4 Results
4.1 Coverage
4.1.1 Geographical coverage
Should probably include a map here of the countries that we have included. Here is a list instead to start with
A complete set of trait and Aquamap data (allowing the calculation of climate hazard) was gathered for 147 species caught in European waters, representing 90.3% of the total value of fish and shellfish landings in Europe. Catch data was available covering 26 countries in Europe: country-wise coverage was at least 78% by value and typically above 90%.
When combined with catch data covering 23 FAO subareas, this resulted in a total of 1300 potential “stocks”. However, many of these stocks made relatively minor contributions to the total catch of the species: stocks comprising less than 5% of the total catch of that species were therefore filtered out, leaving 523 stocks in the analysis.
4.1.2 Fleet Coverage
We should also check the coverage by fleet segment. We are able to do the full analysis for 404 fleet segments, although the coverage of some of these units can be pretty poor.
Nevertheless, we still cover 75% or more of the landings value for more than 70% of fleets. We should probably filter this at some point to focus on the most important and/or best covered fleets.
Number of fleet segements: 358
4.2 Biological metrics
4.2.1 Species-specific metrics
As a cross check that we have implemented the coversion from these metrics to a hazard, we plot one against the other, to ensure that the trend is as intended
So, longer-lived species have a higher hazard score. Tick.
So species with a higher habitat-specificity have a higher hazard. Tick.
4.2.2 Stock-specific metrics
Small stocks are a potential problem in this analysis. We first check the distribution of stock sizes (expressed as value of landings) relative to the total size (value) of the species.
We can therefore see that there are an awful lot of stocks that don’t contribute much landigns value - is is a natural consequence of the way that we have defined a stock in this case. We choose to filter out all stocks that have a landings value less than 5% of the species total.
This leaves us with 523 stocks. Looking at the distribution of TSM’s of these remaining stocks.
And cross checking the hazard implementation.
Low TSMs => higher hazards. Tick. The stripping rises due to the categorical natural of the other metrics.
4.2.3 Species-level hazard
We can look at our biological analysis by integrating the hazard back up to the species level by weighting by the value of landings of each stock.
Looking at the top and bottom ten species
FAO_3A_code | FAO_EN | hazard | rank |
---|---|---|---|
WHE | Whelk | 0.8828335 | 1 |
DEC | Common dentex | 0.8790946 | 2 |
KLK | Smooth callista | 0.8434970 | 3 |
FOR | Forkbeard | 0.7948277 | 4 |
OYF | European flat oyster | 0.7771141 | 5 |
SCE | Great Atlantic scallop | 0.7704106 | 6 |
HKX | Hakes nei | 0.7291516 | 7 |
PRR | Parrotfish | 0.7263368 | 8 |
SVE | Striped venus | 0.7242199 | 9 |
MEG | Megrim | 0.7199062 | 10 |
OCC | Common octopus | 0.2299293 | 139 |
HER | Atlantic herring | 0.2271872 | 140 |
SQI | Northern shortfin squid | 0.2237962 | 141 |
SPR | European sprat | 0.2159665 | 142 |
SQC | Common squids nei | 0.2020260 | 143 |
SQM | Broadtail shortfin squid | 0.1988565 | 144 |
OCZ | Octopuses nei | 0.1905851 | 145 |
BLT | Bullet tuna | 0.1679663 | 146 |
SSH | Scarlet shrimp | 0.1310705 | 147 |
BON | Atlantic bonito | 0.1093046 | 148 |
4.3 Fleet metrics
4.3.1 Fleet hazard metrics
Fleets with the top and bottom 10 hazard scores
fs_name | value.covered | total.val.landings | prop.missing | hazard |
---|---|---|---|---|
EST A27 TM1218 | 860437.0 | 860437.0 | 0.0000000 | 0.0550035 |
LTU A27 TM2440 ° | 13475876.0 | 13475876.0 | 0.0000000 | 0.0588392 |
EST A27 TM2440 ° | 48072014.0 | 48072264.0 | 0.0000052 | 0.0627536 |
POL A27 TM2440 ° | 73972680.3 | 75163367.3 | 0.0158413 | 0.0677118 |
FIN A27 TM2440 ° | 107055789.0 | 107065174.7 | 0.0000877 | 0.0704230 |
FIN A27 TM1824 | 30019850.0 | 30019850.0 | 0.0000000 | 0.0705229 |
FIN A27 TM1218 ° | 10807913.8 | 10983806.6 | 0.0160138 | 0.0742556 |
EST A27 PG1012 | 7613725.0 | 7630744.0 | 0.0022303 | 0.0761999 |
PRT A27 FPO0010 | 1681210.0 | 1741082.0 | 0.0343878 | 0.0825611 |
PRT A27 FPO1012 | 1086829.0 | 1139708.0 | 0.0463970 | 0.0863356 |
BEL A27 PMP1824 ° | 473171.6 | 495884.6 | 0.0458029 | 0.8846257 |
FRA A27 DRB0010 | 1406833.8 | 8547518.7 | 0.8354103 | 0.8850338 |
GBR A27 DRB0010 | 2022678.0 | 2657277.3 | 0.2388156 | 0.8903896 |
FRA A27 FPO1012 | 8163528.9 | 8435296.8 | 0.0322179 | 0.8913679 |
IRL A27 FPO0010 | 4408690.9 | 5406757.3 | 0.1845961 | 0.9163961 |
ESP A27 DRB1012 | 291963.2 | 293883.4 | 0.0065339 | 0.9236067 |
ESP A27 DRB1218 | 2284353.2 | 2285575.2 | 0.0005347 | 0.9291188 |
GBR A27 DRB1012 | 1972195.2 | 2077496.5 | 0.0506866 | 0.9356896 |
IRL A27 DRB2440 ° | 2004905.0 | 2004905.0 | 0.0000000 | 0.9635988 |
ITA A37 DRB1218 ° | 17394834.4 | 17772573.7 | 0.0212541 | 0.9687215 |
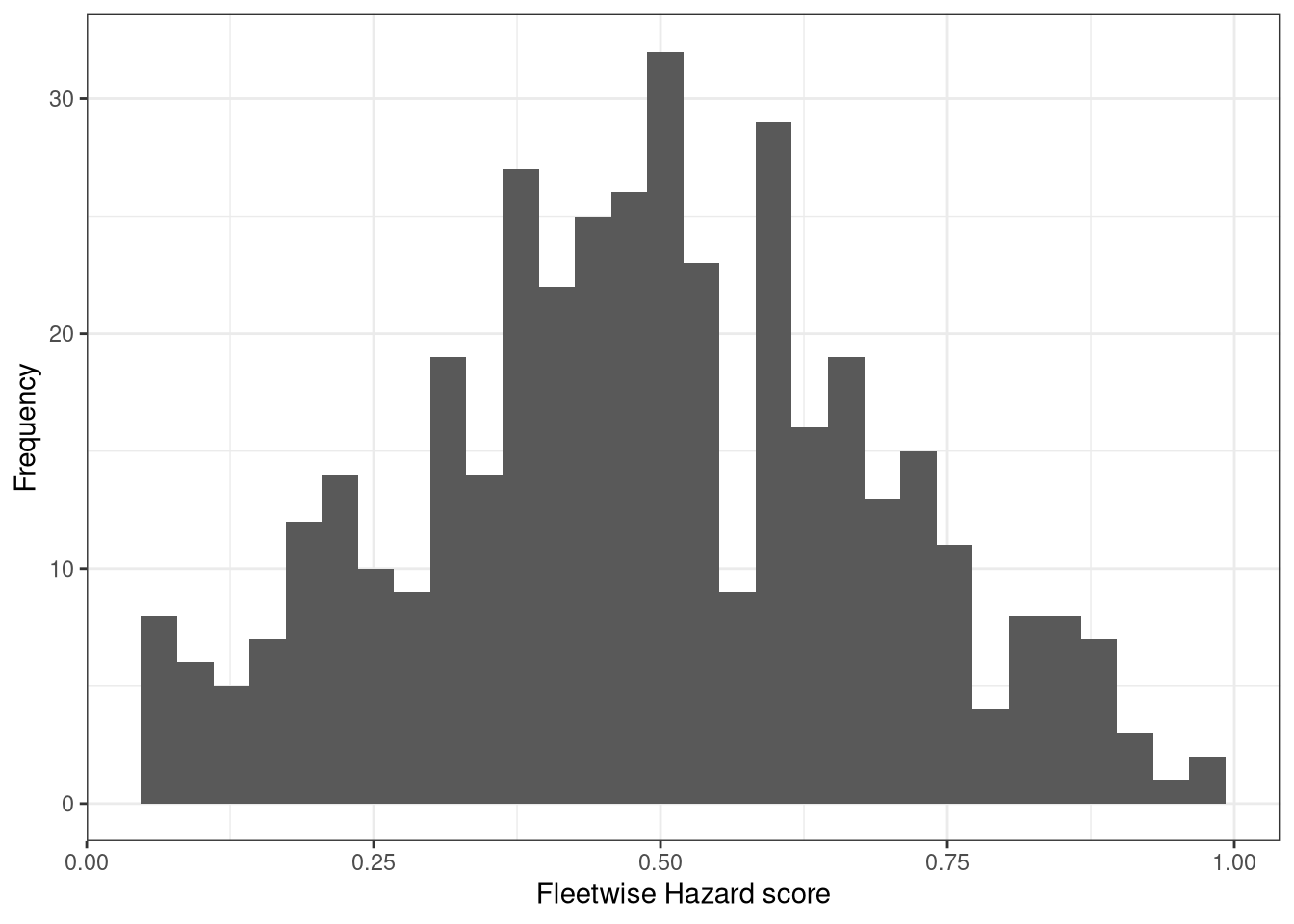
Figure 6: Distribution of hazard metrics
4.3.2 Fleet exposure metrics
We use the Shannon diversity and the Simpson Dominance metrics to characterise the diversity of species that a fishing fleet catching. The distribution of these metrics is as follows.
