Chapter 2 Transcriptomic Profile of RP in Retinal Bipolar Cells
2.1 Introduction
The retina is relatively stable both structurally and functionally after undergoing development. Proper function relies on structure in terms of the synaptic connections between cells of various types. Cellular and molecular mechanisms responsible for the establishment and refinement of these connections during development have been well documented (Zhang et al. 2017; Sanes and Yamagata 2009; Riccomagno and Kolodkin 2015; Margeta and Shen 2010; Luo et al. 2016; Wit and Ghosh 2016). Disease, however, disrupts the stability in adult retina circuitry (Marc and Jones 2003; Marc et al. 2003). Questions about how to repair these circuitry changes is a significant factor that limits the efficacy of repairing vision via gene therapy and/or stem cell therapy (Scholl et al. 2016; Klassen 2016; Garg et al. 2017; Day et al. 2014; Boye et al. 2013).
Many experiments are undertaken with the hope of elucidating the circuitry changes from retinal degeneration. Perhaps the most important of these changes is the rewiring of bipolar cells: second-order cells in the retina that receive input from photoreceptors. Bipolar cell rewiring is likely one source for improving therapies (Day et al. 2014; Boye et al. 2013). This is because replacing lost photoreceptors or halting photoreceptor death does not guarantee that synaptic connections with bipolar cells will return to normal. Without normal synaptic transmission, patients who receive treatment may remain blind.
In this section of the thesis, we will identify changes in gene expression that occur in bipolar cells during photoreceptor degeneration and following photoreceptor rescue, and to identify candidate genes that may halt or reverse bipolar cell rewiring (see Fig 1). Specifically, we will compare gene expression profiles among bipolar cells harvested from healthy retinas, harvested at different stages of degeneration, and harvested following a genetic rescue from photoreceptor degeneration.
2.2 Methods
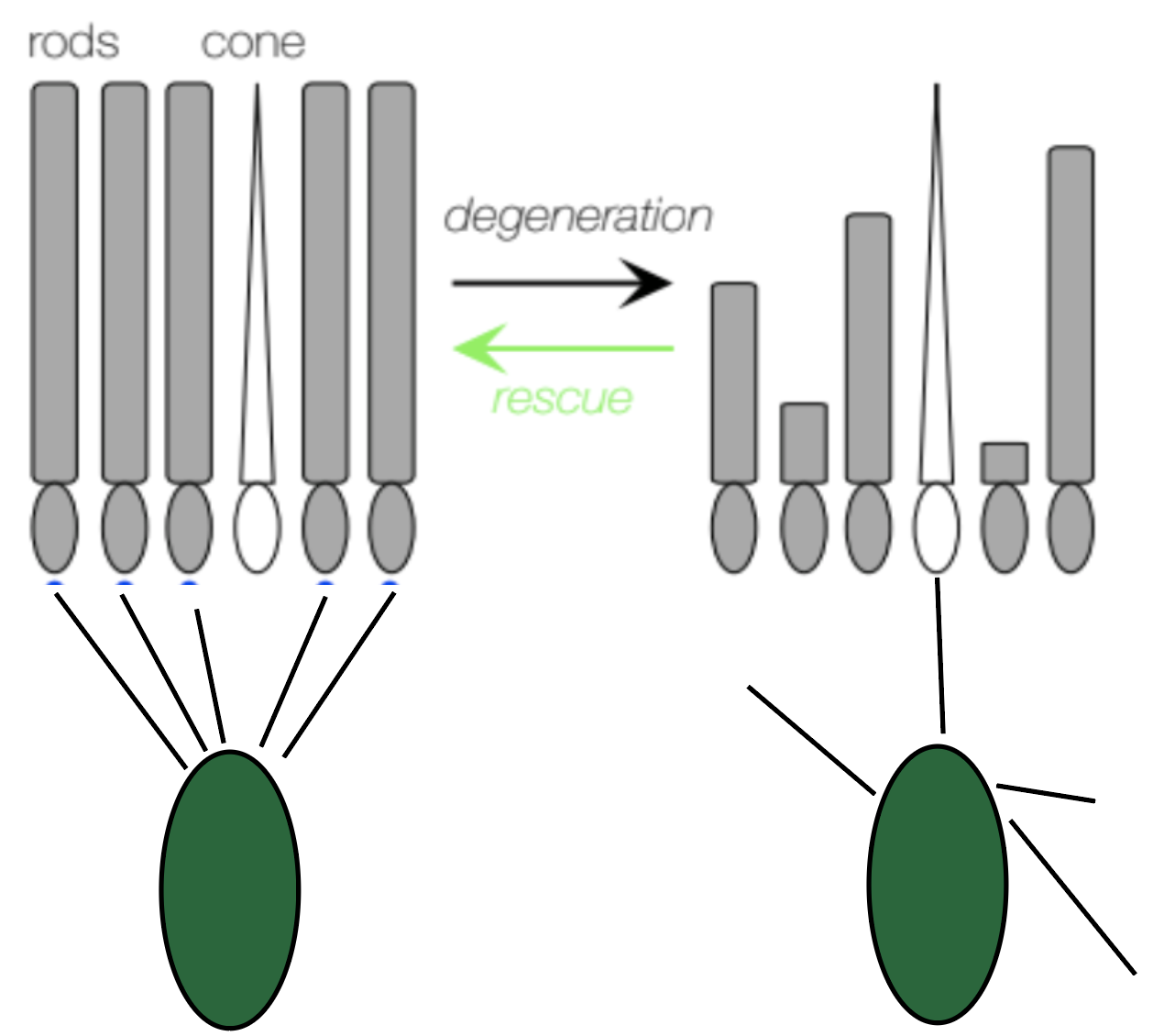
