Chapter 3 Literature Review
In this review of the literature, I define work with data as a key practice, or learning-related activity, across STEM domains. I also define and justify a multi-dimensional framework for understanding engagement, and then review an approach to analyzing data that is ideal for capturing this multidimensionality.
3.1 Defining Work with Data
Some scholars have focused on a few key pieces of data analysis, connected through the use of “data to solve real problems and to answer authentic questions” (Hancock et al., 1992, p. 337). This focus on solving real problems or answering authentic questions-rather than being taught and learned as isolated skills-is an essential part of work with data having the most educational benefits to learners (National Research Council, 2012; see Lehrer and Schauble [2012] for some examples of work with data being used in classroom settings). This approach has primarily been used by mathematics educators, as reflected in its role in statistics curriculum standards (Franklin et al., 2007). In science settings, where answering questions about phenomena serve as the focus of activities, it shares features of the process of engaging in scientific and engineering practices but has been less often studied.
Work with data has been conceived in different ways (i.e., Hancock et al., 1992; Lehrer & Romberg, 1996; Wild & Pfannkuch, 1999). For instance, Wild and Pfannkuch (1999) consider the process in terms of identifying a problem, generating a measurement system and sampling plan, collecting and cleaning the data, exploring the data and carrying out planned analyses, and interpreting the findings from the analysis. Such a process is common in STEM content areas and is instantiated in standards for some (especially mathematics) curricula. Franklin et al.’s guidelines focus on the Framework for statistical problem solving: formulating questions, collecting data, analyzing data, and interpreting results (2007). The goals of this framework and its components are similar to Hancock et al.’s (1992) description of data modeling, the process of “using data to solve real problems and to answer authentic questions” (p. 337). Hancock et al. (1992) focus in on two goals, data creation and analysis, arguing that the former (data creation) is under emphasized in classroom contexts. Scholars have subsequently expanded Hancock et al.’s definition of data modeling to include six components: asking questions, generating measures, collecting data, structuring data, visualizing data, and making inferences in light of variability (see Lehrer & Schauble, 2004, for using this conceptualization of data modeling applied to the task of understanding plant growth). The last of these components is crucial across all of the visions of data modeling reviewed here and distinguishes these processes from other aspects of data analysis: Accounting for variability (or uncertainty) is central to solving real-world problems with data and the process of data modeling.
Because there is not an agreed-upon definition of work with data–particularly across subject area domains (i.e., across all of the STEM content areas)–I focus on the core aspects that scholars have most often included in their conceptualizations of work with data. These core components, synthesized from definitions across studies, are better for understanding work with data across STEM content areas–as in the present study–than the components from specific examples, which were developed for use in only one domain. The aspects of work with data that have been articulated in prior studies are distilled into five key aspects for use in this study. They are:
- Asking questions: Generating questions that can be answered with empirical evidence
- Making observations: Watching phenomena and noticing what is happening concerning the phenomena or problem being investigated
- Generating data: The process of figuring out how or why to inscribe an observation as data about phenomena, as well as generating tools for measuring or categorizing
- Data modeling: Activities involving the use of simple statistics, such as the mean and standard deviation, as well as more complicated models, such as linear models and extensions of the linear model
- Interpreting and communicating findings: Activities related to identifying a driving question regarding the phenomena that the question is about
These five synthesized aspects of work with data are not stand-alone practices but are a part of a cycle. This process is a cycle is not only because each aspect follows that before it, but also because the overall process is iterative. For example, interpreting findings leads to new questions and subsequent engagement in work with data. Also, scholars have pointed out some key features of how work with data is carried out that impact their effectiveness as a pedagogical approach. These key features include an emphasis on making sense of real-world phenomena and iterative cycles of engaging in work with data and collaboration and dialogue, through which ideas and findings are critiqued and subject to critique, and revised over time (McNeill & Berland, 2017; Lee & Wilkerson, 2018).
3.2 The role of working with data in STEM learning environments
Working with data can serve as an organizing set of practices for engaging in inquiry in STEM learning settings (Lehrer & Schauble, 2015). Data are both encountered and generated by learners, and so opportunities for learners to work with data provide many opportunities to leverage their curiosity because processes of inquiry can be grounded in phenomena that learners themselves can see and manipulate or phenomena that learners are interested in. Also important, becoming proficient in work with data can provide learners with an in-demand capability in society, owing to the number of occupations, from education to entrepreneurship, that demand or involve taking action based on data (Wilkerson & Fenwick, 2017). Furthermore, becoming proficient in work with data can be personally empowering because of the parts of our lives–from paying energy bills to interpreting news articles–that use data.