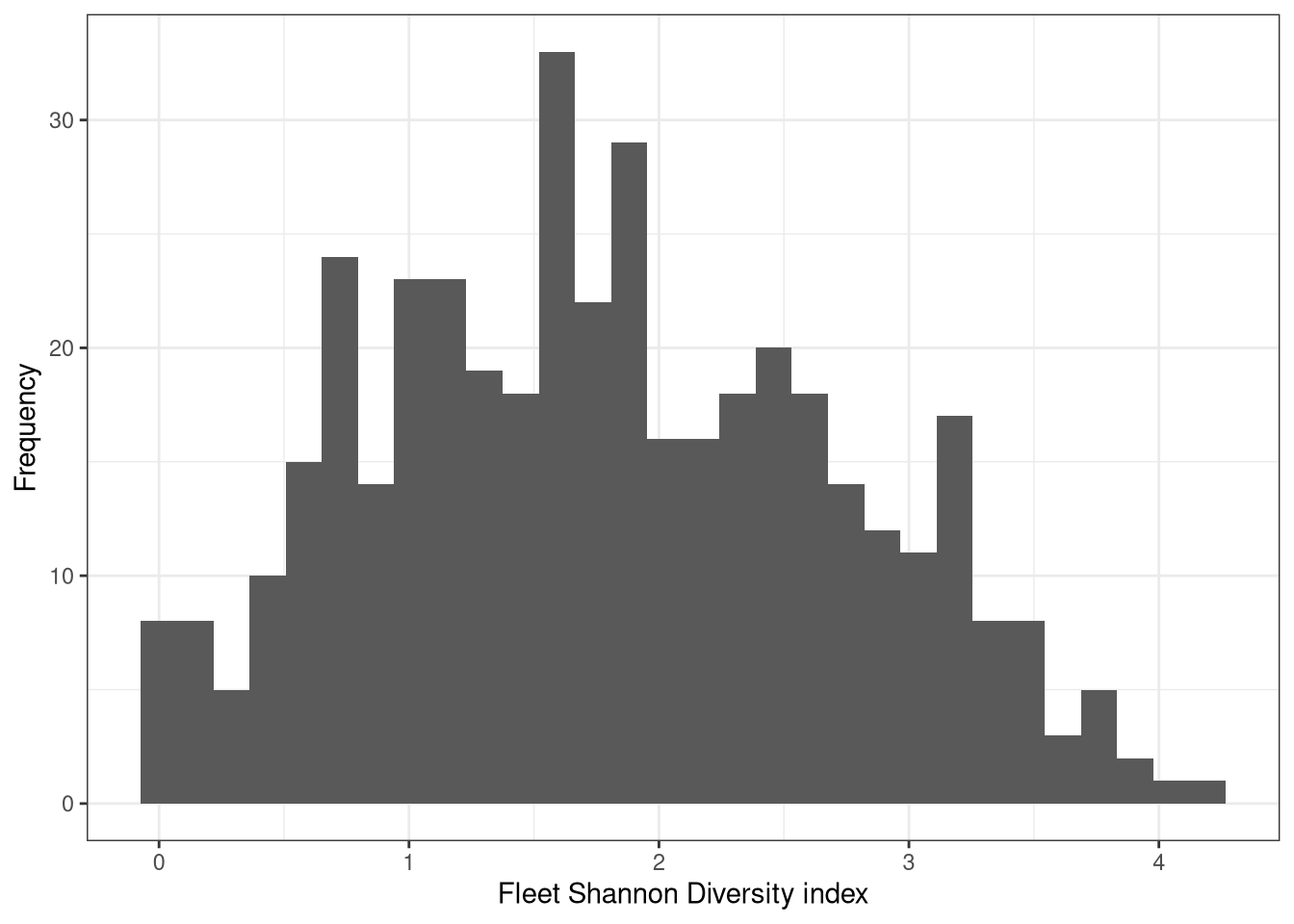
Figure 7: Fleetwsie Shannon Diversity index
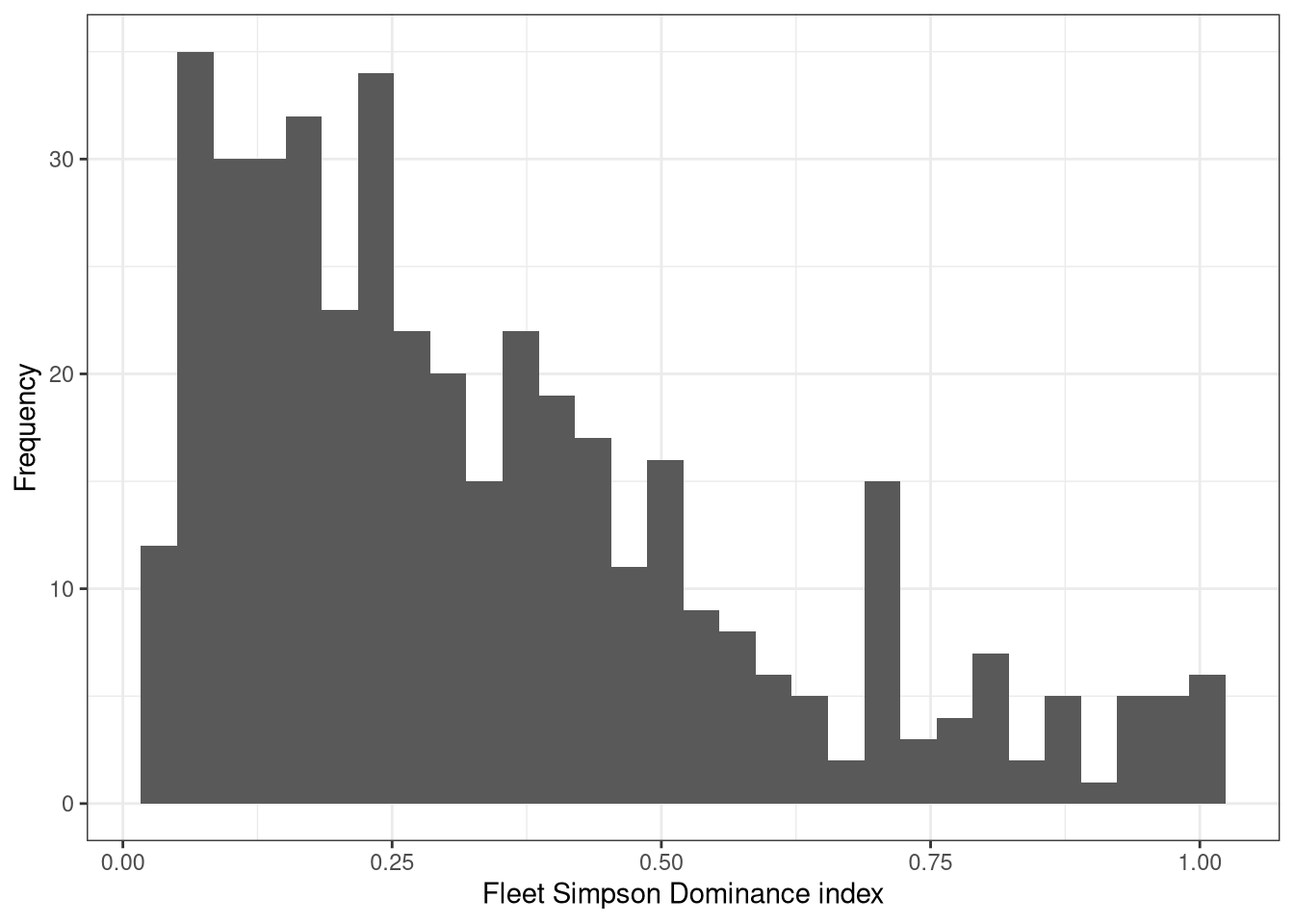
Figure 8: Fleetwise Simpson Dominance
A correlation between these two metrics is expected.
