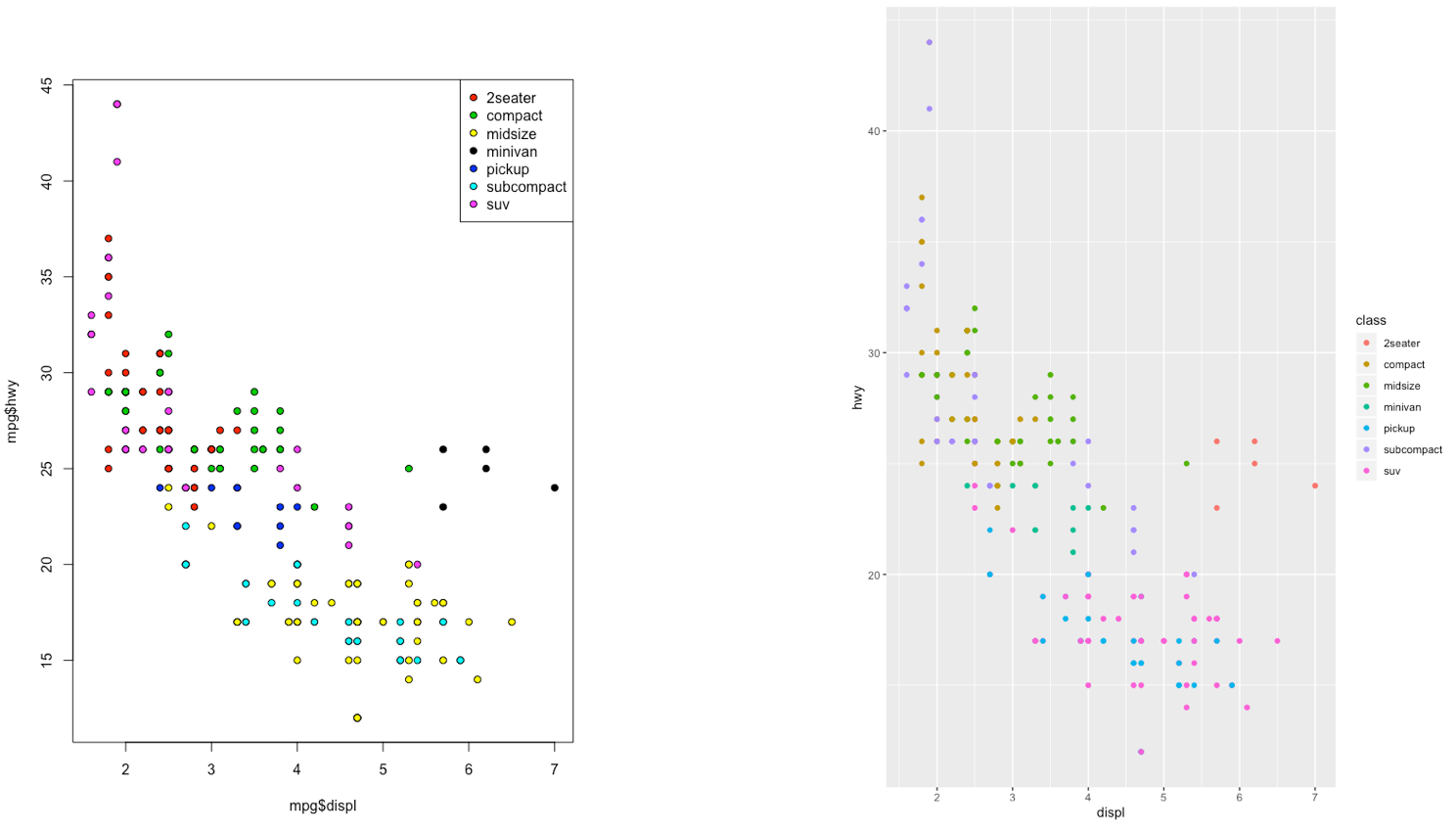
Figure 7.2: Comparison between standard and ggplot2 plots
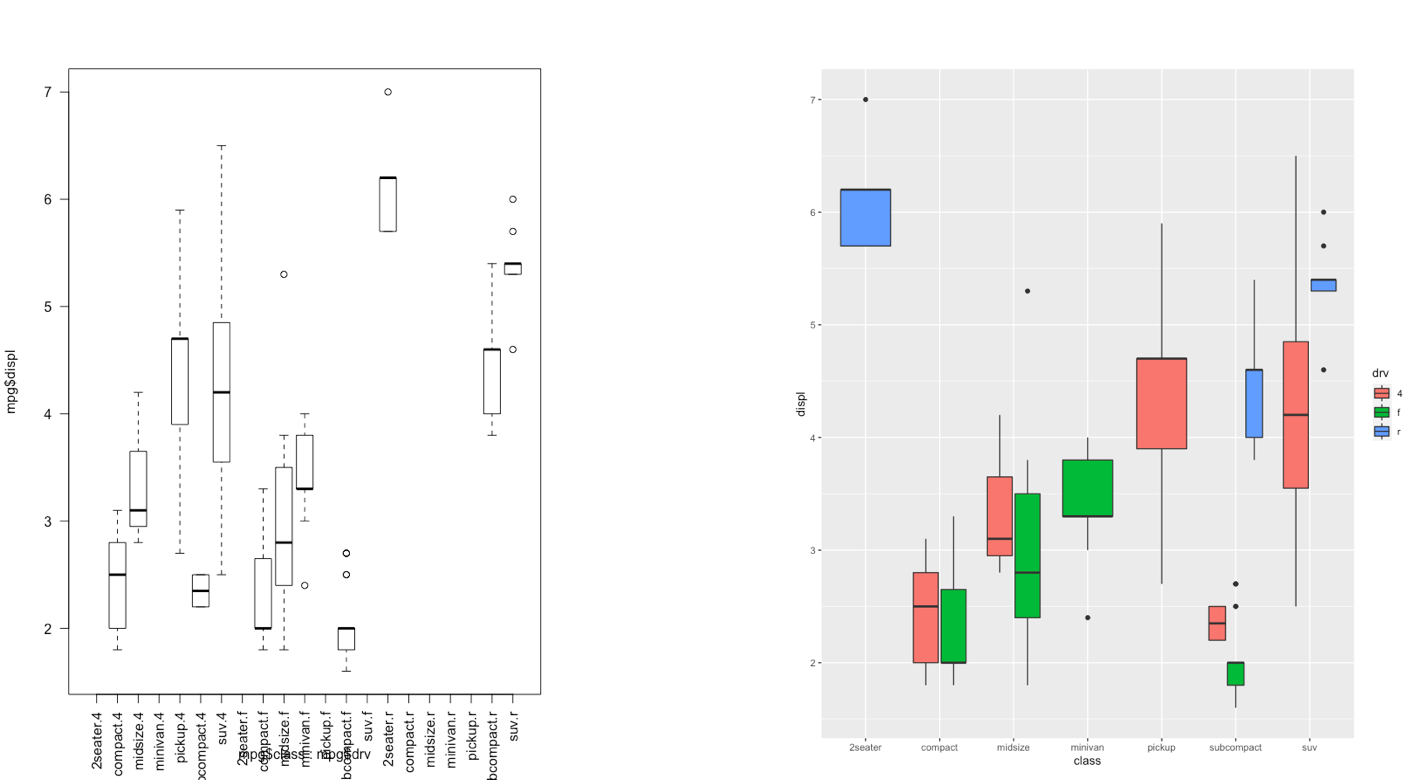
Figure 7.3: Comparison between standard and ggplot2 plots
With ggplot2
a plot is defined by several layers, as shown in Figure 7.4. The first layer specifies the coordinate system, then we can have several geometries each with aesthetics specification.