Recent educational reform efforts emphasize work with data (i.e., the scientific and engineering practices in the NGSS and the standards for mathematical practice in the Common Core State Standards). However, work with data is uncommon in many classroom settings, even classrooms emphasizing recent science education reform efforts; McNeill & Berland, 2017; Miller, Manz, Russ, Stroupe, & Berland, advance online publication). As a result, learning environments suited to engaging in work with data, but not explicitly designed to support it, may be valuable to study because they may serve as incubators of these rare and challenging learning activities.
Outside-of-school programs, in particular, are a potentially valuable setting to explore engagement in work with data, because of the combined pedagogical and technical expertise of their staff and the open-ended nature of the activities that are possible to carry out during them. Staff or youth activity leaders for these programs include educators and scientists, engineers, and others with the technical experience. Additionally, the programs were designed to involve learners in the types of real-world practices experienced by experts in STEM disciplines. Attendance in such programs is associated with many benefits to learners (Green, Lee, Constance, & Hynes, 2013; see Lauer et al., 2006, for a review). These programs are also a good context for understanding work with data because little research has examined how data are part of the experiences of youth during them.
3.3 What is Known About How Youth Work with Data
There is a good amount of past research on cognitive capabilities as outcomes from working with data. Much of this (laboratory-based) research has focused on how children develop the capability to inductively reason from observations (Gelman & Markman, 1987). Other research has focused on the development of causal, or mechanistic, reasoning, among young children (Gopnik & Sobel, 2000; Gopnik, Sobel, Schulz, & Glymour, 2001), often from a Piagetian, individual-development focused tradition (i.e., Piaget & Inhelder, 1969). A key outcome of engaging in work with data has to do with how learners account for variability (Lehrer, Kim, & Schauble, 2007; Petrosino et al., 2003; Lesh, Middleton, Caylor, & Gupta, 2008; Lee, Angotti, & Tarr, 2010), arguably the main goal of engaging in work with data (Konold & Pollatsek, 2002). From this research, we know that learners can develop the capacity to reason about variability.
Past research has also shown that there are strategies that can support work with data. These include the design of technological tools and the development of curricula. From this research, we know about specific strategies and learning progressions for learners to develop this capability. For example, past research has illustrated the role of measurement in exposing learners in a direct way to sources of variability (Petrosino et al., 2003) or the place of relevant phenomena, such as manufacturing processes, such as the size of metallic bolts, which can help learners to focus on “tracking a process by looking at its output” (Konold & Pollatsek, 2002, p. 282).
Finally, past research has shown that different aspects of work with data pose unique opportunities and challenges. Asking empirical questions requires experience and ample time to ask a question that is both able to be answered with data and which is sustaining and worth investigating (Bielik, 2016; Hasson & Yarden, 2012). Making observations and generating data, such as of the height of the school’s flagpole, requires negotiation not only of what to measure, but how and how many times to measure it (Lehrer, Kim, & Schauble, 2007). Regarding modeling, not only teaching students about models, such as that of the mean, but also asking them to create them, are valuable and practical (Lehrer & Schauble, 2004; Lehrer, Kim, & Jones, 2011), but also time-intensive. Interpreting findings, especially in light of variability through models, and communicating answers to questions, means not only identifying error but understanding its sources, and can be supported through exploring models that deliberately represent the data poorly, but can be instructive for probing the benefits and weaknesses of models (Konold & Pollatsek, 2002; Lee & Hollebrands, 2008; Lehrer, Kim, & Schauble, 2007).
Though very valuable past research that has been carried out, valuable insight into how learners and youth participate in different aspects of work with data through the lens of engagement has not been explored. This work can compliment past research by showing, for instance, by showing how certain strategies of work with data or how enacting aspects of work with data in particular ways engage learners (two foci of past research). Consider the practice of modeling data, commonly described as a-or the-key part of many data analyses (Konold, Finzer, & Kreetong, 2017). When modeling data, learners may use data they generated and structured in a data set on their own or may model already-processed, or use already-plotted, data (McNeill & Berland, 2017). How challenging do students perceive the different enactments of these activities to be and how do learners perceive their competence regarding them? Importantly, how hard are learners working? How much do they feel they are learning? Knowing more about these beliefs, characteristics, and processes could help us to develop informed recommendations for teachers and designers intending to bring about opportunities for learners to engage in work with data in a better-supported way that is sustained over time.