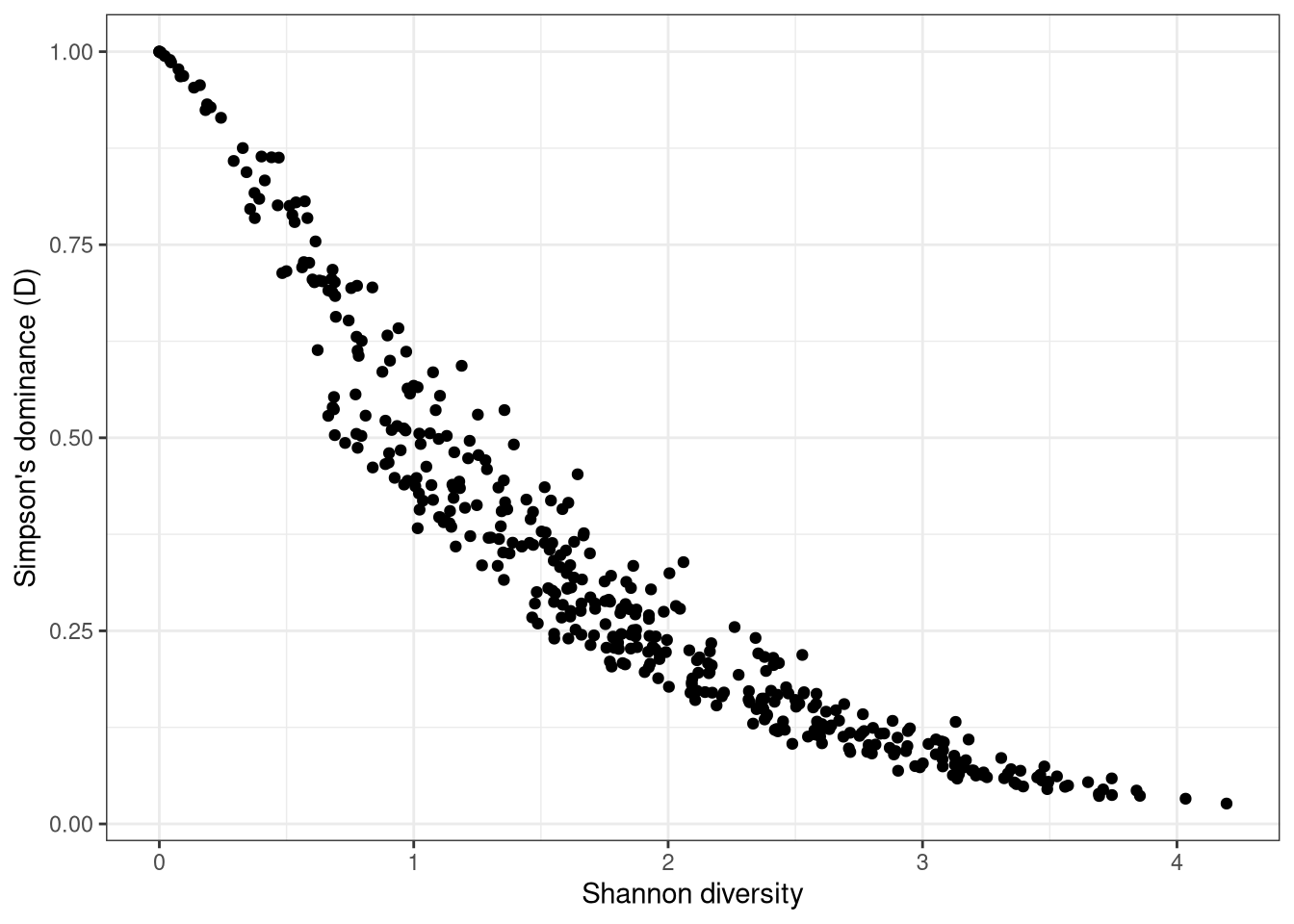
Figure 9: Relationship between Shannon Diversity H’ and Simpson’s Dominance D of fishery landings, for EU fleet segments in 2016
Clearly there is a strong relationship between Shannon diversity of fishery landings and Simpson’s dominance (D), although this is not linear (figure 1).
We combine the two metrics together into a into exposure metrics. Checking that we have done this correctly:
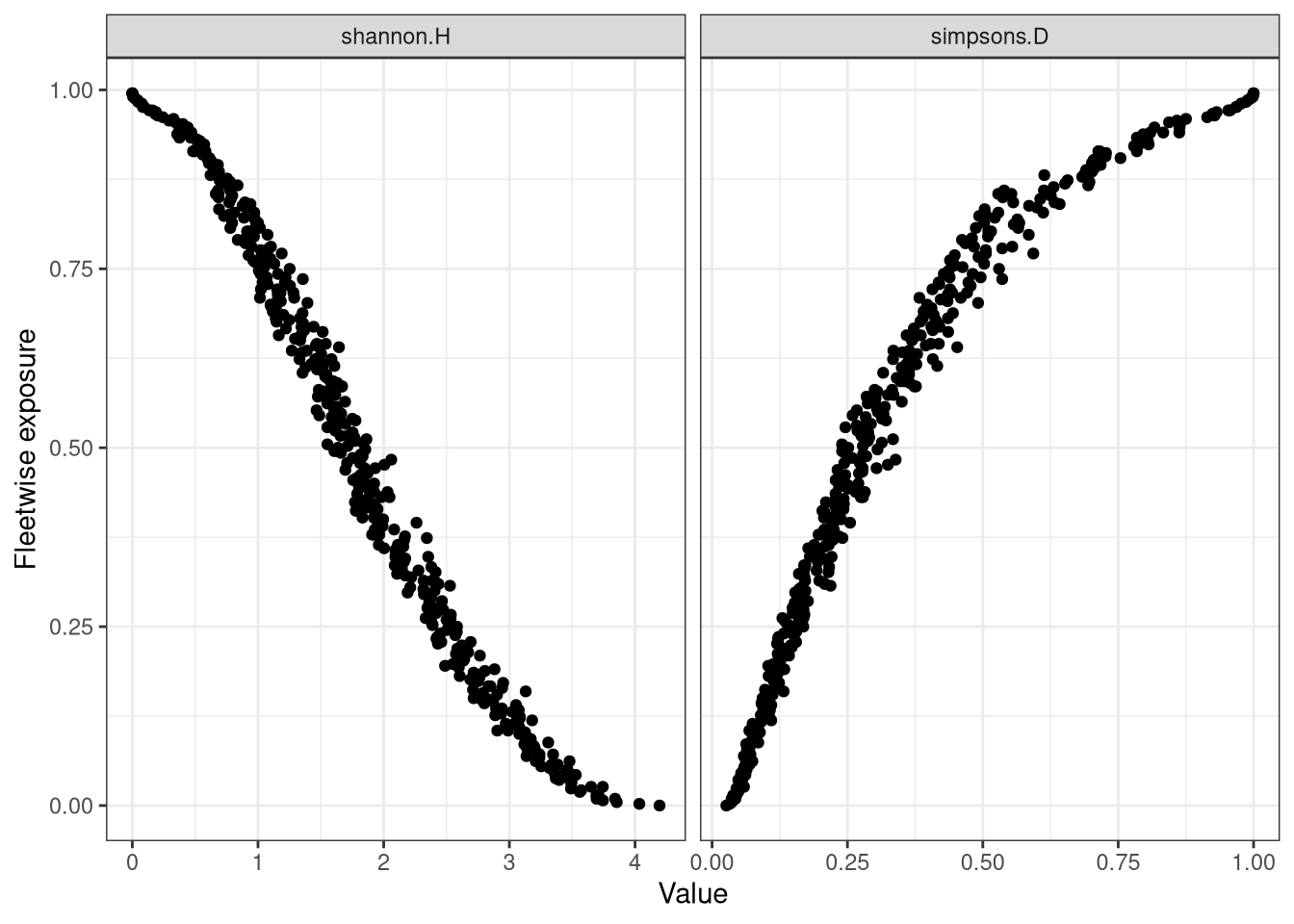
Figure 10: Exposure vs Shannon Index
So fleets with higher diversity have lower exposure. Tick. And fleets that are dominated by a few species have a higher exposure, which is what we want.
