2 Text mining as a tool for literature synthesis in Ecology
Text mining refers to the “automatic discovery of previously unknown information from unstructured textual data” (Hearst 1999). A typical text mining process looks forward to extracting and derive new information from an input of text based on particular characteristics of the text such as word patterns and frequencies. This process aggregates a different set of statistical, linguistic, database processing and machine learning techniques to analyze a natural lenguage written text, to model classification predictions and/or create new databases with the extracted information.
Text mining has been broadly applied to interdisciplinary fields such as marketing, social media, history, government, etc. In Sciences, text mining techniques have been particularly successful in the field of bio-medicine, with most of the research development in this field prompted due to the increasing availability of digital biomedical literature and databases (Krallinger et al., 2005). The use of text mining in biomedicine is vast and has applications ranging from document classification to the identification of novel drug targets, potential novel protein functions and protein - protein interactions (Krallinger et al., 2005; Korkontzelos et al. 2016) (Figure 2.1). An overview of some software tools used for applications of text mining on Biomedical Sciences can be found in the UK’s National Centre for Text Mining (NaCTem) [website] (http://www.nactem.ac.uk/software.php). (NaCTem - University of Manchester. 2016)
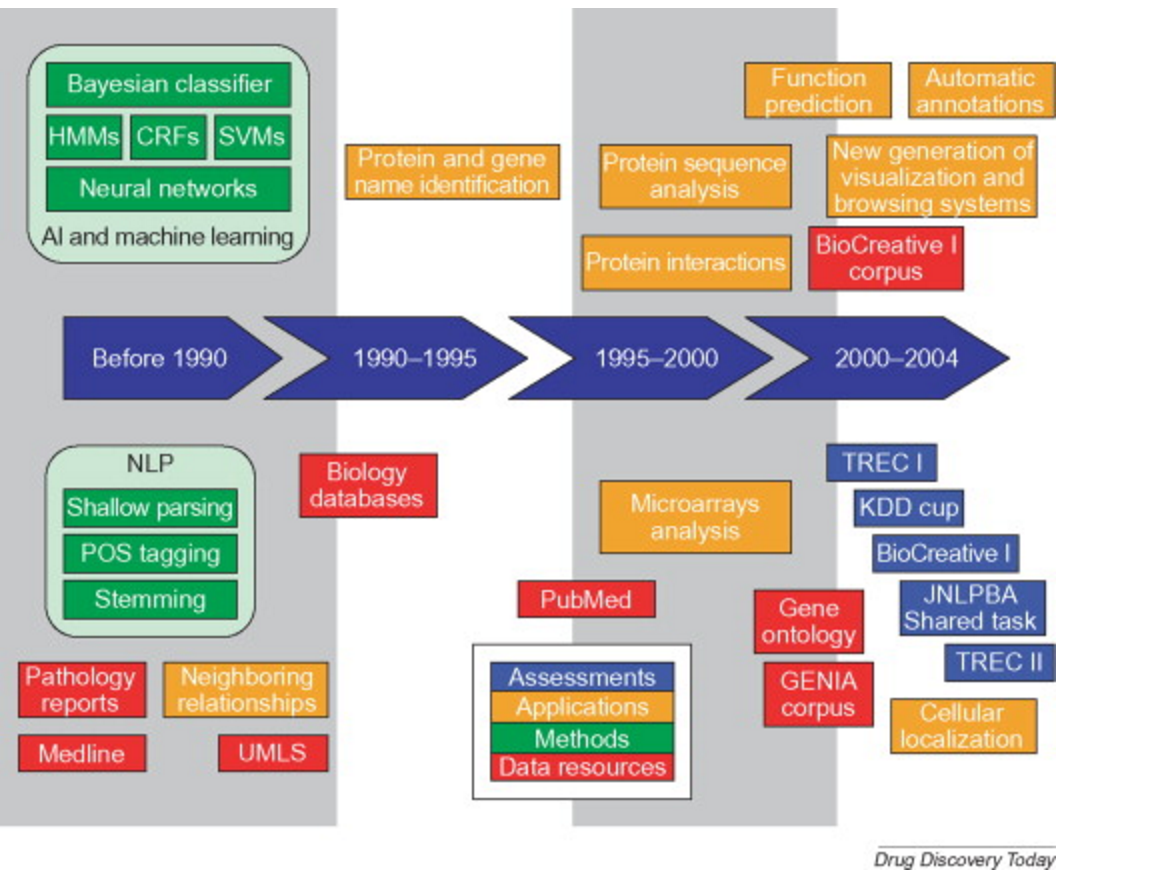
Figure 2.1: Historical (1990 - 2004) perspective on the use of Text mining and Natural Language Processing techniques in biomedicine. (obtained from Krallinger et al. (2005)
The current body of published literature in Ecology, despite known shortfalls (Martin 2012; Proença et al. 2016; Wilson et al. 2016), holds most of the available information about Earth’s biodiversity and represents centuries of ecological research (Thessen et al. 2016). Projects like the Biodiversity Heritage Library (BHL) have made an extensive effort to increase the digital availability of old biology texts with over than 33 million pages scanned and published online in their website. Applications of text mining techniques in Ecology has been much more limited than in bio-medicine. Nonetheless, the interest is currently increasing as the field moves towards becoming a data - driven science, generating new insights and hypothesis by the analysis of pre-existing data (Thessen et al. 2012; 2016; Poissot et al. 2015).