3.4 Engagement in General and in STEM Domains
In this section, the nature of engagement is discussed regarding general features that have been identified across content area domains, conditions that support engagement, and differences between engagement in general and in STEM settings. This is followed by a discussion of two key features of engagement: its dynamic, or context-dependent, characteristics, and its multidimensional nature. Finally, I describe methods for capturing these two features empirically through an approach called the Experience Sampling Method, or ESM, and describe how multidimensional data, collected by ESM, can be analyzed.
Engagement is defined in this study as active involvement, or investment, in activities (Fredricks, Blumenfeld, & Paris, 2004). Explaining how learners are involved in activities and tasks is especially important if we want to know about what aspects of work with data are most engaging (and in what ways), and therefore can serve as examples for others advancing work with data as well as those calling for greater support for engagement. Apart from being focused on involvement, engagement is often thought of as a meta-construct, that is, one that is made up of other constructs (Skinner & Pitzer, 2012; Skinner, Kindermann, & Furrer, 2009). By defining engagement as a meta-construct, scholars characterize it in terms of cognitive, behavioral, and affective dimensions that are distinct yet interrelated (Fredricks, 2016).
We know from past research that the cognitive, behavioral, and affective dimensions of engagement can be distinguished (Wang & Eccles, 2012; Wang & Holcombe, 2010) and that while there are long-standing concerns about the conceptual breadth of engagement (Fredricks et al., 2016), careful justification and thoughtful use of multidimensional engagement constructs and measures is warranted. Engagement is also considered to be changing in response to individual, situation or moment contextual factors, Skinner and Pitzer’s (2012) model of motivational dynamics, highlighting the community, school, classroom, and even learning activity, shows the context-dependent nature of engagement on the basis of the impacts of these factors on learners’ engagement.
Engagement in STEM settings shares characteristics with engagement across disciplines, yet there are some distinct aspects to it (Greene, 2015). While one type of engagement—behavioral—is associated with achievement-related outcomes, many STEM practices call for engagement in service of other outcomes, especially around epistemic and agency-related dimensions (Sinatra et al., 2015,). For example, many scholars have defined scientific and engineering practices as cognitive practices, which involve applying epistemic considerations around sources of evidence and the nature of explanatory processes (see Berland et al. 2016, Stroupe, 2014).
The emphasis on developing new knowledge and capabilities by engaging in STEM practices must be reflected in how the cognitive dimension of engagement is measured. Because of the importance of constructing knowledge to engagement in STEM practices, then, I define cognitive engagement in terms of learning something new or getting better at something. While sometimes defined in terms of extra-curricular involvement or following directions, I define behavioral engagement in this study as working hard on learning-related activities (Fredricks et al., 2004; Singh, Granville, & Dika, 2002). Finally, I define affective engagement as emotional responses to activities, such as being excited, angry, or relaxed (Pekrun & Linnenbrink-Garcia, 2012).
Finally, some critical conditions facilitate engagement. Emergent Motivation Theory (EMT; Csikszentmihalyi, 1990), provides a useful lens for understanding these conditions. From EMT, a critical condition for engagement that can change dynamically, from moment to moment, is how difficult individuals perceive an activity to be, or its perceived challenge. Another critical condition is how good at an activity an individual perceives themselves to be, or their perceived competence. What is most important–and necessary concerning being engaged–is being both challenged by and good at a particular activity.
Past research has supported this conjecture (Csikszentmihalyi, 1990). As one empirical example, Shernoff et al. (2016) demonstrated that the interaction of challenge and competence was associated with positive forms of engagement. These findings suggest that learners’ perceptions of the challenge of the activity, and their perceptions of how skillful they are, are important conditions that co-occur with learners’ engagement. Conceptualizing perceptions of challenge and competence as conditions, rather than factors that influence engagement, is in recognition of their co-occurrence within individuals, in that youth experience engagement and their perceptions of the activity (perceived challenge) and of themselves (perceive competence) together and at the same time. Thus, these two conditions (challenge and competence) are considered together with engagement in this study, as described in the section below on analyzing multidimensional data on engagement.
3.5 Youth characteristics that may affect their engagement
Past research suggests learners or youths’ characteristics, such as their interest in the domain of study, impact their cognitive, behavioral, and affective engagement (Shernoff et al., 2003; Shernoff et al., 2016; Shumow, Schmidt, & Zaleski, 2013). These are both moment-to-moment, context-dependent conditions that support engagement (like those discussed above, perceptions of challenge and competence) as well as youth-specific factors. These factors are at the level of individual differences (i.e., youths’ more stable interest in STEM domains), and may impact engagement, as described in this section.