Plotting the list of fleet diversity.
Least diverse fleets
fs_name | sum.prop | sum.value | shannon.H | simpsons.D | n.species | exposure |
---|---|---|---|---|---|---|
IRL A27 DRB2440 ° | 1 | 2004905 | 0.0000000 | 1.0000000 | 1 | 0.9952381 |
MLT A37 PS2440 | 1 | 360500 | 0.0000000 | 1.0000000 | 1 | 0.9952381 |
PRT A37 FPO2440 | 1 | 116207 | 0.0000000 | 1.0000000 | 1 | 0.9952381 |
ESP A27 DRB1218 | 1 | 2285575 | 0.0049305 | 0.9989311 | 3 | 0.9928571 |
DEU A27 TBB1012 ° | 1 | 61863 | 0.0058449 | 0.9986430 | 3 | 0.9904762 |
ROU A37 PMP1218 ° | 1 | 4157697 | 0.0214070 | 0.9944113 | 10 | 0.9880952 |
Most diverse fleets
fs_name | sum.prop | sum.value | shannon.H | simpsons.D | n.species | exposure |
---|---|---|---|---|---|---|
ESP A37 PMP0612 | 1 | 4814591 | 3.743704 | 0.0589389 | 347 | 0.0261905 |
FRA A37 DFN0612 | 1 | 1337565 | 3.744439 | 0.0373679 | 203 | 0.0071429 |
ESP A37 DTS2440 | 1 | 5647283 | 3.841203 | 0.0431922 | 476 | 0.0095238 |
ITA A37 PGP0612 | 1 | 21059018 | 3.854408 | 0.0363553 | 135 | 0.0047619 |
ESP A37 DTS1218 | 1 | 4437824 | 4.033915 | 0.0326257 | 701 | 0.0023810 |
ESP A37 DTS1824 | 1 | 11824853 | 4.194966 | 0.0263463 | 746 | 0.0000000 |
Catch diversity in 2016 ranged from zero (where a fleet caught a single resource) to 4.19, where a multitude of different fish and shellfish species were targeted. The lowest diversity of catches was observed for the fleet segments IRL-A27-DRB2440, MLT-A37-PS2440 and PRT-A37-FPO2440, fishing exclusively on Great Atlantic scallop (Pecten maximus), Chub mackerel (Scomber colias) and Striped soldier shrimp (Plesionika edwardsii) respectively. By contrast, the highest diversity of landings was observed for ESP-A37-DTS1824, with catch records for 746 different species.
4.3.3 Fleet vulnerability metrics
Visualisation of the distribution of metrics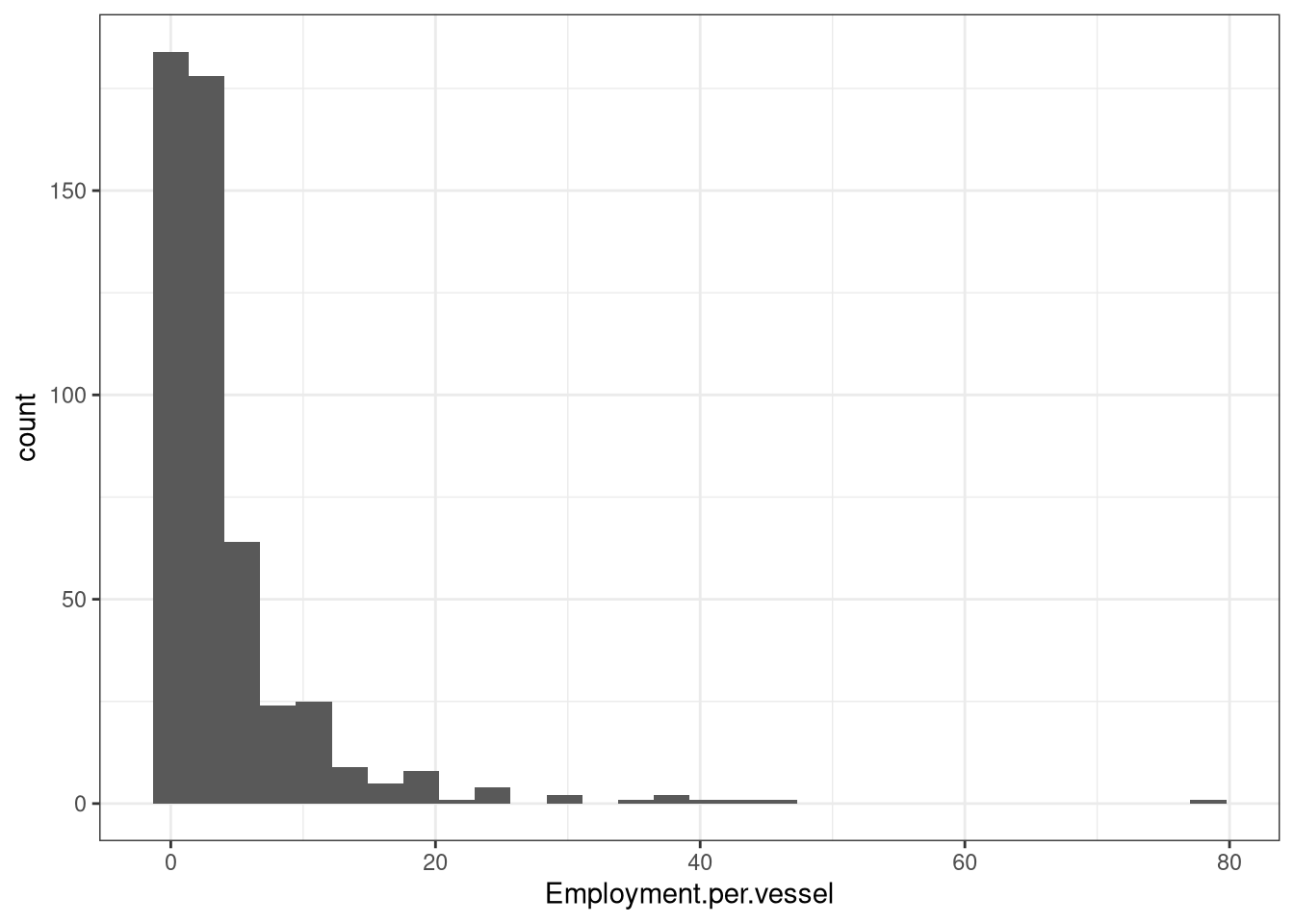
Figure 11: Employment per vessel
This is a potentially problematic metric, in retrospect, as it is really a metric of vessel size…
QUESTION: Should we retain it? How should we normalise it if we keep it?
