Chapter 3 The Impact of Land Use
3.1 Influencing Direction
Before discussing the impact of land use on travel, the first question is, does land use characteristics is the treatment affecting the outcome of travel behavior? Or the affecting direction is opposite? Technically, randomized experiments can identify the causal relationship between them. But in real life, it is impossible to setup some experimental areas and randomly assign people to live in these areas. Another way is to observe the dynamic of these factors to figure out the direction of influences.
Muller (2004) reviews the evolution of the U.S. urban form and describe the four eras of intrametropolitan growth (Figure ) in U.S. history: the walking-horsecar era (1800s – 1890s), the electric streetcar or transit era (1890s – 1920s), the recreational automobile era (1930s – 1950s), and the freeway era (1950s – 2010s) (Rodrigue, Comtois, and Slack 2016). Each of the four-stage urban transportation development has its dominated spatial structure, which is hard represented by other socio-economic concepts. Each era has the distinctive travel mode, range of distance, and land-use patterns. People can see that the innovation of transportation technology is a determining constraint to other factors and a main driving force to launch the next era.
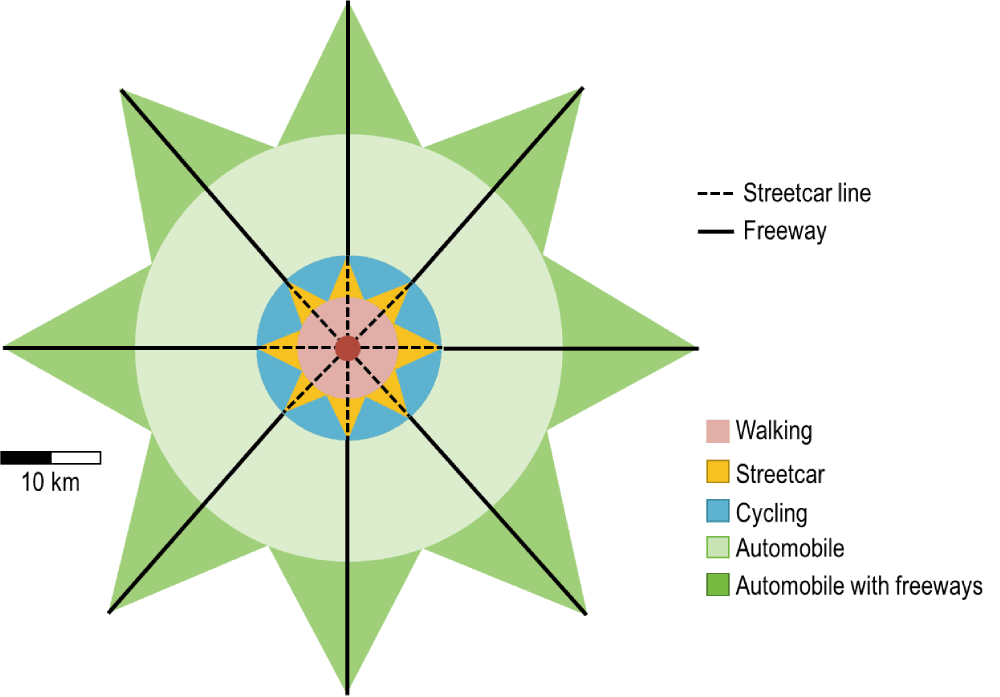
Figure 3.1: One Hour Commuting According to Different Urban Transportation Modes. Source: P.Hugill (1995), World Trade since 1431, p. 213.
New transportation tools shifted people’s travel modes, extended travel distance, and reshaped the urban form. In causal inference, the new tools is called confounder or common cause who affect both treatment and outcome. But what is the relationship between travel and land use? A simple way is to observe the sequence fo events to happen. In each transition period, the new tools and new modes began ahead of the new urban development. Even today multiple travel modes exist in old town but suburban seldom has old mode like streetcar. Thus, land use is more like a outcome rather than treatment.
However, the urban form remains relatively more stable than individual’s travel behavior in each era. A family may be used to driving in Texas and turn to use the subway when they move to New York and vice versa. Given a period, VMT could be affected by density and other factors. It doesn’t make sense that VMT will change the density in the short term. Therefore, land use can be treated as indepedent variables in the context of the current stage of urban development. The relationship of land use with respect to travel can hold for a period of time.
Over the long term, the relationships among travel, land use, and other physical, socioeconomic, demographic factors were interactive and iterative. D. M. Levinson and Krizek (2018) emphasize transportation is a necessary but not a sufficient factor for any development. The change of eras is a comprehensive outcome of socioeconomic and technological development. Which factor caused which effect does not have a simple answer. A conservative view is that land use and travel behavior are determined simultaneously by the transportation costs (Pickrell 1999).