The access to the body of research literature in Ecology, creates many challenges related to the discovery, standardization and digitization of data. Published ecological literature is heterogeneous in nature (Thessen 2012) (Figure 2.2) and most of digitized literature is available as merely a digital copy of the original article (Lyal 2016). Articles now are typically published in PDF format and even though there is an increasing trend of published literature and data available through open - access journals (Björk et al. 2009; Reichman et al. 2011; Thessen et al. 2016), there is still a good portion of the published literature locked behind paywalls (Caroll & McArthur. 2013). The internet facilitates the accessibility of scientific articles. However, our current way of obtaining literature may carry severe bias implications as the process of a literature search becomes unreproducible, unstandardized and dependent from the particular query abilities and access credentials (via institutions or personal) of the researcher.
API’s are pieces of software that allows communication between sofware applications. There still discussion about openess vs privatization of API’s (Gangadharan 2009) but the usefulness of them is out of debate. The use of API’s have greatly contributed to the recent development of new software applications as it allows the use of specific functions already developed to complement the development of a new one. There is a wide variety of API’s available for all different set of tasks related with the search of information in Ecology (Appendix Table 1). Likewise, several packages, written in different languages are continuously being developed allowing the integration of many functionalities and pieces of software for specific purposes.
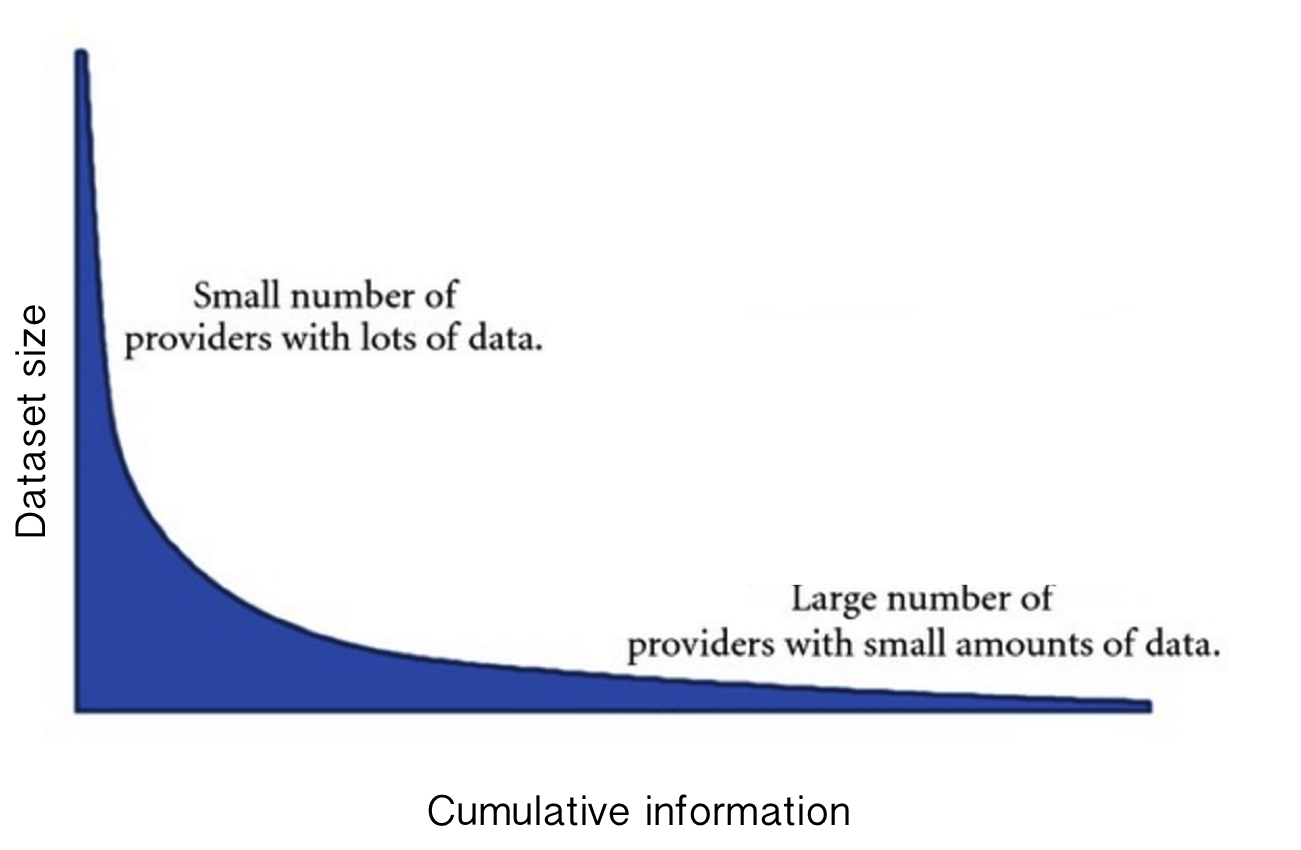
Figure 2.2: Long tail on dataset size distribution in Biology. Big datasets are concentrated on few sources with limited information; whereas large amounts of information is scattered on many sources with small dataset sizes. (modified from Thessen et al. (2012))