Figure 2.1: Graphical abstract illustrating changes in rods and bipolar cells during degeneration and following rescue.
We will use the CNGB1 mouse model, but will also genetically rescue some mice. The neoloxP cassette that induces RP can be removed through cre-mediated recombination. To obtain temporal control of Cre-mediated rod rescue, we have crossed these mice with CAG-CreER mice. Offspring mice (henceforth, called Cngb1neo/neo), when fed tamoxifen, express Cre in rod photoreceptors, which removes the neoloxP cassette and induces normal Cngb1 expression. Thus, tamoxifen administration cures these mice of RP, mimicking gene therapy. This system allows us to monitor changes in bipolar cell gene expression as a function of rod photoreceptor death and following a treatment that cures these animals of RP.
We have collected samples of bipolar cell RNA from several cohorts of animals. Samples from animals with rod degeneration and will be collected at P30, P90 and P210. Similarly, we have collected samples from control animals without degeneration at the same ages. Finally, we have collected samples from animals treated at P30 and P90 with tamoxifen. For these two treatment groups, we have analyzed two timepoints post treatment: 1 month and 3 months. This allows us to assay how bipolar cell gene expression changes immediately following a cure for RP and at a much later time point. We have perform RNAseq using the Illumina HiSeq2500 at the Duke Sequencing and Genomic Technologies Core Facility. Collected samples have been pre-processed using Trimmomatic, STAR aligner, and featurecounts to obtain a read count table from all samples.
2.2.1 Model Specifications
We will used DESEQ2 to model gene expression in this experiment. We normalized raw counts across samples by using the TPM method. This technique normalizes by gene length first, then by the sequencing depth. Then, dispersion is measured for each gene using maximum likelihood estimation. Gene-specific dispersion values are shrunk to the expected dispersion value (see Fig 1).