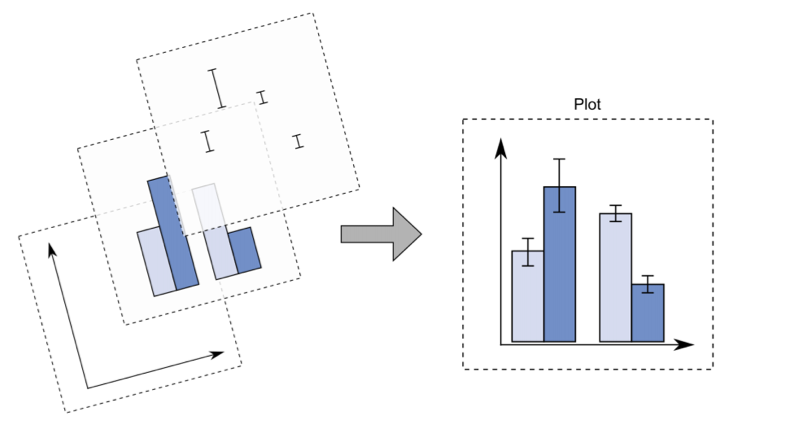
Figure 7.4: The layer structure of the ggplot2
plot
7.2 Data subsetting and the ggplot
function
Instead of working with the entire diamonds
data set, as done in Lecture 4, we will create a smaller data set by sampling randomly 1% of the diamonds by means of the function sample_frac
. As this is a random procedure we set, as usual, the seed in order to have a reproducible outcome. The new (smaller) data set will be called mydiamonds
:
## Rows: 539
## Columns: 10
## $ carat <dbl> 0.72, 1.52, 0.32, 1.10, 1.34, 0.31, 0.26, 0.70, 0.36, 0.37, 1.…
## $ cut <ord> Ideal, Good, Premium, Premium, Ideal, Very Good, Ideal, Premiu…
## $ color <ord> E, J, G, J, H, G, I, G, E, D, I, H, E, D, G, I, G, G, E, H, D,…
## $ clarity <ord> VS2, VS2, SI1, VS2, SI1, VVS1, VS2, SI1, VS1, SI1, VS1, VS2, V…
## $ depth <dbl> 62.8, 63.3, 61.0, 61.2, 62.4, 63.0, 62.0, 61.8, 60.9, 62.7, 60…
## $ table <dbl> 57, 56, 61, 57, 54, 56, 56, 59, 57, 58, 60, 61, 59, 56, 54, 58…
## $ price <int> 2835, 7370, 612, 3696, 7659, 710, 385, 2184, 782, 874, 6279, 1…
## $ x <dbl> 5.71, 7.27, 4.41, 6.66, 7.05, 4.26, 4.13, 5.68, 4.60, 4.58, 6.…
## $ y <dbl> 5.73, 7.33, 4.38, 6.61, 7.08, 4.28, 4.09, 5.59, 4.63, 4.55, 6.…
## $ z <dbl> 3.59, 4.62, 2.68, 4.06, 4.41, 2.69, 2.55, 3.48, 2.81, 2.86, 4.…
The most important function of the ggplot2
library is the ggplot
function.
All ggplot
plots begin with a call to ggplot supplying the data:
where geom_function
is a generic function for a geometry layer; see here for the list of all the available geometries.
For starting a new empty plot we can proceed by using one of the following codes:
To add components and layers to the empty plot we will use the +
symbol.
7.3 Scatterplot
We begin with a scatterplot displaying price
on the y-axis and carat
on the x-axis; the necessary geometry is implemented with geom_point
:
The argument
mapping
specifies the set of aesthetic mappings, created by aes
, which describe the visual characteristics that represent the data, e.g. position, size, color, shape, transparency, fill, etc. From the scatterplot we observe that a positive non linear relationship exists between carat
and price
.
It is also possible to specify a different color for each point according to the corresponding category of cut
:
Note that automatically a legend is added that explains which level corresponds to each color. From the plot we do not observe a clear clustering of the diamonds according to their quality.
If instead we are interested in changing the point color making all the points blue we have to include the color
option outside the aes
specification:
Thus, if an aesthetic like the color is linked to data (e.g.
cut
) it is put into aes()
, otherwise it is put outside.