Although randomized experiments about travel and land use are impossible, once the simultaneous relationship holds, the regression model still can explore the associations between travel and land use.
3.2 Influencing Factors
A large mount of studies try to identify the influencing factors and evaluate the effect size. Previous research demonstrated that there is not a single factor determining travel behavior. When choosing continues response, like VMT, the basic regression model is:
\[\begin{aligned} \mathbf{Y}=\mathbf{X}\boldsymbol{\beta}+\boldsymbol{\varepsilon} \end{aligned}\]
where \(\mathbf{Y}\) are the variable of VMT. \(\mathbf{X}\) are all relevant covariates with corresponding coefficients \(\boldsymbol{\beta}\). \(\boldsymbol{\varepsilon}\) are a random error terms with expected value of \(0\) and variance \(\sigma^2\).
When the response is categorical variable, such as binary data of driving versus not, polytomous data of mode choice, or count data of trip frequency, the proper model is:
\[\begin{aligned} E[\mathbf{Y}|\mathbf{X}]=\mu=g^{-1}(\mathbf{X}\boldsymbol{\beta}) \end{aligned}\]
Where \(E[\mathbf{Y}|\mathbf{X}]\) is the expected value of individual/household-level travel variable \(\mathbf{Y}\) conditional on \(\mathbf{X}\); \(\mathbf{X}\boldsymbol{\beta}\) is a linear combination (Ditto.); \(g(\cdot)\) is a link function.
For the complexity of travel behavior, many other objective or subjective factors could change people’s travel decisions. They include but are not limited to physical, socio-demographic, individual, and policy determinants. It seems impossible to make an exhaustive list.
A dichotomy of individual versus environmental factors is a most common framework. All relevant factors involving personal or household characteristics is treated as internal factors. While the built-environment and other environmental factors have external influences. Disaggregate analysis usually chooses this structure because the models can distinguish the different sources of variation from individual and environmental factors. Take for example VMT models, the model is as follow.
\[\begin{aligned} \mathbf{Y}=\mathbf{X}_\mathrm{I}\boldsymbol{\beta}_\mathrm{I}+\mathbf{X}_\mathrm{E}\boldsymbol{\beta}_\mathrm{E}+\boldsymbol{\varepsilon} \end{aligned}\]
where \(\mathbf{X}_\mathrm{I}\) are travelers’ internal characteristics; \(\mathbf{X}_\mathrm{E}\) are built environment and other environment covariates.
Previous research have identified many internal factors such as employment status, income level, lifecycle stage, habits, and preferences, having strong impact on travel. Therefore, a sufficient model should contain these influencing factors. One purpose is to increase the explanatory power. Another purpose is to control self-selection and other independent variables.
Although the internal factors, psychological, socio-demographic, and economic characteristics contribute the primary variation in these models, urban studies’ attentions are on built-environment factors such as urban density, design, and transit services. Because these factors can be intervened by policies and planning. This part of discussion will be placed in the next section separately.
3.2.1 Individual Factors
Here briefly introduces several significant influencing factors.
Income levels
Vehicle ownership
Working status
Major life events/lifecycle
Some major life events, such as transitions to higher school, labor market, marriage, parenthood, retirement, and relocation, could provide windows of opportunity for travel behavior change (Larouche et al. 2020). The results of a scoping review show that relocation prompts people to reflect on their travel behaviors. Entry into the labor market often increases driving while retirement may have more walking or cycling for fewer time constraints. The effects of school transition may have different directions due to the environmental differences. Marriage is not significant because couples may live together before marriage. In a similar way, parents may have more car use several years after childbirth for the purposes of childcare, school, recreation, etc.
Psychological factors
Attitude
Habit
3.2.2 Environmental Factors
- Natural Environment
Physical Environment and other factors
- Infrastructure supplement
Infrastructure supplement is also a set of strong explanatory variables. It has been proven that road capacity and parking space
- policy interventions
(e.g., restrictions on car use) Travel Demand Management (TDM): The levels of land use policy tools. “largely remain under a local government’s authority,” “This imbalanced institutional setting and fragmented government system”
Urban Development Policies (UBG,TOD, Zoning)
There are many complex unknown interaction effects between individual and environmental variables. Some research found that the habit discontinuity hypothesis, major life events may provide windows of opportunity during which individuals may reconsider their travel behaviors and be more sensitive to behavior change interventions (Verplanken et al. 2008). Similarly, ‘changes in built environment attributes may be particularly salient for behaviour change following relocation.’