DESEQ2 uses a generalized linear model of the form: \[\begin{equation} K_{ij} ~ NB(\mu_{ij}, \alpha_{i}) \\ \mu_{ij} = s_{j}q_{ij} \\ log_2(q_{ij}) = x_{j} \beta_i \tag{2.1} \end{equation}\]
where counts \(K_{ij}\) for gene i, sample j are modeled using a negative binomial distribution with fitted mean \(\mu_{ij}\) and a gene-specific dispersion parameter \(\alpha_{i}\). The fitted mean is composed of a sample-specific size factor \(s_{j}\) and a parameter \(q_{ij}\) proportional to the expected true concentration of fragments for sample j. The coefficients \(\beta_{i}\) give the log2 fold changes for gene i.
Instead of using continuous covariates, we binned them into factors. This simplification averages the effects of each bin such as treatment length and treatment time. In addition, we also have few time points so a continuous covariate did not make much sense.
2.2.1.1 Regularization
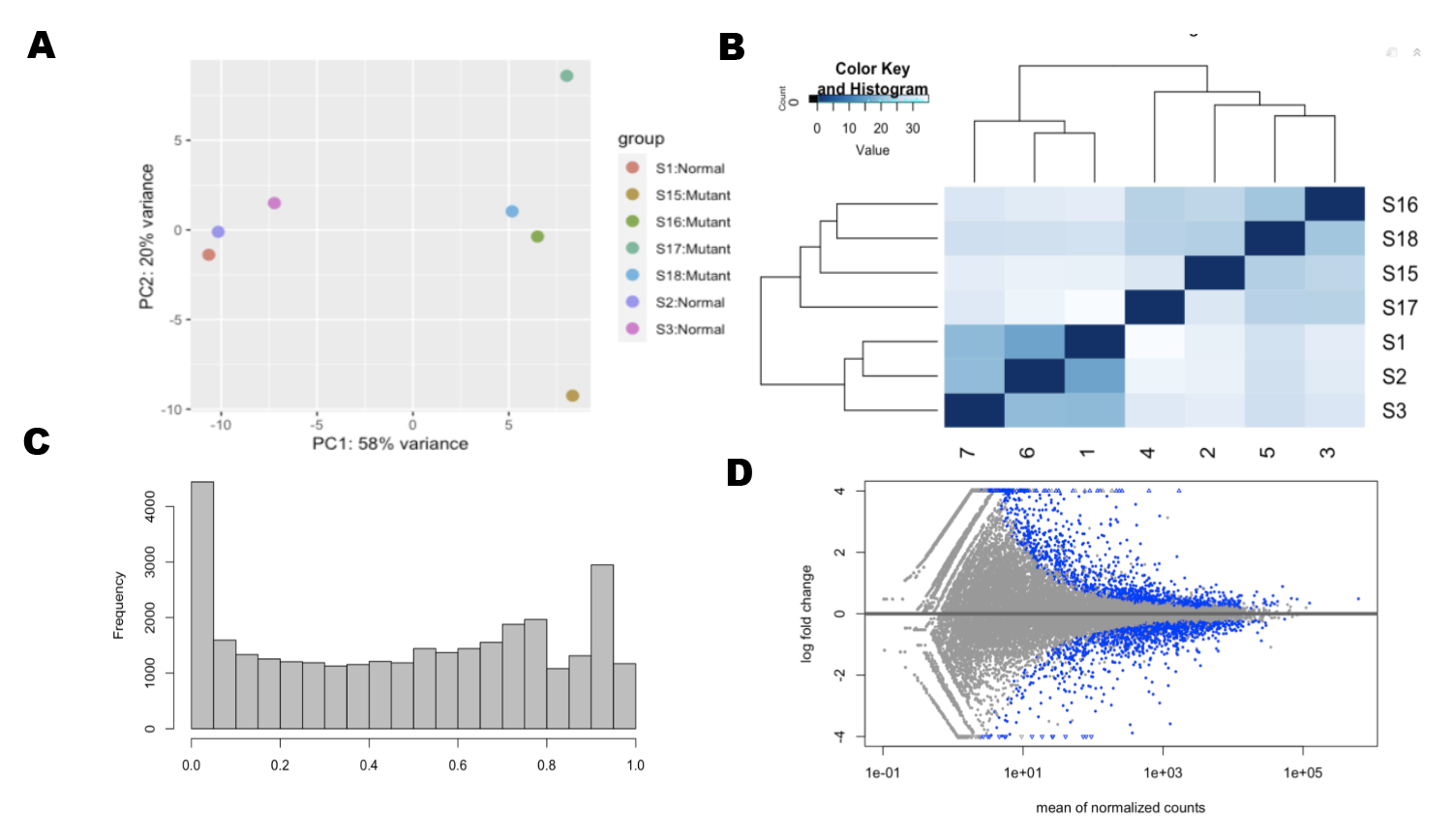
Figure 2.2: Data Exploration (A) Principle Components Analysis (B)Euclidian Distance Analysis (C)P-Value distribution is skewed to 1 (D) Differential expressed genes