There is also the possibility to set the color according to a condition, e.g. cut == "Premium"
:
In this case the red color is used when the condition is false, and the green color when it is true.
It is also possible to set a different shape - instead of points - according to the categories of cut
:
## Warning: Using shapes for an ordinal variable is not advised
Finally, an alternative for considering the distribution of carat
and price
conditionally on cut
is to produce 5 separate scatterplots according to the 5 categories of cut
. In this case we use the facet which defines how data are split among panels. The default facet puts all the data in a single panel, while facet_wrap
and
facet_grid()
allow you to specify different types of small multiple plot.
mydiamonds %>%
ggplot() +
geom_point(mapping = aes(x=carat,y=price), color="blue") +
geom_smooth(aes(x=carat,y=price)) +
facet_wrap(~cut)
## `geom_smooth()` using method = 'loess' and formula 'y ~ x'
Note that in all the facets reported in the previous plot a new geometry has been included by means of geom_smooth
, which adds in the plot a smooth line that can be ease the interpretation of the pattern in the plots.
7.4 Boxplot
The boxplot can be used to study the distribution of a quantitative variable (e.g. price
) conditioning on the categories of a factor (e.g. cut
). It can be obtained by using the geom_boxplot
geometry, where x
is given by the qualitative variable:
The quality category with highest (lowest) median price is Premium (Ideal). Fair quality diamonds are characterized by less variability in terms of price and are not characterized by extreme price values as happened for the other categories.
It is also possible to choose a different fill color and contour color for all the boxes by using fill
and color
:
In the previous plot all the boxes are characterized by the same fill and contour color. If we instead interested in using different fill colors according to cut
we have to specify the aesthetics with aes
:
7.5 Histogram and density plot
When the aim is the analysis of the distribution of a continuos variable like price
an histogram can be used. This is implemented by using the geom_histogram
geometry:
## `stat_bin()` using `bins = 30`. Pick better value with `binwidth`.
Note that in this case we need to specify only the
x
variable, while the y
is computed automatically by ggplot and corresponds to the count
variable (i.e. how many observations for each class of price values). This is given by the fact that every geometry has a default stat
specification. For the histogram the default computation is stat_bin
which uses 30 bins and computes the following variables:
- count
, the number of observations in each bin;
- density
, the density of observations in each bin (percentage of total / bar width);
- x
, the centre of the bin.
The histogram is a fairly crude estimator of the variable distribution. As an alternative it is possible to use the (non parametric) Kernel Density Estimation (see here) implemented in ggplot by geom_density
(only x
has to be specified):
Note that the y-axis range is completely different with respect to the one of the histogram.
To combine together in a single plot the histogram and the density function, it is first of all necessary to produce an histogram which uses on the y-axis density instead of counts. The old approach to obtain density also in the y-axis of the histogram uses y=..density..
. A more modern approach adopts the function after_stat
which refers to the generated variable density
:
mydiamonds %>%
ggplot() +
geom_histogram(aes(x=price,y=after_stat(density)),
fill="lightblue",color="black") +
geom_density(aes(x=price))
## `stat_bin()` using `bins = 30`. Pick better value with `binwidth`.
Note that the aes
settings can be specified separately for each layer or globally in the ggplot
function. See here below for a different specification (global now) of the previous plot:
mydiamonds %>%
ggplot(aes(x=price)) + # your global specifications
geom_histogram(aes(y=after_stat(density)),
fill="lightblue",color="black") +
geom_density()
## `stat_bin()` using `bins = 30`. Pick better value with `binwidth`.
7.6 Barplot
The barplot can be used to represent the distribution of a categorical variable, such as for example cut
. It can be obtained by using the geom_bar
geometry:
Similarly to the histogram, the y-axis is computed automatically and is given by counts (for
geom_bar
we have that stat="count"
). If we are interested in percentages instead of absolute counts we can use the after_stat
function and compute a transformation of count
:
mydiamonds %>%
ggplot() +
geom_bar(aes(x=cut,
y=after_stat(count/nrow(mydiamonds)*100)))+
ylab("my y-axis label") +
xlab("my x-axis label")
The functions
xlab
and ylab
can be used to specify a different label for the two axes.