3.3 Built-Environment Variables
At the macro scale, the concepts of ‘land use’ and ‘urban form’ are often exchangeable, The same thing is about ‘land use’ and ‘built environment’ at the meso and micro scales.
“Attributes of the built environment influence travel by making travel to opportunities more or less convenient and attractive” (Domencich and McFadden 1975; D. M. Levinson, Marshall, and Axhausen 2017; Litman 2017).
The research purpose of travel behavior is not only to describe travel itself, but also to find some tools to affect people’s behavior. Land Use is the primary focus of attention because policy or planning might be able to intervene current and future land use, further achieve the goal of behavior change.
3.3.1 Density
Density is the first built-environment factor added to the model. Early research of automobile trips and urban density can go back sixty years (Mitchell and Rapkin 1954). H. S. Levinson and Wynn (1963) suggest that the people who lived in high-density neighborhoods make fewer automobile trips. This argument stimulated an enormous volume of work. Although later studies construct more complex models, density factor stays in most of built environment-travel model even today.
The most influential aggregate studies start from Newman and Kenworthy. They published a series of studies to show a strong negative correlation between per capita fuel use and gross population density (GPD).9 Their sample covers from thirty-two to fifty-eight global cities (P. G. Newman and Kenworthy 1989a; P. Newman and Kenworthy 2015) and produced very convincing results. Their research points out the relationship rather than estimating the effect size. In this way, the denser cities have less fuel consumption, which implies less automobile dependence. This is a succinct argument and is widely accepted by planners and policy makers.
The criticisms include their ideological grounds, dataset, and model specification10. A criticism is that, for aggregated data, the population variable on both sides of the equation artificially creates a hyperbolic function (Equation (3.1)). In many disaggregated studies, the effects of density are not significant and have a small magnitude.
\[\begin{equation} \begin{split} & \text{VMT}_{average} =\beta\cdot \text{Density} +\cdots\\ \implies & \frac{\text{VMT}_{total}}{\text{Population}} = \beta\cdot \frac{\text{Population}}{\text{Area}} +\cdots\\ \implies & \text{VMT}_{total} = \beta\cdot \frac{(\text{Population})^2}{\text{Area}} +\cdots \end{split} \tag{3.1} \end{equation}\]Another criticism argues that the global comparisons are not valid, such as comparing Hong Kong and Houston. Reid Ewing et al. (2018) created a subset with only US Metropolitan areas from Jeffrey R. Kenworthy and Laube (1999) ‘s original data set. They fit the same model but get a much lower \(R^2\) (0.096) than Kenworthy and Laube’s (0.72). A similar work by Fanis (2019) shows the low \(R^2\) for US cities (0.1838) and European cities (0.2804) when deconstructing Newman and Kenworthy’s data by continent. Actually, any research question has its corresponding sampling design. Choosing a cutoff from the whole data often gets a different result. These criticisms are unfair for Kenworthy and Laube’s work. But it is true that the US cities have higher VMT and lower density than other countries’ cities. Recent evidence over a 20-year period from other cities and counties show that the status quo of the U.S. only represents a small window of the global trend(Jeffrey R. Kenworthy 2017).
Density variable have some advantages. Density is calculated by population size and area size from census data, which are widely available over the country. In contrast, some individual variables are not as measurable and accurate as density. Some studies found density might be an intermediate variable or proxy to other land use variables such as land use mix, street network, and transit services (Reid Ewing and Cervero 2010; Handy 2005). The divisions of the statistical units in the U.S. are from an uniform criteria at multiple scales. Thus, density values is more objective, and comparable comparing other measurements.
Density is an informative factor. Except for the mean and variance, The moments function for Urban density such as skewness, kurtosis, or rank, all can be the predictor candidates. Scolar also explore more delicate measurements of density such as Propotional Weighted Density to replace overall density. “population-weighted density is equal to conventional density plus the variance of density across the subareas used for its calculation divided by the conventional density” (Ottensmann 2018). Some studies use a geographically weighted regression (GWR)11 procedure to identify significant employment density peaks.
Urban density can be represented by many approximate variables – built-up density, residential density, employment density, destination density, or CBD density. Here this paper focus on population density – how many people live in a square mile of land - and involves others if possible.
3.3.2 D-variables
One trenchant criticism of Newman and Kenworthy’s work is that the univariate or bivariate models may leave some critical factors out. In a recent debate (Fanis 2019), Newman clarified that “All our work shows that there are multiple causes of car dependence and multiple implications.” Since travel behavior is a multi-dimensional issue, more socio-demographic and built environment variables were added to the multivariate analysis. The work started from adding three ‘Ds’ variables, density, diversity, and design (Cervero and Kockelman 1997), extended to five ‘Ds,’ adding destination accessibility and distance to transit (Reid Ewing and Cervero 2001). It even grows to seven with the addition of demand management and demographics (TODO).