A factor that can support engagement is how teachers support learning practices (Strati, Schmidt, & Maier, 2017). Particularly concerning work with data, which is demanding not only for learners but also teachers (Lehrer & Schauble, 2015; Wilkerson, Andrews, Shaban, Laina, & Gravel, 2016), sustained support from those leading youth activities is an essential component of learners being able to work with data. Thus, how youth activity leaders plan and enact activities related to work with data can have a large impact on students’ engagement. Furthermore, because of the importance of work with data across STEM domains, carrying out ambitious activities focused on work with data may plausibly have a substantial impact on the extent to which youth engage in summer STEM program settings. Consequently, this study considers work with data through the use of a coding frame that characterizes the extent to which teachers are supporting specific STEM practices in their instruction, including aspects of work with data.
Other factors that impact youths’ engagement are individual characteristics and differences. In recognition of differences among learners in their tendency to engage in different (higher or lower) ways in specific activities based in part on individual differences (Hidi & Renninger, 2006), learners’ interest in STEM before the start of the programs is also considered as a factor that can impact engagement. Knowing about whether and to what extent youths’ interest before participating in summer STEM programs explains their engagement during them is a key question in its own right. It is also important regarding properly understanding the effects of other factors, such as working with data, above and beyond the effect of pre-program interest. In addition to this interest, I also consider the gender and the racial and ethnic group of youth, as past research has indicated these as factors that influence engagement in STEM (Bystydzienski, Eisenhart, & Bruning, 2015; Shernoff & Schmidt, 2008). To include the racial and ethnic group of students, I also include youth being part of an under-represented minority (URM) group. To sum up, youths’ pre-program interest, gender, and URM group membership are considered as individual factors that may impact youths’ engagement.
3.6 Challenges of Measuring Engagement as a Contextually-Dependent and Multidimensional Construct
Because of the way engagement has been thought of as having context-dependent characteristics and being multi-dimensional, it is challenging to use engagement (when conceptualized in such a way) in empirical studies. One methodological approach that has benefits concerning the context-dependent and multidimensional nature of engagement is the ESM. Some scholars have explored or extolled benefits to its use in their recent work (e.g., Strati et al., 2017; Turner & Meyer, 2000; Sinatra et al., 2015). This study employs the Experience Sampling Method (ESM; Hektner, Schmidt, & Csikszentmihalyi, 2007) where learners answer short questions about their experience when signaled. ESM involves asking (usually using a digital tool and occasionally a diary) participants short questions about their experiences. ESM is particularly well-suited to understanding the context-dependent nature of engagement because students answered brief surveys about their experience when they were signaled, minimally interrupting them from the activity they are engaged in and also seeking to collect measures about learners’ experience when signaled (Hektner et al., 2007). The ESM approach is both sensitive to changes in engagement over time, as well as between learners and allows us to understand engagement and how factors impact it in more nuanced and complex ways (Turner & Meyer, 2000). Though time-consuming to carry out, ESM can be a robust measure that leverages the benefits of both observational and self-report measures, allowing for some ecological validity and the use of closed-form questionnaires amenable to quantitative analysis (Csikszentmihalyi & Larson, 1987). Despite the logistic challenge of carrying out ESM in large studies, some scholars have referred to it as the gold standard for understanding individual’s subjective experience (Schwarz, Kahneman, & Xu, 2009).
Research has shown us how the use of ESM can lead to distinct contributions to our understanding of learning and engagement. This work also suggests how ESM can be put to use in the present study. For example, Shernoff, Csikszentmihalyi, Schneider, and Shernoff (2003) examined engagement through the use of measures aligned with flow theory, namely, using measures of concentration, interest, and enjoyment (Csikszentmihalyi, 1997). In a study using the same measures of engagement, Shernoff et al. (2016) used an observational measure of challenge and control (or environmental complexity) and found that it significantly predicted engagement, as well as self-esteem, intrinsic motivation, and academic intensity. Schneider et al. (2016) and Linnansaari et al. (2015) examined features of optimal learning moments or moments in which students report high levels of interest, skill, and challenge, as well as their antecedents and consequences. Similar to ESM in that through its use engagement can be studied in a more context-sensitive, still other scholars have used daily diary studies to examine engagement as a function of autonomy-supportive classroom practices (Patall, Vasquez, Steingut, Trimble, & Pituch, 2015; Patall, Steingut, Vasquez, Trimble, & Freeman, 2017). This past research that used ESM (or daily diary studies) to study engagement has shown that ESM can be used to understand fine-grained differences in learning activities, such as the aspects of work with data that are the focus of this study.