Prior to a principle components analysis, we removed the dependence of the variance to the mean. For this step, we used a regularized log transformation (rlog) to transform the original count data to the log2 scale. We fitted a model with a term for each sample and a prior distribution on the coefficients which is estimated from the data.
\[\begin{equation} log_{2}(q_{ij}) = \beta_{i0} + \beta_{ij} \tag{2.2} \end{equation}\]
Here \(q_{ij}\) is a parameter proportional to the expected true concentration of fragments for gene i and sample j. \(\beta_{i0}\) is an intercept which does not undergo shrinkage, \(\beta_{ij}\) is the sample-specific effect which is shrunk toward zero based on the dispersion-mean trend over the entire dataset. The trend typically captures high dispersions for low counts, and therefore these genes exhibit higher shrinkage from the rlog.
We then ran a principle components analysis of our samples in each test we ran. In addition, we looked at euclidian distances between samples. These exploration techniques help assess similarities between samples and determine any outliers.
2.2.1.2 Adjusted P Values
To be more conservative, we use p values adjusted for the false discovery rate. This method allows us to limit the number of genes we falsely identify as differentially expressed. We set this value to 10 percent.
2.3 Results
2.3.1 Differential Genes Induced by Degeneration, Treatment, Treatment Time
We used RNAseq to investigate the possible gene expression change in bipolar cells induced by retinal degeneration. We used principle components analysis and euclidian distances to determine that there were sufficient similarities between samples in each group and that there were also a significant number of differentially expressed genes (see Fig 1 A-B). After applying p-value and false discovery rate thresholds, we found 1500 differentially expressed genes. By running ontology analysis of these genes, we found many were related to retina and sensory system development.
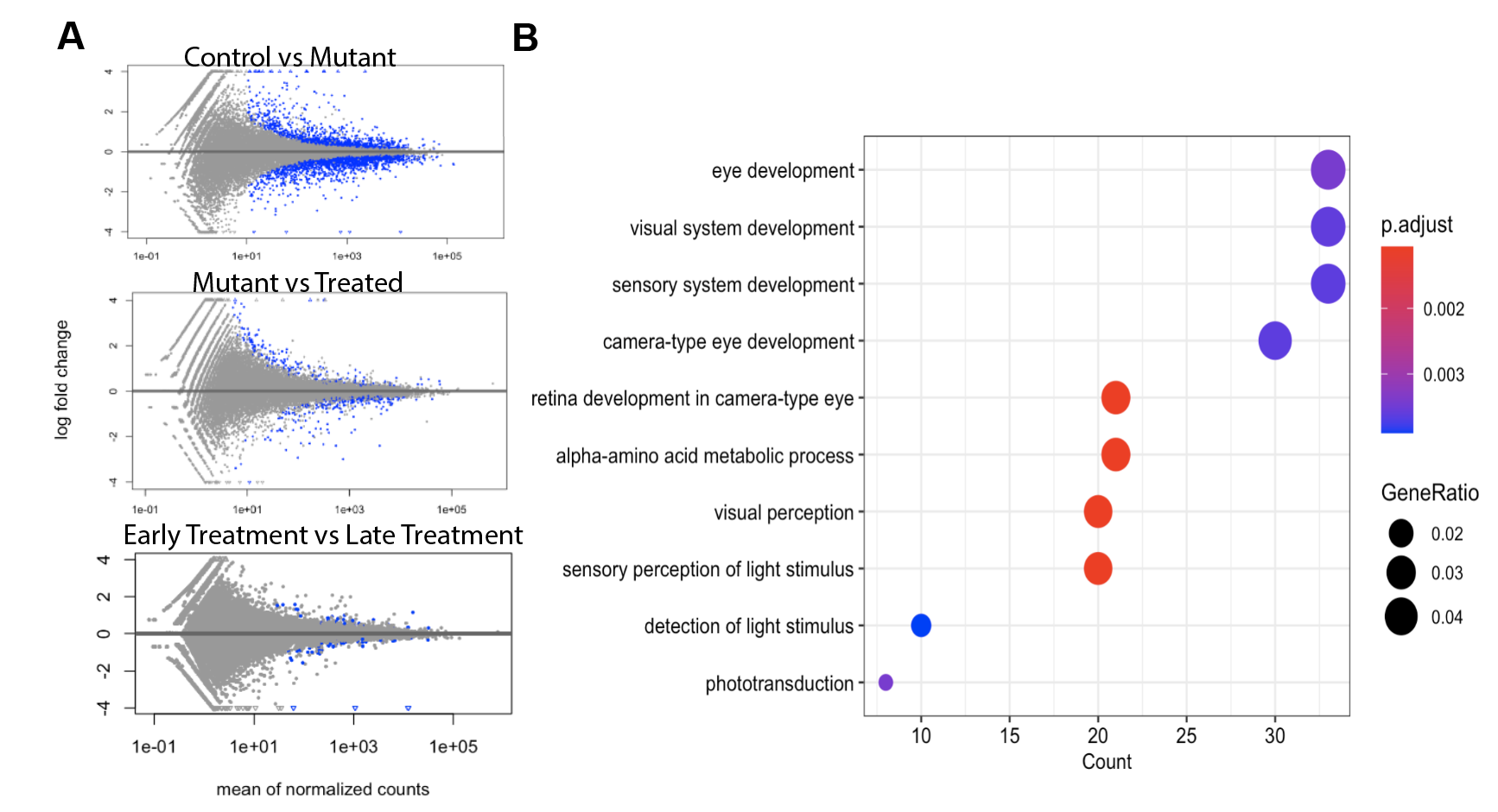
Figure 2.3: (A) Differential expressed genes (B) Ontology of differentially expressed genes from control vs mutant comparison
In evaluating genetic rescue vs non rescued retinas, we found that only 150 genes were differentially expressed in this comparison. In evaluating an early rescue vs late rescue, we found that there were few genes that were differentially expressed. In the rescue-unrescued comparison and the diseased-control comparison, we found 66 genes were coexpressed. 5 genes were isolated related to axonal guidance and dendritic connections.