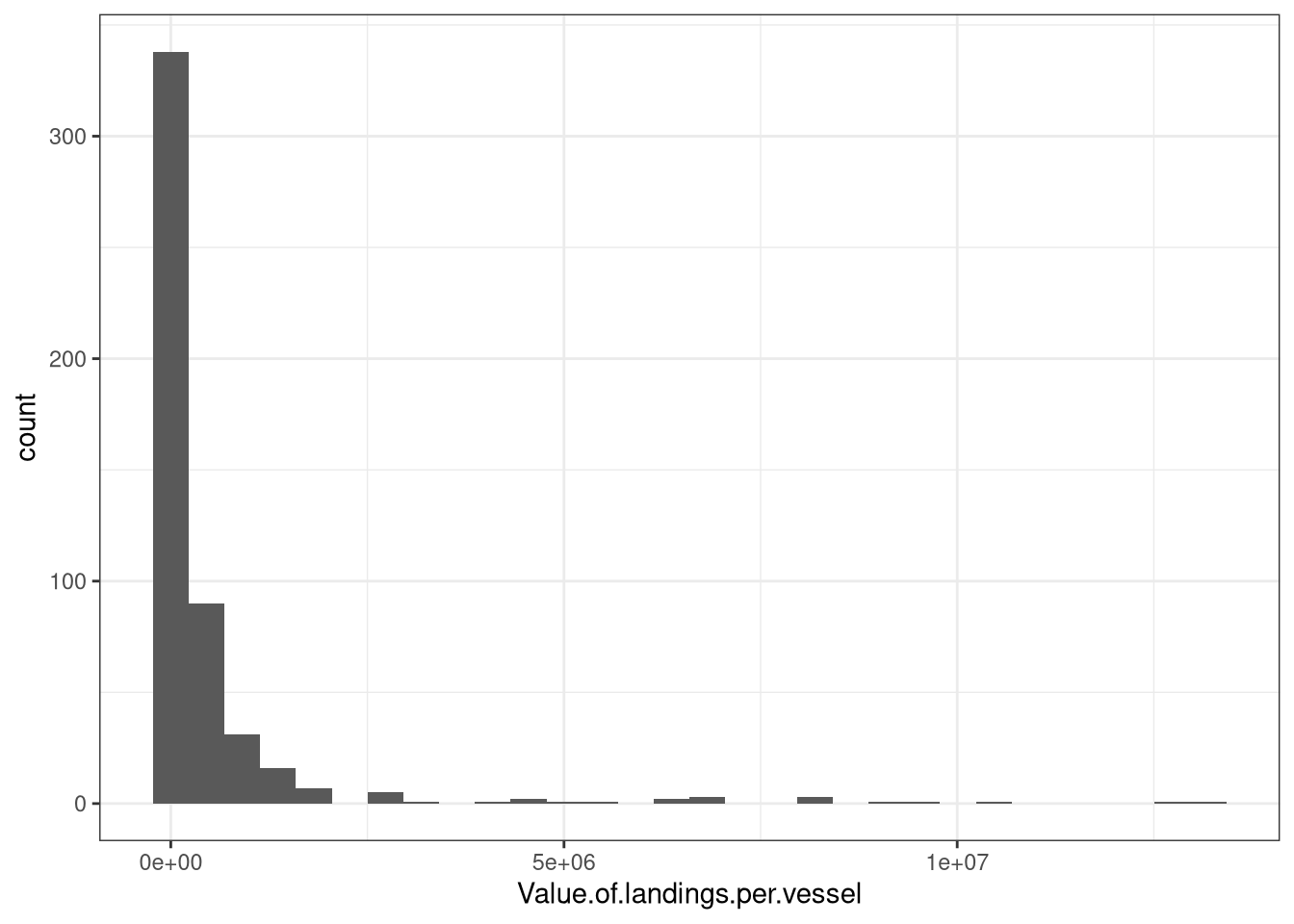
Figure 12: Value of landings
Hmmm. Similar problem.
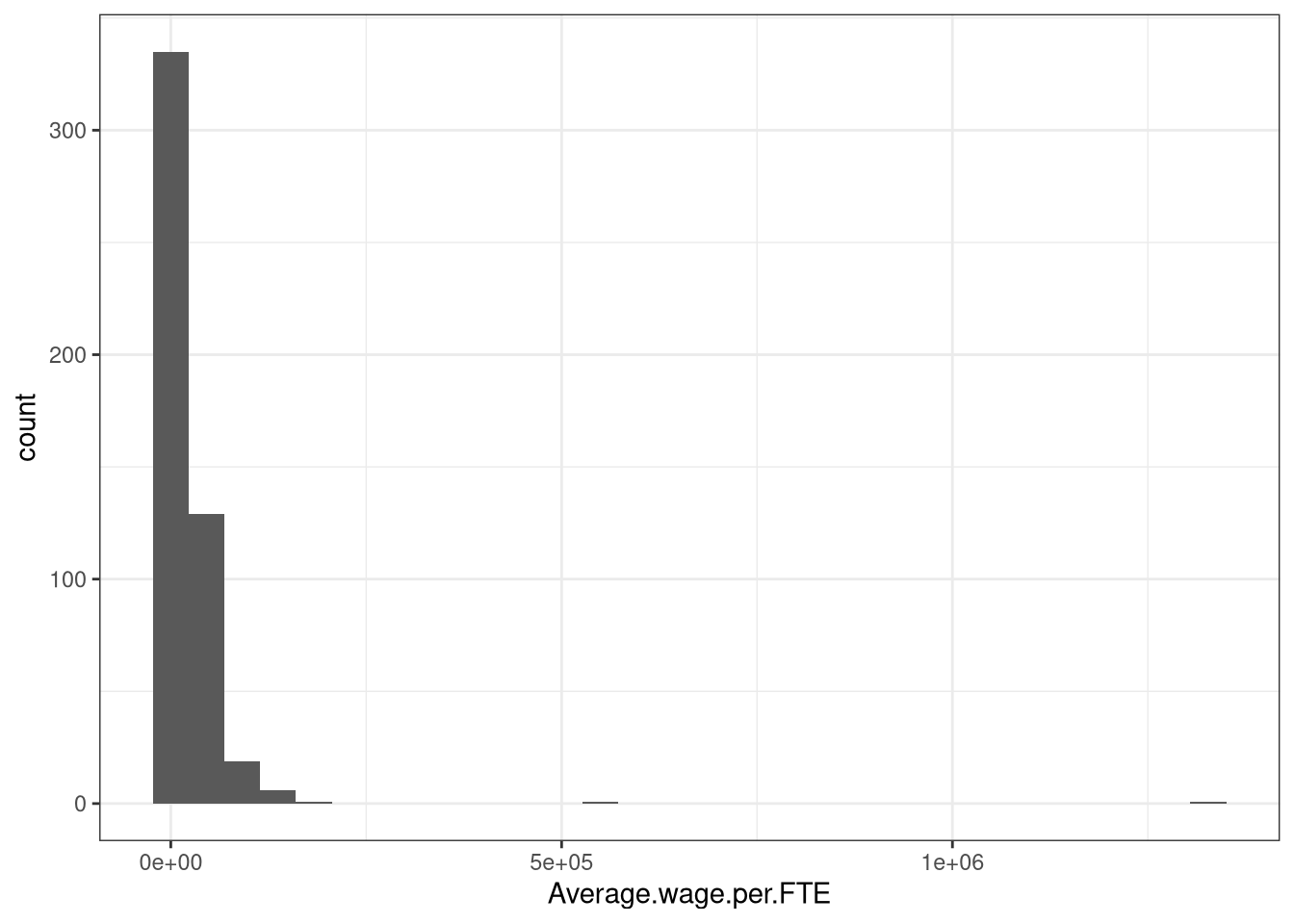
Figure 13: Average wage per FTE
Looks like a couple of outliers there that need to be polished up…
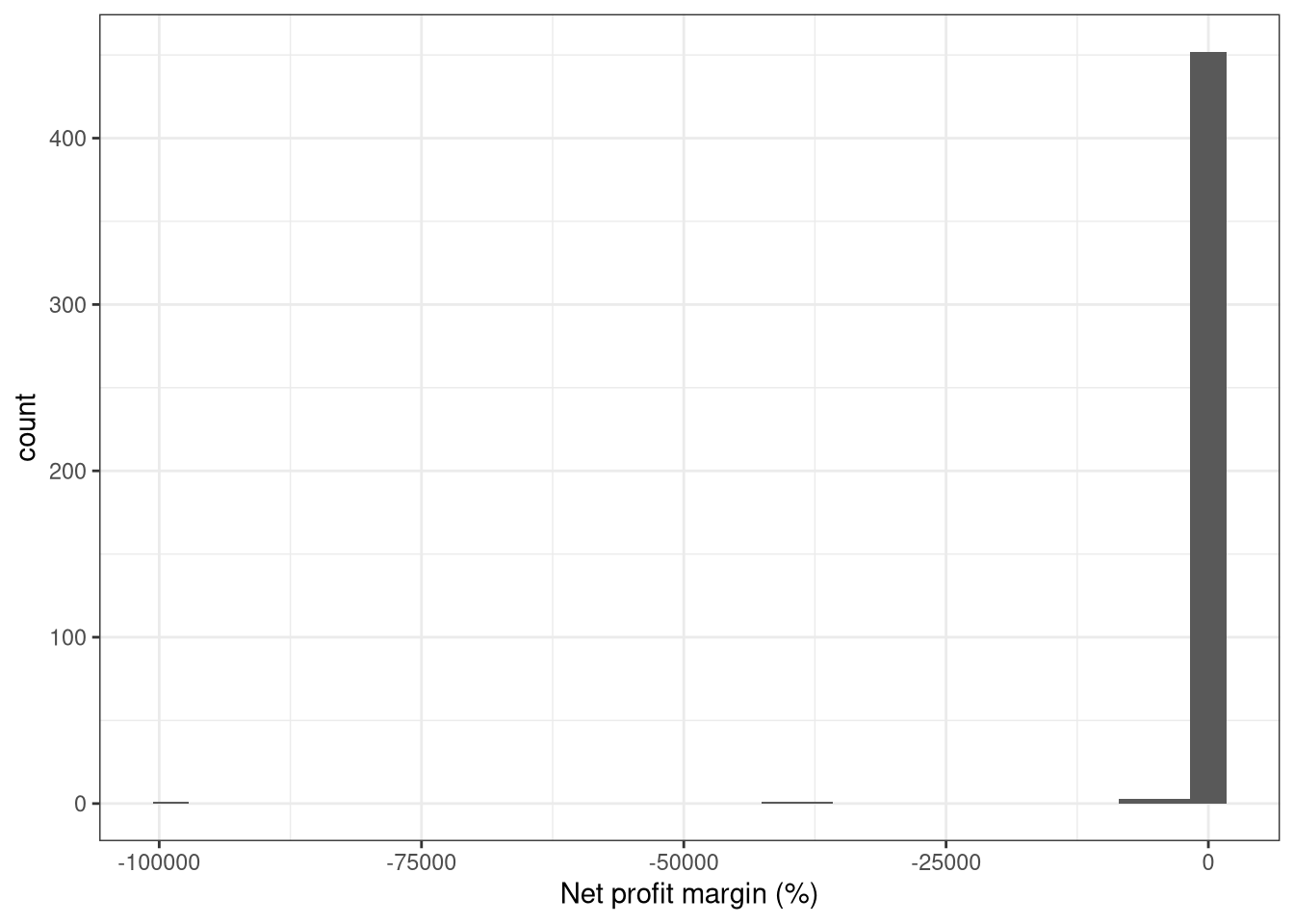
Figure 14: Net profit margin
Hmmm. A rather biased distribution. Lets crunch this figure down a bit…
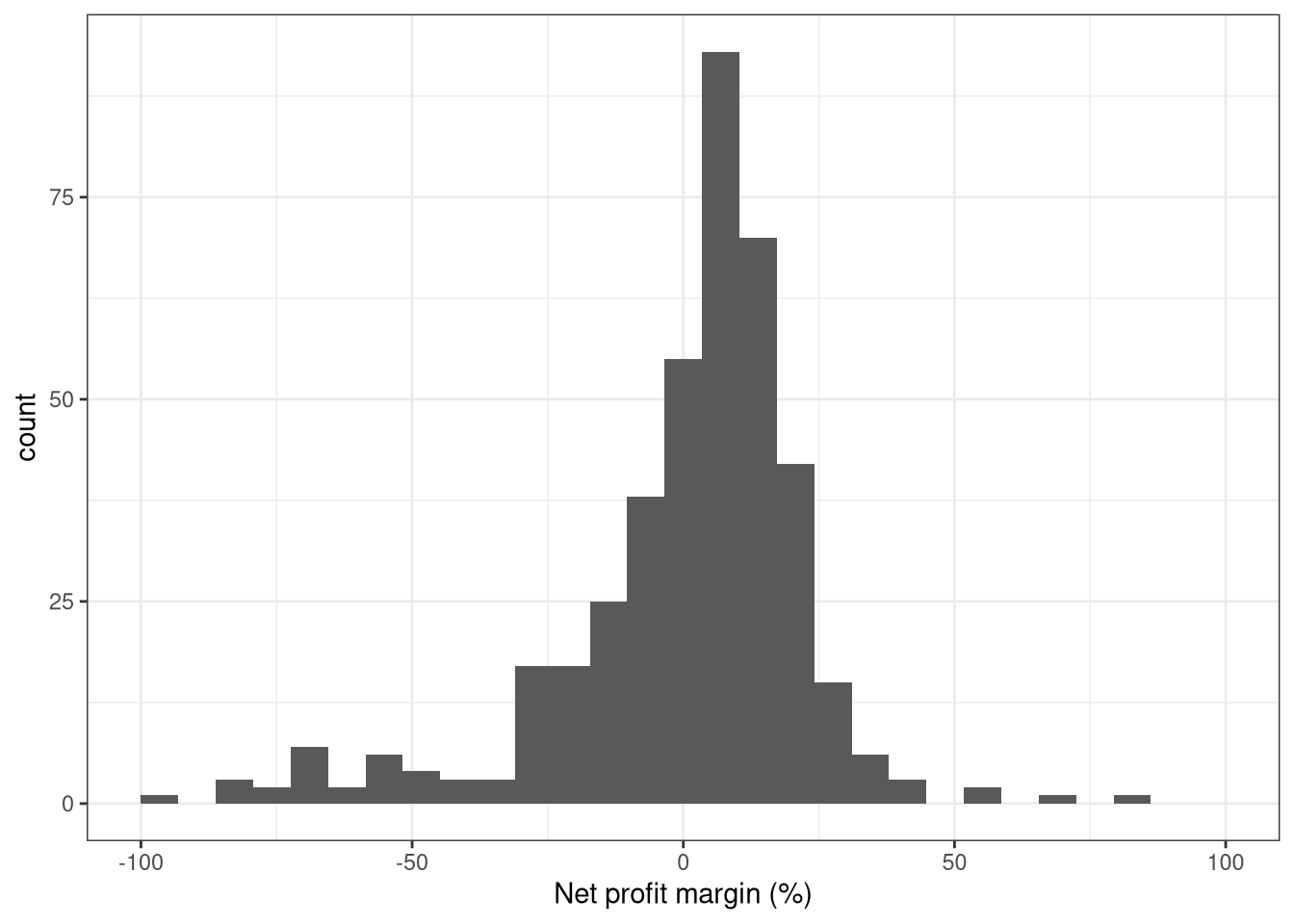
Figure 15: Net profit margin 2