It is also possible to take into account in the barplot another qualitative variable such as for example clarity
that will be used to set the bar fill aesthetic:
Note that the bars are automatically stacked and each colored rectangle represents a combination of
cut
and clarity
. The stacking is performed automatically by the position adjustment given by the position
argument (by default it is set to position = "stack"
). Other possibilities are "dodge"
and "fill"
:
The option
position = "fill"
is similar to stacking but each set of stacked bars has the same height (corresponding to 100%); this makes the comparison between groups easier. The option position = "dodge"
places the rectangles side by side.
An alternative consists in the use of facet_wrap
that will create 8 separate plots (as the number of categories of clarity
) each representing the conditional cut
distribution:
7.7 Exercises Lecture 4 and 5
7.7.1 Exercise 1
Write the code for the following operations:
- Simulate a vector of 15 values from the continuous Uniform distribution defined between 0 and 10 (see
?runif
). Set the seed equal to 99. - Round the numbers in the vector to two digits.
- Sort the values in the vector in descending order (see
?sort
) - Display a few of the largest values with
head()
.
Use both the standard R
programming approach and the more modern approach based on the use of the pipe %>%
(remember to load the tidyverse
library).
7.7.2 Exercise 2
Consider the mtcars
data set which is avalaible in R
. Type the following code to explore the variables included in the data set (for an explanation of the variables see ?mtcars
:
## Rows: 32
## Columns: 11
## $ mpg <dbl> 21.0, 21.0, 22.8, 21.4, 18.7, 18.1, 14.3, 24.4, 22.8, 19.2, 17.8,…
## $ cyl <dbl> 6, 6, 4, 6, 8, 6, 8, 4, 4, 6, 6, 8, 8, 8, 8, 8, 8, 4, 4, 4, 4, 8,…
## $ disp <dbl> 160.0, 160.0, 108.0, 258.0, 360.0, 225.0, 360.0, 146.7, 140.8, 16…
## $ hp <dbl> 110, 110, 93, 110, 175, 105, 245, 62, 95, 123, 123, 180, 180, 180…
## $ drat <dbl> 3.90, 3.90, 3.85, 3.08, 3.15, 2.76, 3.21, 3.69, 3.92, 3.92, 3.92,…
## $ wt <dbl> 2.620, 2.875, 2.320, 3.215, 3.440, 3.460, 3.570, 3.190, 3.150, 3.…
## $ qsec <dbl> 16.46, 17.02, 18.61, 19.44, 17.02, 20.22, 15.84, 20.00, 22.90, 18…
## $ vs <dbl> 0, 0, 1, 1, 0, 1, 0, 1, 1, 1, 1, 0, 0, 0, 0, 0, 0, 1, 1, 1, 1, 0,…
## $ am <dbl> 1, 1, 1, 0, 0, 0, 0, 0, 0, 0, 0, 0, 0, 0, 0, 0, 0, 1, 1, 1, 0, 0,…
## $ gear <dbl> 4, 4, 4, 3, 3, 3, 3, 4, 4, 4, 4, 3, 3, 3, 3, 3, 3, 4, 4, 4, 3, 3,…
## $ carb <dbl> 4, 4, 1, 1, 2, 1, 4, 2, 2, 4, 4, 3, 3, 3, 4, 4, 4, 1, 2, 1, 1, 2,…
Use the following code to create a new variable named car_model
that contains the names of the cars, now available as row names.