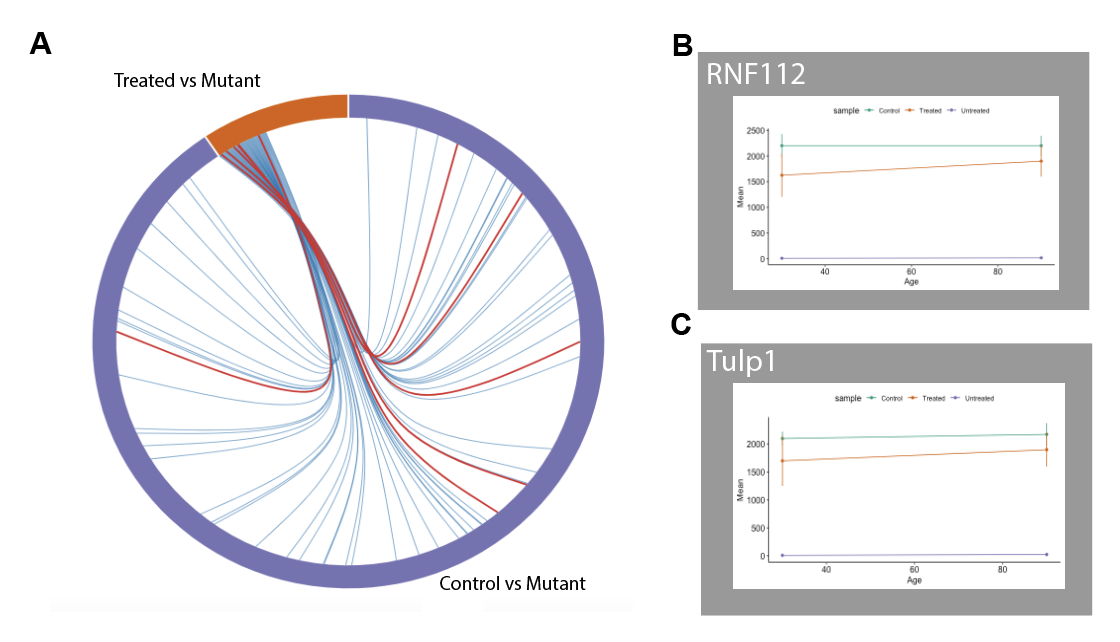
Figure 2.4: (A) Shared genes between two comparisons. Red lines indicate retina related genes. (B) Gene expression of post-synaptic regulating gene (C) Gene expression of pre-synaptic regulating gene
Rnf112 and Tulp1 were two relevant genes that we found were regulated during RP. Rnf112 is related to the post-synaptic contact in dendrites and Tulp1 is involved in presynaptic contact. Both of these genes were downregulated in mutant, but were more highly expressed in the genetic rescue and the controlled.
2.4 Discussion
Patients with RP have circuitry changes in the inner and outer retina (Cuenca et al. 2004; Jones et al. 2003; Lu et al. 2013). Because bipolar cells are intermediate cells they contain a crucial role in signal transduction and eventually the loss of vision. In this study, we conducted RNAseq and related bioinformatic analysis to comprehensively understand potential genes and pathways that lead to vision loss during RP. In addition, we isolated genes that may be involved in the recovery of the retinal circuitry.
Our results indicated that RP induced abnormal gene expression mainly related to eye development, visual system development, and sensory perception of light. We were also able to find potential genetic targets during RP in particular Rnf112 and Tulp1. These genes controlled synaptic connections of the bipolar cells during degeneration and recovery.
Future steps include verification and genetic treatment testing. To confirm that these genes are involved during retinal degeneration, researchers should run quantitative real time PCR. Eventually, to develop a genetic treatment, one could use CRISPR to test the viability of these genetic treatment options. For treatments, further studies should also try to determine specifically which time points these treatments are effective and when damage is unrepairable.
References
Boye, S. E., S. L. Boye, A. S. Lewin, and W. W. Hauswirth. 2013. “A Comprehensive Review of Retinal Gene Therapy.” Journal Article. Mol Ther 21 (3): 509–19. https://doi.org/10.1038/mt.2012.280.
Cuenca, N., I. Pinilla, Y. Sauve, B. Lu, S. Wang, and R. D. Lund. 2004. “Regressive and Reactive Changes in the Connectivity Patterns of Rod and Cone Pathways of P23h Transgenic Rat Retina.” Journal Article. Neuroscience 127 (2): 301–17. https://doi.org/10.1016/j.neuroscience.2004.04.042.