Other research shows us that there are newer approaches to analyzing ESM data that can contribute insights into the context-dependent nature of engagement in a more fine-grained way. For example, Strati et al. (2017) explored the relations between engagement to measures of teacher support, finding associations between instrumental support and engagement and powerfully demonstrating the capacity of ESM to understand some of the context-dependent nature of engagement. Similarly, Poysa et al. (2017) used a similar data analytic approach as Strati et al. (2017), that is, use of crossed effects models for variation within both students and time points, both within and between days. These studies establish the value of the use of ESM to understand the context-dependent nature of engagement and that such an approach may be able to be used to understand engaging in work with data. Additionally, these recent studies (particularly the study by Strati and colleagues) show that how effects at different levels are treated, namely, how variability at these levels is accounted for through random effects as part of mixed effects models, is a key practical consideration for the analysis of ESM data.
One powerful and increasingly widely used way to examine context-dependent constructs, such as engagement, is the use of profiles of, or groups of variables that are measured. This profile approach is especially important given the multidimensional nature of engagement. In past research, profiles are commonly used as part of what is described as person-oriented approaches (Bergman & Magnusson, 1997; Bergman, Magnusson, & El Khouri, 2003), those used to consider the way in which psychological constructs are experienced together and at once in the experiences of learners. Note that in the present study, ESM involves asking youth about to report on their experience at the time they were signaled (rather than, for example, before or after the program, which traditional surveys are well-suited for). In this study, profiles of engagement are used in the service of understanding how students engage in work with data in a more holistic way. There are some recent studies taking a profile approach to the study of engagement (i.e., Salmela-Aro, Moeller, Schneider, Spicer, & Lavonen, 2016a; Salmela-Aro, Muotka, Alho, Hakkarainen, & Lonka, 2016b; Van Rooij, Jansen, & van de Grift, 2017; Schmidt, Rosenberg, & Beymer, 2018), though none have done so to study youths’ engagement in work with data.
The profile approach has an important implication for how we analyze data collected from ESM about youths’ engagement, in particular when we consider how to understand engagement as a multi-dimensional construct, and one with momentary, or instructional episode-specific, conditions (Csikszentmihalyi, 1990). We know from past research that engagement can be explained through different patterns among its components (Bergman & Magnusson, 1997; Bergman et al., 2003), in the present case its cognitive, behavioral, and affective components. Because learners’ engagement includes cognitive, behavioral, and affective aspects experienced together at the same time, it can be experienced as a combined effect that is categorically distinct from the effects of the individual dimensions of engagement. This combined effect can be considered as profiles of engagement.
Past studies have considered profiles of cognitive, behavioral, and affective aspects of engagement. For example, to account for the context-dependent nature of engagement, some past studies have used other measures to predict engagement, such as the use of in-the-moment resources and demands (Salmela-Aro et al., 2016b) and the use of instructional activities and choice (Schmidt et al., 2018). A potential way to extend this past research is to account for not only engagement (cognitive, behavioral, and affective), but also the intricately connected perceptions of challenge and competence. This analytic approach is especially important since a profile approach emphasizes the holistic nature of engagement and the impact of not only external but also intra-individual factors. Accordingly, youths’ perceptions of the challenge of the activity and their competence at it are used along with the measures of engagement to construct profiles of engagement. Thus, the profiles of engagement include youths’ responses to five ESM items for their cognitive, behavioral, and affective engagement and their perceptions of how challenging the activity they were doing is and of how competent at the activity they are.
3.7 Need for the Present Study
While many scholars have argued that work with data can be understood in terms of the capabilities learners develop and the outcome learners achieve, there is a need to understand learners’ experiences working with data. The present study does this through the use of contemporary engagement theory and innovative methodological and analytic approaches. Doing this can help us to understand work with data in terms of learner’s experience, which we know from past research impacts what and how students learn (Sinatra et al., 2015). Knowing more about students’ engagement can help us to design activities and interventions focused around work with data. In addition to this need to study engagement in work with data through the lens of engagement, no research has yet examined work with data in the context of summer STEM programs, though such settings are potentially rich with opportunities for highly engaged youth to analyze authentic data sources.
3.8 Conceptual Framework and Research Questions
To summarize this section, the present study is about how learning activities involving various aspects of work with data can be understood in terms of engagement. Its context is out-of-school-time STEM enrichment programs designed to meet guidelines for best practices. The conceptual framework in the present study is presented in Figure 2.1 and is laid out in the remainder of this section.