Outliers aside, this is not a bad distribution.
QUESTION: Shall we rescale it to +/-50, and trim the rest…?
For the sake of this analysis, we have only based vulnerability on the Net Profit Margin, while we decide whether to include the rest..
Looking at the total vulnerability distribution.
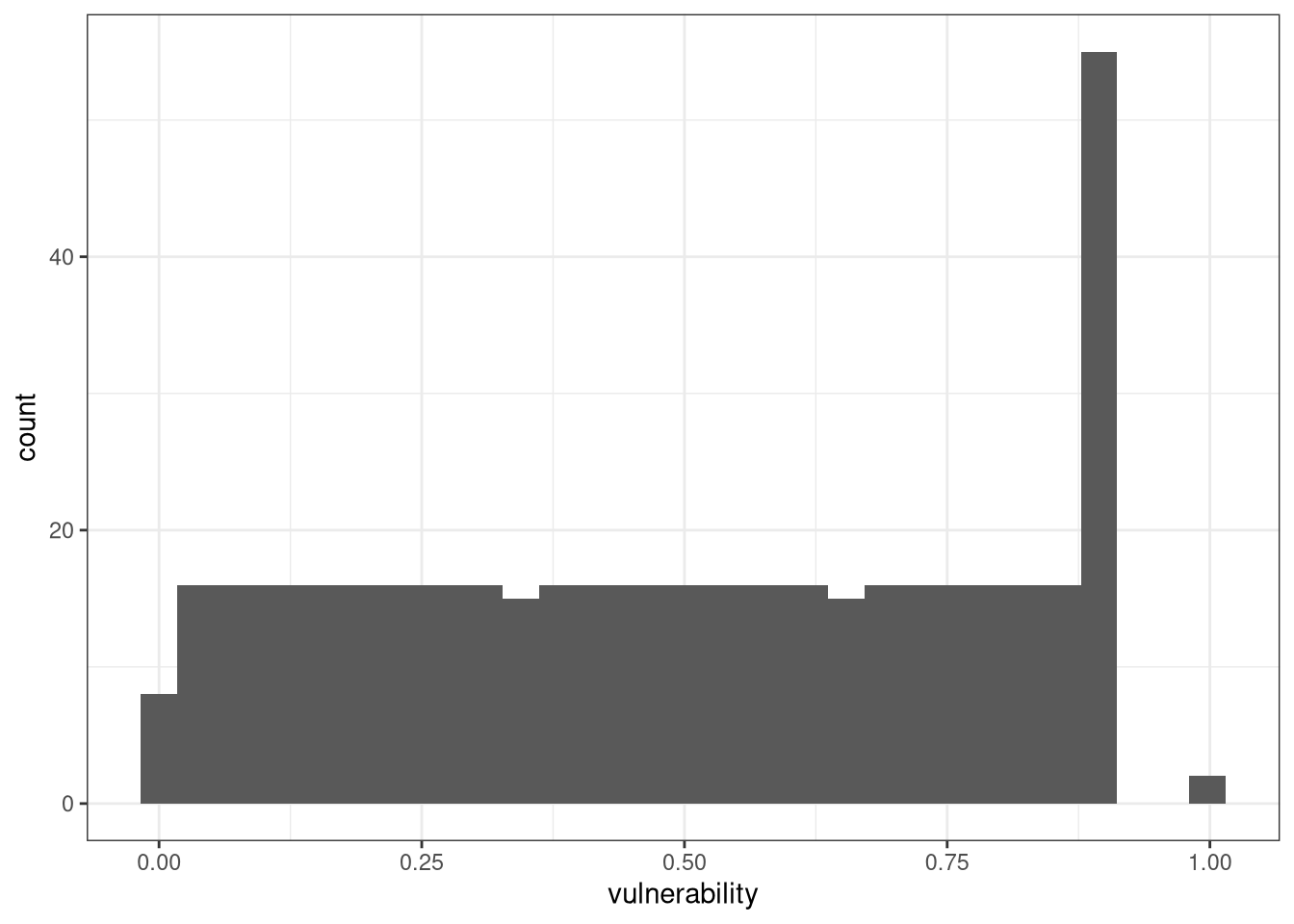
Figure 16: Vulnerability distribution
This doesn’t look so bad, but at the same time the outliers probably don’t help things, and should be fixed.