## Rows: 32
## Columns: 12
## $ car_model <chr> "Mazda RX4", "Mazda RX4 Wag", "Datsun 710", "Hornet 4 Drive"…
## $ mpg <dbl> 21.0, 21.0, 22.8, 21.4, 18.7, 18.1, 14.3, 24.4, 22.8, 19.2, …
## $ cyl <dbl> 6, 6, 4, 6, 8, 6, 8, 4, 4, 6, 6, 8, 8, 8, 8, 8, 8, 4, 4, 4, …
## $ disp <dbl> 160.0, 160.0, 108.0, 258.0, 360.0, 225.0, 360.0, 146.7, 140.…
## $ hp <dbl> 110, 110, 93, 110, 175, 105, 245, 62, 95, 123, 123, 180, 180…
## $ drat <dbl> 3.90, 3.90, 3.85, 3.08, 3.15, 2.76, 3.21, 3.69, 3.92, 3.92, …
## $ wt <dbl> 2.620, 2.875, 2.320, 3.215, 3.440, 3.460, 3.570, 3.190, 3.15…
## $ qsec <dbl> 16.46, 17.02, 18.61, 19.44, 17.02, 20.22, 15.84, 20.00, 22.9…
## $ vs <dbl> 0, 0, 1, 1, 0, 1, 0, 1, 1, 1, 1, 0, 0, 0, 0, 0, 0, 1, 1, 1, …
## $ am <dbl> 1, 1, 1, 0, 0, 0, 0, 0, 0, 0, 0, 0, 0, 0, 0, 0, 0, 1, 1, 1, …
## $ gear <dbl> 4, 4, 4, 3, 3, 3, 3, 4, 4, 4, 4, 3, 3, 3, 3, 3, 3, 4, 4, 4, …
## $ carb <dbl> 4, 4, 1, 1, 2, 1, 4, 2, 2, 4, 4, 3, 3, 3, 4, 4, 4, 1, 2, 1, …
Use the following code to define two factors for cyl
and am
which are now considered as dbl
variables:
- How many observations and variables are available?
- Print (on the screen) the
hp
variable using theselect()
function. Try also to use thepull()
function. Which is the difference? - Print out all but the
hp
column using theselect()
function. - Print out the following variables:
mpg
,hp
,vs
,am
,gear
. Suggestion: use:
if necessary. - Provide the frequency distribution (absolute, relative and percentage) for the
am
variable (transmission). - Consider the variables
wt
(weight) andmpg
(miles/gallon). By usingplot()
and thenggplot()
produce the scatterplot which displays consumption as a function of weight. Which kind of relationship does exist between the two variables? - Consider the
ggplot
code used for the previous sub-exercise. Plot the same variables but use the variableam
(transmission) to color the points. Comment the plot. - Create a new variable
km_per_litre
using themutate()
function. Suggestion: 1 mpg is 0.425 km/l. Save the variable in the dataframe. Provide the average value ofkm_per_litre
conditioning onam
(transmission). For which kind of transmission is the average fuel consumption higher? - Reorder the observations so that you can easily find the car with the lowest consumption.
- Select all the observations which have
mpg>20
andhp>100
and compute the average for thekm_per_litre
variable. - Compute the distribution (absolute frequencies) of the car by
gear
. Moreover, compute the mean consumption (mpg
) conditionally on thegear
. - Create a new variable named
performance
given byhp/mpg
. Filter the first 10 best cars in terms of performance (suggestion: use the functiontop_n
). - Display
hp
(gross horsepower) as a function ofcyl
by usingggplot()
. Usefacet_wrap
to produce different plots according toam
(transmisison). Which kind of relationship does exist between the two variables?
7.7.3 Exercise 3
Consider the iris
dataset available in R. It contains the measurements in centimeters of the variables sepal length and width and petal length and width, respectively, for some flowers from each of 3 species of iris. See ?iris
.