There are five aspects of work with data synthesized from past research (i.e., Hancock et al., 1992; Lehrer & Romberg, 1996; Wild & Pfannkuch, 1999):
- Asking questions or identifying problems
- Making observations
- Generating data
- Data modeling
- Interpreting and communicating findings
In Figure 2.3, engagement in work with data is associated with different profiles of engagement. The theoretical framework for the profile approach suggests that engagement is a multi-dimensional construct consisting of cognitive, behavioral, and affective dimensions of engagement and perceptions of challenge and competence. Also, a pre-program measure of youths’ pre-program interest in STEM, along with youths’ gender and URM status, are hypothesized to be associated with the profiles and the relations of work with data and the profiles.
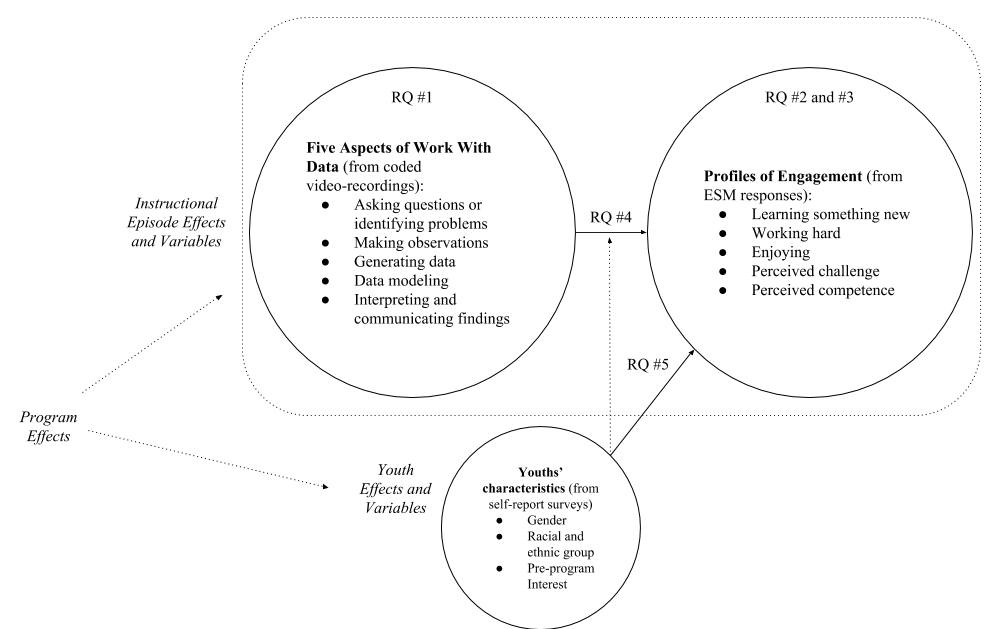
Figure 3.1: A conceptual framework for this study and research questions
Regarding research questions 2-5, the ESM responses that make up the profiles are associated with different “levels.” These levels, or groups, which may introduce dependencies that violate statistical assumptions of the independence of the responses, are commonly considered in the Hierarchical Linear Modeling (also known as multi-level or mixed effects modeling) literature as random effects (Gelman & Hill, 2007; West, Welch, & Galecki, 2015). In this study, three levels that can be modeled as random effects to account for the dependencies they introduce: Youth, instructional episode (which are indicators for the moments–or segments–in which youth are asked to respond to the ESM signal), and the program. Thus, these are not predictor variables, but rather are the levels that are present given the approach to data collection and the sampling procedure. Interpreting their effects is not a goal of this study, but accounting for them in the models used, as in this study, is essential and is done through the use of random effects.
Pre-program interest, gender, and URM status are predictor variables at the youth level. The aspects of work with data are predictor variables at the instructional episode level. There are no predictor variables at the program level, in part due to the small number of programs (and the resulting low statistical power of any variables added at this level). Pre-program interest, gender, and URM status, and the aspects of work with data are used as predictor variables, while the three levels (youth, instructional episode, and program) are accounted for in the mixed effects modeling strategy.
The five research questions, then, are:
- What is the frequency and nature of opportunities for youth to engage in each of the five aspects of work with data in summer STEM programs?
- What profiles of engagement emerge from data collected via ESM in the programs?
- What are sources of variability for the profiles of engagement?
- How do the five aspects of work with data relate to profiles of engagement?
- How do youth characteristics relate to profiles of engagement?