Checking the correlations with the metrics against vulnerability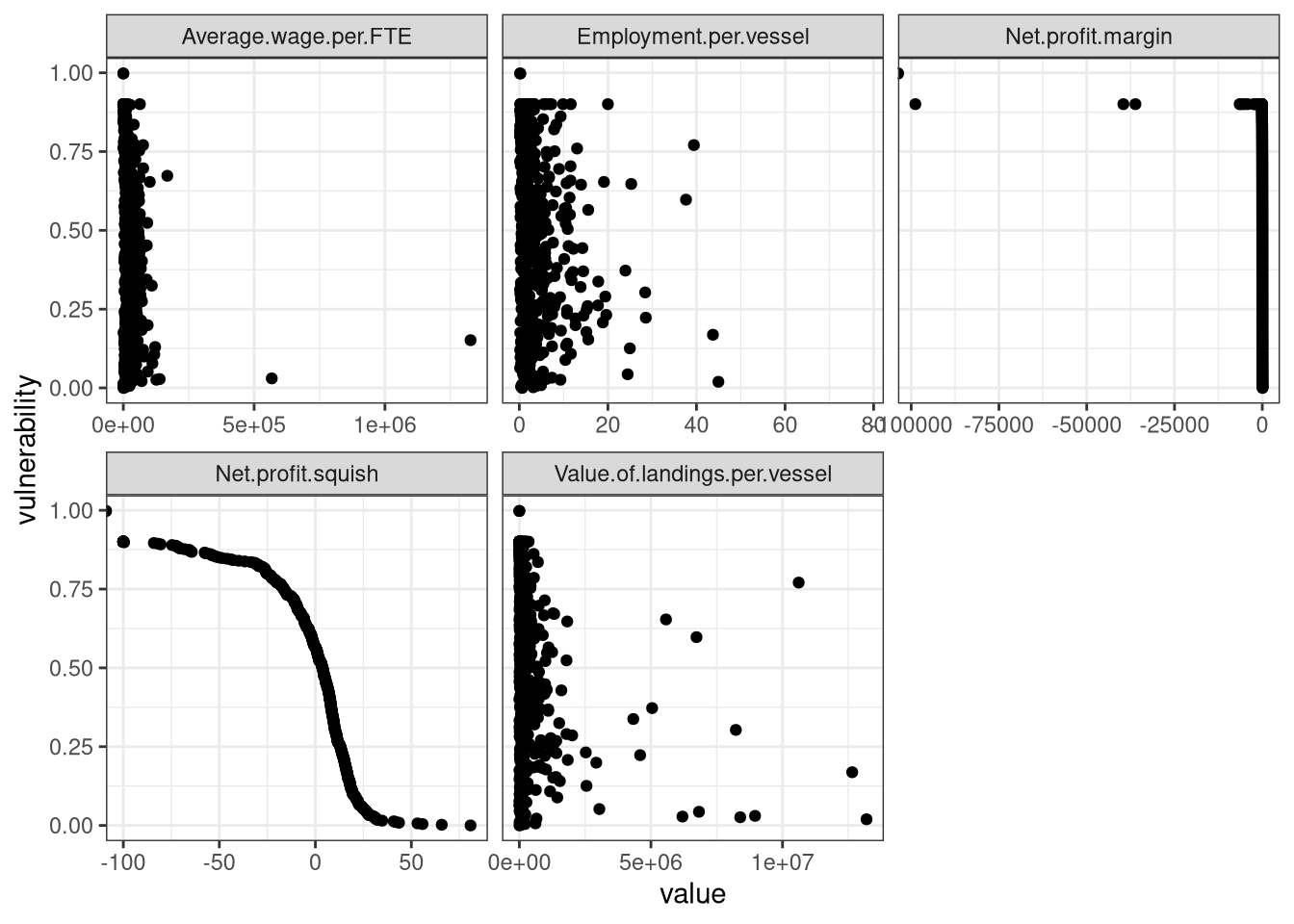
Figure 17: Correlation check
Which highlights the problems quite nicely… There is also some issues here with the directionality that we need to fix.
I think we probably need to rethink these metrics. Net profit margin is a very useful metric, but I’m not so sure about the others, particularly as they scale with vessel size, which I’m not sure we realy want…
4.3.4 Fleet risk
Note that the results here are a bit meaningless until we get the vulnerability under control, but the visualisations will be the same, so we can look at and discuss how we want to approach these.
Firstly, the top and bottom 10
fs_name | country | area | gear | length | geo | total.val.landings | prop.missing | hazard | exposure | vulnerability | risk | country_name | country_2A |
---|---|---|---|---|---|---|---|---|---|---|---|---|---|
DNK A27 TM40XX | DNK | A27 | TM | 40XX | 363359393.3 | 0.007 | 0.050 | 0.536 | 0.020 | 0.000 | Denmark | DK | |
ITA A37 PGP0006 | ITA | A37 | PGP | 0006 | 5721461.6 | 0.459 | 0.573 | 0.015 | 0.042 | 0.003 | Italy | IT | |
PRT A27 DFN1012 | PRT | A27 | DFN | 1012 | 356623.0 | 0.390 | 0.449 | 0.032 | 0.154 | 0.006 | Portugal | PT | |
PRT A27 DTS0010 | PRT | A27 | DTS | 0010 | 381875.0 | 0.275 | 0.491 | 0.141 | 0.025 | 0.008 | Portugal | PT | |
ESP A37 DFN0612 | ESP | A37 | DFN | 0612 | 630328.7 | 0.477 | 0.578 | 0.027 | 0.081 | 0.011 | Spain | ES | |
ESP A37 DRB1218 | ESP | A37 | DRB | 1218 | 167984.4 | 0.460 | 0.112 | 0.387 | 0.213 | 0.014 | Spain | ES | |
PRT A27 PGP0010 | PRT | A27 | PGP | 0010 | 9661098.0 | 0.206 | 0.323 | 0.176 | 0.230 | 0.017 | Portugal | PT | |
ESP A27 PS2440 | ESP | A27 | PS | 2440 | 34961229.8 | 0.174 | 0.146 | 0.333 | 0.258 | 0.020 | Spain | ES | |
PRT A27 TBB0010 | PRT | A27 | TBB | 0010 | 263508.0 | 0.439 | 0.367 | 0.149 | 0.249 | 0.022 | Portugal | PT | |
ITA A37 PMP1218 ° | ITA | A37 | PMP | 1218 | ° | 449075.6 | 0.242 | 0.186 | 0.444 | 0.148 | 0.025 | Italy | IT |
IRL A27 TM0010 ° | IRL | A27 | TM | 0010 | ° | 114528.2 | 0.069 | 0.134 | 0.916 | NA | NA | Ireland | IE |
IRL A27 TM1218 ° | IRL | A27 | TM | 1218 | ° | 447382.0 | 0.607 | 0.221 | 0.692 | NA | NA | Ireland | IE |
IRL A27 TM2440 | IRL | A27 | TM | 2440 | 31648490.3 | 0.067 | 0.087 | 0.429 | NA | NA | Ireland | IE | |
IRL A27 TM40XX | IRL | A27 | TM | 40XX | 125299317.0 | 0.067 | 0.067 | 0.613 | NA | NA | Ireland | IE | |
POL A27 DTS40XX | POL | A27 | DTS | 40XX | 6263477.9 | 0.012 | 0.266 | 0.896 | NA | NA | Poland | PL | |
POL OFR TM40XX | POL | OFR | TM | 40XX | 53086199.0 | 0.635 | 0.826 | 0.769 | NA | NA | Poland | PL | |
PRT A27 DTS1012 | PRT | A27 | DTS | 1012 | 269941.0 | 0.392 | 0.591 | 0.117 | NA | NA | Portugal | PT | |
PRT A27 PGP1824 | PRT | A27 | PGP | 1824 | 167591.0 | 0.188 | 0.094 | 0.489 | NA | NA | Portugal | PT | |
PRT A27 TBB1012 ° | PRT | A27 | TBB | 1012 | ° | 268783.0 | 0.611 | 0.243 | 0.313 | NA | NA | Portugal | PT |
PRT OFR HOK2440 IWE° | PRT | OFR | HOK | 2440 | IWE° | 4408259.0 | 0.766 | 0.923 | 0.600 | NA | NA | Portugal | PT |
Now, viewing by vessel size
By country and fishing area.