Day, T. P., L. C. Byrne, D. V. Schaffer, and J. G. Flannery. 2014. “Advances in Aav Vector Development for Gene Therapy in the Retina.” Journal Article. Adv Exp Med Biol 801: 687–93. https://doi.org/10.1007/978-1-4614-3209-8_86.
Garg, A., J. Yang, W. Lee, and S. H. Tsang. 2017. “Stem Cell Therapies in Retinal Disorders.” Journal Article. Cells 6 (1). https://doi.org/10.3390/cells6010004.
Jones, B. W., C. B. Watt, J. M. Frederick, W. Baehr, C. K. Chen, E. M. Levine, A. H. Milam, M. M. Lavail, and R. E. Marc. 2003. “Retinal Remodeling Triggered by Photoreceptor Degenerations.” Journal Article. J Comp Neurol 464 (1): 1–16. https://doi.org/10.1002/cne.10703.
Klassen, H. 2016. “Stem Cells in Clinical Trials for Treatment of Retinal Degeneration.” Journal Article. Expert Opin Biol Ther 16 (1): 7–14. https://doi.org/10.1517/14712598.2016.1093110.
Lu, B., C. W. Morgans, S. Girman, R. Lund, and S. Wang. 2013. “Retinal Morphological and Functional Changes in an Animal Model of Retinitis Pigmentosa.” Journal Article. Vis Neurosci 30 (3): 77–89. https://doi.org/10.1017/S0952523813000011.
Luo, J., P. G. McQueen, B. Shi, C. H. Lee, and C. Y. Ting. 2016. “Wiring Dendrites in Layers and Columns.” Journal Article. J Neurogenet 30 (2): 69–79. https://doi.org/10.3109/01677063.2016.1173038.
Marc, R. E., and B. W. Jones. 2003. “Retinal Remodeling in Inherited Photoreceptor Degenerations.” Journal Article. Mol Neurobiol 28 (2): 139–47. https://doi.org/10.1385/MN:28:2:139.
Marc, R. E., B. W. Jones, C. B. Watt, and E. Strettoi. 2003. “Neural Remodeling in Retinal Degeneration.” Journal Article. Prog Retin Eye Res 22 (5): 607–55. https://doi.org/10.1016/s1350-9462(03)00039-9.
Margeta, M. A., and K. Shen. 2010. “Molecular Mechanisms of Synaptic Specificity.” Journal Article. Mol Cell Neurosci 43 (3): 261–7. https://doi.org/10.1016/j.mcn.2009.11.009.
Riccomagno, M. M., and A. L. Kolodkin. 2015. “Sculpting Neural Circuits by Axon and Dendrite Pruning.” Journal Article. Annu Rev Cell Dev Biol 31: 779–805. https://doi.org/10.1146/annurev-cellbio-100913-013038.
Sanes, J. R., and M. Yamagata. 2009. “Many Paths to Synaptic Specificity.” Journal Article. Annu Rev Cell Dev Biol 25: 161–95. https://doi.org/10.1146/annurev.cellbio.24.110707.175402.
Scholl, H. P., R. W. Strauss, M. S. Singh, D. Dalkara, B. Roska, S. Picaud, and J. A. Sahel. 2016. “Emerging Therapies for Inherited Retinal Degeneration.” Journal Article. Sci Transl Med 8 (368): 368rv6. https://doi.org/10.1126/scitranslmed.aaf2838.
Wit, J. de, and A. Ghosh. 2016. “Specification of Synaptic Connectivity by Cell Surface Interactions.” Journal Article. Nat Rev Neurosci 17 (1): 22–35. https://doi.org/10.1038/nrn.2015.3.
Zhang, C., A. L. Kolodkin, R. O. Wong, and R. E. James. 2017. “Establishing Wiring Specificity in Visual System Circuits: From the Retina to the Brain.” Journal Article. Annu Rev Neurosci 40: 395–424. https://doi.org/10.1146/annurev-neuro-072116-031607.