## Rows: 150
## Columns: 5
## $ Sepal.Length <dbl> 5.1, 4.9, 4.7, 4.6, 5.0, 5.4, 4.6, 5.0, 4.4, 4.9, 5.4, 4.…
## $ Sepal.Width <dbl> 3.5, 3.0, 3.2, 3.1, 3.6, 3.9, 3.4, 3.4, 2.9, 3.1, 3.7, 3.…
## $ Petal.Length <dbl> 1.4, 1.4, 1.3, 1.5, 1.4, 1.7, 1.4, 1.5, 1.4, 1.5, 1.5, 1.…
## $ Petal.Width <dbl> 0.2, 0.2, 0.2, 0.2, 0.2, 0.4, 0.3, 0.2, 0.2, 0.1, 0.2, 0.…
## $ Species <fct> setosa, setosa, setosa, setosa, setosa, setosa, setosa, s…
- Compute the frequency distribution (absolute, relative and percentage frequencies) of
Species
. Which are the available species? - Compute the average and the standard deviation for all the numeric column of
iris
. Then compute the same statistics by conditioning on species. Suggestion: use the functionsummarise_if
(see?summarise_if
, in this case test if the columnis.numeric
): - Compute the average of
Sepal.Length
andPetal.Length
bySpecies
. Which is the species with the highest average sepal/petal length? - Plot
Petal.Width
as a function ofPetal.Length
with a scatterplot. Use the species for the color and shape of the points. Which kind of relationship does it exist? - Add to the data frame a new variable called
Ratio.Sepal
which is given bySepal.Length/Petal.Width
. Compute the mode of this variable (by computing the frequency distribution and then reordering by the frequencies).
7.7.4 Exercise 4
Consider the data available in the fev.csv file (use fevdata
as the name of the dataframe). The dataset examines if respiratory function in children was influenced by exposure to smoking at home. The included variables are:
Age
FEV
: forced expiratory volume in liters (lung capacity)Ht
: height measured in inchesGender
: 0=female, 1=maleSmoke
: exposure to smoking (0=no, 1=yes)
- Import the data in
R
and check the type of variables contained in the dataframe. - Trasform
Gender
andSmoke
into factors. - Compute the univariate and bivariate contingency table (with percentage frequencies) for the new factors
Gender
andSmoke
. - Represent graphically height, age and FEV by histogram or barplot. Avoid using count on the y-axis. Comment the plots.
- Using boxplots study the distribution of
Age
and thenHt
conditioned onGender
. Comment the plots. - Using a scatterplot represent
FEV
as a function ofHt
. Use different colors according to the smoke information. Comment. - Produce different scatterplots representing
FEV
as a function ofHt
according to the gender categories. Include also in the plots a smooth line. Comment the plots.
7.7.5 Exercise 5
Consider the Titanic data contained in the file titanic_tr.csv. This is a subset (with 891 observations and 11 variables) of the original dataset. The included variables are the following:
pclass
: passenger class (first, second or third)survived
: survived (1) or died (0)name
: passenger namesex
: passenger sexage
: passenger agesibSp
: number of siblings/spouses aboardparch
: number of parents/children aboardticket
: ticket numberfare
: fare (cost of the ticket)cabin
: cabin idembarked
: port of embarkation (S = Southampton, C = Cherbourg, Q = Queenstown)
- Import the data and explore them. Transform the variable
survived
,pclass
andsex
into factors. - Represent graphically the distribution of the variable
fare
. Moreover, compute the average ticket price paid by passengers. Finally, compute the percentage of tickets paid more than 100$. - Represent graphically the distribution of the variable
age
. Compute the average age. Pay attention to missing values. Consider the possibility of using thena.rm
option of the functionmean
(see?mean
). - Study the distribution of
sex
by using a barplot. Derive also the corresponding table frequency distribution. - By using a graphical representation study the distribution of age conditionally on gender. Moreover, compute the mean age by gender.
- Compute the (absolute and percentage) bivariate distribution of
sex
andsurvived
(percentages should be computed with respect to the total sample size). Moreover, produce the corresponding plot which represents the two factors. - Derive the percentage distribution of
survived
conditioned onsex
. Produce also the corresponding plot. - Filter by sex and consider only males and compute the frequency distribution of the variable
embarked
. Produce the corresponding plot. - Create a new variable called
agecat
with two categories (minor
if age < 18,major
otherwise). Then derive the frequency distribution ofagecat
. - Produce a scatterplot with
age
on the x-axis andfare
on the y-axis. Use a different point color according to gender. - Study the relationship between
age
andfare
, as you did in the previous sub-exercise, producing sub-plots according toembarked
.