QUESTION: I’m not quite sure how whether to retain the other fishing region fleets, or just keep it to the FAO27 and FAO37 areas - we don’t have very much biological information outside these regions.
QUESTION: I can also plot this as a map, based on the median value…
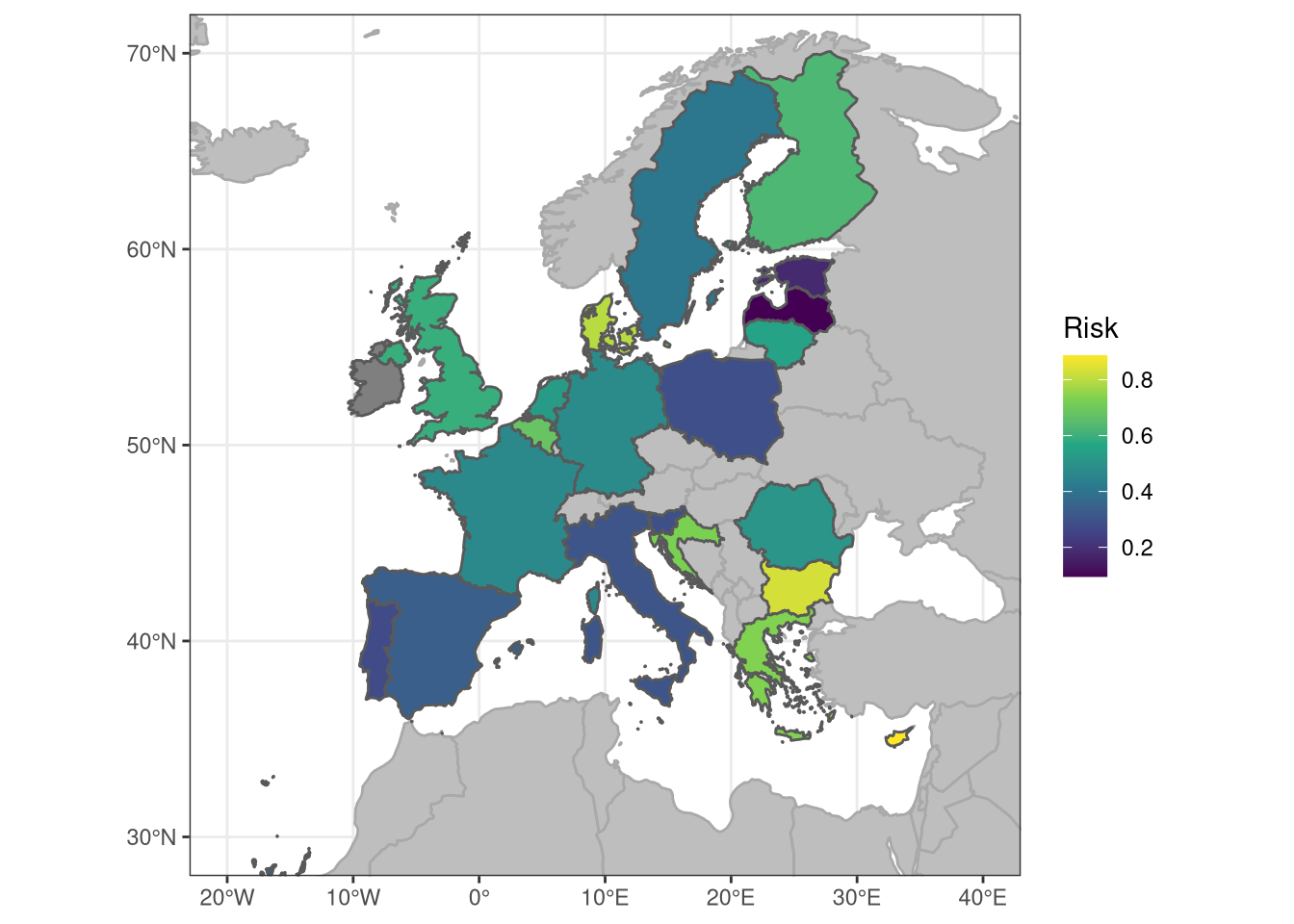
Figure 18: Fleetwise climate risk for the 23 EU coastal nations
TODO: Ireland needs to be investigated - it looks like it is missing some of the flet economic data => no vul => no risk.
TODO: I will separate France and Spain into Atlantic and Mediterranean fleets as well on this map
And by gear. Looks like quite some variability here.
A table of gear types, sorted by median risk.
Code | Description | Risk |
---|---|---|
DRB | Dredgers | 0.845 |
PGO | Vessels using other passive gears | 0.706 |
TBB | Beam trawlers | 0.661 |
FPO | Vessels using pots and/or traps | 0.623 |
MGO | Vessel using other active gears | 0.585 |
PMP | Vessels using active and passive gears | 0.583 |
DFN | Drift and/or fixed netters | 0.574 |
MGP | Vessels using polyvalent active gears only | 0.487 |
HOK | Vessels using hooks | 0.466 |
PGP | Vessels using polyvalent passive gears only | 0.450 |
PS | Purse seiners | 0.380 |
DTS | Demersal trawlers and/or demersal seiners | 0.375 |
PG | Passive Gears | 0.331 |
TM | Pelagic trawlers | 0.276 |
4.4 Regional Metrics
4.4.1 Regional hazard
4.4.2 Regional exposure
Similar relationships between the catch dominance and catch diversity are seen at the regional levels as are seen for the fleets.
Check on the scaling and tendency
Plotting the exposure score geographically
Note the clear north-south gradient here. Several countries are still missing from this analysis, as we don’t have data resolved by NUTS regions for them (e.g. Norway, Finland, parts of Sweden, SE Europe). We need to find a strategy how to deal with this.
4.4.3 Regional vulnerability
Currently this is only based on GDP per capita. Other metrics can be added. Plotting the distribution of values.
Checking the scaling and directionality
Distribution map.
4.4.4 Regional risk
We calculate risk as the average of the hazard, exposure and vulnerability scores. This may need to be improved. The top and bottom ten regions by risk are then
region | hazard | exposure | vulnerability | risk | country |
---|---|---|---|---|---|
IE05 | 0.490 | 0.146 | 0.000 | 0.000 | IE |
NO | 0.135 | 0.534 | 0.031 | 0.010 | NO |
ITI4 | 0.394 | 0.049 | 0.286 | 0.020 | IT |
UKM5 | 0.221 | 0.466 | 0.051 | 0.031 | UK |
NL32 | 0.115 | 0.612 | 0.041 | 0.041 | NL |
NL33 | 0.067 | 0.592 | 0.112 | 0.051 | NL |
DE50 | 0.096 | 0.621 | 0.071 | 0.061 | DE |
FRE1 | 0.173 | 0.087 | 0.561 | 0.071 | FR |
FRG0 | 0.279 | 0.126 | 0.418 | 0.082 | FR |
ITI1 | 0.413 | 0.019 | 0.429 | 0.092 | IT |
UKE1 | 0.942 | 0.718 | 0.510 | 0.969 | UK |
UKM8 | 0.990 | 0.903 | 0.296 | 0.980 | UK |
RO | 0.298 | 0.961 | 0.990 | 0.990 | RO |
UKC1 | 0.971 | 0.835 | 0.653 | 1.000 | UK |
BE25 | 0.250 | 0.563 | NA | NA | BE |
EE00 | 0.010 | 0.854 | NA | NA | EE |
EL30 | 0.558 | 0.194 | NA | NA | EL |
IS | 0.625 | NA | NA | NA | IS |
LT02 | 0.885 | 0.845 | NA | NA | LT |
LV00 | 0.038 | 0.864 | NA | NA | LV |
Putting it on a map
Putting it up as a boxplot
Interesting results. Lots of details there to discuss!