Ds’ Framework is a significance-centered mixed factor sets and becomes one of the most influential ideas among the built environment–travel literature in the past decades. Using household-level or person-level data, these studies tried to disclose more complex relationships among the candidate factors by adding more relevant variables.
The criticism from Handy (2018) says the 5Ds variables may not be independent. A meta-analysis found that spatial multicollinearity is widespread in this field (Gim 2013). Although previous studies usually check multicollinearity issues, these variables still correlate with each other in some ways and may have strong interaction effects.
3.3.3 Synthesized Index
To address the multicollinearity and interactions issues, Clifton (2017) suggest to convert the various environmental characteristics to built environment indices. Some research try to use ‘compactness indices’ to replace the single density measurement.12 The primary method is principal components analysis (PCA) or principal components regression (PCR), which synthesizes many variables to four dimensions: development density, land use mix, activity centering, and street connectivity. The advantage of this method is to increase the elasticity value significantly. A recent study shows that the elasticity of VMT with respect to a county compactness index is -0.78. The disadvantage of this method is that the internal mechanisms of the indices are still not clear. Another disadvantage is that the various indices are not comparable. For example, the Urban Liveability Index (ULI) by Higgs et al. (2019) includes many indicators supporting health and wellbeing. The association between ULI and travel mode choice is a specific result unless it is widely applied on other studies and cities.
A smart application of synthesize method (common factor analysis) is to control the physiological effects in models. Hong, Shen, and Zhang (2014) convert eight attitudinal questions in the 2006 Household Activity Survey to three factors: Ease, Convenience, and Pro-transit. They fit the model using two geographic scales: 1-km buffer and traffic analysis zone (TAZ). After contolling the attitudinal effects, the nonresidential density and distance from CBD have significant effects on VMT at the TAZ level.
3.3.4 Meta-Analaysis
To get a general, comparable outcome, Reid Ewing and Cervero (2010) collect more than 200 related studies and try to summarize the elasticity values using meta-analysis. Elasticity measures the percentage change in response with respect to a 1 percent increase in a predictor. Thus, it is a dimensionless parameter. After screening, sixty-two studies were selected. But divided by modes (VMT, walking, and transit use), only nine studies were used to calculate the weighted-average elasticities of VMT with respect to population density. Of nine papers, six gave zero or insignificant elasticity, while three showed significant negative values (two are -0.04 and one is -0.12). The weighted-average elasticity of job density (sample size = 9) was zero. The largest elasticity of VMT belonged to Destination accessibility, -0.20 with respect to Job accessibility by auto (sample size = 5) and -0.22 with respect to Distance to downtown (sample size = 3). Due to the small sample size, the confidence intervals were not available. The authors call for more relevant studies to refine the results.
Stevens (2017a) also try to explain the different outcomes using a meta-regression method. Based on the results from 37 studies, they found the magnitude of effects is small and suggest that compacting development has a tiny influence on driving. His work triggers a new round of discussion (Handy 2017; Knaap, Avin, and Fang 2017; Nelson 2017; Manville 2017; Clifton 2017)
In Reid Ewing and Cervero (2017) ‘s reply, they agreed with the values of elasticity but emphasize that Stevens’ results (-0.15 ~ -0.22) are not small actually. In a forthcoming study by Ewing and Cervero, an update elasticity of VMT per capita with respect to density is -0.15 (Reid Ewing et al. 2018). In Stevens (2017b) ’s response to commentaries , he clarified the research goals and important questions.
After the two milestones for meta-analysis of built environment and travel behavior, a recent update re-examines the post-2010 empirical literature and summarized the most common factors (Aston et al. 2021). They are Density, Urban design, Local land use mix, mono/polycentrism and centrality, and Regional jobs-housing balance
3.4 Discussion
References
P. G. Newman and Kenworthy (1989a);P. G. Newman and Kenworthy (1989b);J. R. Kenworthy et al. (1999);P. Newman et al. (2006);P. Newman and Kenworthy (2011a);P. Newman and Kenworthy (2011b);P. Newman (2014);P. Newman and Kenworthy (2015);Jeffrey R. Kenworthy (2017)↩︎
Gordon and Richardson (1989);Dujardin et al. (2012);Perumal and Timmons (2017)↩︎
“Geographically Weighted Regression - an Overview ScienceDirect Topics” (n.d.)↩︎
Reid Ewing, Meakins, et al. (2014);Hamidi and Ewing (2014);Hamidi et al. (2015);Reid Ewing, Hamidi, and Grace (2016)↩︎